AI Survey Data Processing: Streamline Your Workflow
AI Survey Data Processing: Streamline Your Workflow
AI Survey Data Processing: Streamline Your Workflow



Businesses conduct online surveys to gather valuable insights directly from their target audience which includes customers, employees and competitors. These surveys help in understanding customer preferences, employee satisfaction, market trends, and product feedback, which are crucial for informed decision-making. According to a report by Statista, 71% of businesses use surveys to measure customer satisfaction and loyalty.
But, manually processing survey data is time-consuming and error-prone. AI survey data processing automates survey analysis faster and more accurate insights. It enables businesses to make data-driven decisions efficiently. In this blog, we will explore how AI survey data processing works, its impact on different industries, and real-world use cases.
How AI Survey Data Processing Works
Traditional online surveys, including drag-and-drop form builders, face signifit challenges in survey data processing. Manual data cleaning and analyzing open-ended responses often requires intensive human effort. Adding to it, it's difficult to integrate data from various sources and generate real-time insights.
In contrast, AI survey form builders like Metaforms automate data cleaning, employ natural language processing (NLP) for analyzing open-ended responses, and provide real-time analytics. They seamlessly integrate multiple data sources, enhancing accuracy and efficiency. AI survey form builders offer a more technically advanced solution, streamlining the entire data processing workflow compared to traditional survey models.
AI survey data processing involves several steps that transform raw survey data into actionable insights. Here’s a detailed breakdown of the process:
Step-by-Step Guide to AI Survey Data Processing
AI survey data processing encompasses several stages, each designed to automate and optimize the handling of survey data from collection to actionable insights. Here's a detailed breakdown of the steps involved:
1. Data Collection Automation
Description: AI-driven surveys streamline data collection by automating the process of gathering responses through various channels such as emails, social media, and web embeds.
Multi-Channel Integration: AI integrates with multiple platforms, allowing surveys to be distributed and responses collected across diverse channels.
Real-Time Collection: Responses are collected in real-time, ensuring timely data availability for analysis.
Example: An e-commerce site uses AI to collect feedback from customers via email and pop-up surveys on the website.
2. Data Validation
Description: AI automatically validates the incoming data to ensure accuracy and consistency, reducing errors and the need for manual corrections.
Automatic Error Detection: AI identifies and flags inconsistent or invalid responses (e.g., conflicting answers, incomplete responses).
Real-Time Correction: Errors be corrected in real-time, prompting respondents to amend their inputs before submission.
Example: In a customer feedback survey, AI detects when a respondent skips a required question and prompts them to complete it before submission.
3. Data Cleaning
Description: AI tools clean the data by removing duplicates, handling missing values, and standardizing entries.
Deduplication: Removes repeated responses.
Missing Value Handling: Fills in missing data or highlights gaps for further action.
Standardization: Normalizes data formats for consistent analysis.
Example: A market research firm uses AI to standardize dates and remove duplicate entries from survey responses.
4. Natural Language Processing (NLP) for Open-Ended Responses
Description: AI employs NLP to analyze and categorize open-ended responses, extracting meaningful insights from qualitative data.
Sentiment Analysis: Determines the emotional tone of responses.
Topic Modeling: Identifies common themes and topics in textual responses.
Categorization: Automatically groups similar feedback for easier analysis.
Example: An airline uses NLP to analyze customer feedback, identifying common issues related to flight delays and service quality.
5. Data Enrichment
Description: AI enriches the survey data by integrating it with external data sources or additional internal databases to provide a more comprehensive analysis.
External Integration: Combines survey data with CRM systems, social media, or other databases for enriched insights.
Contextual Enhancement: Adds contextual information that enhances the understanding of responses.
Example: A B2B company enriches survey responses with CRM data to correlate customer satisfaction with purchase history.
6. Real-Time Data Analysis
Description: AI processes and analyzes the data in real-time, providing immediate insights and visualizations.
Immediate Insights: Generates reports and analytics as responses come in, allowing for prompt decision-making.
Automated Dashboards: Creates dynamic dashboards that update automatically with incoming data.
Example: A tech startup uses AI to analyze user feedback from beta testers in real-time, identifying issues and areas for improvement instantly.
7. Predictive Analytics
Description: AI predictive models forecasts trends, behaviors, and outcomes based on the collected survey data.
Trend Forecasting: Predicts future trends from current data.
Behavior Analysis: Anticipates customer behavior patterns and potential actions.
Outcome Prediction: Estimates likely outcomes based on survey responses.\
Example: A telecom company predicts customer churn by analyzing satisfaction survey data and identifying at-risk customers.
8. Data Visualization
Description: AI creates visual representations of the data, such as charts, graphs, and dashboards, making it easier to interpret and communicate findings.
Interactive Visuals: Provides interactive charts and dashboards for deeper exploration.
Customizable Reports: Offers flexible reporting options tailored to different stakeholder needs.
Example: A financial services firm uses AI to generate interactive dashboards that visualize customer satisfaction trends over time.
9. Automated Reporting
Description: AI generates comprehensive reports automatically, summarizing key findings and insights from the survey data.
Summary Reports: Compiles essential metrics and insights into easily digestible formats.
Detailed Analysis: Provides in-depth analysis reports for more granular insights.
Example: An educational institution receives automated reports on student feedback, highlighting areas for curriculum improvement.
10. Actionable Recommendations
Description: AI provides actionable recommendations based on the survey data analysis, guiding businesses on next steps.
Data-Driven Suggestions: Offers practical suggestions derived from data trends.
Strategic Insights: Helps inform strategic decisions with evidence-based recommendations.
Example: A retail brand receives AI-generated recommendations to enhance customer service based on feedback trends showing a need for quicker response times.
Use Case: AI-Powered Survey Data Processing for a Retail Brand
Scenario: A retail brand conducts a customer satisfaction survey to improve its shopping experience.
AI Survey Data Processing Steps Implemented:
1. Data Collection: AI distributes the survey via email and social media, collecting responses in real-time.
2. Data Validation: AI validates responses, ensuring completeness and consistency.
3. Data Cleaning: AI removes duplicate responses and handles missing data.
4. NLP for Open-Ended Responses: AI analyzes open-ended feedback for common themes and sentiments.
5. Data Enrichment: AI enriches survey data with purchase history from the CRM system.
6. Real-Time Analysis: AI processes and analyzes data as it is collected, providing immediate insights.
7. Predictive Analytics: AI predicts future purchasing behavior based on satisfaction levels.
8. Data Visualization: AI generates visual reports and dashboards for easy interpretation.
9. Automated Reporting: AI delivers comprehensive summary and detailed reports.
10. Actionable Recommendations: AI provides recommendations for improving the shopping experience.
Outcome: The retail brand uses the insights and recommendations to enhance customer service, streamline operations, and increase customer satisfaction.
AI Survey Data Processing for Different Industries
AI survey data processing significantly enhances the efficiency and effectiveness of data analysis across various industries. Here are four industries that benefit immensely from AI survey analytics and reports:
1. Retail
Retailers use AI to analyze customer feedback, identify popular products, and detect purchasing trends. AI-driven insights help in inventory management, marketing strategies, and improving customer experience. According to a McKinsey report, retailers that leverage AI increase their operating margins by up to 60% .
Example: A retail company uses AI to analyze customer feedback from an online survey. The AI tool identifies a high demand for sustainable products, prompting the company to expand its eco-friendly product line, resulting in a 25% increase in sales.
2. Healthcare
Healthcare providers use AI to process patient feedback, improve service quality, and enhance patient satisfaction. AI helps predict patient needs and optimize resource allocation. Accenture estimates that AI could save the healthcare industry $150 billion annually by 2026 .
Example: A hospital uses AI to analyze patient satisfaction surveys. The AI identifies common complaints about long wait times and suggests operational changes, leading to a 30% reduction in wait times and improved patient satisfaction.
3. Finance
Financial institutions use AI to analyze customer feedback, detect fraud, and assess risk. AI helps in personalizing services and improving customer satisfaction. According to PwC, AI could contribute up to $15.7 trillion to the global economy by 2030, with the financial sector being a signifit beneficiary .
Example: A bank uses AI to process customer feedback from various channels. The AI identifies recurring issues with a mobile banking app and suggests improvements, leading to a 40% increase in user satisfaction and reduced customer churn.
4. Education
Educational institutions use AI to analyze student feedback, identify areas for improvement, and tailor educational programs. AI enhances student engagement and learning outcomes. A study by the e-learning industry indicates that AI increases student retention rates by 35% .
Example: A university uses AI to analyze course evaluation surveys. The AI identifies specific courses with low satisfaction rates and recommends curriculum adjustments, resulting in improved student satisfaction and retention.
Sample AI Survey Data Analytics Report
Survey Overview
Survey Title: Customer Satisfaction Survey
Number of Respondents: 1,000
Survey Period: January 1, 2023 - January 31, 2023
Key Metrics
Overall Satisfaction Score: 4.2/5
Net Promoter Score (NPS): 45
Customer Effort Score (CES): 2.5/5
Sentiment Analysis
Positive Feedback: 65%
Neutral Feedback: 20%
Negative Feedback: 15%
Common Themes
Positive: Quality of customer service, product reliability, fast shipping
Negative: Website usability, product availability, customer support response time
Predictive Insights
Future Trends: Increased demand for online customer support, preference for sustainable products
Recommendations: Improve website usability, enhance product availability, invest in sustainable product lines
AI Survey Data Processing Best Practices
AI survey data processing leverages artificial intelligence and machine learning to handle the entire data lifecycle—from collection to analysis. To maximize the effectiveness and accuracy of AI-powered survey data processing, businesses should adhere to the following best practices:
1. Ensure Data Quality from the Start
Example: A retail company deploying customer satisfaction surveys should use AI-powered validation to check for incomplete responses or inconsistencies as data is entered, rather than addressing these issues post-collection.
Why It Matters: High-quality data is the foundation of accurate analysis. AI filters out duplicates, handles missing values, and validates responses in real-time, which ensures that the data collected is reliable and usable.
Tip: Implement AI algorithms that detect anomalies and outliers in real-time, allowing immediate correction and re-engagement of respondents if necessary.
2. Implement Adaptive Survey Techniques
Example: A software company conducting user experience surveys use AI to adapt questions based on user responses, ensuring more relevant and personalized feedback.
Why It Matters: Adaptive surveys enhance respondent engagement and data relevance. AI-driven adaptive questioning adjusts the survey flow based on previous answers, which keeps respondents interested and reduces survey fatigue.
Tip: Use AI to create dynamic surveys that modify questions in real-time based on user input, ensuring each respondent's journey through the survey is tailored to their responses.
3. Utilize Advanced Data Cleaning Methods
Example: A healthcare organization surveying patient satisfaction employs AI to automatically clean data by identifying and correcting input errors, handling missing values, and removing irrelevant data points.
Why It Matters: AI enhances data cleaning efficiency by automating the detection and correction of errors, reducing the manual effort and time required. This leads to more accurate datasets for analysis.
Tip: Integrate AI algorithms that handle natural language data to parse and clean open-ended responses, standardize entries, and reconcile inconsistencies.
4. Leverage Natural Language Processing (NLP)
Example: An e-commerce business using surveys to gather customer feedback on new product lines use NLP to analyze sentiments and categorize open-ended responses efficiently.
Why It Matters: NLP enables the processing of textual data at scale, extracting meaningful insights from qualitative feedback. It helps in understanding sentiments, themes, and the context behind responses.
Tip: Incorporate NLP tools to analyze open-ended responses for sentiment, categorize feedback, and identify emerging themes without manual intervention.
5. Enable Real-Time Data Processing
Example: A financial services firm conducting client feedback surveys use AI to analyze responses in real-time and promptly generate actionable insights for immediate decision-making.
Why It Matters: Real-time data processing enables businesses to act swiftly on feedback, enhancing responsiveness and allowing immediate adjustments to strategies or operations based on current data.
Tip: Use AI-driven dashboards that provide live updates on survey responses and key metrics, enabling quick data-driven decisions.
6. Integrate Predictive Analytics
Example: A telecom company uses AI to predict customer churn based on survey responses about service satisfaction, allowing proactive retention efforts.
Why It Matters: Predictive analytics helps anticipate future trends and behaviors based on current data, enabling businesses to implement preventive measures or capitalize on upcoming opportunities.
Tip: Employ AI models that forecast trends from survey data, such as customer satisfaction leading to churn or product feedback predicting future sales trends.
7. Ensure Data Security and Privacy
Example: A legal firm conducting client satisfaction surveys must use AI to anonymize responses and ensure that sensitive data is protected against unauthorized access.
Why It Matters: AI tools enhance data security by automating encryption, anonymization, and compliance checks, safeguarding respondent information and adhering to privacy regulations.
Tip: Utilize AI to continuously monitor data access patterns and enforce security protocols, ensuring compliance with data protection standards like GDPR.
8. Create Comprehensive Visualizations
Example: A marketing agency analyzing campaign effectiveness through client surveys use AI to create detailed visualizations of key metrics, making it easier to interpret and present findings.
Why It Matters: Visual representations of data make insights more accessible and understandable, aiding in effective communication of survey results to stakeholders.
Tip: Leverage AI tools to generate interactive dashboards and visualizations to customize based on the user’s role and data needs.
9. Optimize Survey Design Using AI Insights
Example: A tech startup analyzes survey completion rates and feedback with AI to identify patterns in survey design that affect respondent engagement, and refine future surveys accordingly.
Why It Matters: Continuous improvement of survey design based on AI-derived insights leads to higher response rates and better data quality.
Tip: Use AI analytics to review past survey performance and identify design elements that enhance or hinder respondent engagement.
10. Foster Transparency and Interpretability
Example: A nonprofit organization sharing impact assessment results from surveys should use AI to clearly explain how data is processed and analyzed, ensuring stakeholders understand the results.
Why It Matters: Transparency in AI-driven processes builds trust and ensures that the insights derived from data are understandable and actionable by all stakeholders.
Tip: Provide clear documentation and user-friendly interfaces that explain AI processing steps and the basis of insights generated from survey data.
Conclusion:
AI-powered survey data processing transforms traditional survey methodologies by automating data collection, enhancing data quality, and providing real-time, actionable insights. By adhering to best practices like adaptive survey techniques, advanced data cleaning, and real-time processing, businesses achieve more accurate and efficient survey outcomes. Leveraging AI tools not only improves survey design and data security but also enables predictive analytics and comprehensive visualizations, driving better business decisions and fostering deeper customer understanding. As AI continues to evolve, integrating these best practices ensures that businesses stay ahead in gathering and interpreting valuable customer insights effectively.
AI-powered surveys provide a scalable and cost-effective method to reach a broad audience, facilitating the collection of real-time data. They drive strategic planning, marketing initiatives, and operational improvements. AI survey data processing used in AI-native form builders like Metaforms provides insight survey analytics reports that helps businesses to zero in on finer details about their potential customers and loyal customers. Sign-up with Metaforms today.
Businesses conduct online surveys to gather valuable insights directly from their target audience which includes customers, employees and competitors. These surveys help in understanding customer preferences, employee satisfaction, market trends, and product feedback, which are crucial for informed decision-making. According to a report by Statista, 71% of businesses use surveys to measure customer satisfaction and loyalty.
But, manually processing survey data is time-consuming and error-prone. AI survey data processing automates survey analysis faster and more accurate insights. It enables businesses to make data-driven decisions efficiently. In this blog, we will explore how AI survey data processing works, its impact on different industries, and real-world use cases.
How AI Survey Data Processing Works
Traditional online surveys, including drag-and-drop form builders, face signifit challenges in survey data processing. Manual data cleaning and analyzing open-ended responses often requires intensive human effort. Adding to it, it's difficult to integrate data from various sources and generate real-time insights.
In contrast, AI survey form builders like Metaforms automate data cleaning, employ natural language processing (NLP) for analyzing open-ended responses, and provide real-time analytics. They seamlessly integrate multiple data sources, enhancing accuracy and efficiency. AI survey form builders offer a more technically advanced solution, streamlining the entire data processing workflow compared to traditional survey models.
AI survey data processing involves several steps that transform raw survey data into actionable insights. Here’s a detailed breakdown of the process:
Step-by-Step Guide to AI Survey Data Processing
AI survey data processing encompasses several stages, each designed to automate and optimize the handling of survey data from collection to actionable insights. Here's a detailed breakdown of the steps involved:
1. Data Collection Automation
Description: AI-driven surveys streamline data collection by automating the process of gathering responses through various channels such as emails, social media, and web embeds.
Multi-Channel Integration: AI integrates with multiple platforms, allowing surveys to be distributed and responses collected across diverse channels.
Real-Time Collection: Responses are collected in real-time, ensuring timely data availability for analysis.
Example: An e-commerce site uses AI to collect feedback from customers via email and pop-up surveys on the website.
2. Data Validation
Description: AI automatically validates the incoming data to ensure accuracy and consistency, reducing errors and the need for manual corrections.
Automatic Error Detection: AI identifies and flags inconsistent or invalid responses (e.g., conflicting answers, incomplete responses).
Real-Time Correction: Errors be corrected in real-time, prompting respondents to amend their inputs before submission.
Example: In a customer feedback survey, AI detects when a respondent skips a required question and prompts them to complete it before submission.
3. Data Cleaning
Description: AI tools clean the data by removing duplicates, handling missing values, and standardizing entries.
Deduplication: Removes repeated responses.
Missing Value Handling: Fills in missing data or highlights gaps for further action.
Standardization: Normalizes data formats for consistent analysis.
Example: A market research firm uses AI to standardize dates and remove duplicate entries from survey responses.
4. Natural Language Processing (NLP) for Open-Ended Responses
Description: AI employs NLP to analyze and categorize open-ended responses, extracting meaningful insights from qualitative data.
Sentiment Analysis: Determines the emotional tone of responses.
Topic Modeling: Identifies common themes and topics in textual responses.
Categorization: Automatically groups similar feedback for easier analysis.
Example: An airline uses NLP to analyze customer feedback, identifying common issues related to flight delays and service quality.
5. Data Enrichment
Description: AI enriches the survey data by integrating it with external data sources or additional internal databases to provide a more comprehensive analysis.
External Integration: Combines survey data with CRM systems, social media, or other databases for enriched insights.
Contextual Enhancement: Adds contextual information that enhances the understanding of responses.
Example: A B2B company enriches survey responses with CRM data to correlate customer satisfaction with purchase history.
6. Real-Time Data Analysis
Description: AI processes and analyzes the data in real-time, providing immediate insights and visualizations.
Immediate Insights: Generates reports and analytics as responses come in, allowing for prompt decision-making.
Automated Dashboards: Creates dynamic dashboards that update automatically with incoming data.
Example: A tech startup uses AI to analyze user feedback from beta testers in real-time, identifying issues and areas for improvement instantly.
7. Predictive Analytics
Description: AI predictive models forecasts trends, behaviors, and outcomes based on the collected survey data.
Trend Forecasting: Predicts future trends from current data.
Behavior Analysis: Anticipates customer behavior patterns and potential actions.
Outcome Prediction: Estimates likely outcomes based on survey responses.\
Example: A telecom company predicts customer churn by analyzing satisfaction survey data and identifying at-risk customers.
8. Data Visualization
Description: AI creates visual representations of the data, such as charts, graphs, and dashboards, making it easier to interpret and communicate findings.
Interactive Visuals: Provides interactive charts and dashboards for deeper exploration.
Customizable Reports: Offers flexible reporting options tailored to different stakeholder needs.
Example: A financial services firm uses AI to generate interactive dashboards that visualize customer satisfaction trends over time.
9. Automated Reporting
Description: AI generates comprehensive reports automatically, summarizing key findings and insights from the survey data.
Summary Reports: Compiles essential metrics and insights into easily digestible formats.
Detailed Analysis: Provides in-depth analysis reports for more granular insights.
Example: An educational institution receives automated reports on student feedback, highlighting areas for curriculum improvement.
10. Actionable Recommendations
Description: AI provides actionable recommendations based on the survey data analysis, guiding businesses on next steps.
Data-Driven Suggestions: Offers practical suggestions derived from data trends.
Strategic Insights: Helps inform strategic decisions with evidence-based recommendations.
Example: A retail brand receives AI-generated recommendations to enhance customer service based on feedback trends showing a need for quicker response times.
Use Case: AI-Powered Survey Data Processing for a Retail Brand
Scenario: A retail brand conducts a customer satisfaction survey to improve its shopping experience.
AI Survey Data Processing Steps Implemented:
1. Data Collection: AI distributes the survey via email and social media, collecting responses in real-time.
2. Data Validation: AI validates responses, ensuring completeness and consistency.
3. Data Cleaning: AI removes duplicate responses and handles missing data.
4. NLP for Open-Ended Responses: AI analyzes open-ended feedback for common themes and sentiments.
5. Data Enrichment: AI enriches survey data with purchase history from the CRM system.
6. Real-Time Analysis: AI processes and analyzes data as it is collected, providing immediate insights.
7. Predictive Analytics: AI predicts future purchasing behavior based on satisfaction levels.
8. Data Visualization: AI generates visual reports and dashboards for easy interpretation.
9. Automated Reporting: AI delivers comprehensive summary and detailed reports.
10. Actionable Recommendations: AI provides recommendations for improving the shopping experience.
Outcome: The retail brand uses the insights and recommendations to enhance customer service, streamline operations, and increase customer satisfaction.
AI Survey Data Processing for Different Industries
AI survey data processing significantly enhances the efficiency and effectiveness of data analysis across various industries. Here are four industries that benefit immensely from AI survey analytics and reports:
1. Retail
Retailers use AI to analyze customer feedback, identify popular products, and detect purchasing trends. AI-driven insights help in inventory management, marketing strategies, and improving customer experience. According to a McKinsey report, retailers that leverage AI increase their operating margins by up to 60% .
Example: A retail company uses AI to analyze customer feedback from an online survey. The AI tool identifies a high demand for sustainable products, prompting the company to expand its eco-friendly product line, resulting in a 25% increase in sales.
2. Healthcare
Healthcare providers use AI to process patient feedback, improve service quality, and enhance patient satisfaction. AI helps predict patient needs and optimize resource allocation. Accenture estimates that AI could save the healthcare industry $150 billion annually by 2026 .
Example: A hospital uses AI to analyze patient satisfaction surveys. The AI identifies common complaints about long wait times and suggests operational changes, leading to a 30% reduction in wait times and improved patient satisfaction.
3. Finance
Financial institutions use AI to analyze customer feedback, detect fraud, and assess risk. AI helps in personalizing services and improving customer satisfaction. According to PwC, AI could contribute up to $15.7 trillion to the global economy by 2030, with the financial sector being a signifit beneficiary .
Example: A bank uses AI to process customer feedback from various channels. The AI identifies recurring issues with a mobile banking app and suggests improvements, leading to a 40% increase in user satisfaction and reduced customer churn.
4. Education
Educational institutions use AI to analyze student feedback, identify areas for improvement, and tailor educational programs. AI enhances student engagement and learning outcomes. A study by the e-learning industry indicates that AI increases student retention rates by 35% .
Example: A university uses AI to analyze course evaluation surveys. The AI identifies specific courses with low satisfaction rates and recommends curriculum adjustments, resulting in improved student satisfaction and retention.
Sample AI Survey Data Analytics Report
Survey Overview
Survey Title: Customer Satisfaction Survey
Number of Respondents: 1,000
Survey Period: January 1, 2023 - January 31, 2023
Key Metrics
Overall Satisfaction Score: 4.2/5
Net Promoter Score (NPS): 45
Customer Effort Score (CES): 2.5/5
Sentiment Analysis
Positive Feedback: 65%
Neutral Feedback: 20%
Negative Feedback: 15%
Common Themes
Positive: Quality of customer service, product reliability, fast shipping
Negative: Website usability, product availability, customer support response time
Predictive Insights
Future Trends: Increased demand for online customer support, preference for sustainable products
Recommendations: Improve website usability, enhance product availability, invest in sustainable product lines
AI Survey Data Processing Best Practices
AI survey data processing leverages artificial intelligence and machine learning to handle the entire data lifecycle—from collection to analysis. To maximize the effectiveness and accuracy of AI-powered survey data processing, businesses should adhere to the following best practices:
1. Ensure Data Quality from the Start
Example: A retail company deploying customer satisfaction surveys should use AI-powered validation to check for incomplete responses or inconsistencies as data is entered, rather than addressing these issues post-collection.
Why It Matters: High-quality data is the foundation of accurate analysis. AI filters out duplicates, handles missing values, and validates responses in real-time, which ensures that the data collected is reliable and usable.
Tip: Implement AI algorithms that detect anomalies and outliers in real-time, allowing immediate correction and re-engagement of respondents if necessary.
2. Implement Adaptive Survey Techniques
Example: A software company conducting user experience surveys use AI to adapt questions based on user responses, ensuring more relevant and personalized feedback.
Why It Matters: Adaptive surveys enhance respondent engagement and data relevance. AI-driven adaptive questioning adjusts the survey flow based on previous answers, which keeps respondents interested and reduces survey fatigue.
Tip: Use AI to create dynamic surveys that modify questions in real-time based on user input, ensuring each respondent's journey through the survey is tailored to their responses.
3. Utilize Advanced Data Cleaning Methods
Example: A healthcare organization surveying patient satisfaction employs AI to automatically clean data by identifying and correcting input errors, handling missing values, and removing irrelevant data points.
Why It Matters: AI enhances data cleaning efficiency by automating the detection and correction of errors, reducing the manual effort and time required. This leads to more accurate datasets for analysis.
Tip: Integrate AI algorithms that handle natural language data to parse and clean open-ended responses, standardize entries, and reconcile inconsistencies.
4. Leverage Natural Language Processing (NLP)
Example: An e-commerce business using surveys to gather customer feedback on new product lines use NLP to analyze sentiments and categorize open-ended responses efficiently.
Why It Matters: NLP enables the processing of textual data at scale, extracting meaningful insights from qualitative feedback. It helps in understanding sentiments, themes, and the context behind responses.
Tip: Incorporate NLP tools to analyze open-ended responses for sentiment, categorize feedback, and identify emerging themes without manual intervention.
5. Enable Real-Time Data Processing
Example: A financial services firm conducting client feedback surveys use AI to analyze responses in real-time and promptly generate actionable insights for immediate decision-making.
Why It Matters: Real-time data processing enables businesses to act swiftly on feedback, enhancing responsiveness and allowing immediate adjustments to strategies or operations based on current data.
Tip: Use AI-driven dashboards that provide live updates on survey responses and key metrics, enabling quick data-driven decisions.
6. Integrate Predictive Analytics
Example: A telecom company uses AI to predict customer churn based on survey responses about service satisfaction, allowing proactive retention efforts.
Why It Matters: Predictive analytics helps anticipate future trends and behaviors based on current data, enabling businesses to implement preventive measures or capitalize on upcoming opportunities.
Tip: Employ AI models that forecast trends from survey data, such as customer satisfaction leading to churn or product feedback predicting future sales trends.
7. Ensure Data Security and Privacy
Example: A legal firm conducting client satisfaction surveys must use AI to anonymize responses and ensure that sensitive data is protected against unauthorized access.
Why It Matters: AI tools enhance data security by automating encryption, anonymization, and compliance checks, safeguarding respondent information and adhering to privacy regulations.
Tip: Utilize AI to continuously monitor data access patterns and enforce security protocols, ensuring compliance with data protection standards like GDPR.
8. Create Comprehensive Visualizations
Example: A marketing agency analyzing campaign effectiveness through client surveys use AI to create detailed visualizations of key metrics, making it easier to interpret and present findings.
Why It Matters: Visual representations of data make insights more accessible and understandable, aiding in effective communication of survey results to stakeholders.
Tip: Leverage AI tools to generate interactive dashboards and visualizations to customize based on the user’s role and data needs.
9. Optimize Survey Design Using AI Insights
Example: A tech startup analyzes survey completion rates and feedback with AI to identify patterns in survey design that affect respondent engagement, and refine future surveys accordingly.
Why It Matters: Continuous improvement of survey design based on AI-derived insights leads to higher response rates and better data quality.
Tip: Use AI analytics to review past survey performance and identify design elements that enhance or hinder respondent engagement.
10. Foster Transparency and Interpretability
Example: A nonprofit organization sharing impact assessment results from surveys should use AI to clearly explain how data is processed and analyzed, ensuring stakeholders understand the results.
Why It Matters: Transparency in AI-driven processes builds trust and ensures that the insights derived from data are understandable and actionable by all stakeholders.
Tip: Provide clear documentation and user-friendly interfaces that explain AI processing steps and the basis of insights generated from survey data.
Conclusion:
AI-powered survey data processing transforms traditional survey methodologies by automating data collection, enhancing data quality, and providing real-time, actionable insights. By adhering to best practices like adaptive survey techniques, advanced data cleaning, and real-time processing, businesses achieve more accurate and efficient survey outcomes. Leveraging AI tools not only improves survey design and data security but also enables predictive analytics and comprehensive visualizations, driving better business decisions and fostering deeper customer understanding. As AI continues to evolve, integrating these best practices ensures that businesses stay ahead in gathering and interpreting valuable customer insights effectively.
AI-powered surveys provide a scalable and cost-effective method to reach a broad audience, facilitating the collection of real-time data. They drive strategic planning, marketing initiatives, and operational improvements. AI survey data processing used in AI-native form builders like Metaforms provides insight survey analytics reports that helps businesses to zero in on finer details about their potential customers and loyal customers. Sign-up with Metaforms today.
Businesses conduct online surveys to gather valuable insights directly from their target audience which includes customers, employees and competitors. These surveys help in understanding customer preferences, employee satisfaction, market trends, and product feedback, which are crucial for informed decision-making. According to a report by Statista, 71% of businesses use surveys to measure customer satisfaction and loyalty.
But, manually processing survey data is time-consuming and error-prone. AI survey data processing automates survey analysis faster and more accurate insights. It enables businesses to make data-driven decisions efficiently. In this blog, we will explore how AI survey data processing works, its impact on different industries, and real-world use cases.
How AI Survey Data Processing Works
Traditional online surveys, including drag-and-drop form builders, face signifit challenges in survey data processing. Manual data cleaning and analyzing open-ended responses often requires intensive human effort. Adding to it, it's difficult to integrate data from various sources and generate real-time insights.
In contrast, AI survey form builders like Metaforms automate data cleaning, employ natural language processing (NLP) for analyzing open-ended responses, and provide real-time analytics. They seamlessly integrate multiple data sources, enhancing accuracy and efficiency. AI survey form builders offer a more technically advanced solution, streamlining the entire data processing workflow compared to traditional survey models.
AI survey data processing involves several steps that transform raw survey data into actionable insights. Here’s a detailed breakdown of the process:
Step-by-Step Guide to AI Survey Data Processing
AI survey data processing encompasses several stages, each designed to automate and optimize the handling of survey data from collection to actionable insights. Here's a detailed breakdown of the steps involved:
1. Data Collection Automation
Description: AI-driven surveys streamline data collection by automating the process of gathering responses through various channels such as emails, social media, and web embeds.
Multi-Channel Integration: AI integrates with multiple platforms, allowing surveys to be distributed and responses collected across diverse channels.
Real-Time Collection: Responses are collected in real-time, ensuring timely data availability for analysis.
Example: An e-commerce site uses AI to collect feedback from customers via email and pop-up surveys on the website.
2. Data Validation
Description: AI automatically validates the incoming data to ensure accuracy and consistency, reducing errors and the need for manual corrections.
Automatic Error Detection: AI identifies and flags inconsistent or invalid responses (e.g., conflicting answers, incomplete responses).
Real-Time Correction: Errors be corrected in real-time, prompting respondents to amend their inputs before submission.
Example: In a customer feedback survey, AI detects when a respondent skips a required question and prompts them to complete it before submission.
3. Data Cleaning
Description: AI tools clean the data by removing duplicates, handling missing values, and standardizing entries.
Deduplication: Removes repeated responses.
Missing Value Handling: Fills in missing data or highlights gaps for further action.
Standardization: Normalizes data formats for consistent analysis.
Example: A market research firm uses AI to standardize dates and remove duplicate entries from survey responses.
4. Natural Language Processing (NLP) for Open-Ended Responses
Description: AI employs NLP to analyze and categorize open-ended responses, extracting meaningful insights from qualitative data.
Sentiment Analysis: Determines the emotional tone of responses.
Topic Modeling: Identifies common themes and topics in textual responses.
Categorization: Automatically groups similar feedback for easier analysis.
Example: An airline uses NLP to analyze customer feedback, identifying common issues related to flight delays and service quality.
5. Data Enrichment
Description: AI enriches the survey data by integrating it with external data sources or additional internal databases to provide a more comprehensive analysis.
External Integration: Combines survey data with CRM systems, social media, or other databases for enriched insights.
Contextual Enhancement: Adds contextual information that enhances the understanding of responses.
Example: A B2B company enriches survey responses with CRM data to correlate customer satisfaction with purchase history.
6. Real-Time Data Analysis
Description: AI processes and analyzes the data in real-time, providing immediate insights and visualizations.
Immediate Insights: Generates reports and analytics as responses come in, allowing for prompt decision-making.
Automated Dashboards: Creates dynamic dashboards that update automatically with incoming data.
Example: A tech startup uses AI to analyze user feedback from beta testers in real-time, identifying issues and areas for improvement instantly.
7. Predictive Analytics
Description: AI predictive models forecasts trends, behaviors, and outcomes based on the collected survey data.
Trend Forecasting: Predicts future trends from current data.
Behavior Analysis: Anticipates customer behavior patterns and potential actions.
Outcome Prediction: Estimates likely outcomes based on survey responses.\
Example: A telecom company predicts customer churn by analyzing satisfaction survey data and identifying at-risk customers.
8. Data Visualization
Description: AI creates visual representations of the data, such as charts, graphs, and dashboards, making it easier to interpret and communicate findings.
Interactive Visuals: Provides interactive charts and dashboards for deeper exploration.
Customizable Reports: Offers flexible reporting options tailored to different stakeholder needs.
Example: A financial services firm uses AI to generate interactive dashboards that visualize customer satisfaction trends over time.
9. Automated Reporting
Description: AI generates comprehensive reports automatically, summarizing key findings and insights from the survey data.
Summary Reports: Compiles essential metrics and insights into easily digestible formats.
Detailed Analysis: Provides in-depth analysis reports for more granular insights.
Example: An educational institution receives automated reports on student feedback, highlighting areas for curriculum improvement.
10. Actionable Recommendations
Description: AI provides actionable recommendations based on the survey data analysis, guiding businesses on next steps.
Data-Driven Suggestions: Offers practical suggestions derived from data trends.
Strategic Insights: Helps inform strategic decisions with evidence-based recommendations.
Example: A retail brand receives AI-generated recommendations to enhance customer service based on feedback trends showing a need for quicker response times.
Use Case: AI-Powered Survey Data Processing for a Retail Brand
Scenario: A retail brand conducts a customer satisfaction survey to improve its shopping experience.
AI Survey Data Processing Steps Implemented:
1. Data Collection: AI distributes the survey via email and social media, collecting responses in real-time.
2. Data Validation: AI validates responses, ensuring completeness and consistency.
3. Data Cleaning: AI removes duplicate responses and handles missing data.
4. NLP for Open-Ended Responses: AI analyzes open-ended feedback for common themes and sentiments.
5. Data Enrichment: AI enriches survey data with purchase history from the CRM system.
6. Real-Time Analysis: AI processes and analyzes data as it is collected, providing immediate insights.
7. Predictive Analytics: AI predicts future purchasing behavior based on satisfaction levels.
8. Data Visualization: AI generates visual reports and dashboards for easy interpretation.
9. Automated Reporting: AI delivers comprehensive summary and detailed reports.
10. Actionable Recommendations: AI provides recommendations for improving the shopping experience.
Outcome: The retail brand uses the insights and recommendations to enhance customer service, streamline operations, and increase customer satisfaction.
AI Survey Data Processing for Different Industries
AI survey data processing significantly enhances the efficiency and effectiveness of data analysis across various industries. Here are four industries that benefit immensely from AI survey analytics and reports:
1. Retail
Retailers use AI to analyze customer feedback, identify popular products, and detect purchasing trends. AI-driven insights help in inventory management, marketing strategies, and improving customer experience. According to a McKinsey report, retailers that leverage AI increase their operating margins by up to 60% .
Example: A retail company uses AI to analyze customer feedback from an online survey. The AI tool identifies a high demand for sustainable products, prompting the company to expand its eco-friendly product line, resulting in a 25% increase in sales.
2. Healthcare
Healthcare providers use AI to process patient feedback, improve service quality, and enhance patient satisfaction. AI helps predict patient needs and optimize resource allocation. Accenture estimates that AI could save the healthcare industry $150 billion annually by 2026 .
Example: A hospital uses AI to analyze patient satisfaction surveys. The AI identifies common complaints about long wait times and suggests operational changes, leading to a 30% reduction in wait times and improved patient satisfaction.
3. Finance
Financial institutions use AI to analyze customer feedback, detect fraud, and assess risk. AI helps in personalizing services and improving customer satisfaction. According to PwC, AI could contribute up to $15.7 trillion to the global economy by 2030, with the financial sector being a signifit beneficiary .
Example: A bank uses AI to process customer feedback from various channels. The AI identifies recurring issues with a mobile banking app and suggests improvements, leading to a 40% increase in user satisfaction and reduced customer churn.
4. Education
Educational institutions use AI to analyze student feedback, identify areas for improvement, and tailor educational programs. AI enhances student engagement and learning outcomes. A study by the e-learning industry indicates that AI increases student retention rates by 35% .
Example: A university uses AI to analyze course evaluation surveys. The AI identifies specific courses with low satisfaction rates and recommends curriculum adjustments, resulting in improved student satisfaction and retention.
Sample AI Survey Data Analytics Report
Survey Overview
Survey Title: Customer Satisfaction Survey
Number of Respondents: 1,000
Survey Period: January 1, 2023 - January 31, 2023
Key Metrics
Overall Satisfaction Score: 4.2/5
Net Promoter Score (NPS): 45
Customer Effort Score (CES): 2.5/5
Sentiment Analysis
Positive Feedback: 65%
Neutral Feedback: 20%
Negative Feedback: 15%
Common Themes
Positive: Quality of customer service, product reliability, fast shipping
Negative: Website usability, product availability, customer support response time
Predictive Insights
Future Trends: Increased demand for online customer support, preference for sustainable products
Recommendations: Improve website usability, enhance product availability, invest in sustainable product lines
AI Survey Data Processing Best Practices
AI survey data processing leverages artificial intelligence and machine learning to handle the entire data lifecycle—from collection to analysis. To maximize the effectiveness and accuracy of AI-powered survey data processing, businesses should adhere to the following best practices:
1. Ensure Data Quality from the Start
Example: A retail company deploying customer satisfaction surveys should use AI-powered validation to check for incomplete responses or inconsistencies as data is entered, rather than addressing these issues post-collection.
Why It Matters: High-quality data is the foundation of accurate analysis. AI filters out duplicates, handles missing values, and validates responses in real-time, which ensures that the data collected is reliable and usable.
Tip: Implement AI algorithms that detect anomalies and outliers in real-time, allowing immediate correction and re-engagement of respondents if necessary.
2. Implement Adaptive Survey Techniques
Example: A software company conducting user experience surveys use AI to adapt questions based on user responses, ensuring more relevant and personalized feedback.
Why It Matters: Adaptive surveys enhance respondent engagement and data relevance. AI-driven adaptive questioning adjusts the survey flow based on previous answers, which keeps respondents interested and reduces survey fatigue.
Tip: Use AI to create dynamic surveys that modify questions in real-time based on user input, ensuring each respondent's journey through the survey is tailored to their responses.
3. Utilize Advanced Data Cleaning Methods
Example: A healthcare organization surveying patient satisfaction employs AI to automatically clean data by identifying and correcting input errors, handling missing values, and removing irrelevant data points.
Why It Matters: AI enhances data cleaning efficiency by automating the detection and correction of errors, reducing the manual effort and time required. This leads to more accurate datasets for analysis.
Tip: Integrate AI algorithms that handle natural language data to parse and clean open-ended responses, standardize entries, and reconcile inconsistencies.
4. Leverage Natural Language Processing (NLP)
Example: An e-commerce business using surveys to gather customer feedback on new product lines use NLP to analyze sentiments and categorize open-ended responses efficiently.
Why It Matters: NLP enables the processing of textual data at scale, extracting meaningful insights from qualitative feedback. It helps in understanding sentiments, themes, and the context behind responses.
Tip: Incorporate NLP tools to analyze open-ended responses for sentiment, categorize feedback, and identify emerging themes without manual intervention.
5. Enable Real-Time Data Processing
Example: A financial services firm conducting client feedback surveys use AI to analyze responses in real-time and promptly generate actionable insights for immediate decision-making.
Why It Matters: Real-time data processing enables businesses to act swiftly on feedback, enhancing responsiveness and allowing immediate adjustments to strategies or operations based on current data.
Tip: Use AI-driven dashboards that provide live updates on survey responses and key metrics, enabling quick data-driven decisions.
6. Integrate Predictive Analytics
Example: A telecom company uses AI to predict customer churn based on survey responses about service satisfaction, allowing proactive retention efforts.
Why It Matters: Predictive analytics helps anticipate future trends and behaviors based on current data, enabling businesses to implement preventive measures or capitalize on upcoming opportunities.
Tip: Employ AI models that forecast trends from survey data, such as customer satisfaction leading to churn or product feedback predicting future sales trends.
7. Ensure Data Security and Privacy
Example: A legal firm conducting client satisfaction surveys must use AI to anonymize responses and ensure that sensitive data is protected against unauthorized access.
Why It Matters: AI tools enhance data security by automating encryption, anonymization, and compliance checks, safeguarding respondent information and adhering to privacy regulations.
Tip: Utilize AI to continuously monitor data access patterns and enforce security protocols, ensuring compliance with data protection standards like GDPR.
8. Create Comprehensive Visualizations
Example: A marketing agency analyzing campaign effectiveness through client surveys use AI to create detailed visualizations of key metrics, making it easier to interpret and present findings.
Why It Matters: Visual representations of data make insights more accessible and understandable, aiding in effective communication of survey results to stakeholders.
Tip: Leverage AI tools to generate interactive dashboards and visualizations to customize based on the user’s role and data needs.
9. Optimize Survey Design Using AI Insights
Example: A tech startup analyzes survey completion rates and feedback with AI to identify patterns in survey design that affect respondent engagement, and refine future surveys accordingly.
Why It Matters: Continuous improvement of survey design based on AI-derived insights leads to higher response rates and better data quality.
Tip: Use AI analytics to review past survey performance and identify design elements that enhance or hinder respondent engagement.
10. Foster Transparency and Interpretability
Example: A nonprofit organization sharing impact assessment results from surveys should use AI to clearly explain how data is processed and analyzed, ensuring stakeholders understand the results.
Why It Matters: Transparency in AI-driven processes builds trust and ensures that the insights derived from data are understandable and actionable by all stakeholders.
Tip: Provide clear documentation and user-friendly interfaces that explain AI processing steps and the basis of insights generated from survey data.
Conclusion:
AI-powered survey data processing transforms traditional survey methodologies by automating data collection, enhancing data quality, and providing real-time, actionable insights. By adhering to best practices like adaptive survey techniques, advanced data cleaning, and real-time processing, businesses achieve more accurate and efficient survey outcomes. Leveraging AI tools not only improves survey design and data security but also enables predictive analytics and comprehensive visualizations, driving better business decisions and fostering deeper customer understanding. As AI continues to evolve, integrating these best practices ensures that businesses stay ahead in gathering and interpreting valuable customer insights effectively.
AI-powered surveys provide a scalable and cost-effective method to reach a broad audience, facilitating the collection of real-time data. They drive strategic planning, marketing initiatives, and operational improvements. AI survey data processing used in AI-native form builders like Metaforms provides insight survey analytics reports that helps businesses to zero in on finer details about their potential customers and loyal customers. Sign-up with Metaforms today.
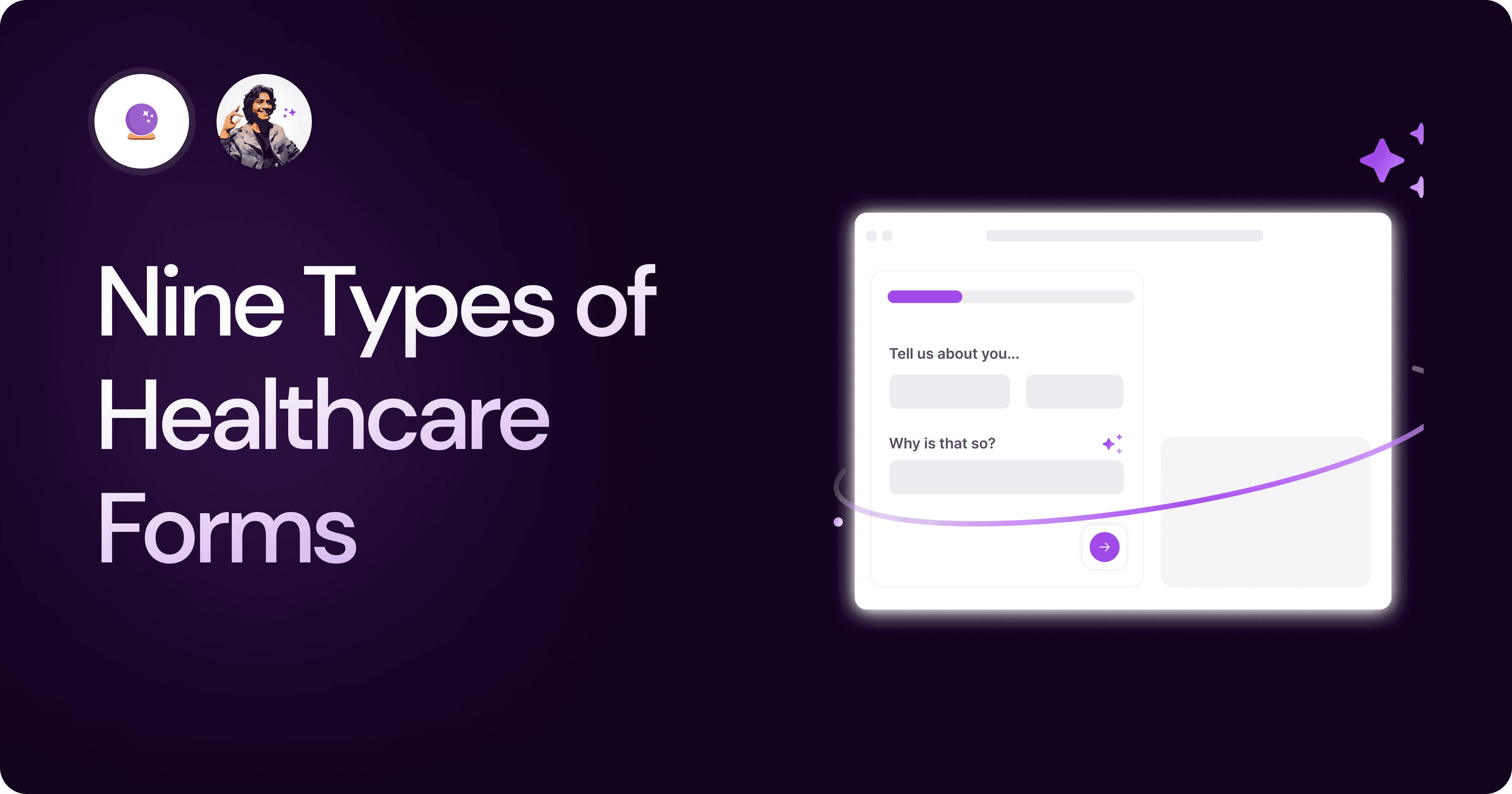
Nine Types of Healthcare and Medical Forms.
Medical forms are a must-have for any healthcare business or practitioner. Learn about the different kinds of medical and healthcare forms.
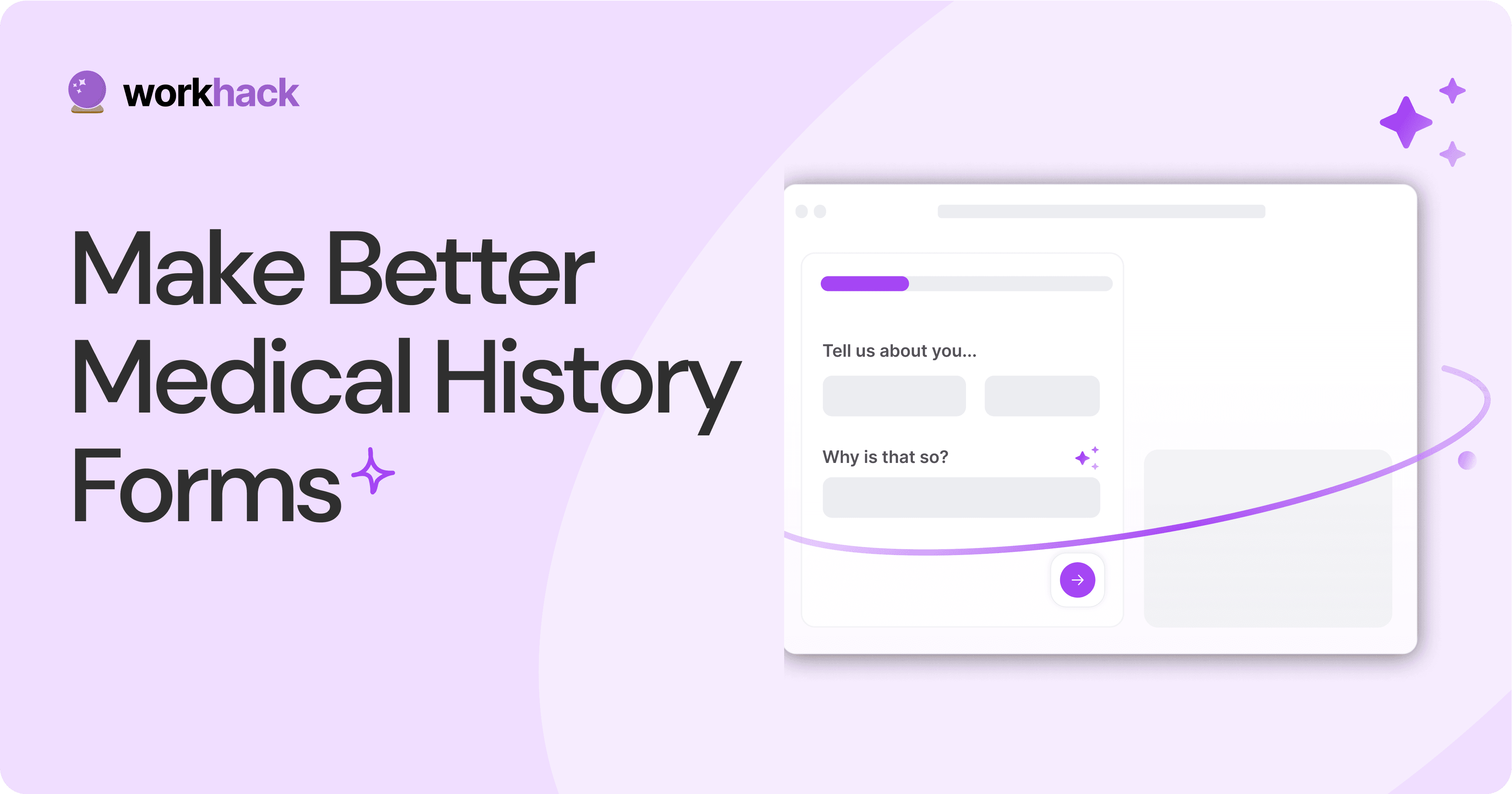
4 Tips for Better Medical History Forms.
Medical history forms are central to patient care, onboarding, and medical administration records. Learn how to make them easier to fill.
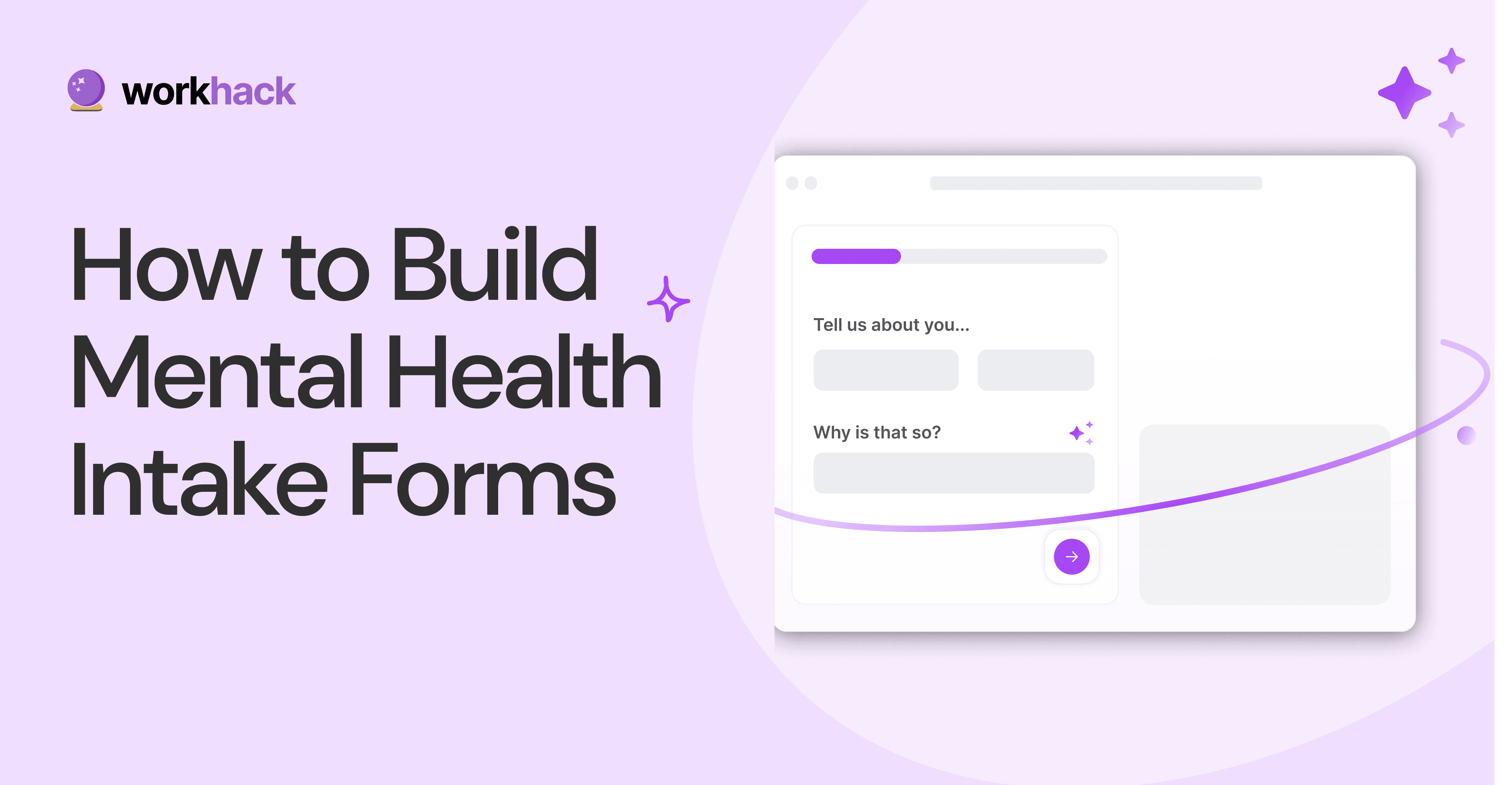
How to Build Mental Health Intake Forms?
Mental health intake forms are not like patient intake forms. Mental health intake forms deal with far more sensitive data and have specific design methods.
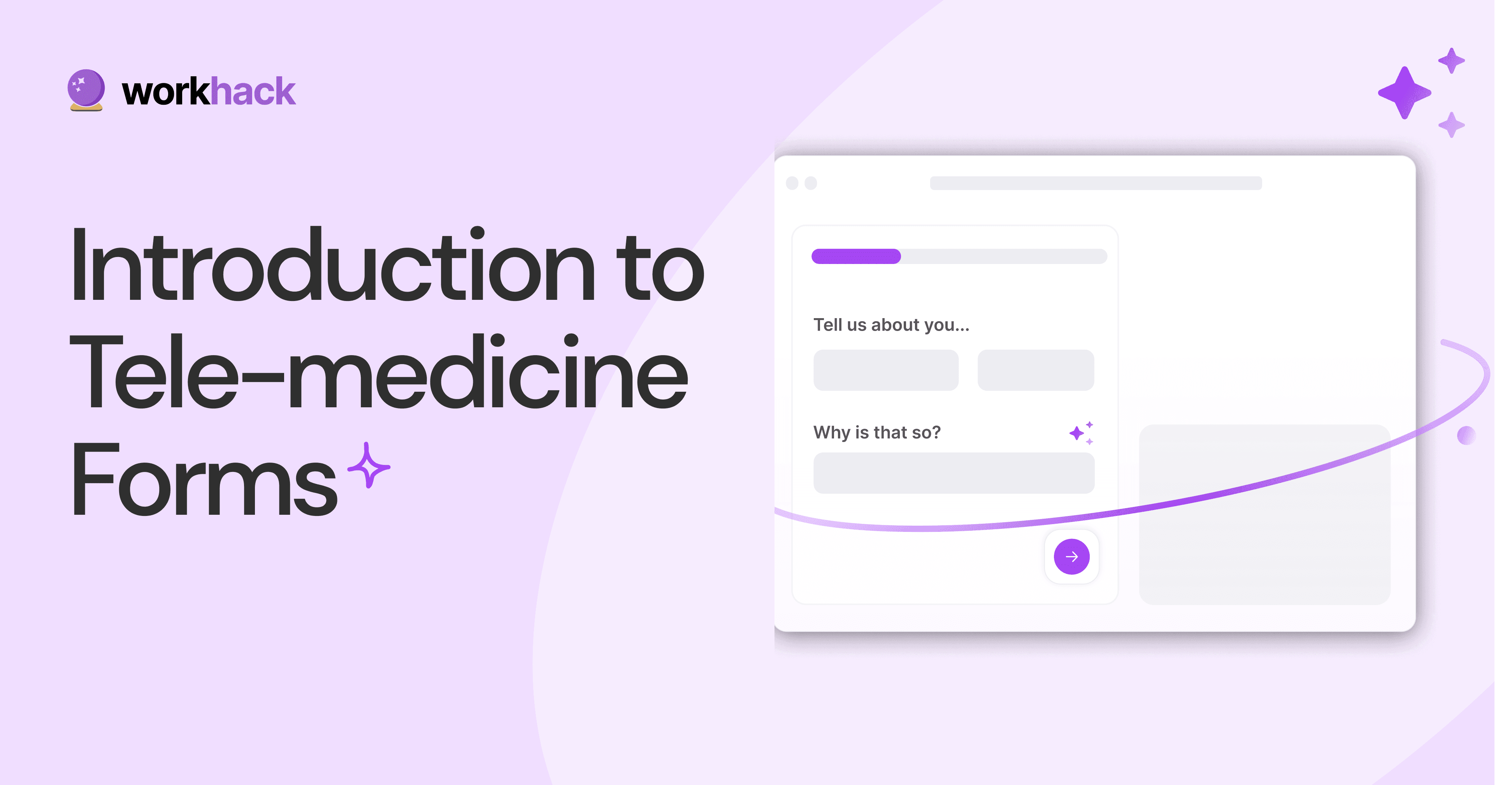
What, Why and How of Telemedicine Forms.
Telemedicine is on the rise and with different form builders out there, which one best suits your needs as a healthcare services provider?
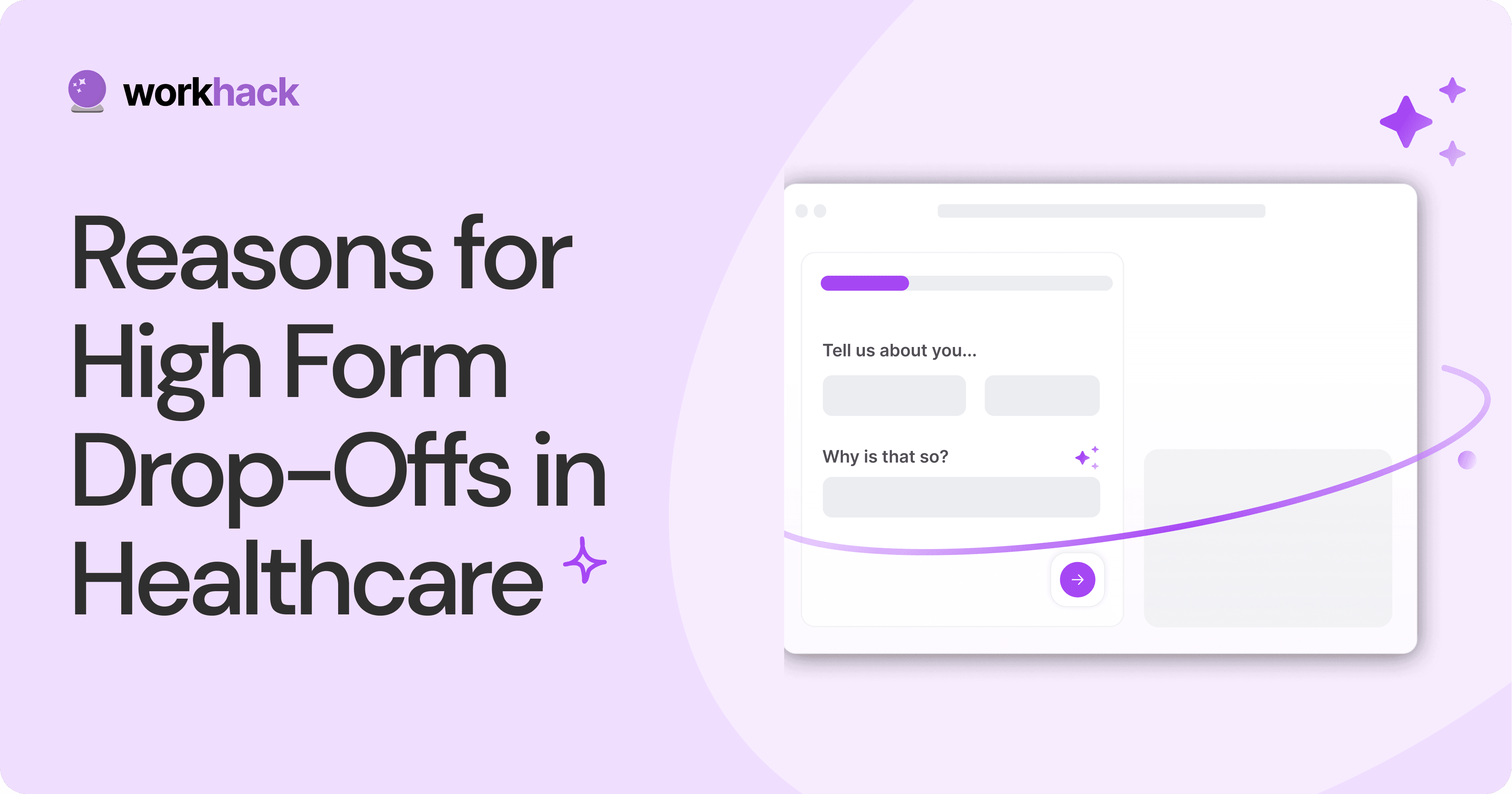
3 Reasons for Major Drop-Offs in Medical Forms.
No matter which healthcare form we pick, there are major drop-off reasons. We shall dive into the top 3 and learn how to resolve them in your next form.
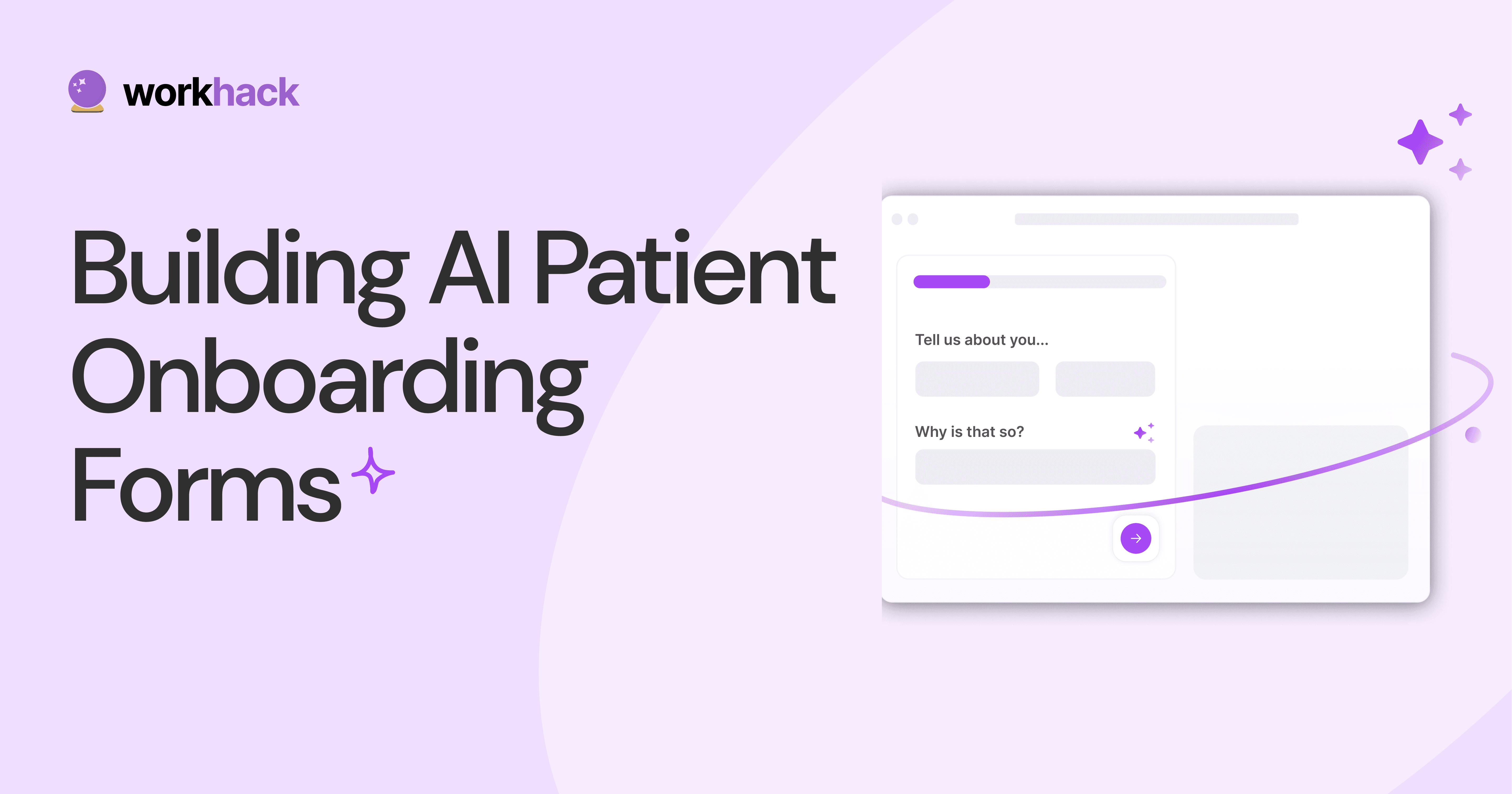
Patient Onboarding Forms - From Click to Clinic.
Patient onboarding forms are the first touchpoint for patients; getting this right for higher conversion rates is a must-have. Learn how to perfect them now.

5 Key Parts of a Good Patient Satisfaction Form.
The goal of patient satisfaction surveys is to course-correct the services of a healthcare provider. Patient feedback leads to a culture of patient-centric care.
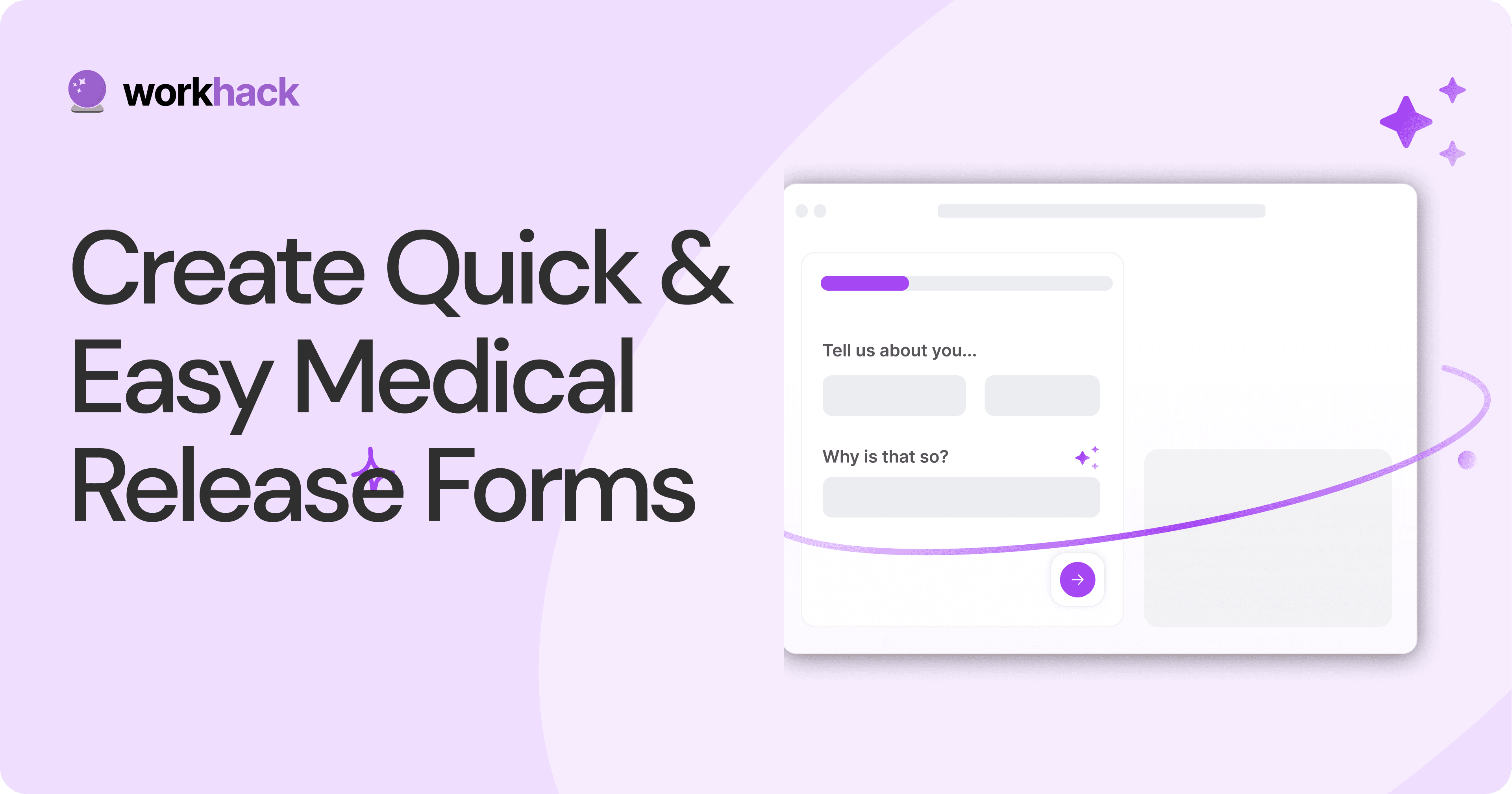
Build Quick and Easy Medical Release Forms.
Every HIPAA-compliant healthcare provider comes across medical release forms that involve details from medical history forms. Can they be shipped fast? Yes.
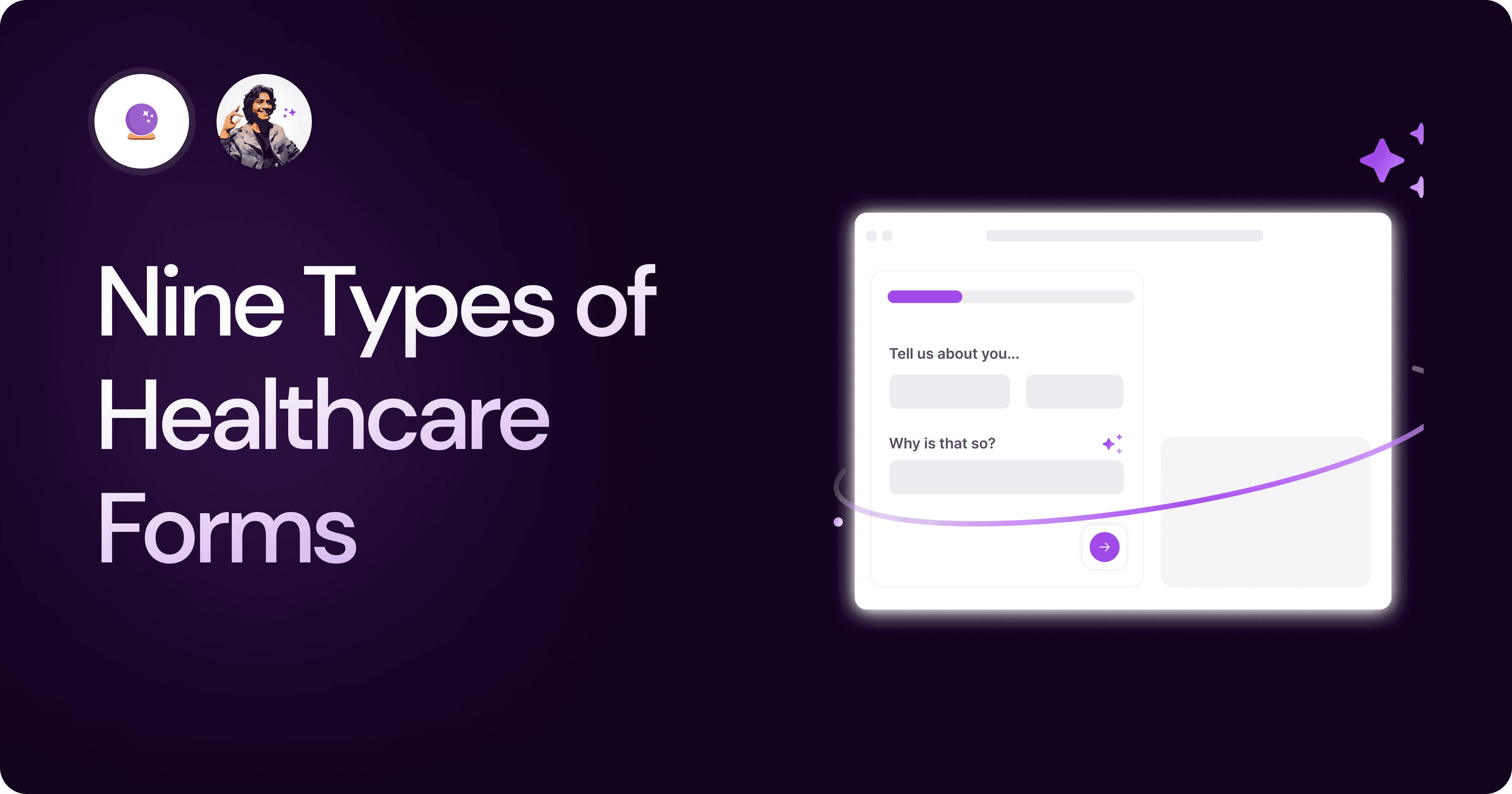
Nine Types of Healthcare and Medical Forms.
Medical forms are a must-have for any healthcare business or practitioner. Learn about the different kinds of medical and healthcare forms.
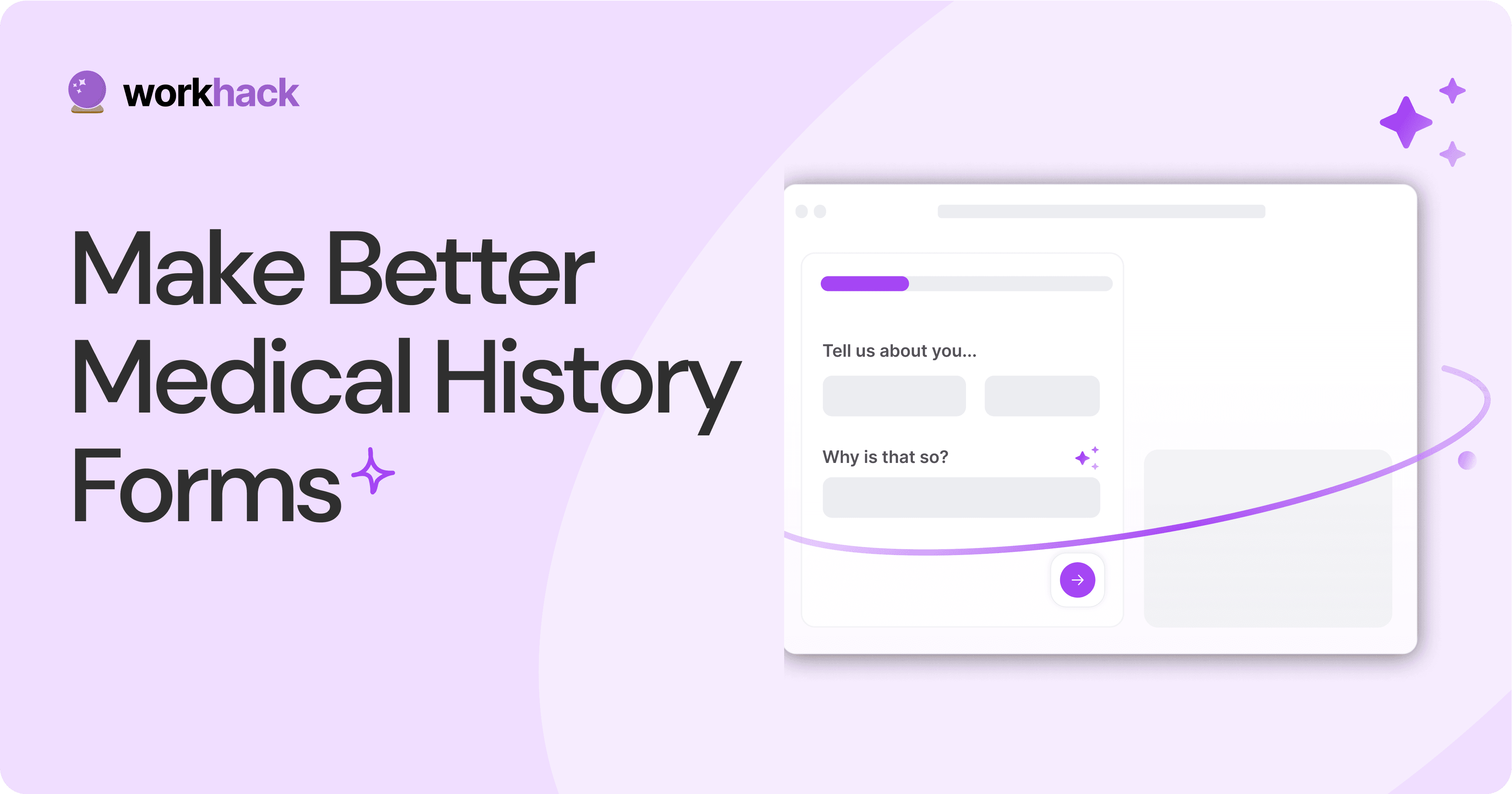
4 Tips for Better Medical History Forms.
Medical history forms are central to patient care, onboarding, and medical administration records. Learn how to make them easier to fill.
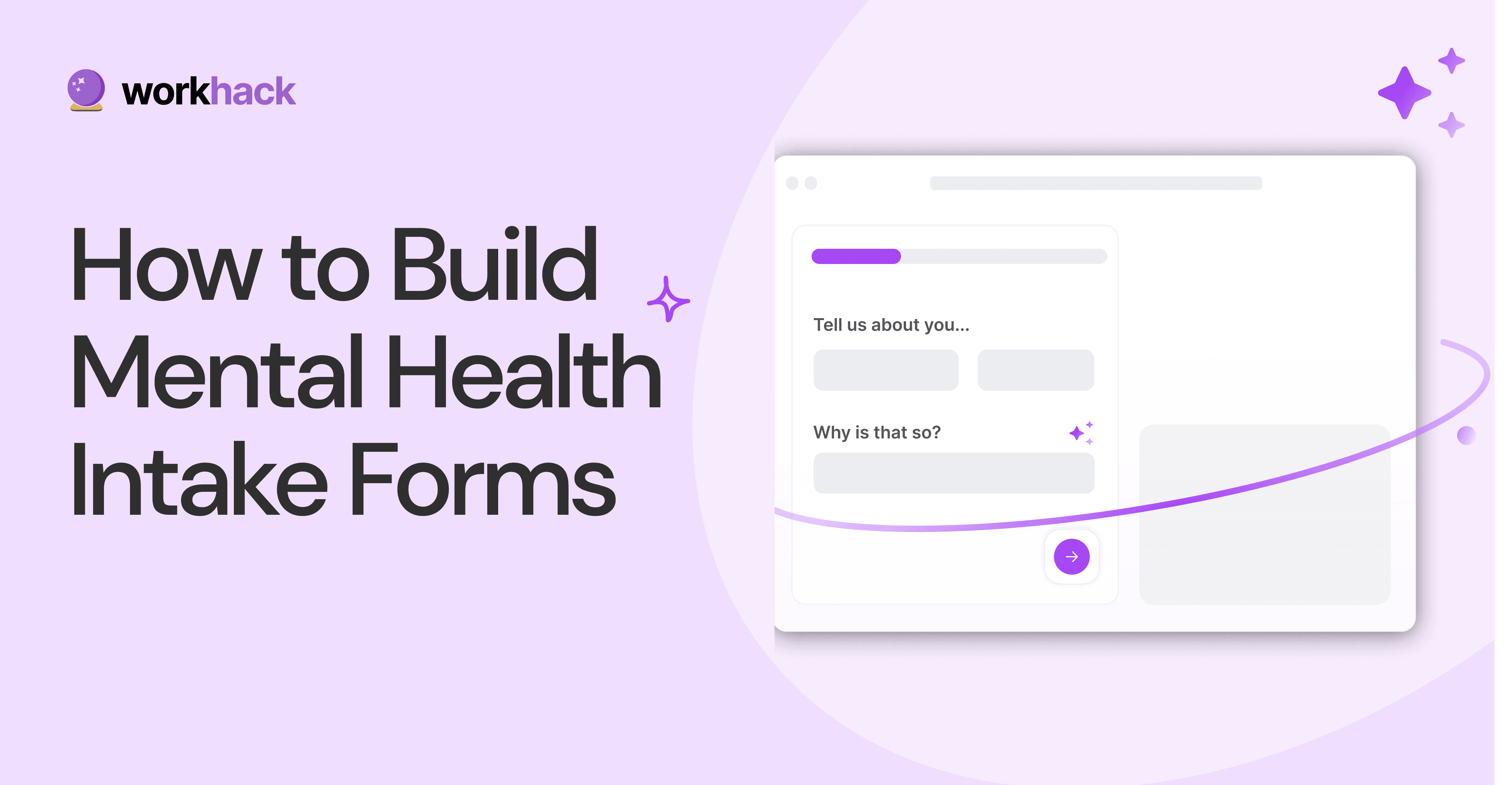
How to Build Mental Health Intake Forms?
Mental health intake forms are not like patient intake forms. Mental health intake forms deal with far more sensitive data and have specific design methods.
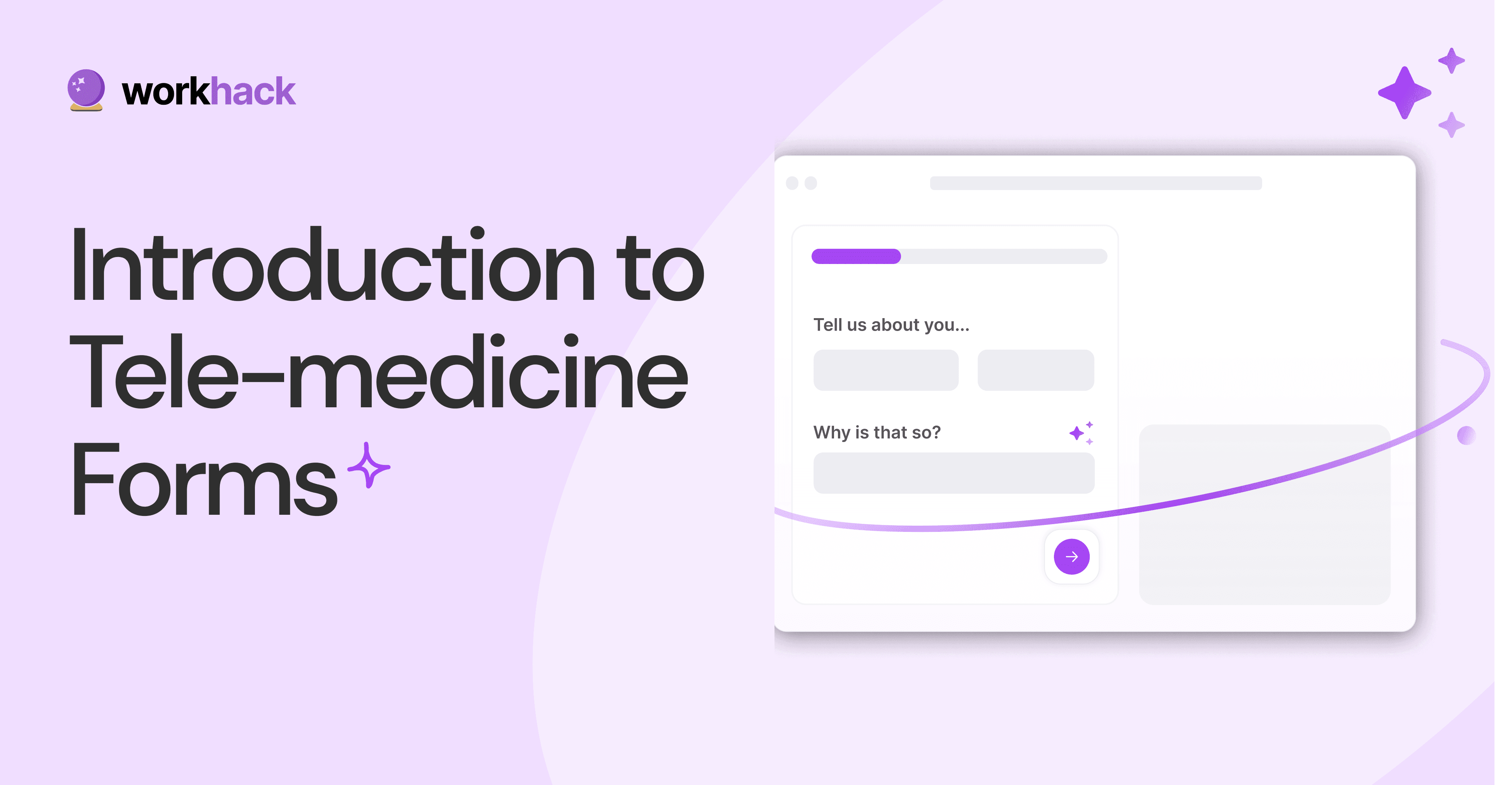
What, Why and How of Telemedicine Forms.
Telemedicine is on the rise and with different form builders out there, which one best suits your needs as a healthcare services provider?
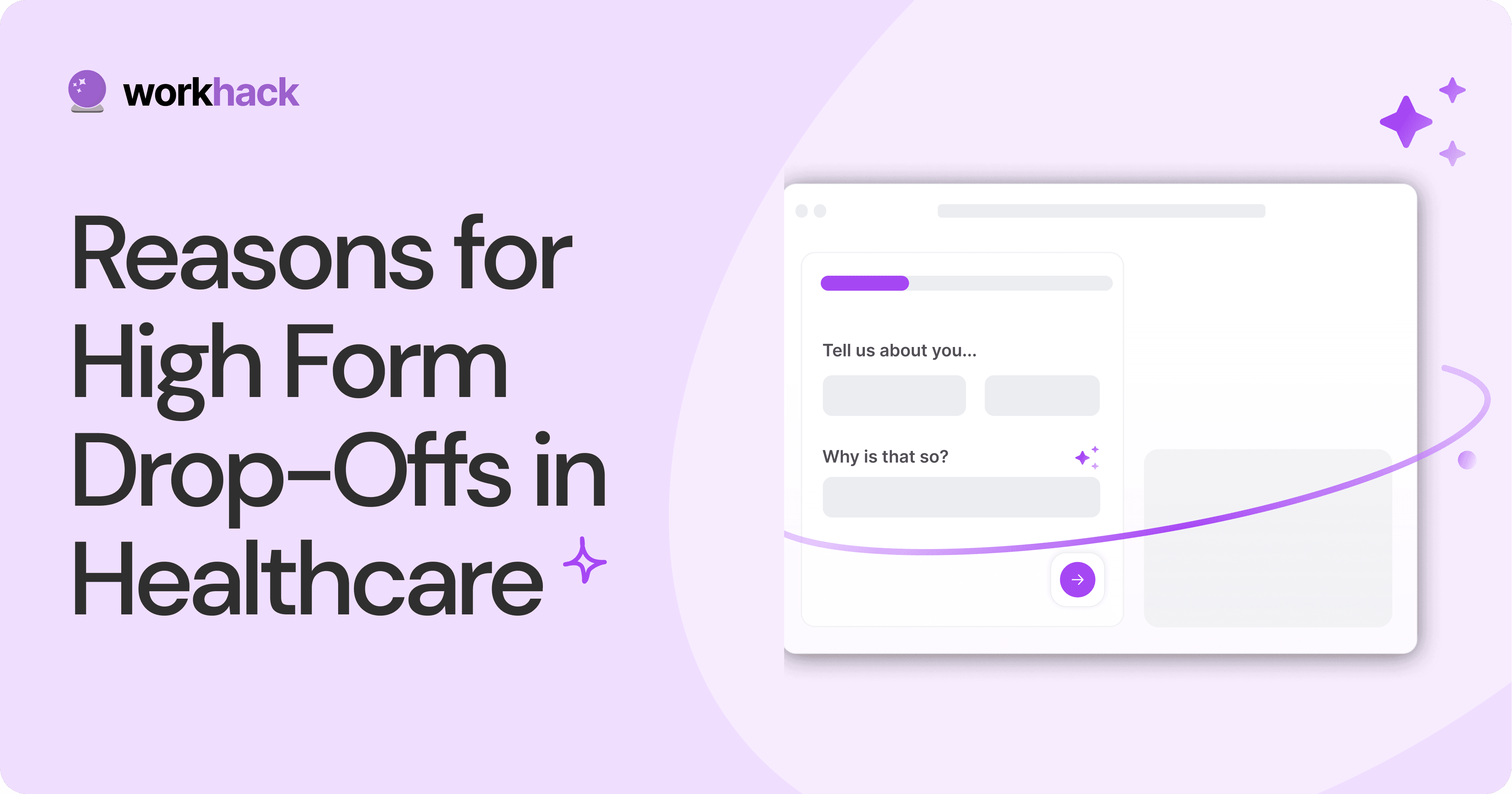
3 Reasons for Major Drop-Offs in Medical Forms.
No matter which healthcare form we pick, there are major drop-off reasons. We shall dive into the top 3 and learn how to resolve them in your next form.
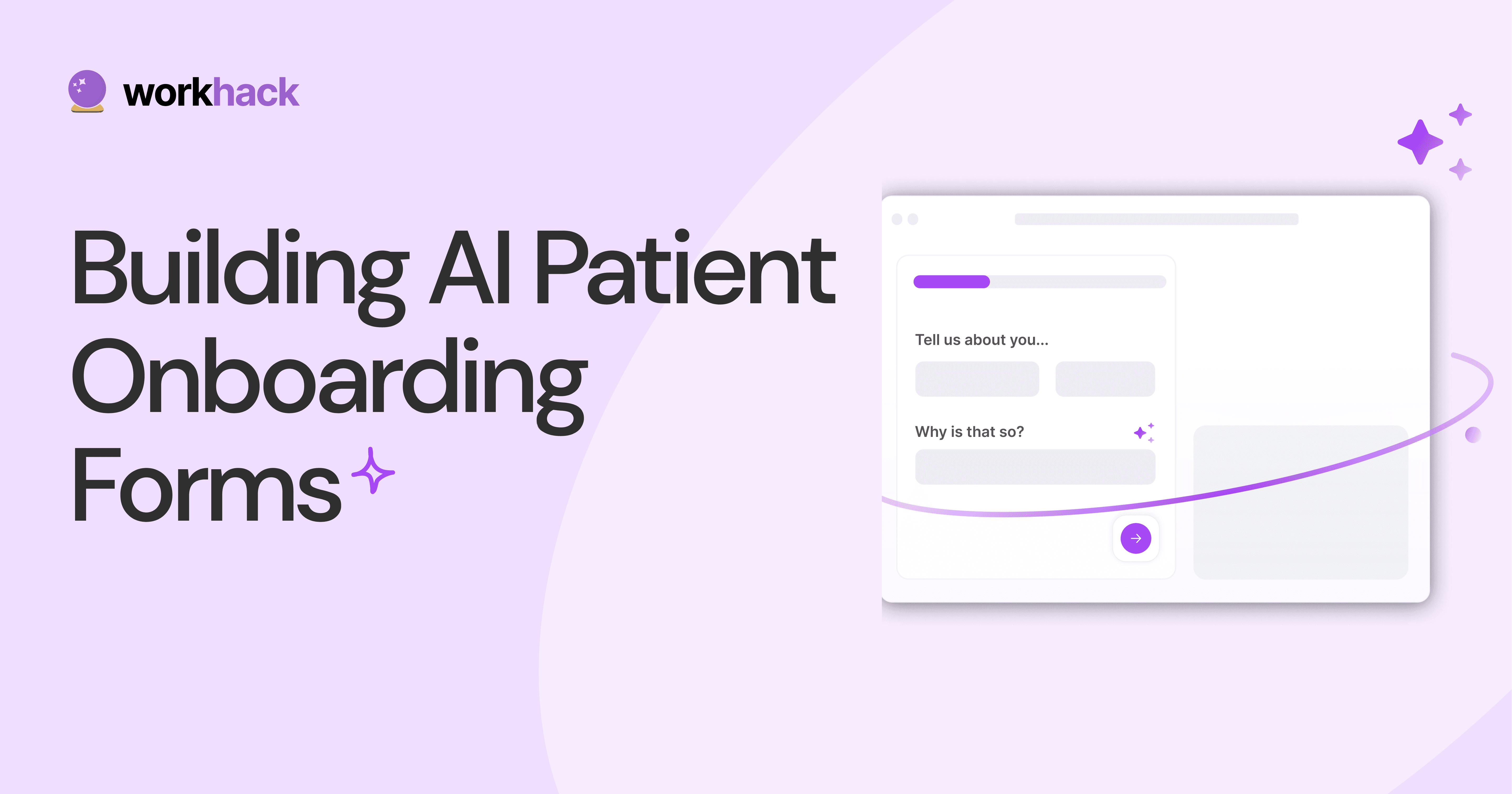
Patient Onboarding Forms - From Click to Clinic.
Patient onboarding forms are the first touchpoint for patients; getting this right for higher conversion rates is a must-have. Learn how to perfect them now.

5 Key Parts of a Good Patient Satisfaction Form.
The goal of patient satisfaction surveys is to course-correct the services of a healthcare provider. Patient feedback leads to a culture of patient-centric care.
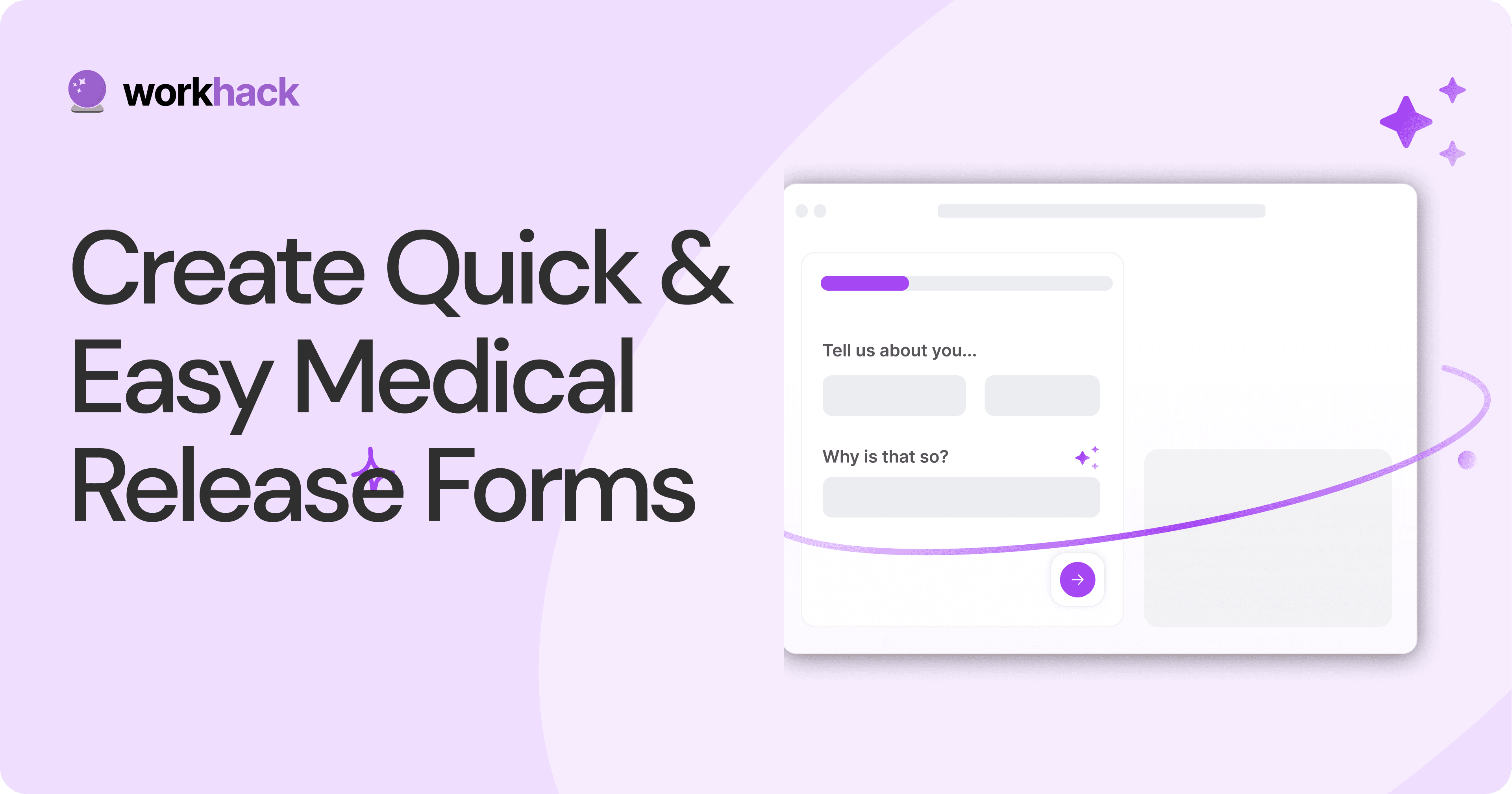
Build Quick and Easy Medical Release Forms.
Every HIPAA-compliant healthcare provider comes across medical release forms that involve details from medical history forms. Can they be shipped fast? Yes.
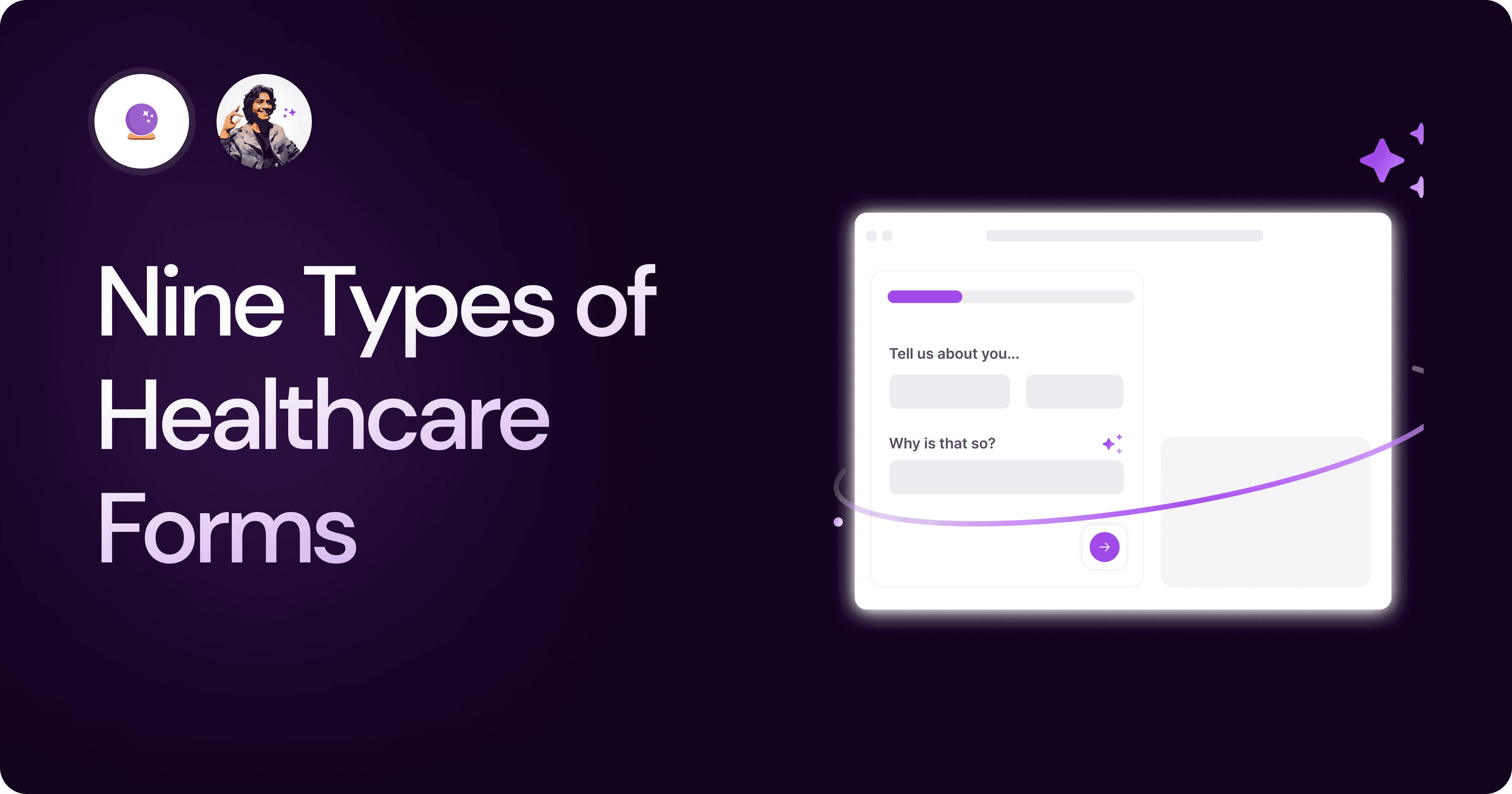
Nine Types of Healthcare and Medical Forms.
Medical forms are a must-have for any healthcare business or practitioner. Learn about the different kinds of medical and healthcare forms.
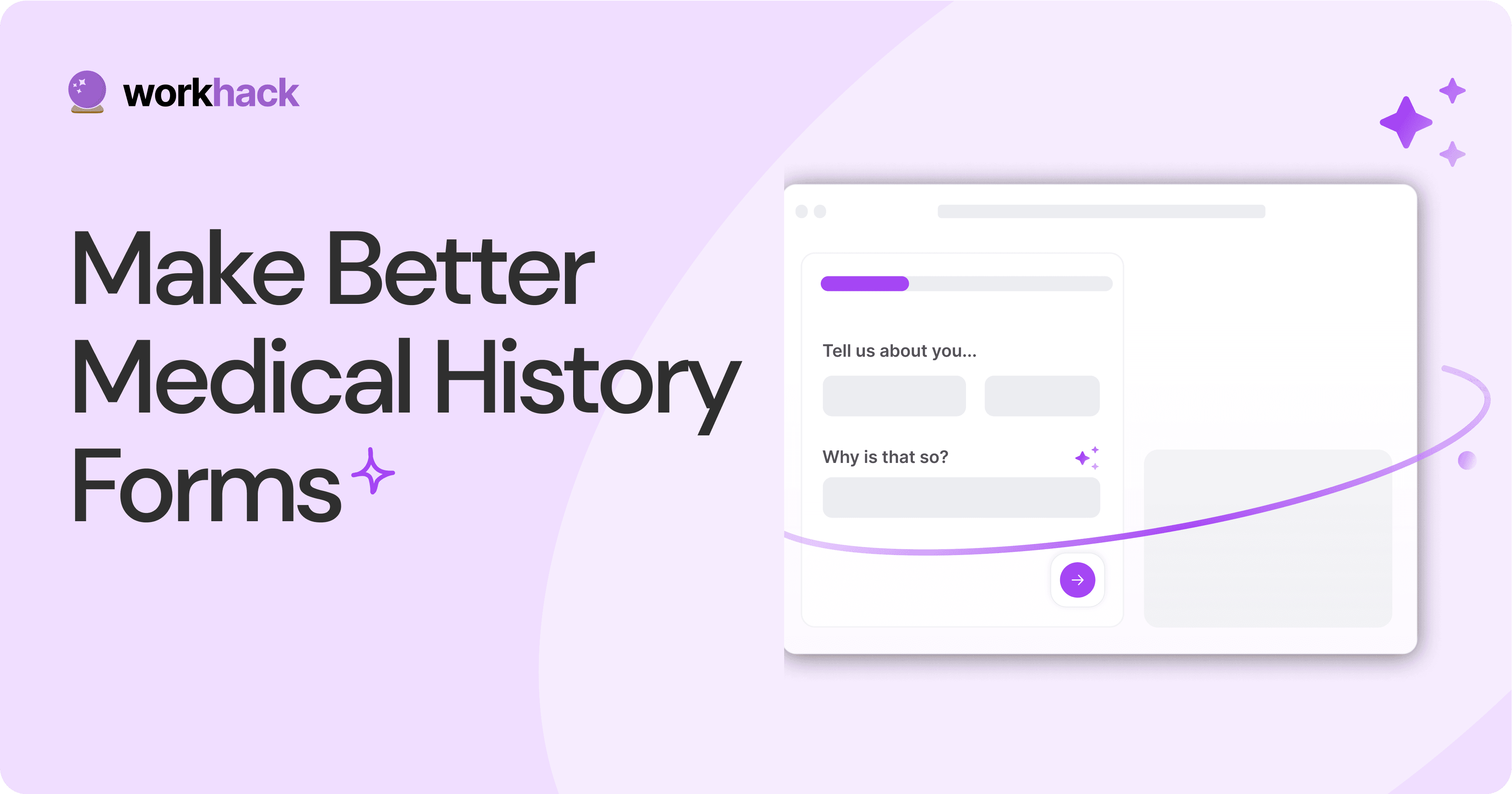
4 Tips for Better Medical History Forms.
Medical history forms are central to patient care, onboarding, and medical administration records. Learn how to make them easier to fill.
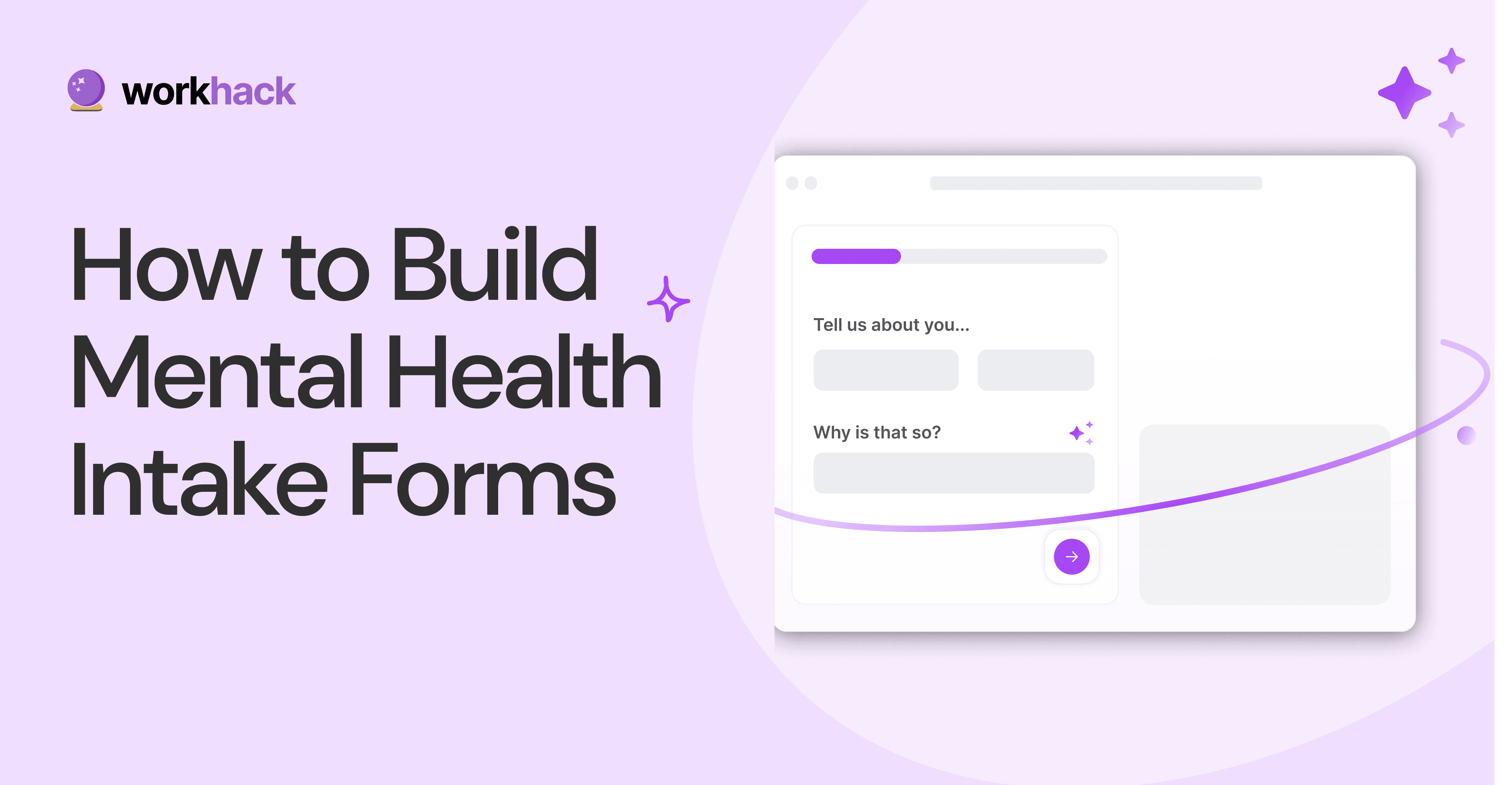
How to Build Mental Health Intake Forms?
Mental health intake forms are not like patient intake forms. Mental health intake forms deal with far more sensitive data and have specific design methods.
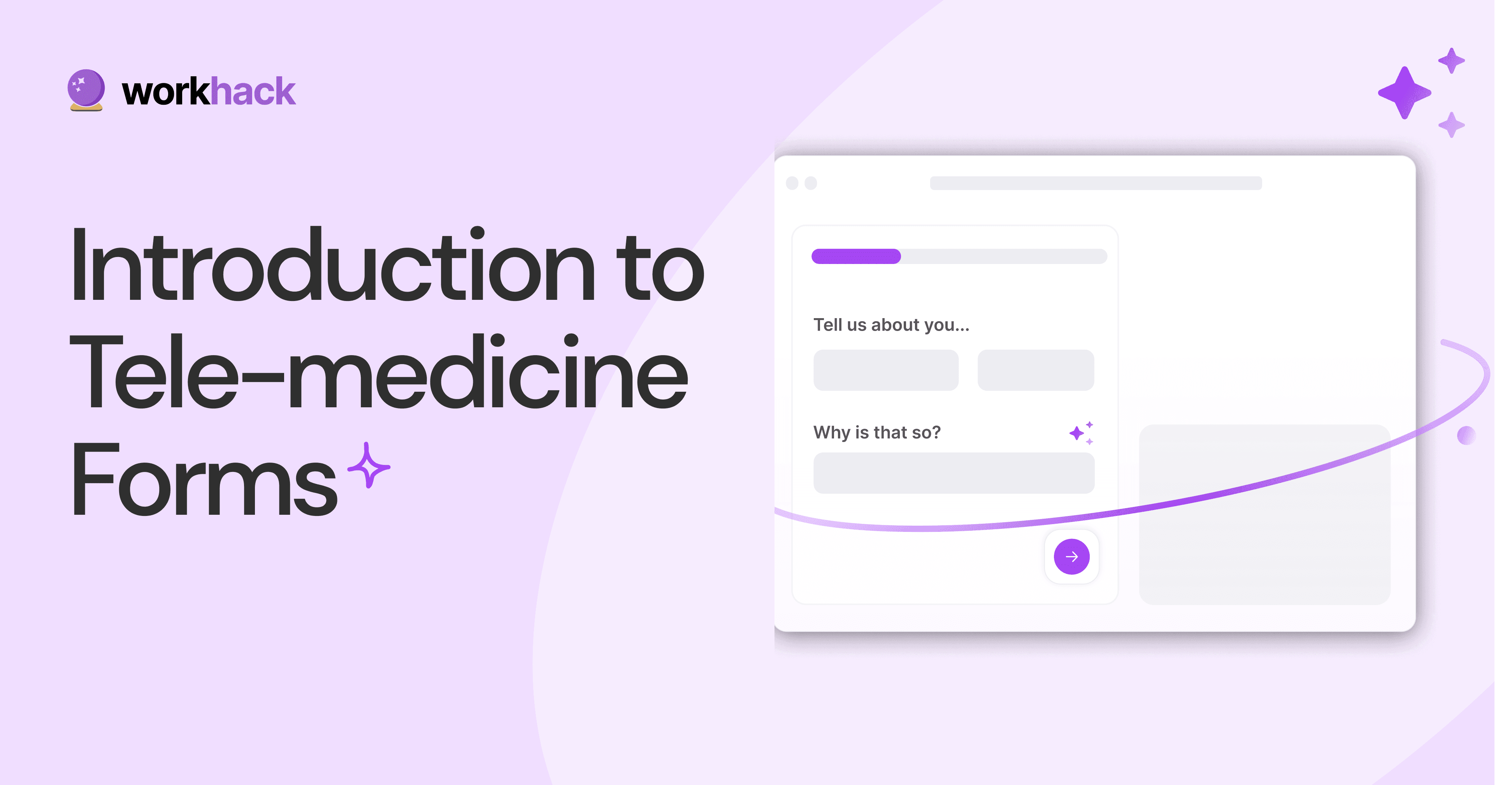
What, Why and How of Telemedicine Forms.
Telemedicine is on the rise and with different form builders out there, which one best suits your needs as a healthcare services provider?
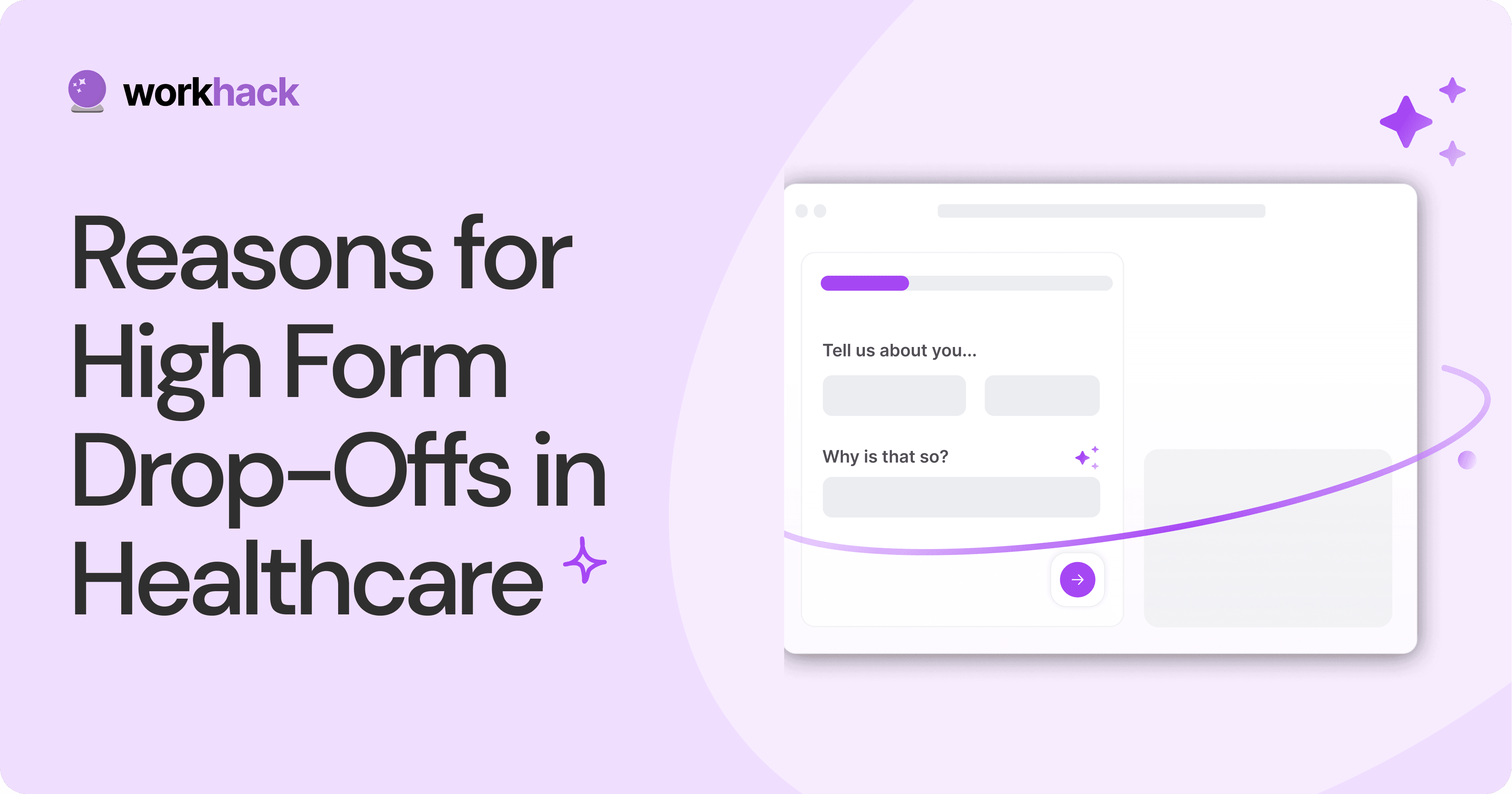
3 Reasons for Major Drop-Offs in Medical Forms.
No matter which healthcare form we pick, there are major drop-off reasons. We shall dive into the top 3 and learn how to resolve them in your next form.
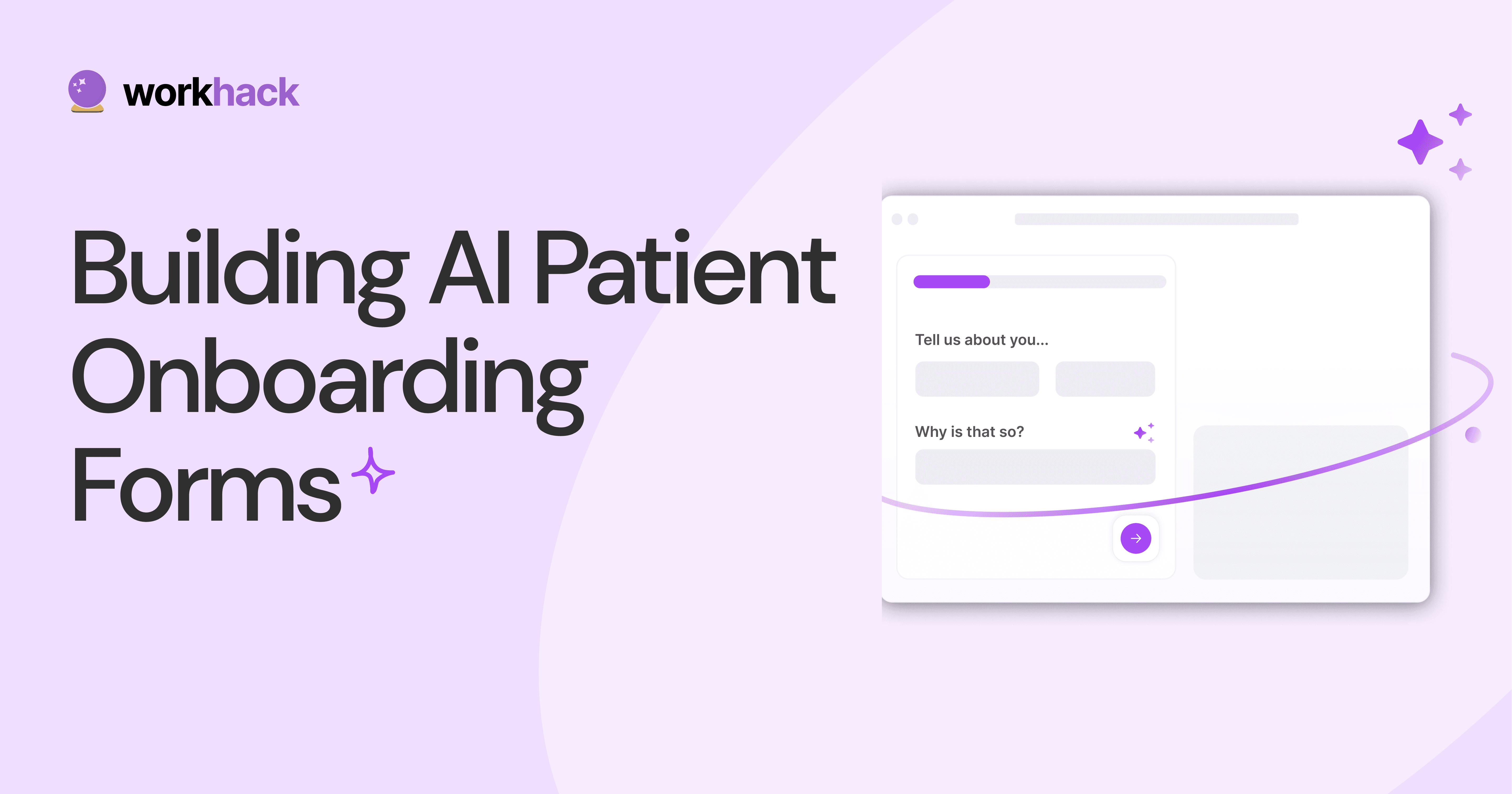
Patient Onboarding Forms - From Click to Clinic.
Patient onboarding forms are the first touchpoint for patients; getting this right for higher conversion rates is a must-have. Learn how to perfect them now.

5 Key Parts of a Good Patient Satisfaction Form.
The goal of patient satisfaction surveys is to course-correct the services of a healthcare provider. Patient feedback leads to a culture of patient-centric care.
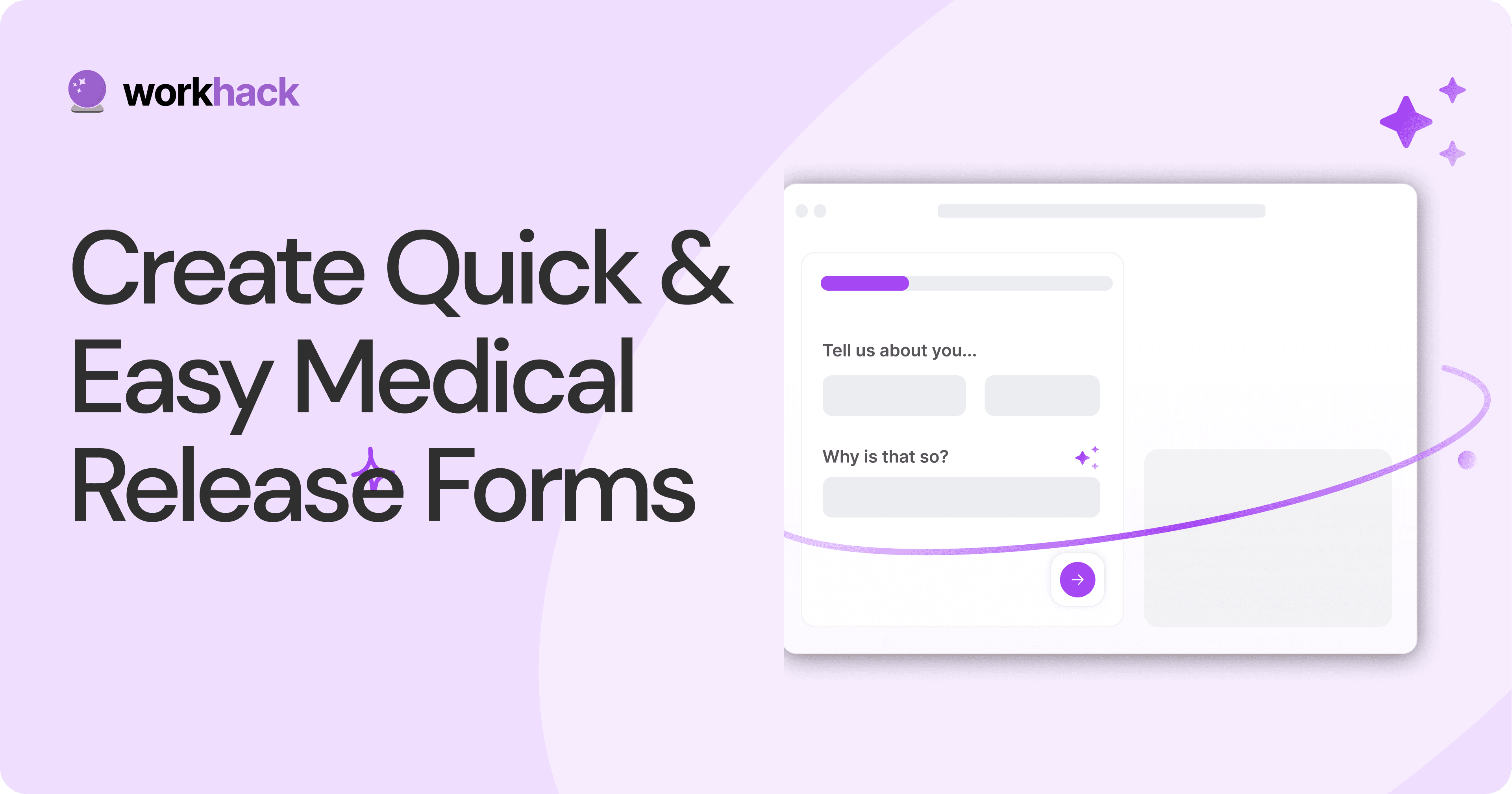
Build Quick and Easy Medical Release Forms.
Every HIPAA-compliant healthcare provider comes across medical release forms that involve details from medical history forms. Can they be shipped fast? Yes.
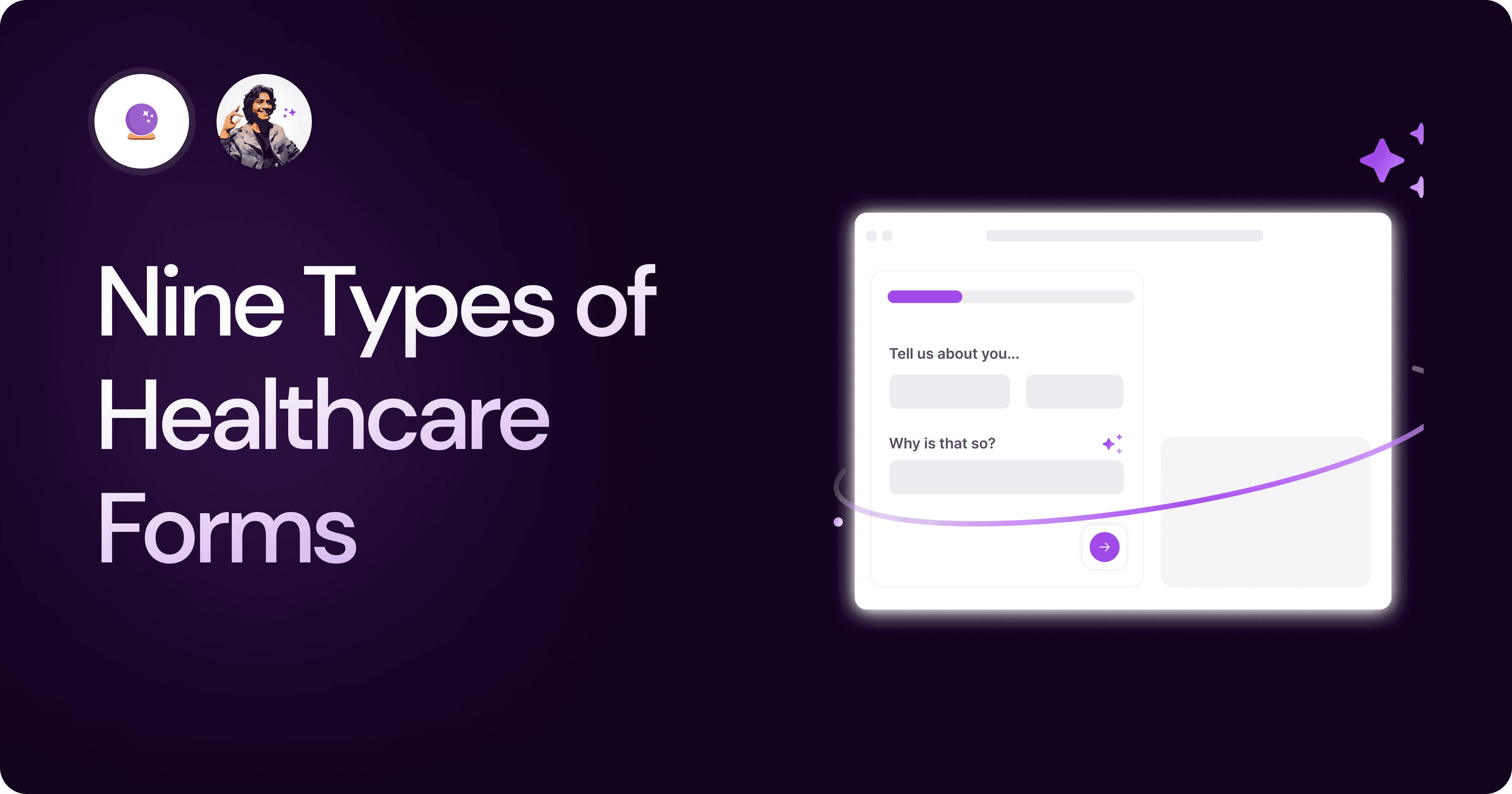
Nine Types of Healthcare and Medical Forms.
Medical forms are a must-have for any healthcare business or practitioner. Learn about the different kinds of medical and healthcare forms.
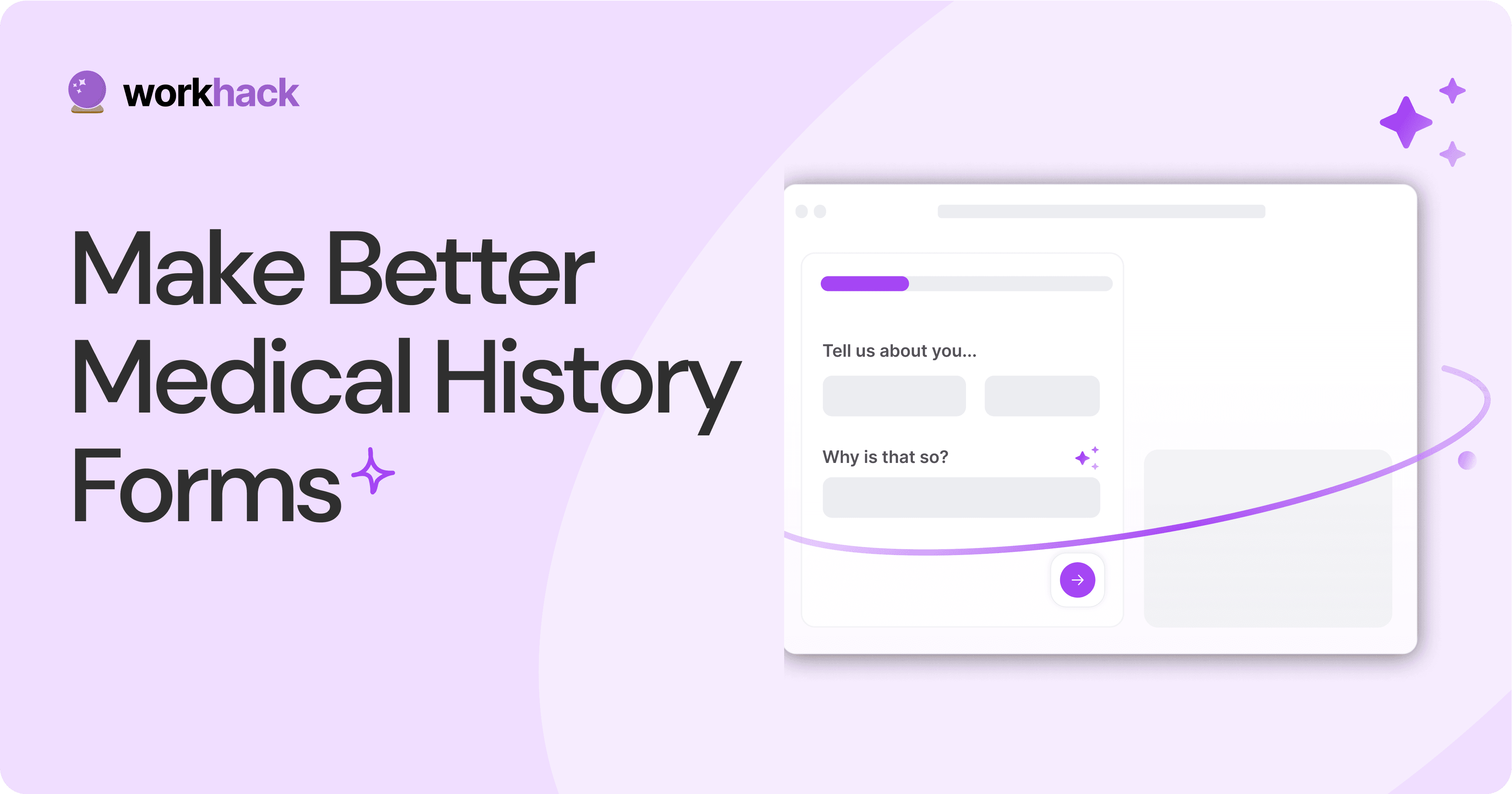
4 Tips for Better Medical History Forms.
Medical history forms are central to patient care, onboarding, and medical administration records. Learn how to make them easier to fill.
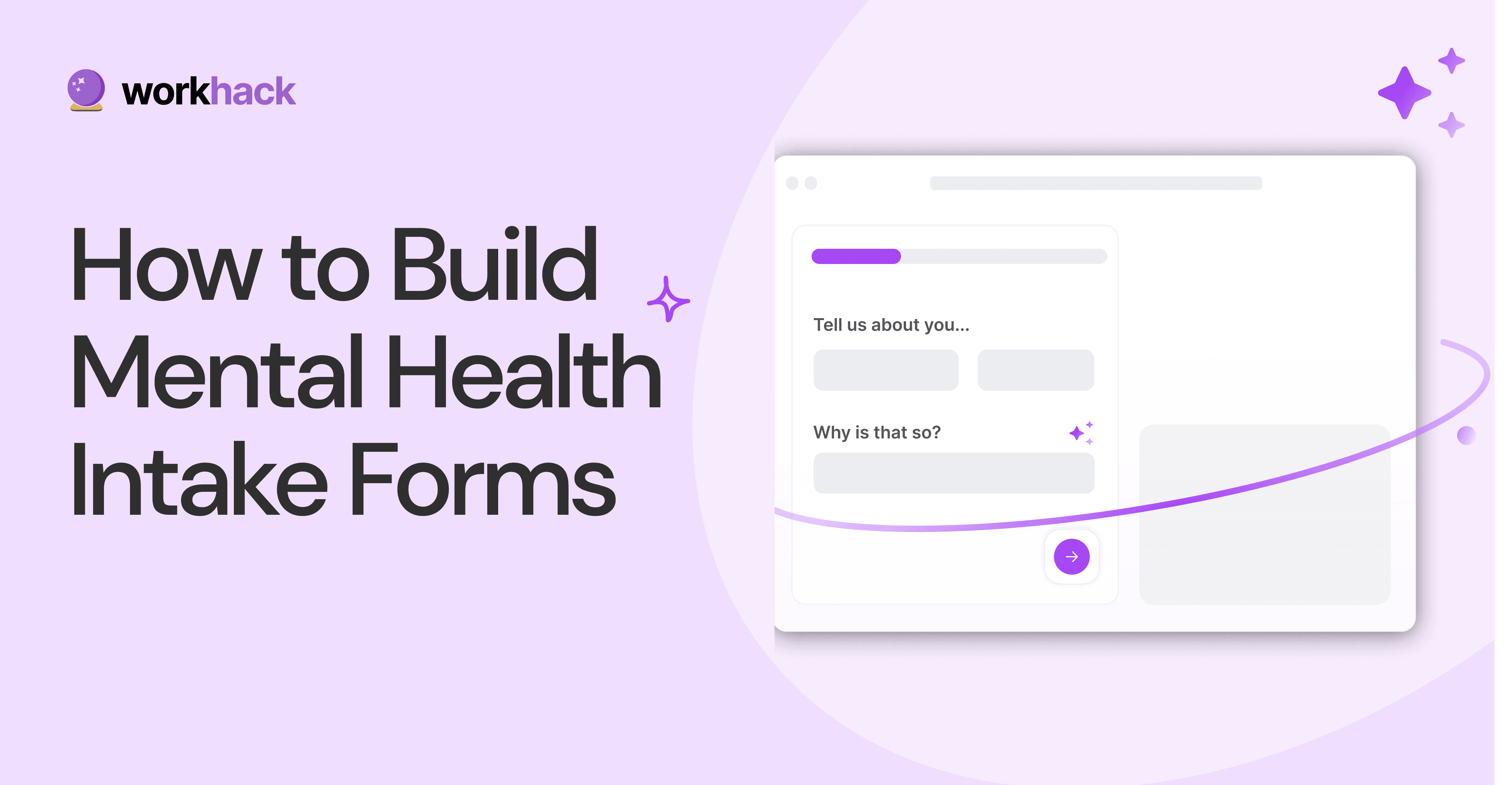
How to Build Mental Health Intake Forms?
Mental health intake forms are not like patient intake forms. Mental health intake forms deal with far more sensitive data and have specific design methods.
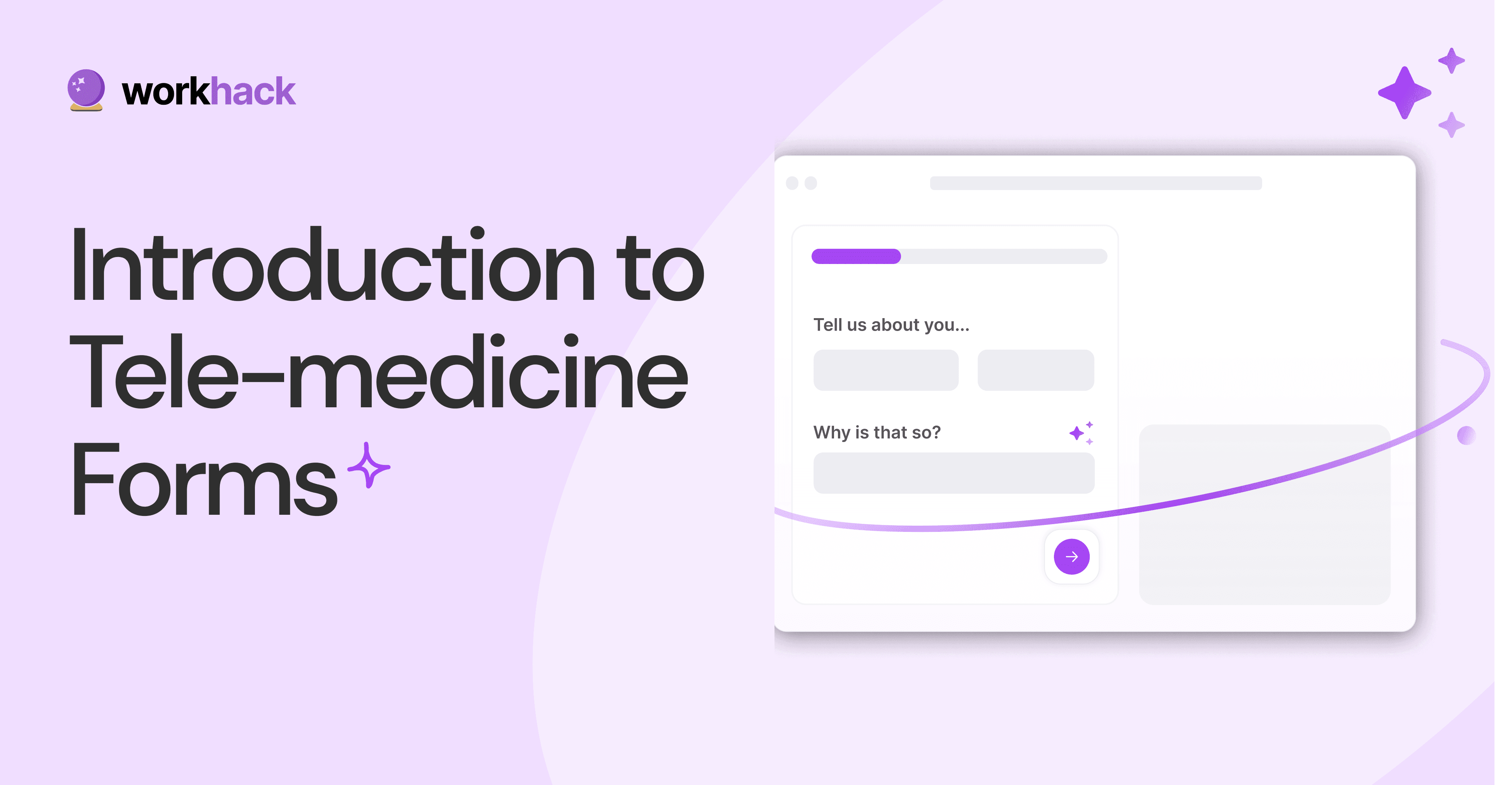
What, Why and How of Telemedicine Forms.
Telemedicine is on the rise and with different form builders out there, which one best suits your needs as a healthcare services provider?
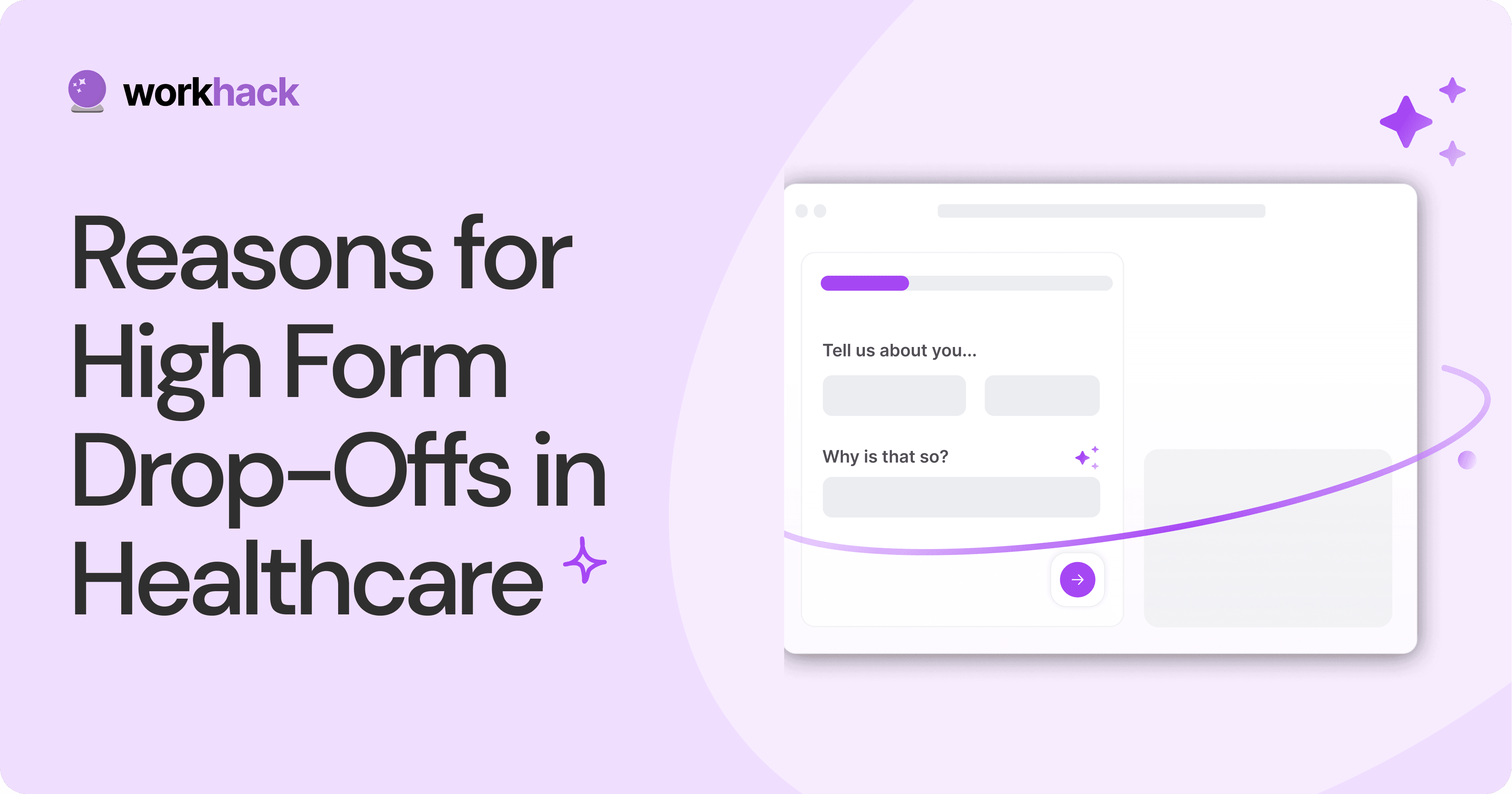
3 Reasons for Major Drop-Offs in Medical Forms.
No matter which healthcare form we pick, there are major drop-off reasons. We shall dive into the top 3 and learn how to resolve them in your next form.
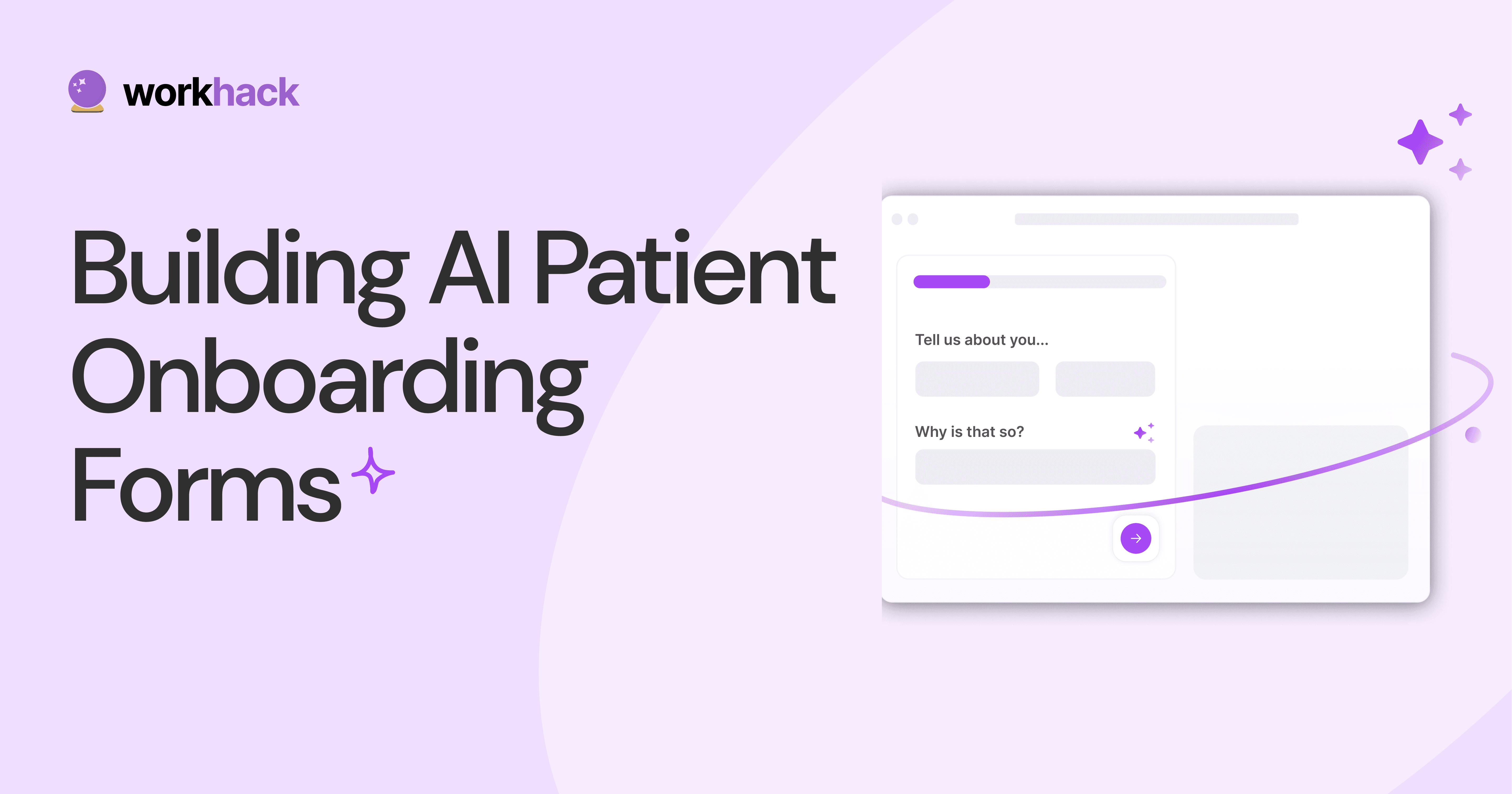
Patient Onboarding Forms - From Click to Clinic.
Patient onboarding forms are the first touchpoint for patients; getting this right for higher conversion rates is a must-have. Learn how to perfect them now.

5 Key Parts of a Good Patient Satisfaction Form.
The goal of patient satisfaction surveys is to course-correct the services of a healthcare provider. Patient feedback leads to a culture of patient-centric care.
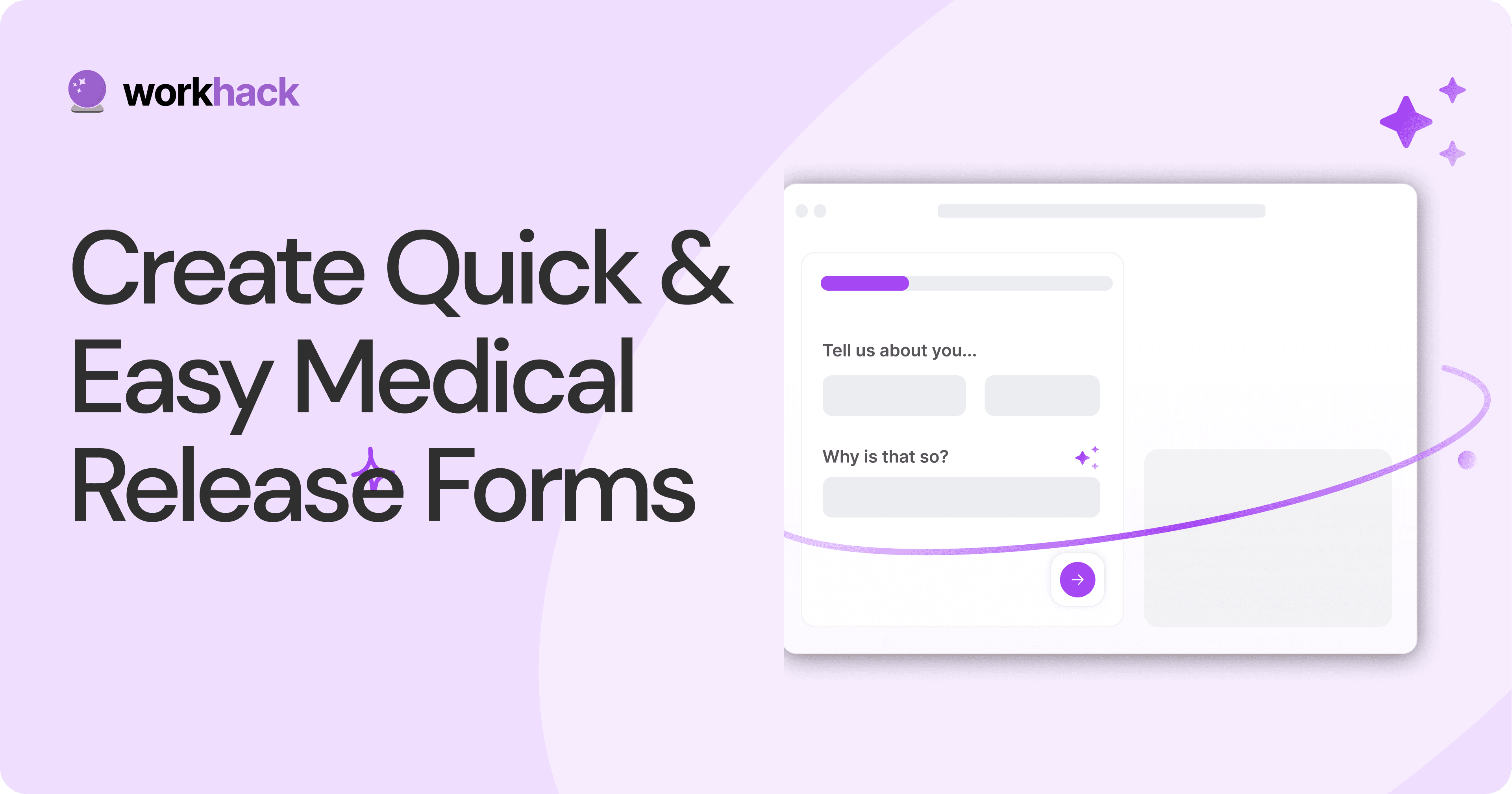
Build Quick and Easy Medical Release Forms.
Every HIPAA-compliant healthcare provider comes across medical release forms that involve details from medical history forms. Can they be shipped fast? Yes.
Subscribe to stay updated.
Subscribe to stay updated.
Subscribe to stay updated.
HC
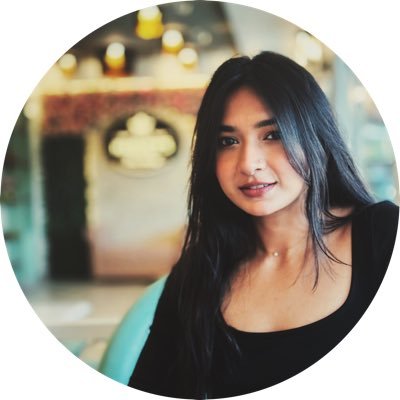
HC
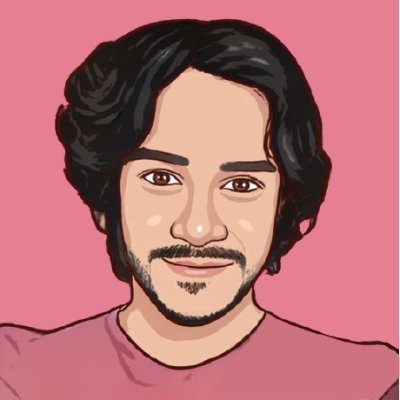
HC
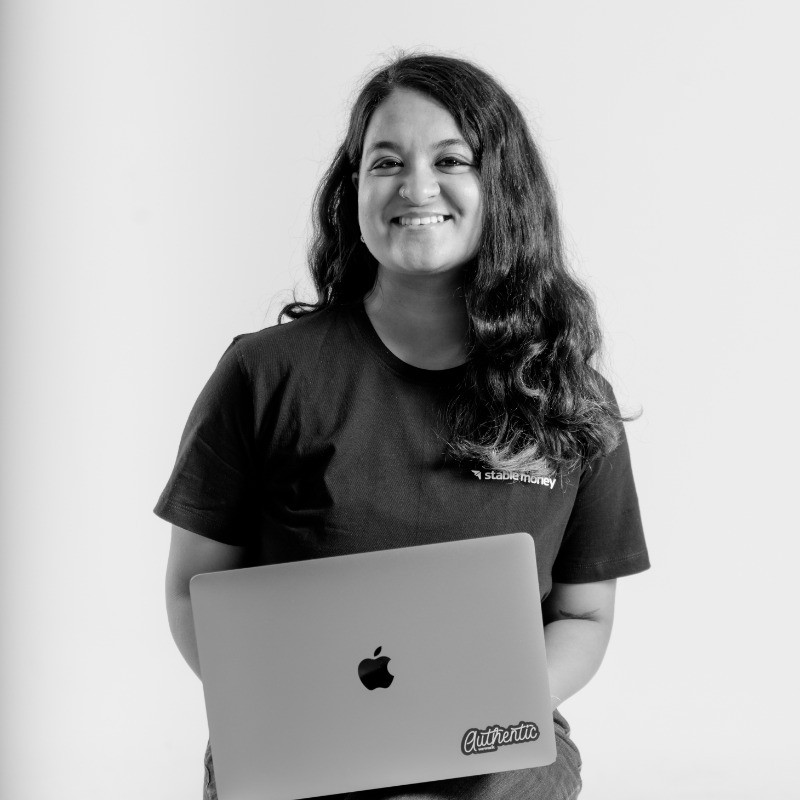
HC
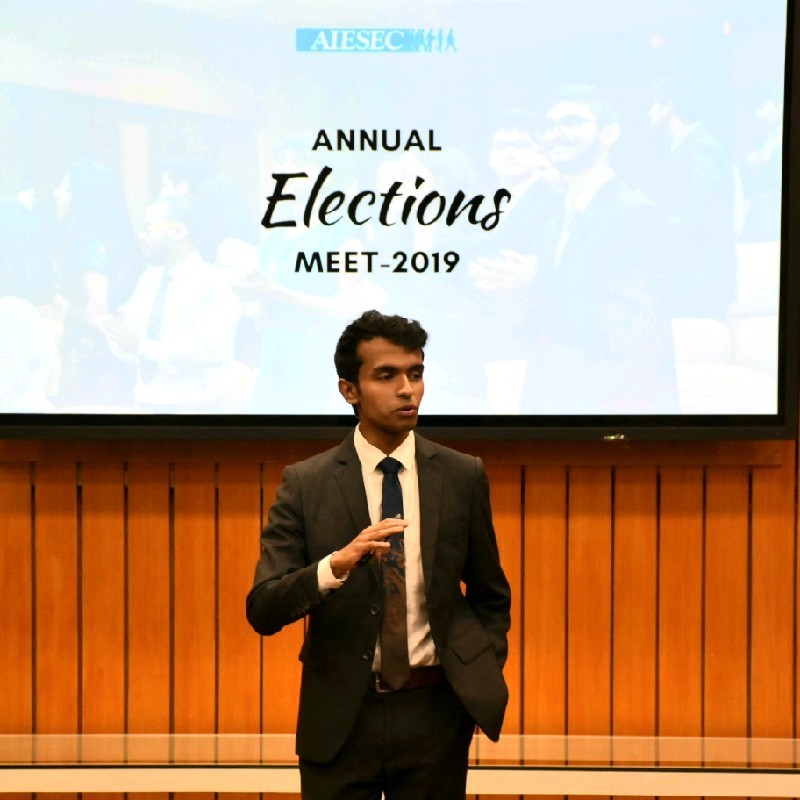
70+ people from across industries read our emails.
HC
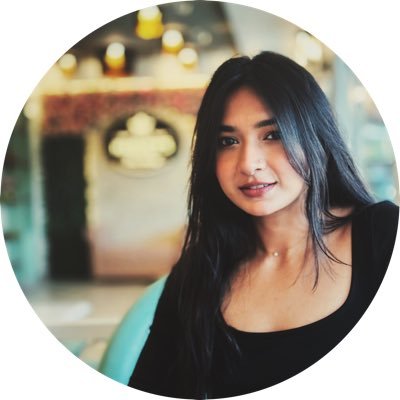
HC
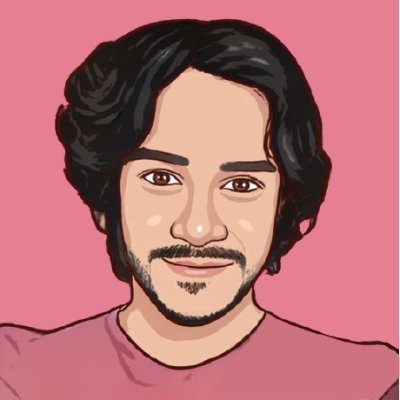
70+ people from across industries read our emails.
HC
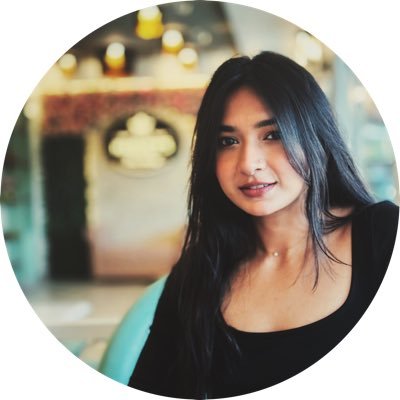
HC
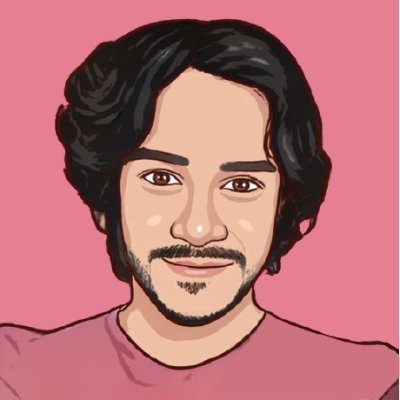
HC
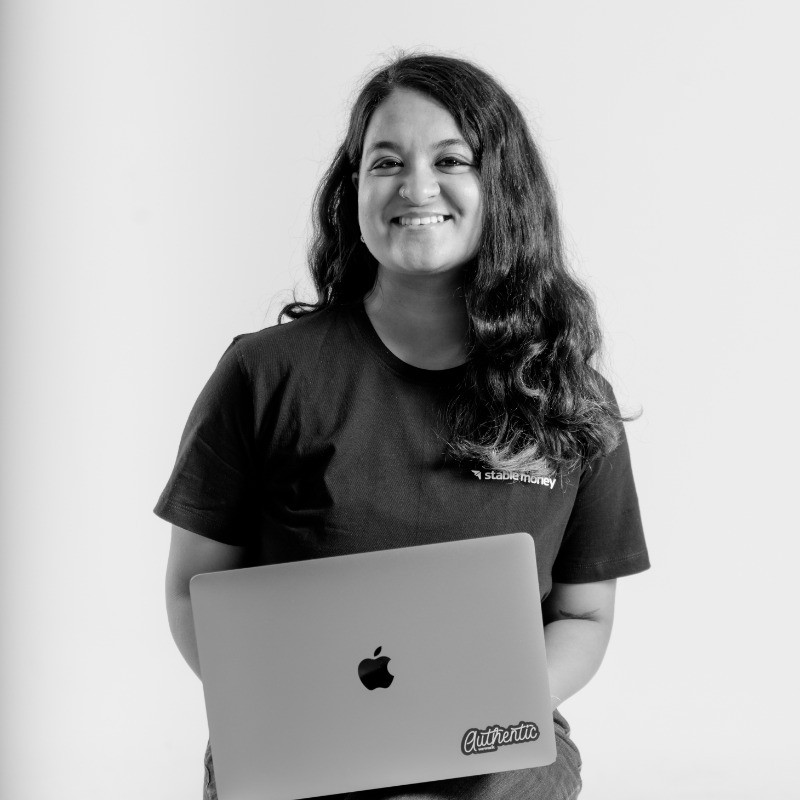
70+ people from across industries read our emails.
Bangalore, India / San Francisco, US
WorkHack Inc. 2023
Bangalore, India
San Francisco, US
WorkHack Inc. 2023
WorkHack Inc. 2023
Bangalore, India / San Francisco, US
WorkHack Inc. 2023
Bangalore, India / San Francisco, US