Challenges of Qualitative Market Research and Large Language Models
Challenges of Qualitative Market Research and Large Language Models
Challenges of Qualitative Market Research and Large Language Models



Introduction
In the dynamic landscape of market research, qualitative insights have long been a cornerstone for understanding consumer behavior, preferences, and motivations. Traditional methods like focus groups, in-depth interviews, and open-ended surveys have provided rich, nuanced data but often pose challenges in terms of scalability, consistency, and speed. Enter Large Language Models (LLMs) – the cutting-edge technology transforming how qualitative market research is conducted. AI-native survey builders like Metaforms leverage LLMs using vast datasets to predict and generate text, offering innovative solutions to age-old research hurdles. They promise to enhance efficiency, accuracy, and depth in qualitative research, but also bring their own set of challenges and considerations. Let’s explore how LLMs are reshaping qualitative market research, their practical applications, and the potential pitfalls to be aware of in this evolving field.
What are Large Language Models (LLMs)?
Large Language Models (LLMs) are sophisticated AI systems designed to predict and generate human-like text based on vast amounts of data. These models, like OpenAI’s ChatGPT or Google’s Bard, use deep learning techniques to understand and generate language by identifying patterns, contexts, and relationships within massive datasets. Unlike traditional algorithms, LLMs can perform a wide range of language-related tasks, from text completion to translation, and even content creation, making them powerful tools for various applications, including market research.
How Do LLMs Impact Qualitative Market Research?
LLMs transform qualitative market research by automating and enhancing processes traditionally reliant on human interpretation and analysis. Here’s how:
1. Data Summarization and Analysis: LLMs can quickly process and summarize large volumes of qualitative data, such as open-ended survey responses, focus group transcripts, and interview notes. This accelerates the research process and provides researchers with concise, actionable insights.
2. Automated Reporting: LLMs can generate reports by organizing qualitative data into coherent narratives. They can draft executive summaries, identify key themes, and highlight significant findings, reducing the manual workload on researchers.
3. Theme and Sentiment Identification: By analyzing patterns in text, LLMs can identify underlying themes, assess sentiment, and gauge brand perceptions. This allows researchers to gain a deeper understanding of consumer attitudes and emotions.
4. Predictive Insights: LLMs can extract embeddings (mathematical representations of text) that can be used in predictive models. For instance, analyzing customer feedback to predict future behavior, such as churn or brand loyalty, provides valuable foresight for strategic planning.
Practical Applications and Use Cases
1. Summarization of Survey Data
Use Case: A global beverage company conducts open-ended surveys across multiple regions to understand consumer preferences. LLMs summarize responses, highlighting regional differences and common themes.
Benefit: Streamlines data analysis, enabling quicker identification of key consumer insights without manual coding.
2. Automated Reporting for Qualitative Data
Use Case: A retail brand conducts a series of focus groups to explore customer reactions to a new product line. LLMs generate initial drafts of reports, including theme identification and participant quotes.
Benefit: Saves time in report preparation, allowing researchers to focus on refining insights and recommendations.
3. Theme and Sentiment Analysis in Social Media Monitoring
Use Case: A fashion brand monitors social media discussions to gauge sentiment about their latest collection. LLMs analyze posts and comments, identifying trends in sentiment and prevalent themes.
Benefit: Provides real-time insights into consumer perceptions, aiding in timely adjustments to marketing strategies.
4. Predictive Modeling of Customer Feedback
Use Case: A telecommunications company uses LLMs to analyze customer service transcripts, predicting potential churn based on the tone and content of conversations.
Benefit: Helps in proactive retention efforts by identifying at-risk customers through qualitative feedback analysis.
Challenges in Understanding and Implementing LLMs
1. Data Quality and Bias: LLMs rely on the quality of the data they are trained on. Poor-quality or biased data can lead to inaccurate predictions and insights. Researchers must ensure that the data used is representative and free from significant biases.
2. Interpretation of Complex Sentiments: While LLMs can identify sentiment, understanding the nuance and context of human emotions in complex topics remains challenging. They may struggle with sarcasm, irony, or cultural subtleties.
3. Risk of Hallucinations: LLMs can generate plausible-sounding but incorrect or fabricated information, known as "hallucinations." This poses a risk when relying on AI-generated insights for critical business decisions.
4. Ethical and Legal Considerations: Using LLMs involves handling sensitive data and intellectual property concerns. Researchers must navigate ethical considerations and ensure compliance with data privacy regulations.
Best Practices for Using LLMs in Qualitative Market Research
1. Combining AI with Human Expertise: Use LLMs to handle large-scale data analysis and reporting, but rely on human researchers for interpreting nuanced findings and making strategic decisions.
2. Ensuring Data Quality: Regularly validate and clean training data to minimize biases and ensure accurate, reliable insights.
3. Implementing Ethical Guidelines: Develop and adhere to ethical guidelines for using LLMs, including data privacy, transparency, and the responsible use of AI-generated content.
4. Continuous Learning and Adaptation: Stay updated with advancements in AI and LLMs. Adapt practices and methodologies to incorporate new capabilities and address emerging challenges.
Model Survey for Qualitative Market Research
Title: Understanding Consumer Preferences for Sustainable Products
Section 1: Demographics
1. What is your age group? [18-24, 25-34, 35-44, 45-54, 55+]
2. What is your gender? [Male, Female, Non-binary, Prefer not to say]
3. Where are you located? [Dropdown list of regions/countries]
Section 2: Consumer Preferences
1. What factors influence your decision to purchase sustainable products?
- Open-ended response
2. Describe a recent experience where you chose a sustainable product over a conventional one. What motivated your choice?
- Open-ended response
3. How important is product sustainability to you compared to other factors like price and quality? Why?
- Open-ended response
Section 3: Brand Perception
1. Which brands do you associate with sustainability? Why?
- Open-ended response
2. How do you perceive the efforts of brands in promoting sustainable practices?
- Open-ended response
Conclusion: Qualitative Market Research and Large Language Models
Large Language Models represent a significant advancement in qualitative market research, offering powerful tools to enhance efficiency and depth of analysis. While they bring numerous benefits, including automated reporting, theme identification, and predictive insights, challenges such as data quality, interpretation nuances, and ethical considerations must be carefully managed.
Metaforms.ai, an AI-native qualitative survey builder integrates LLMs with traditional research methodologies and adhering to best practices, qualitative researchers can bridge the gap between outcomes and tactics, leading to more informed and effective market research strategies. In a rapidly evolving industry, embracing AI-driven tools while maintaining human oversight is key to unlocking the full potential of qualitative insights.
Introduction
In the dynamic landscape of market research, qualitative insights have long been a cornerstone for understanding consumer behavior, preferences, and motivations. Traditional methods like focus groups, in-depth interviews, and open-ended surveys have provided rich, nuanced data but often pose challenges in terms of scalability, consistency, and speed. Enter Large Language Models (LLMs) – the cutting-edge technology transforming how qualitative market research is conducted. AI-native survey builders like Metaforms leverage LLMs using vast datasets to predict and generate text, offering innovative solutions to age-old research hurdles. They promise to enhance efficiency, accuracy, and depth in qualitative research, but also bring their own set of challenges and considerations. Let’s explore how LLMs are reshaping qualitative market research, their practical applications, and the potential pitfalls to be aware of in this evolving field.
What are Large Language Models (LLMs)?
Large Language Models (LLMs) are sophisticated AI systems designed to predict and generate human-like text based on vast amounts of data. These models, like OpenAI’s ChatGPT or Google’s Bard, use deep learning techniques to understand and generate language by identifying patterns, contexts, and relationships within massive datasets. Unlike traditional algorithms, LLMs can perform a wide range of language-related tasks, from text completion to translation, and even content creation, making them powerful tools for various applications, including market research.
How Do LLMs Impact Qualitative Market Research?
LLMs transform qualitative market research by automating and enhancing processes traditionally reliant on human interpretation and analysis. Here’s how:
1. Data Summarization and Analysis: LLMs can quickly process and summarize large volumes of qualitative data, such as open-ended survey responses, focus group transcripts, and interview notes. This accelerates the research process and provides researchers with concise, actionable insights.
2. Automated Reporting: LLMs can generate reports by organizing qualitative data into coherent narratives. They can draft executive summaries, identify key themes, and highlight significant findings, reducing the manual workload on researchers.
3. Theme and Sentiment Identification: By analyzing patterns in text, LLMs can identify underlying themes, assess sentiment, and gauge brand perceptions. This allows researchers to gain a deeper understanding of consumer attitudes and emotions.
4. Predictive Insights: LLMs can extract embeddings (mathematical representations of text) that can be used in predictive models. For instance, analyzing customer feedback to predict future behavior, such as churn or brand loyalty, provides valuable foresight for strategic planning.
Practical Applications and Use Cases
1. Summarization of Survey Data
Use Case: A global beverage company conducts open-ended surveys across multiple regions to understand consumer preferences. LLMs summarize responses, highlighting regional differences and common themes.
Benefit: Streamlines data analysis, enabling quicker identification of key consumer insights without manual coding.
2. Automated Reporting for Qualitative Data
Use Case: A retail brand conducts a series of focus groups to explore customer reactions to a new product line. LLMs generate initial drafts of reports, including theme identification and participant quotes.
Benefit: Saves time in report preparation, allowing researchers to focus on refining insights and recommendations.
3. Theme and Sentiment Analysis in Social Media Monitoring
Use Case: A fashion brand monitors social media discussions to gauge sentiment about their latest collection. LLMs analyze posts and comments, identifying trends in sentiment and prevalent themes.
Benefit: Provides real-time insights into consumer perceptions, aiding in timely adjustments to marketing strategies.
4. Predictive Modeling of Customer Feedback
Use Case: A telecommunications company uses LLMs to analyze customer service transcripts, predicting potential churn based on the tone and content of conversations.
Benefit: Helps in proactive retention efforts by identifying at-risk customers through qualitative feedback analysis.
Challenges in Understanding and Implementing LLMs
1. Data Quality and Bias: LLMs rely on the quality of the data they are trained on. Poor-quality or biased data can lead to inaccurate predictions and insights. Researchers must ensure that the data used is representative and free from significant biases.
2. Interpretation of Complex Sentiments: While LLMs can identify sentiment, understanding the nuance and context of human emotions in complex topics remains challenging. They may struggle with sarcasm, irony, or cultural subtleties.
3. Risk of Hallucinations: LLMs can generate plausible-sounding but incorrect or fabricated information, known as "hallucinations." This poses a risk when relying on AI-generated insights for critical business decisions.
4. Ethical and Legal Considerations: Using LLMs involves handling sensitive data and intellectual property concerns. Researchers must navigate ethical considerations and ensure compliance with data privacy regulations.
Best Practices for Using LLMs in Qualitative Market Research
1. Combining AI with Human Expertise: Use LLMs to handle large-scale data analysis and reporting, but rely on human researchers for interpreting nuanced findings and making strategic decisions.
2. Ensuring Data Quality: Regularly validate and clean training data to minimize biases and ensure accurate, reliable insights.
3. Implementing Ethical Guidelines: Develop and adhere to ethical guidelines for using LLMs, including data privacy, transparency, and the responsible use of AI-generated content.
4. Continuous Learning and Adaptation: Stay updated with advancements in AI and LLMs. Adapt practices and methodologies to incorporate new capabilities and address emerging challenges.
Model Survey for Qualitative Market Research
Title: Understanding Consumer Preferences for Sustainable Products
Section 1: Demographics
1. What is your age group? [18-24, 25-34, 35-44, 45-54, 55+]
2. What is your gender? [Male, Female, Non-binary, Prefer not to say]
3. Where are you located? [Dropdown list of regions/countries]
Section 2: Consumer Preferences
1. What factors influence your decision to purchase sustainable products?
- Open-ended response
2. Describe a recent experience where you chose a sustainable product over a conventional one. What motivated your choice?
- Open-ended response
3. How important is product sustainability to you compared to other factors like price and quality? Why?
- Open-ended response
Section 3: Brand Perception
1. Which brands do you associate with sustainability? Why?
- Open-ended response
2. How do you perceive the efforts of brands in promoting sustainable practices?
- Open-ended response
Conclusion: Qualitative Market Research and Large Language Models
Large Language Models represent a significant advancement in qualitative market research, offering powerful tools to enhance efficiency and depth of analysis. While they bring numerous benefits, including automated reporting, theme identification, and predictive insights, challenges such as data quality, interpretation nuances, and ethical considerations must be carefully managed.
Metaforms.ai, an AI-native qualitative survey builder integrates LLMs with traditional research methodologies and adhering to best practices, qualitative researchers can bridge the gap between outcomes and tactics, leading to more informed and effective market research strategies. In a rapidly evolving industry, embracing AI-driven tools while maintaining human oversight is key to unlocking the full potential of qualitative insights.
Introduction
In the dynamic landscape of market research, qualitative insights have long been a cornerstone for understanding consumer behavior, preferences, and motivations. Traditional methods like focus groups, in-depth interviews, and open-ended surveys have provided rich, nuanced data but often pose challenges in terms of scalability, consistency, and speed. Enter Large Language Models (LLMs) – the cutting-edge technology transforming how qualitative market research is conducted. AI-native survey builders like Metaforms leverage LLMs using vast datasets to predict and generate text, offering innovative solutions to age-old research hurdles. They promise to enhance efficiency, accuracy, and depth in qualitative research, but also bring their own set of challenges and considerations. Let’s explore how LLMs are reshaping qualitative market research, their practical applications, and the potential pitfalls to be aware of in this evolving field.
What are Large Language Models (LLMs)?
Large Language Models (LLMs) are sophisticated AI systems designed to predict and generate human-like text based on vast amounts of data. These models, like OpenAI’s ChatGPT or Google’s Bard, use deep learning techniques to understand and generate language by identifying patterns, contexts, and relationships within massive datasets. Unlike traditional algorithms, LLMs can perform a wide range of language-related tasks, from text completion to translation, and even content creation, making them powerful tools for various applications, including market research.
How Do LLMs Impact Qualitative Market Research?
LLMs transform qualitative market research by automating and enhancing processes traditionally reliant on human interpretation and analysis. Here’s how:
1. Data Summarization and Analysis: LLMs can quickly process and summarize large volumes of qualitative data, such as open-ended survey responses, focus group transcripts, and interview notes. This accelerates the research process and provides researchers with concise, actionable insights.
2. Automated Reporting: LLMs can generate reports by organizing qualitative data into coherent narratives. They can draft executive summaries, identify key themes, and highlight significant findings, reducing the manual workload on researchers.
3. Theme and Sentiment Identification: By analyzing patterns in text, LLMs can identify underlying themes, assess sentiment, and gauge brand perceptions. This allows researchers to gain a deeper understanding of consumer attitudes and emotions.
4. Predictive Insights: LLMs can extract embeddings (mathematical representations of text) that can be used in predictive models. For instance, analyzing customer feedback to predict future behavior, such as churn or brand loyalty, provides valuable foresight for strategic planning.
Practical Applications and Use Cases
1. Summarization of Survey Data
Use Case: A global beverage company conducts open-ended surveys across multiple regions to understand consumer preferences. LLMs summarize responses, highlighting regional differences and common themes.
Benefit: Streamlines data analysis, enabling quicker identification of key consumer insights without manual coding.
2. Automated Reporting for Qualitative Data
Use Case: A retail brand conducts a series of focus groups to explore customer reactions to a new product line. LLMs generate initial drafts of reports, including theme identification and participant quotes.
Benefit: Saves time in report preparation, allowing researchers to focus on refining insights and recommendations.
3. Theme and Sentiment Analysis in Social Media Monitoring
Use Case: A fashion brand monitors social media discussions to gauge sentiment about their latest collection. LLMs analyze posts and comments, identifying trends in sentiment and prevalent themes.
Benefit: Provides real-time insights into consumer perceptions, aiding in timely adjustments to marketing strategies.
4. Predictive Modeling of Customer Feedback
Use Case: A telecommunications company uses LLMs to analyze customer service transcripts, predicting potential churn based on the tone and content of conversations.
Benefit: Helps in proactive retention efforts by identifying at-risk customers through qualitative feedback analysis.
Challenges in Understanding and Implementing LLMs
1. Data Quality and Bias: LLMs rely on the quality of the data they are trained on. Poor-quality or biased data can lead to inaccurate predictions and insights. Researchers must ensure that the data used is representative and free from significant biases.
2. Interpretation of Complex Sentiments: While LLMs can identify sentiment, understanding the nuance and context of human emotions in complex topics remains challenging. They may struggle with sarcasm, irony, or cultural subtleties.
3. Risk of Hallucinations: LLMs can generate plausible-sounding but incorrect or fabricated information, known as "hallucinations." This poses a risk when relying on AI-generated insights for critical business decisions.
4. Ethical and Legal Considerations: Using LLMs involves handling sensitive data and intellectual property concerns. Researchers must navigate ethical considerations and ensure compliance with data privacy regulations.
Best Practices for Using LLMs in Qualitative Market Research
1. Combining AI with Human Expertise: Use LLMs to handle large-scale data analysis and reporting, but rely on human researchers for interpreting nuanced findings and making strategic decisions.
2. Ensuring Data Quality: Regularly validate and clean training data to minimize biases and ensure accurate, reliable insights.
3. Implementing Ethical Guidelines: Develop and adhere to ethical guidelines for using LLMs, including data privacy, transparency, and the responsible use of AI-generated content.
4. Continuous Learning and Adaptation: Stay updated with advancements in AI and LLMs. Adapt practices and methodologies to incorporate new capabilities and address emerging challenges.
Model Survey for Qualitative Market Research
Title: Understanding Consumer Preferences for Sustainable Products
Section 1: Demographics
1. What is your age group? [18-24, 25-34, 35-44, 45-54, 55+]
2. What is your gender? [Male, Female, Non-binary, Prefer not to say]
3. Where are you located? [Dropdown list of regions/countries]
Section 2: Consumer Preferences
1. What factors influence your decision to purchase sustainable products?
- Open-ended response
2. Describe a recent experience where you chose a sustainable product over a conventional one. What motivated your choice?
- Open-ended response
3. How important is product sustainability to you compared to other factors like price and quality? Why?
- Open-ended response
Section 3: Brand Perception
1. Which brands do you associate with sustainability? Why?
- Open-ended response
2. How do you perceive the efforts of brands in promoting sustainable practices?
- Open-ended response
Conclusion: Qualitative Market Research and Large Language Models
Large Language Models represent a significant advancement in qualitative market research, offering powerful tools to enhance efficiency and depth of analysis. While they bring numerous benefits, including automated reporting, theme identification, and predictive insights, challenges such as data quality, interpretation nuances, and ethical considerations must be carefully managed.
Metaforms.ai, an AI-native qualitative survey builder integrates LLMs with traditional research methodologies and adhering to best practices, qualitative researchers can bridge the gap between outcomes and tactics, leading to more informed and effective market research strategies. In a rapidly evolving industry, embracing AI-driven tools while maintaining human oversight is key to unlocking the full potential of qualitative insights.
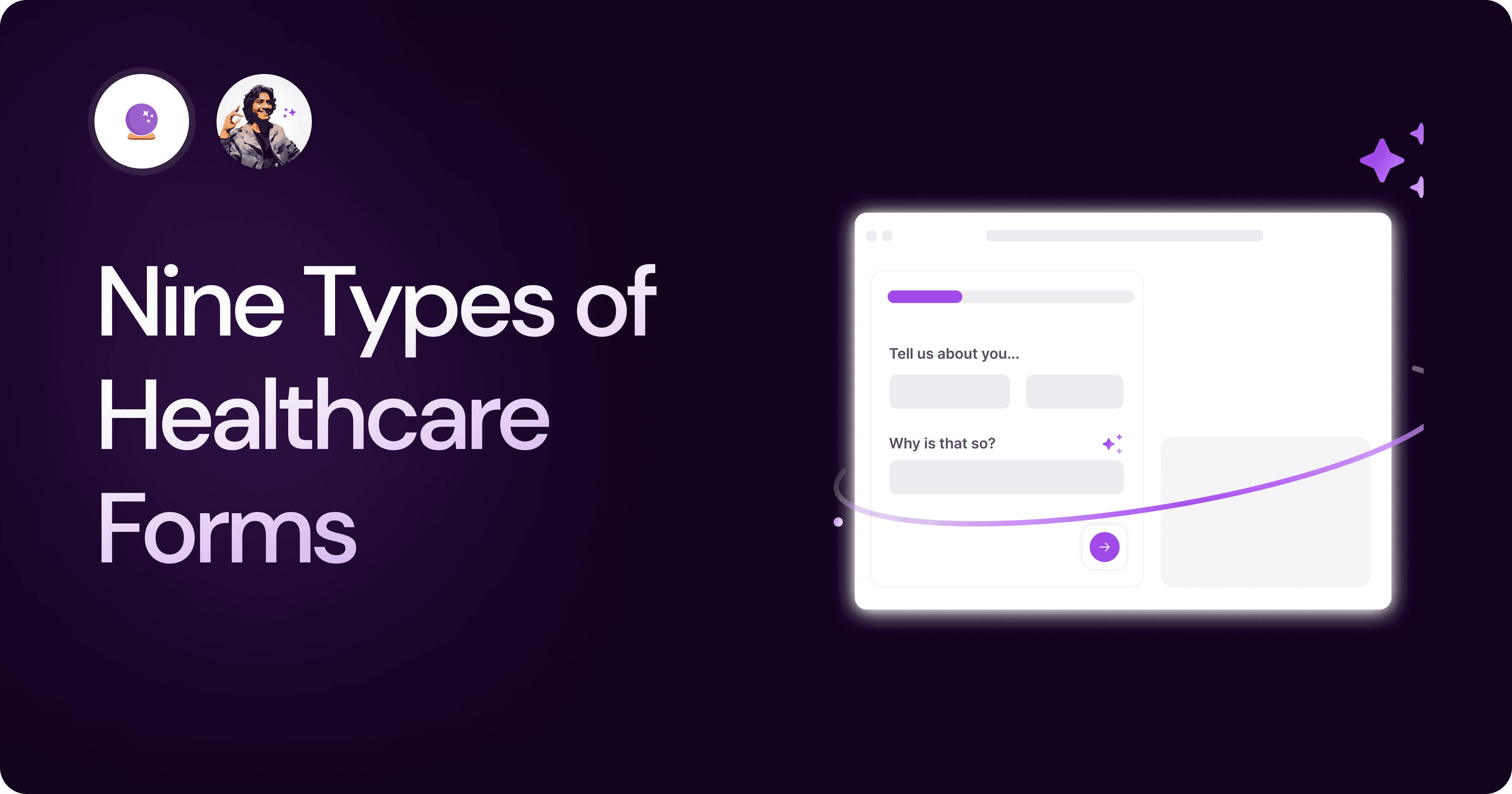
Nine Types of Healthcare and Medical Forms.
Medical forms are a must-have for any healthcare business or practitioner. Learn about the different kinds of medical and healthcare forms.
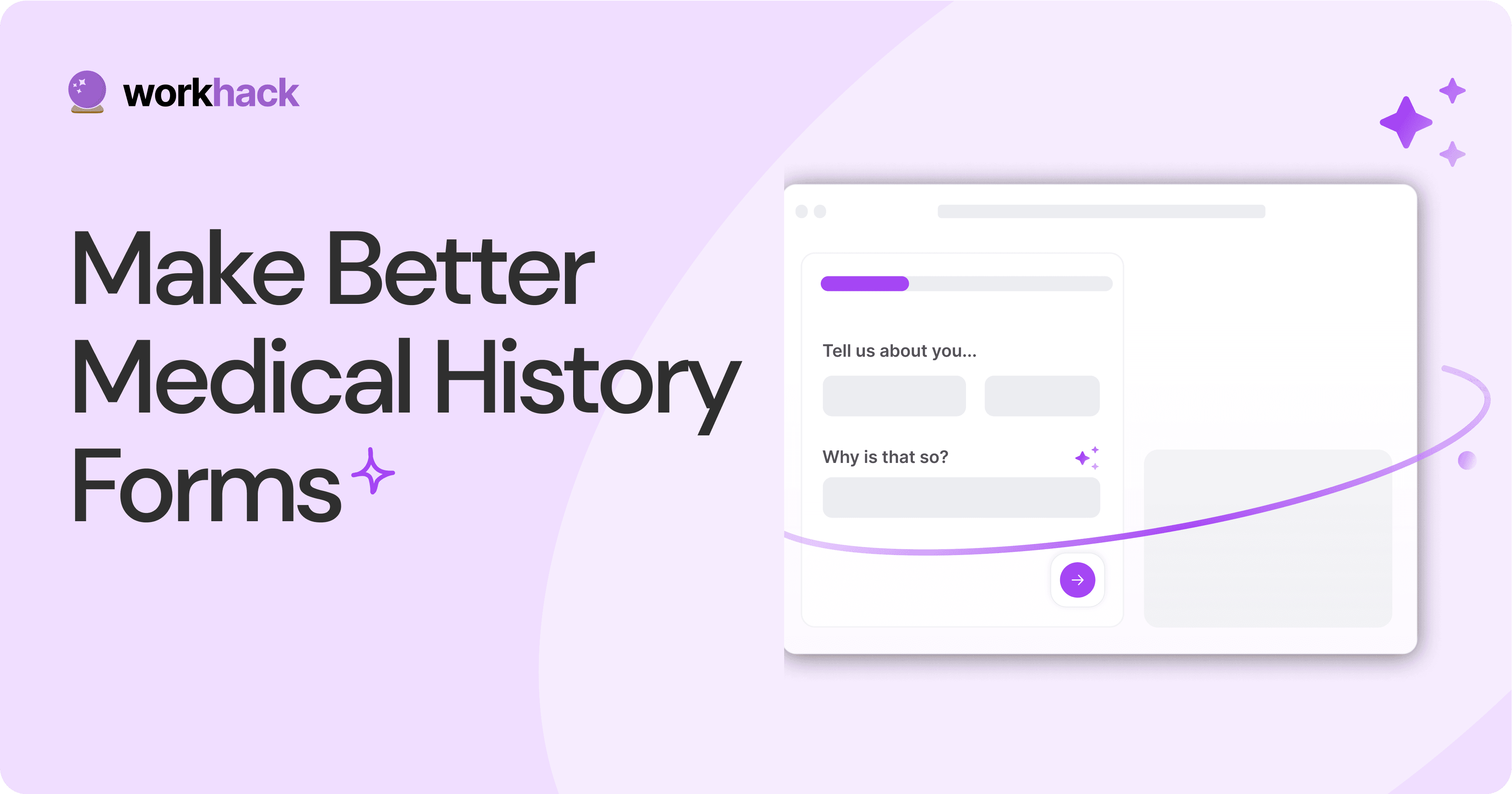
4 Tips for Better Medical History Forms.
Medical history forms are central to patient care, onboarding, and medical administration records. Learn how to make them easier to fill.
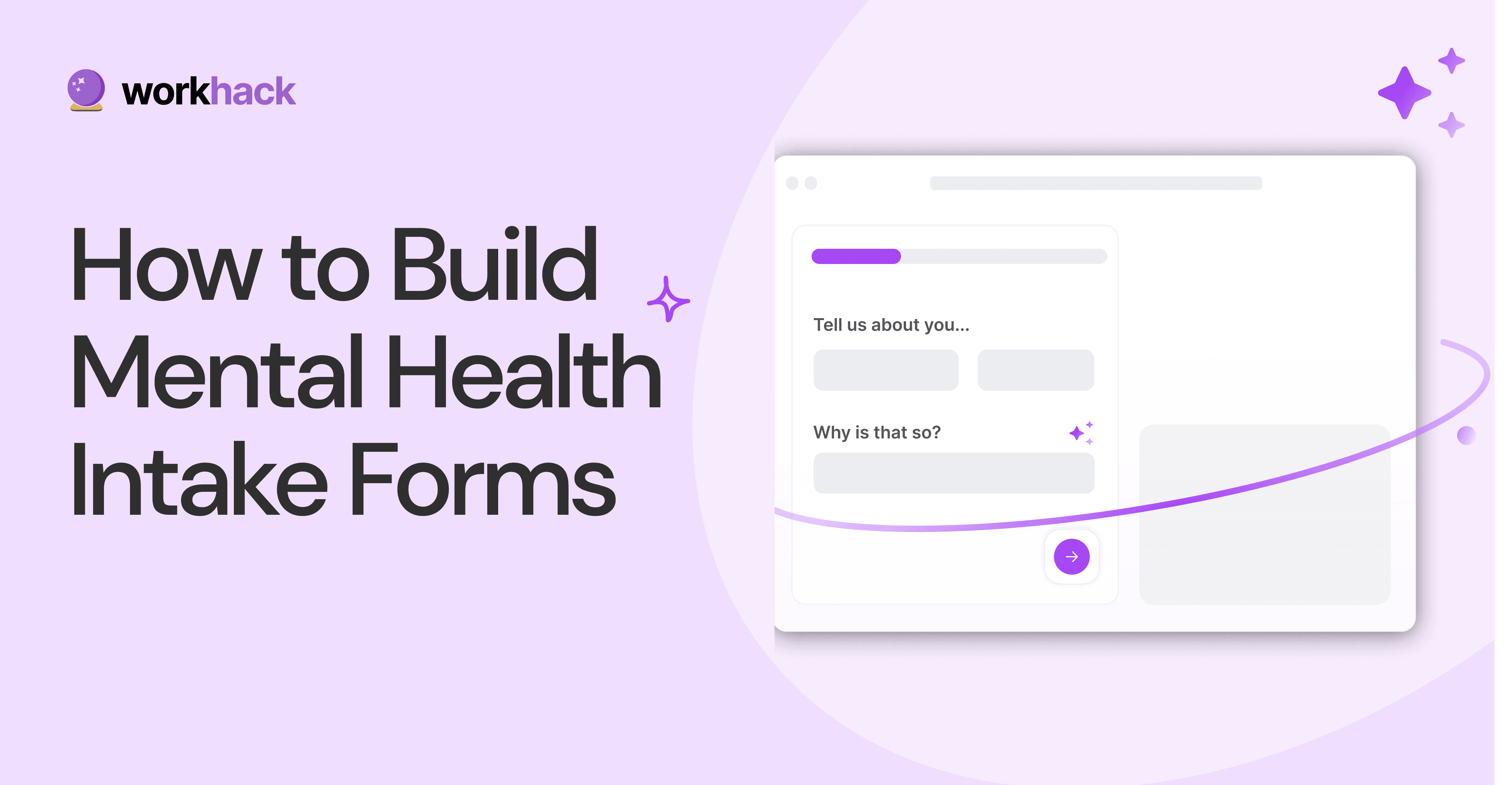
How to Build Mental Health Intake Forms?
Mental health intake forms are not like patient intake forms. Mental health intake forms deal with far more sensitive data and have specific design methods.
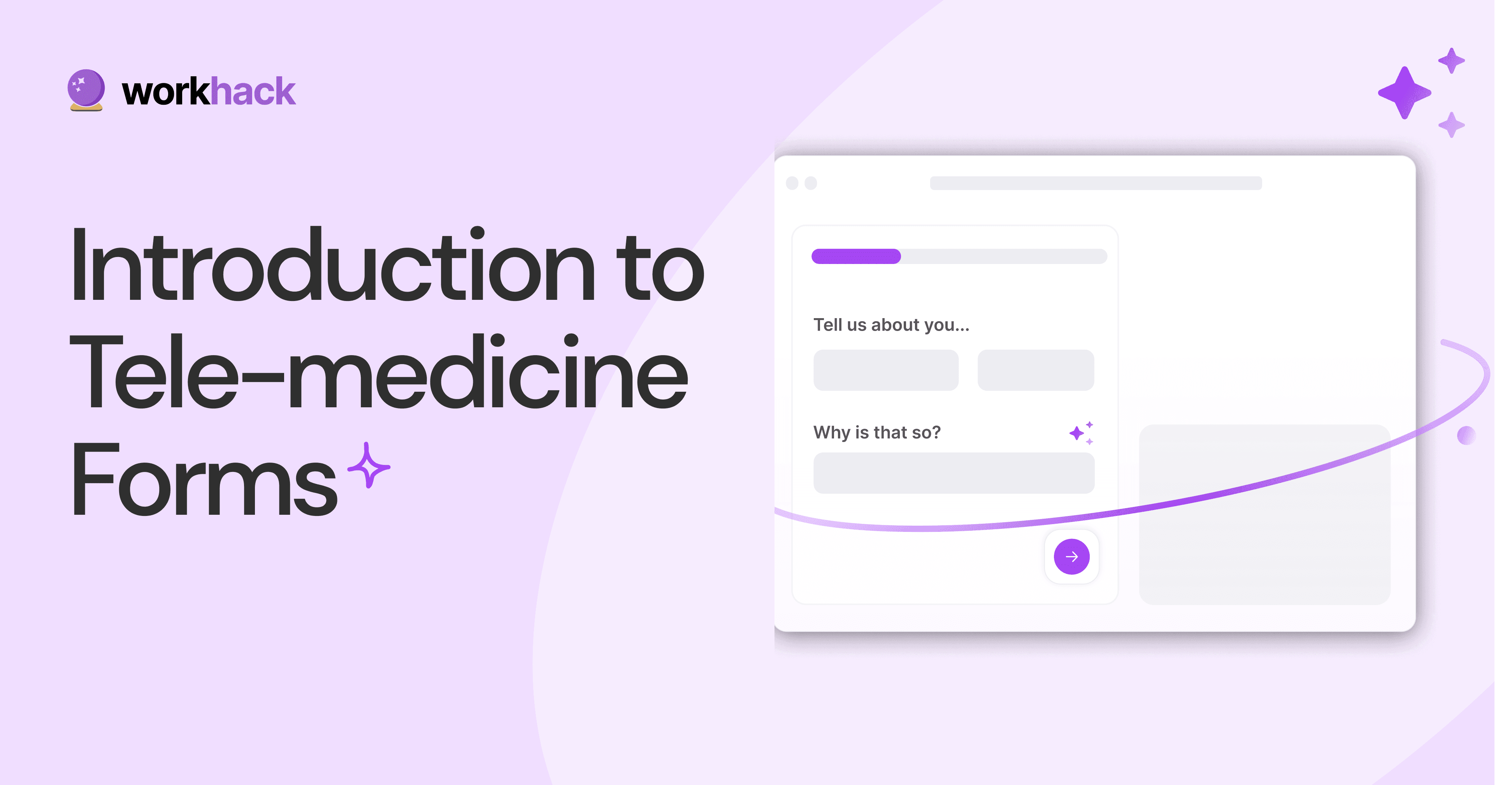
What, Why and How of Telemedicine Forms.
Telemedicine is on the rise and with different form builders out there, which one best suits your needs as a healthcare services provider?
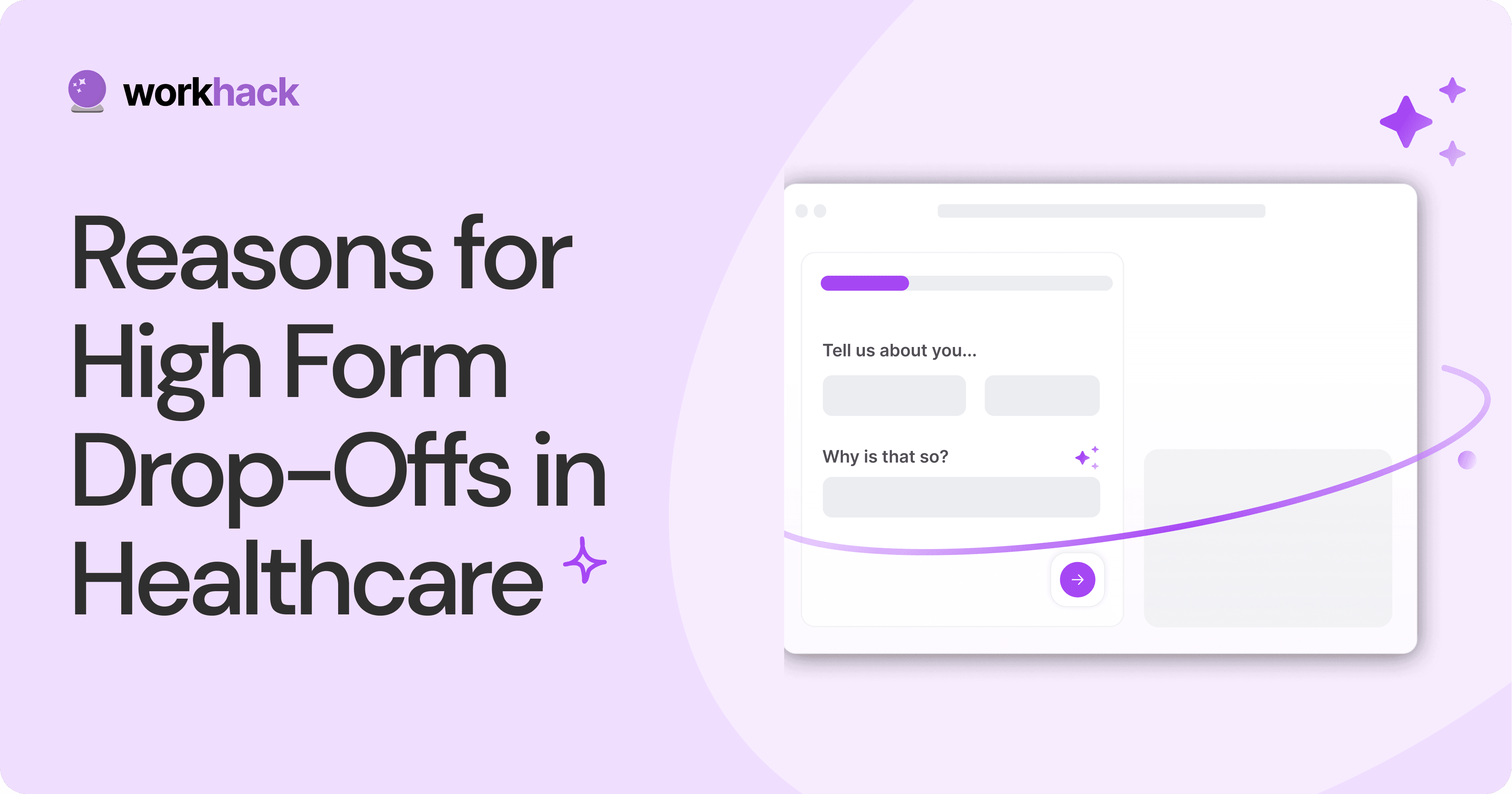
3 Reasons for Major Drop-Offs in Medical Forms.
No matter which healthcare form we pick, there are major drop-off reasons. We shall dive into the top 3 and learn how to resolve them in your next form.
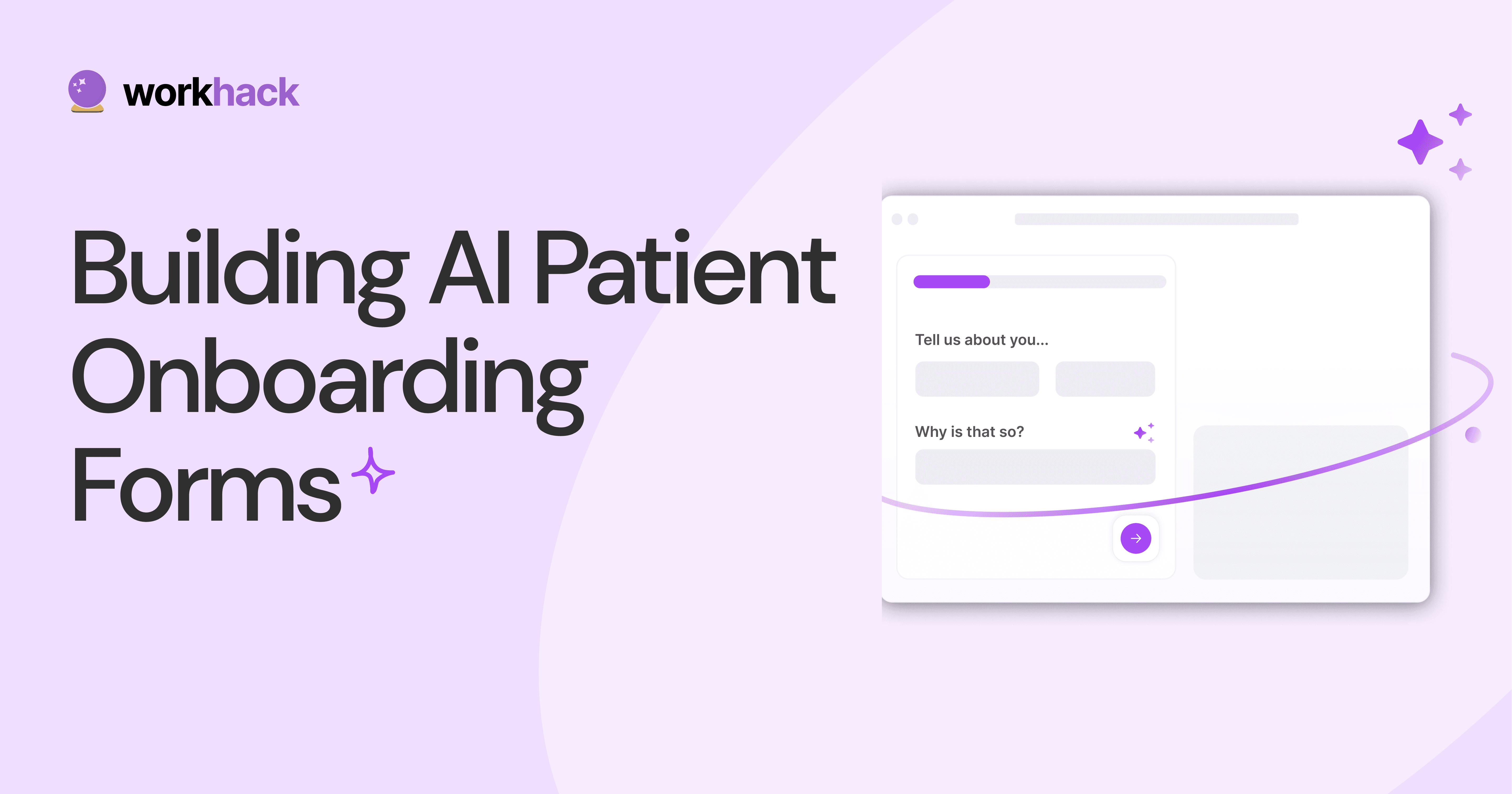
Patient Onboarding Forms - From Click to Clinic.
Patient onboarding forms are the first touchpoint for patients; getting this right for higher conversion rates is a must-have. Learn how to perfect them now.

5 Key Parts of a Good Patient Satisfaction Form.
The goal of patient satisfaction surveys is to course-correct the services of a healthcare provider. Patient feedback leads to a culture of patient-centric care.
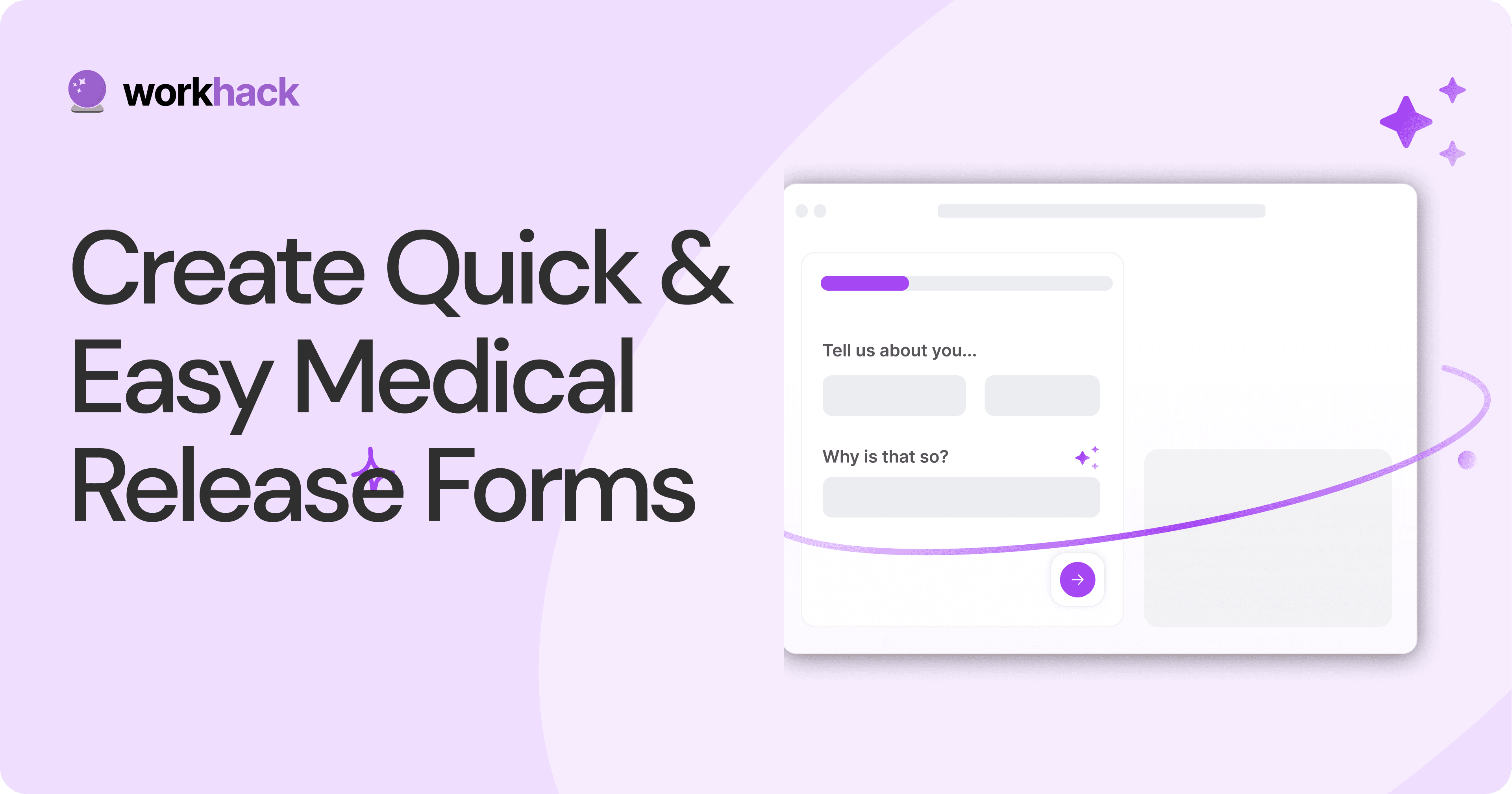
Build Quick and Easy Medical Release Forms.
Every HIPAA-compliant healthcare provider comes across medical release forms that involve details from medical history forms. Can they be shipped fast? Yes.
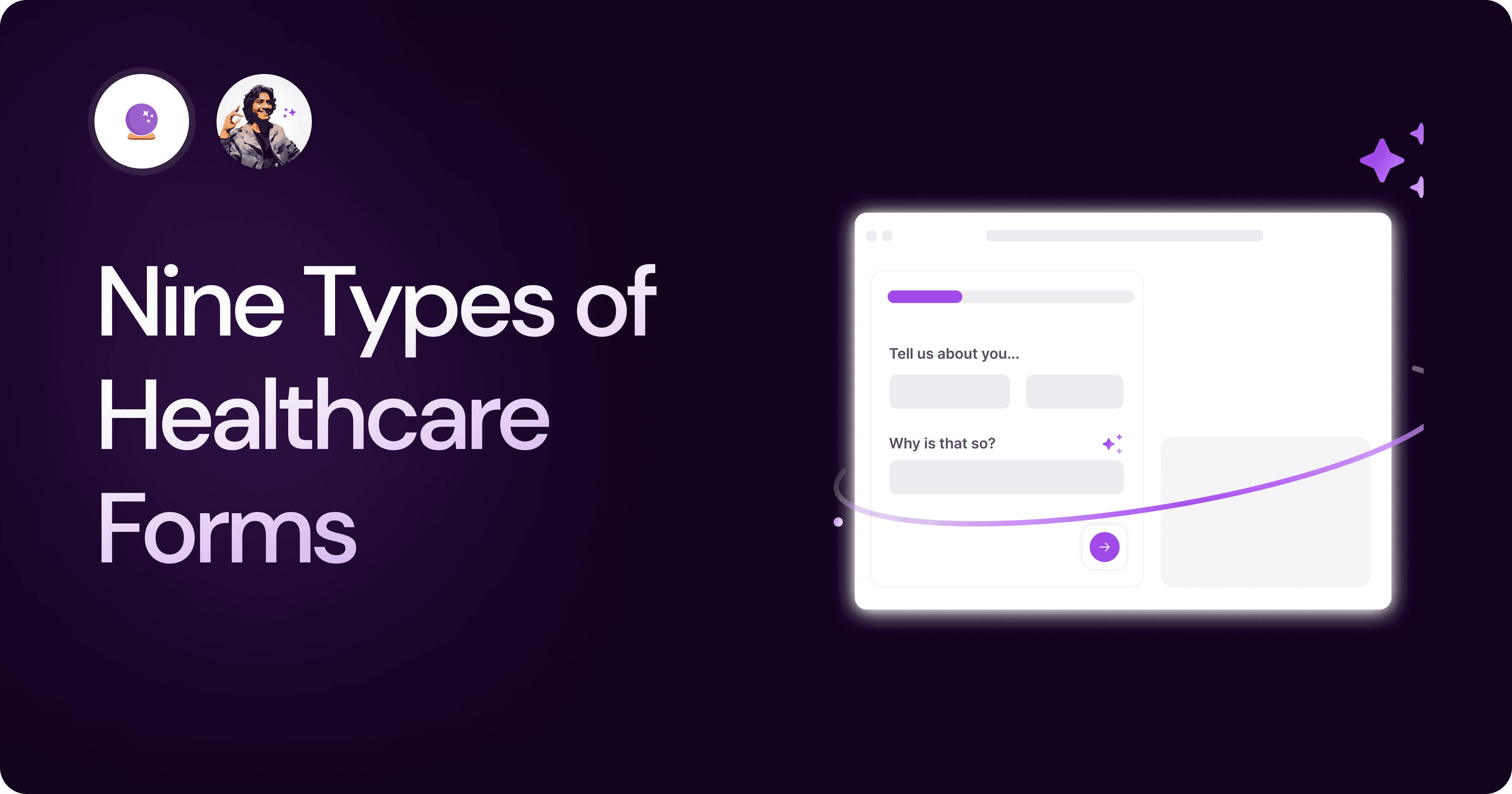
Nine Types of Healthcare and Medical Forms.
Medical forms are a must-have for any healthcare business or practitioner. Learn about the different kinds of medical and healthcare forms.
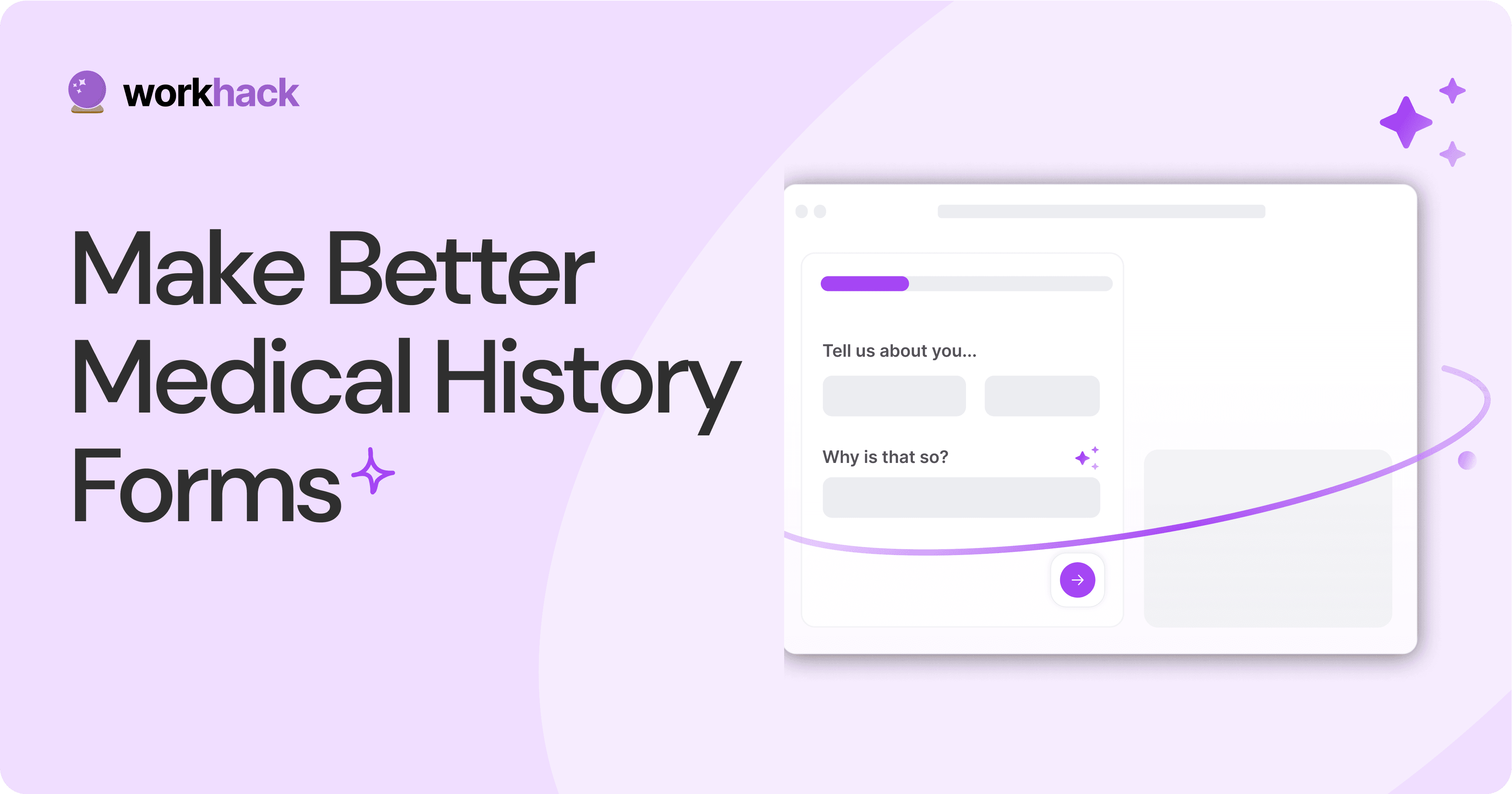
4 Tips for Better Medical History Forms.
Medical history forms are central to patient care, onboarding, and medical administration records. Learn how to make them easier to fill.
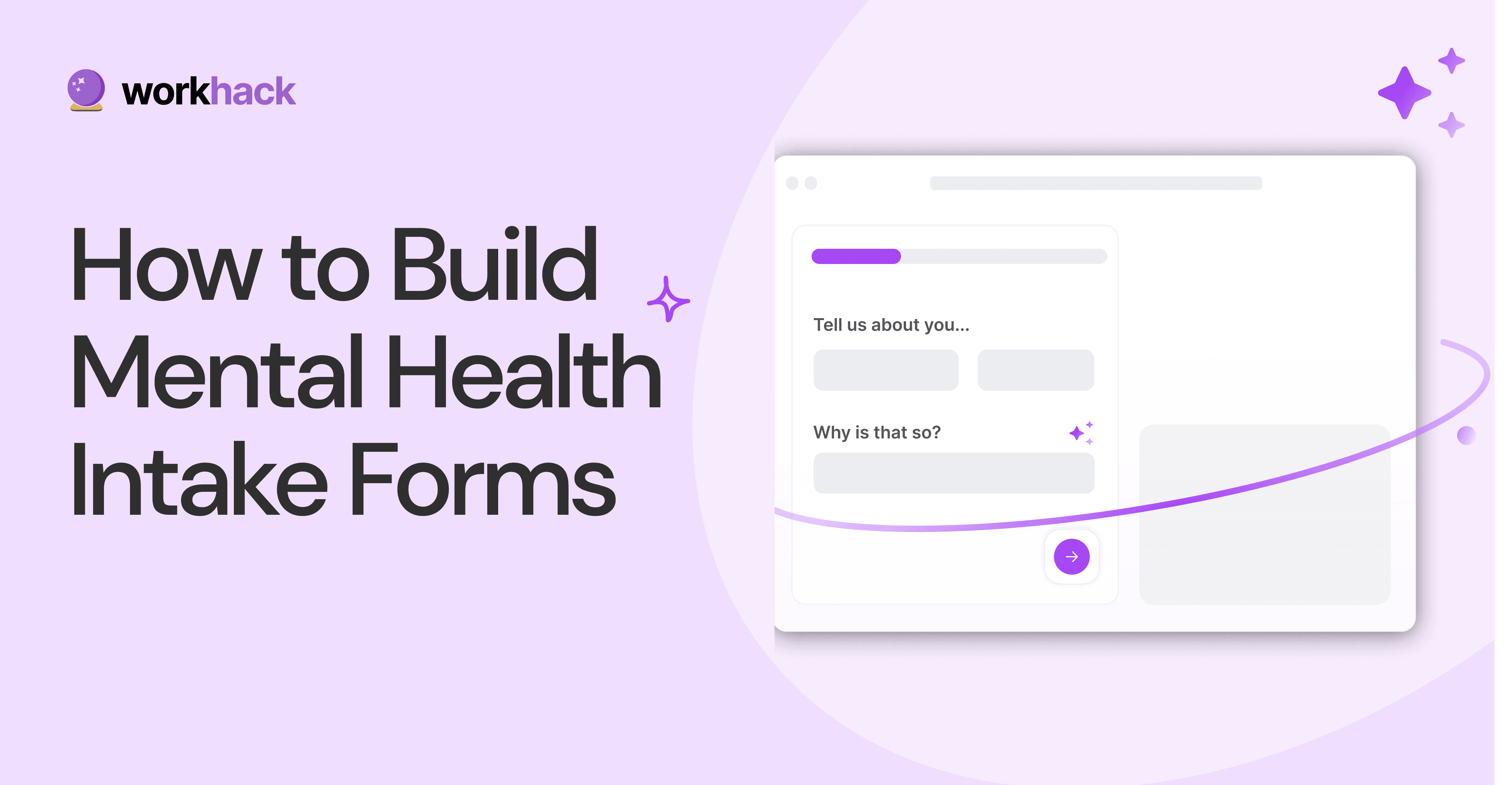
How to Build Mental Health Intake Forms?
Mental health intake forms are not like patient intake forms. Mental health intake forms deal with far more sensitive data and have specific design methods.
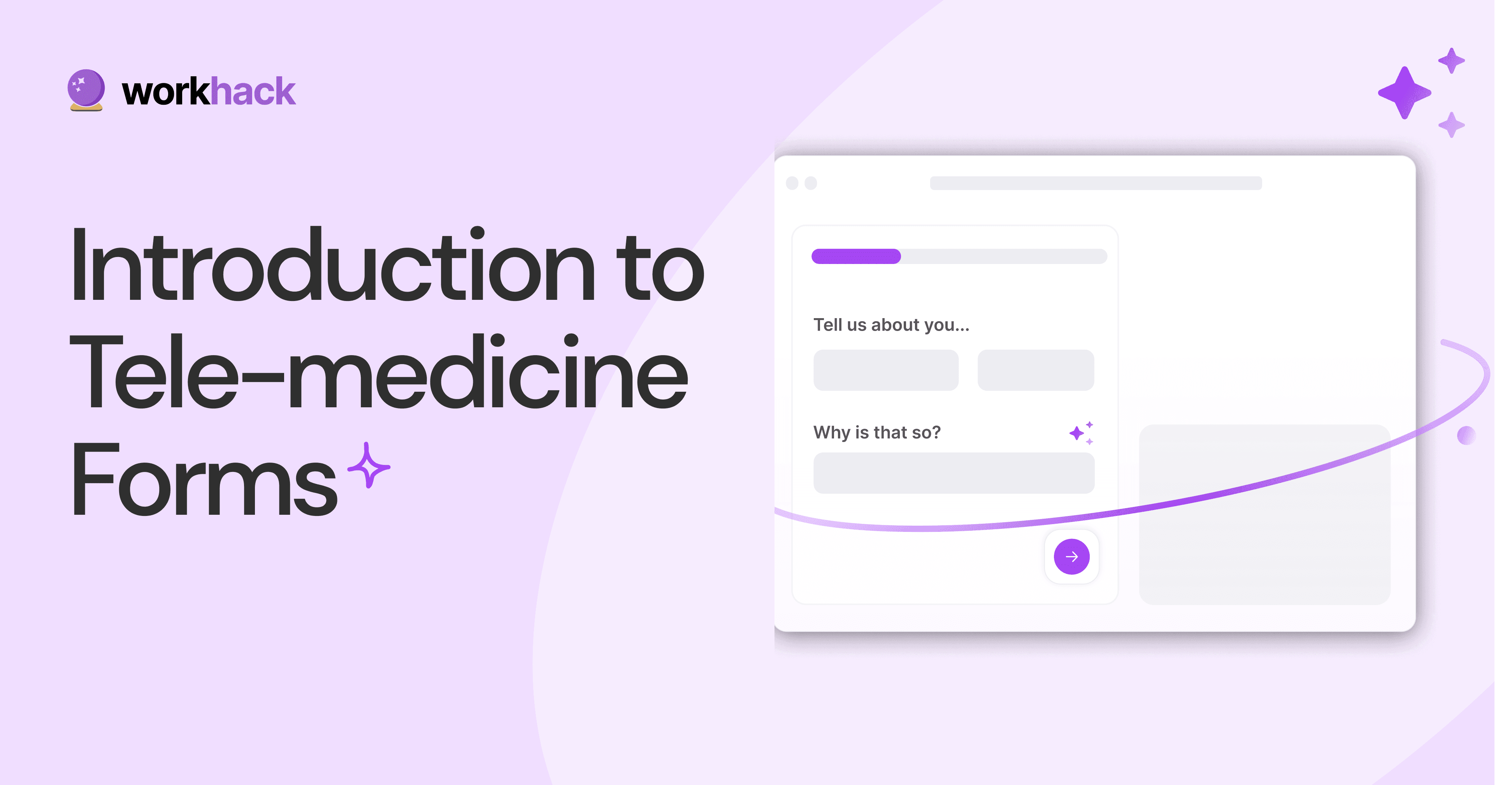
What, Why and How of Telemedicine Forms.
Telemedicine is on the rise and with different form builders out there, which one best suits your needs as a healthcare services provider?
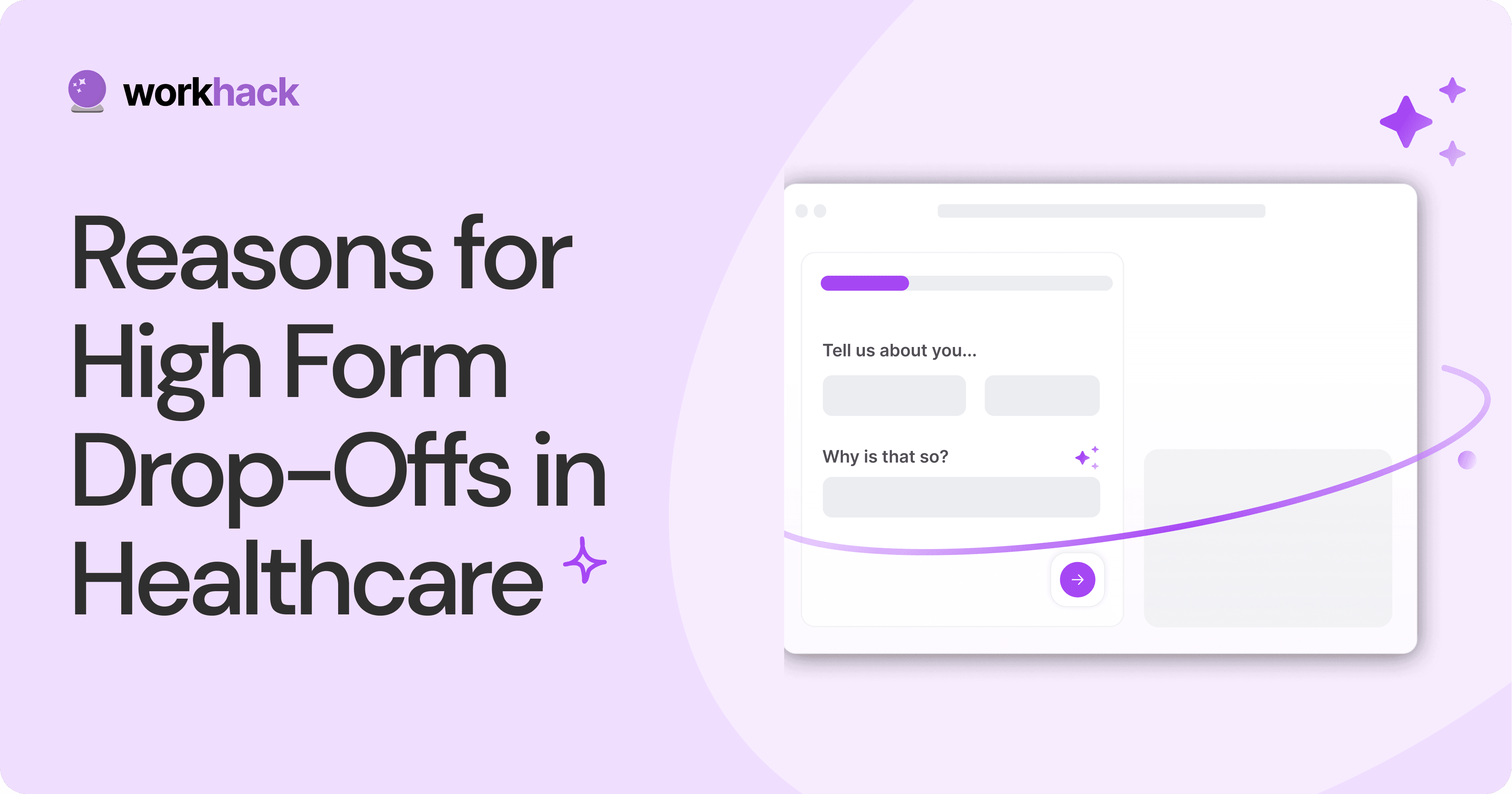
3 Reasons for Major Drop-Offs in Medical Forms.
No matter which healthcare form we pick, there are major drop-off reasons. We shall dive into the top 3 and learn how to resolve them in your next form.
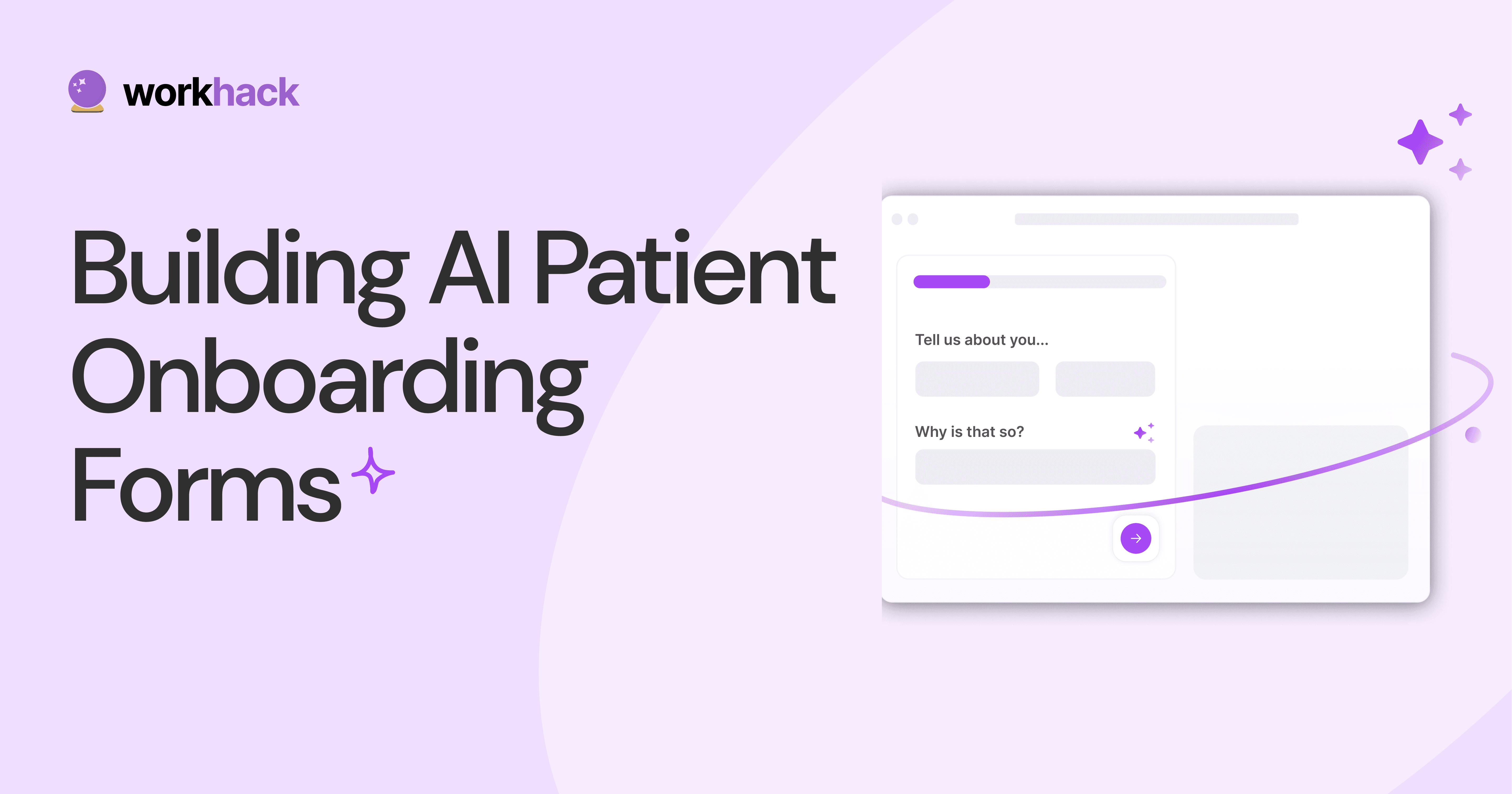
Patient Onboarding Forms - From Click to Clinic.
Patient onboarding forms are the first touchpoint for patients; getting this right for higher conversion rates is a must-have. Learn how to perfect them now.

5 Key Parts of a Good Patient Satisfaction Form.
The goal of patient satisfaction surveys is to course-correct the services of a healthcare provider. Patient feedback leads to a culture of patient-centric care.
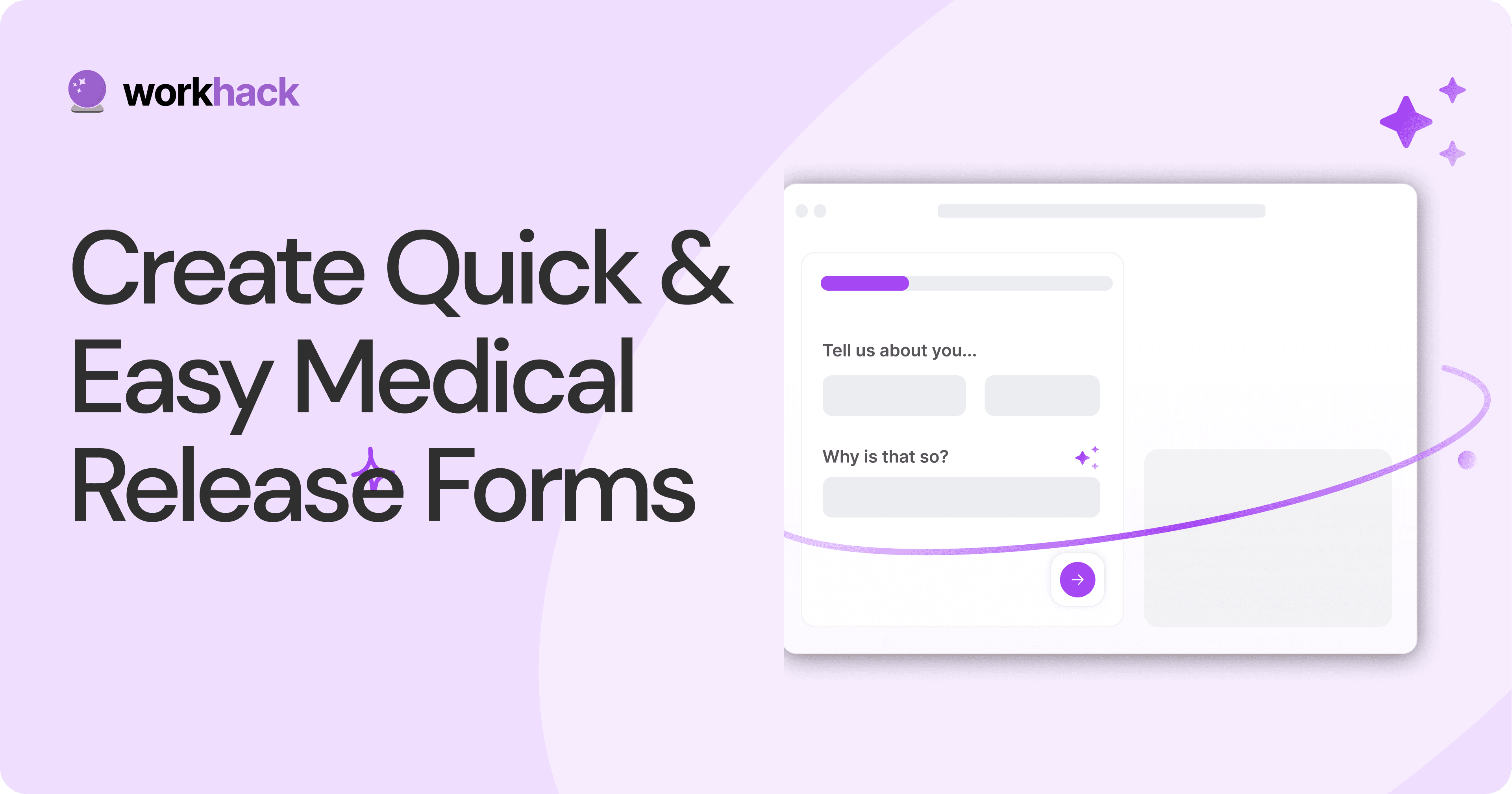
Build Quick and Easy Medical Release Forms.
Every HIPAA-compliant healthcare provider comes across medical release forms that involve details from medical history forms. Can they be shipped fast? Yes.
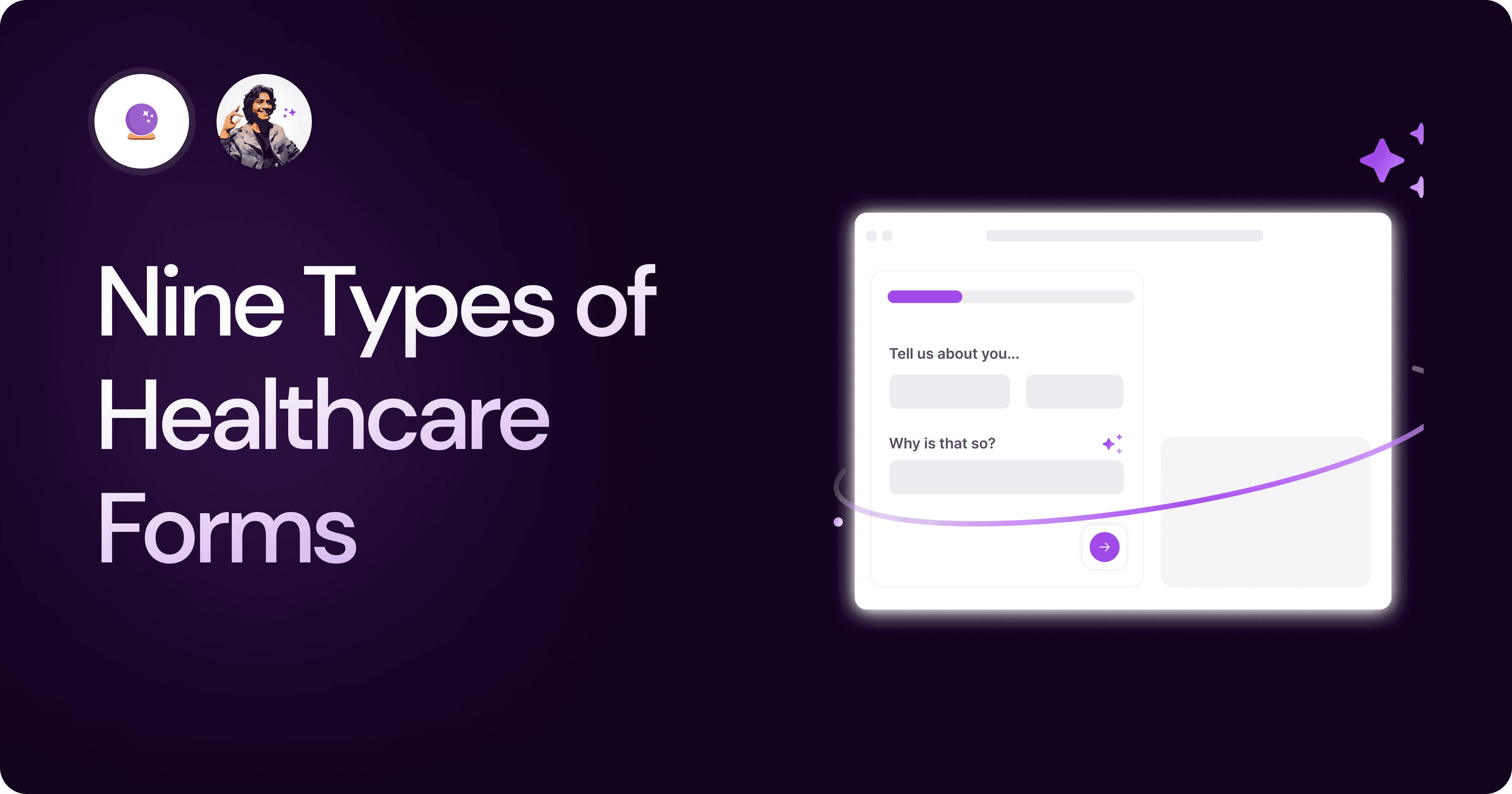
Nine Types of Healthcare and Medical Forms.
Medical forms are a must-have for any healthcare business or practitioner. Learn about the different kinds of medical and healthcare forms.
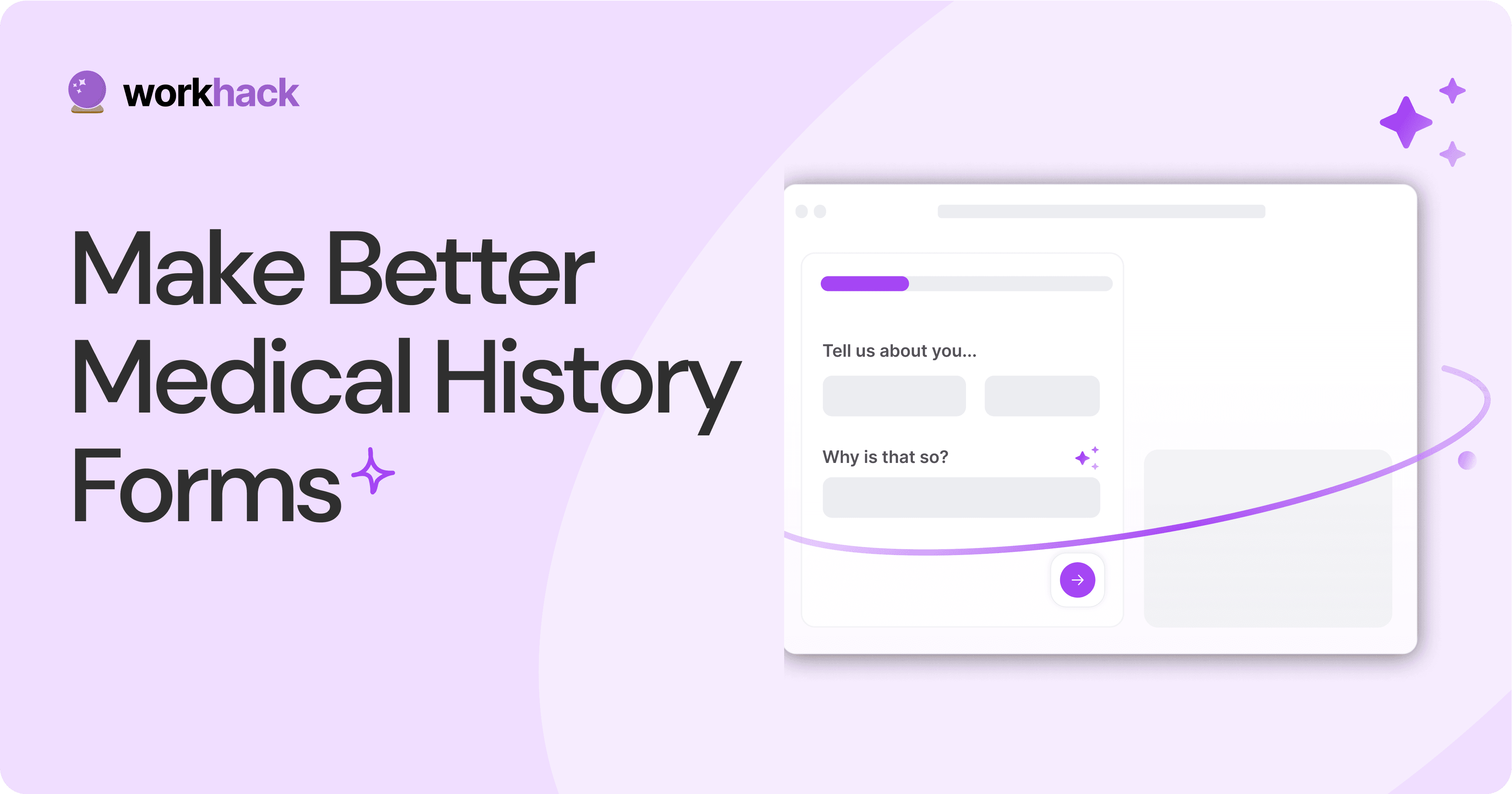
4 Tips for Better Medical History Forms.
Medical history forms are central to patient care, onboarding, and medical administration records. Learn how to make them easier to fill.
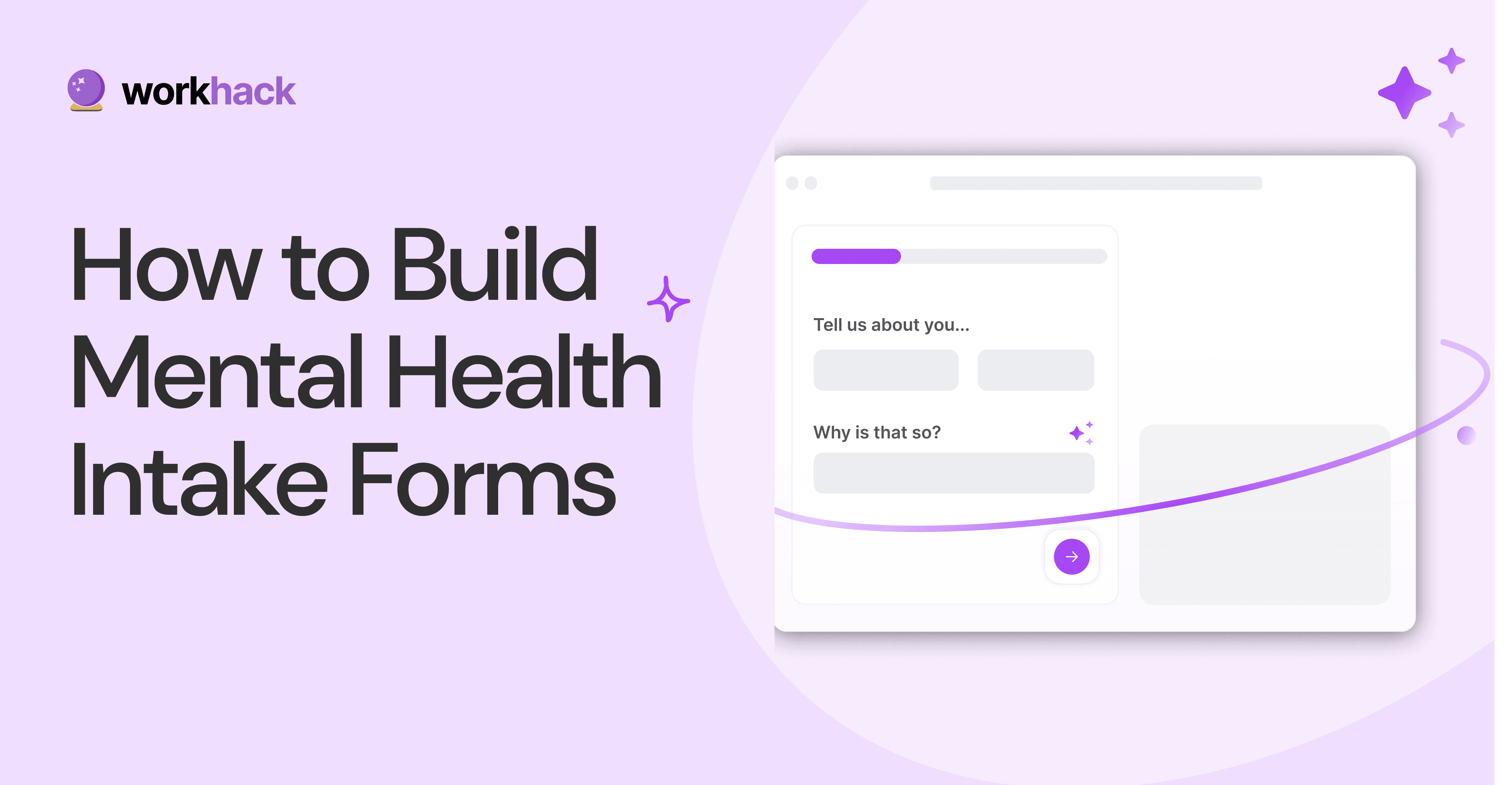
How to Build Mental Health Intake Forms?
Mental health intake forms are not like patient intake forms. Mental health intake forms deal with far more sensitive data and have specific design methods.
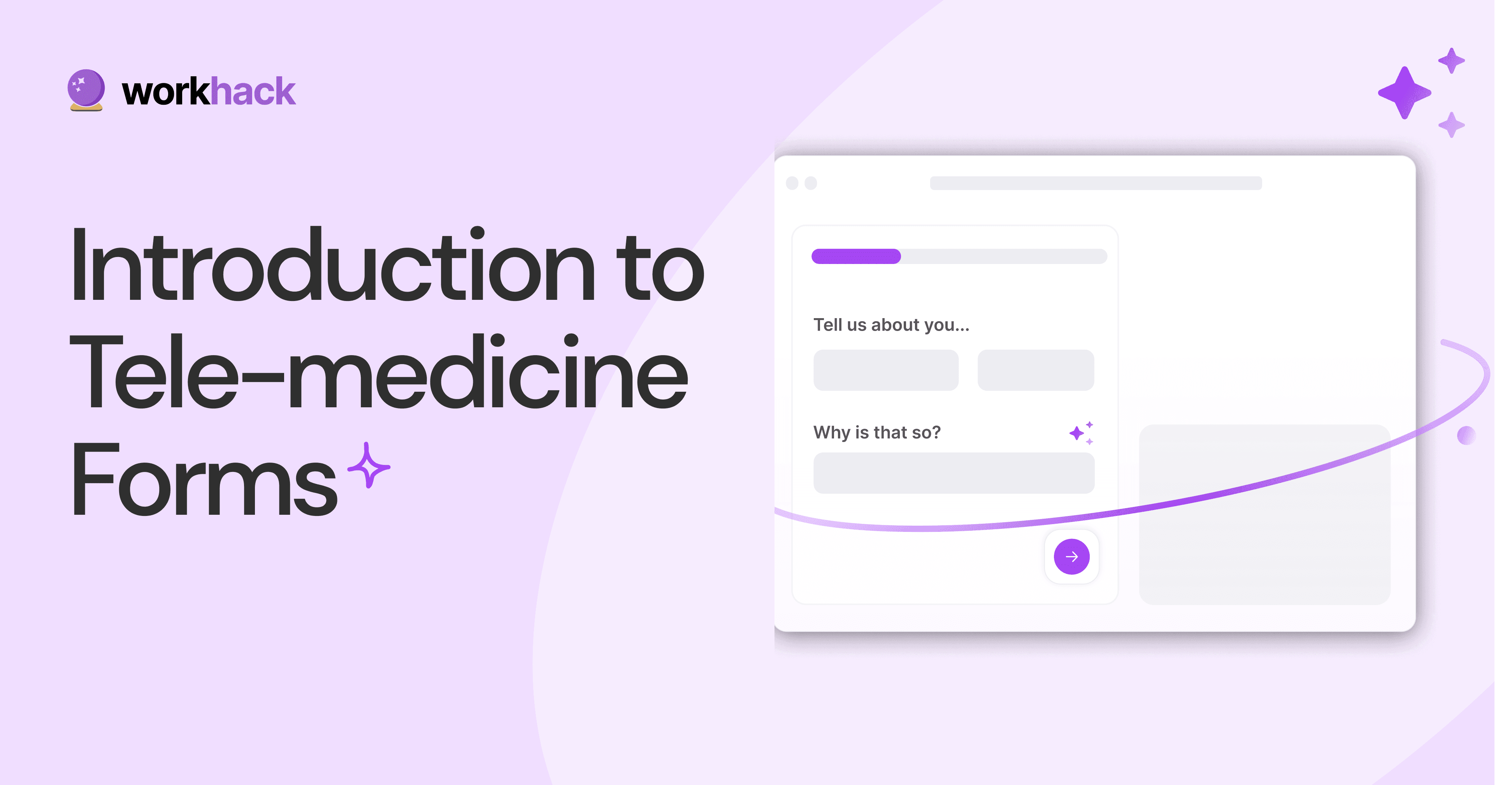
What, Why and How of Telemedicine Forms.
Telemedicine is on the rise and with different form builders out there, which one best suits your needs as a healthcare services provider?
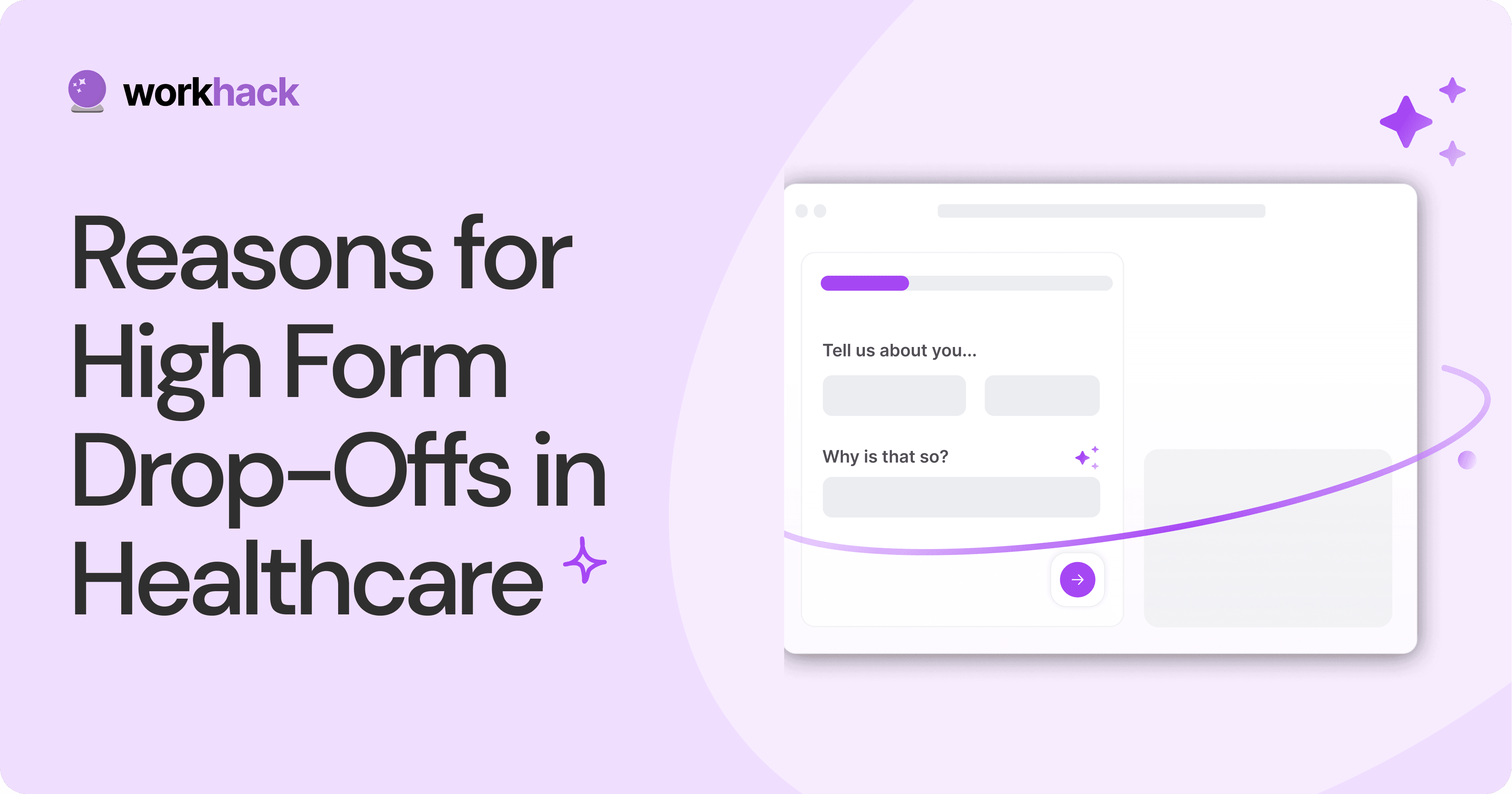
3 Reasons for Major Drop-Offs in Medical Forms.
No matter which healthcare form we pick, there are major drop-off reasons. We shall dive into the top 3 and learn how to resolve them in your next form.
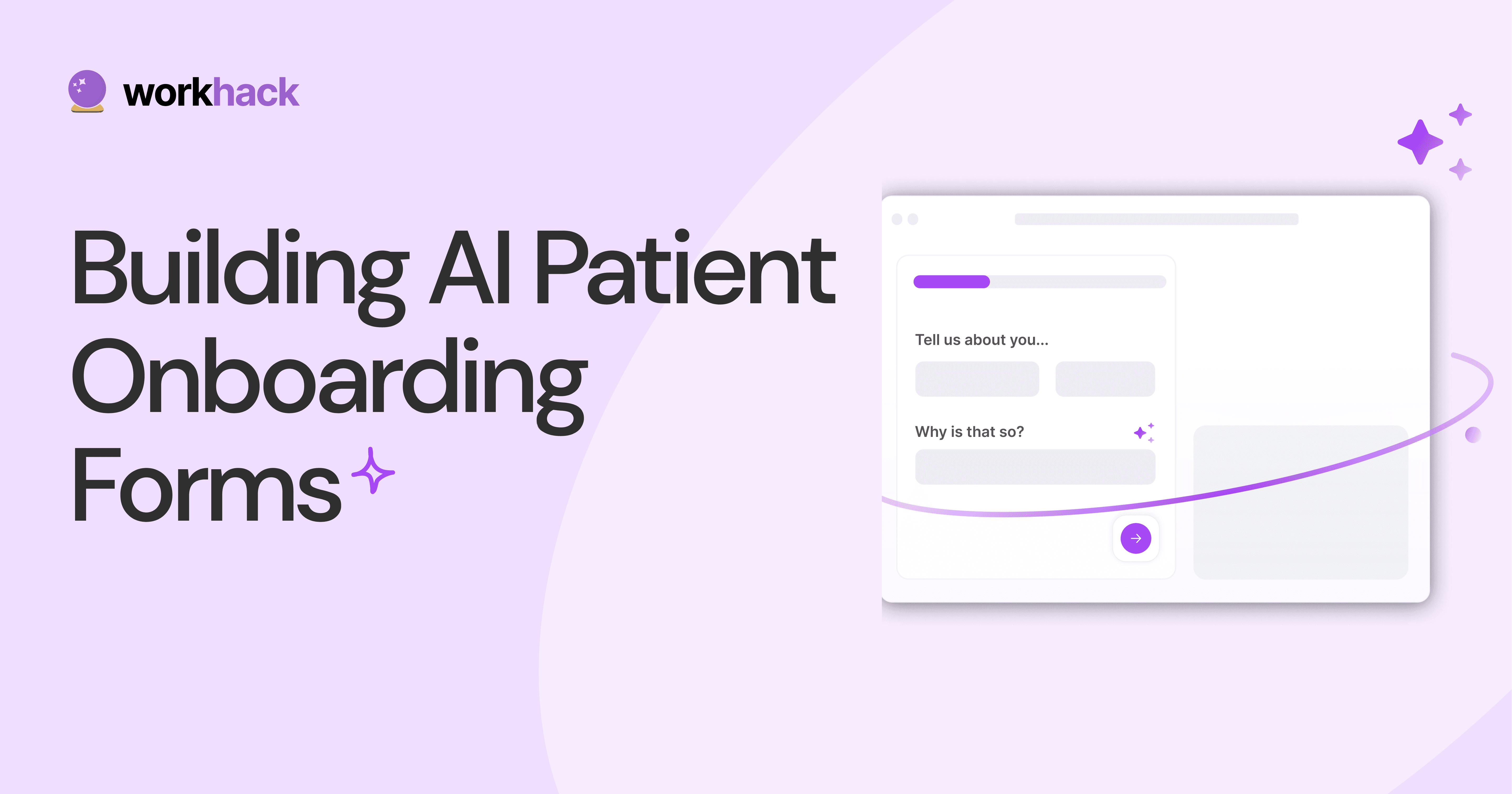
Patient Onboarding Forms - From Click to Clinic.
Patient onboarding forms are the first touchpoint for patients; getting this right for higher conversion rates is a must-have. Learn how to perfect them now.

5 Key Parts of a Good Patient Satisfaction Form.
The goal of patient satisfaction surveys is to course-correct the services of a healthcare provider. Patient feedback leads to a culture of patient-centric care.
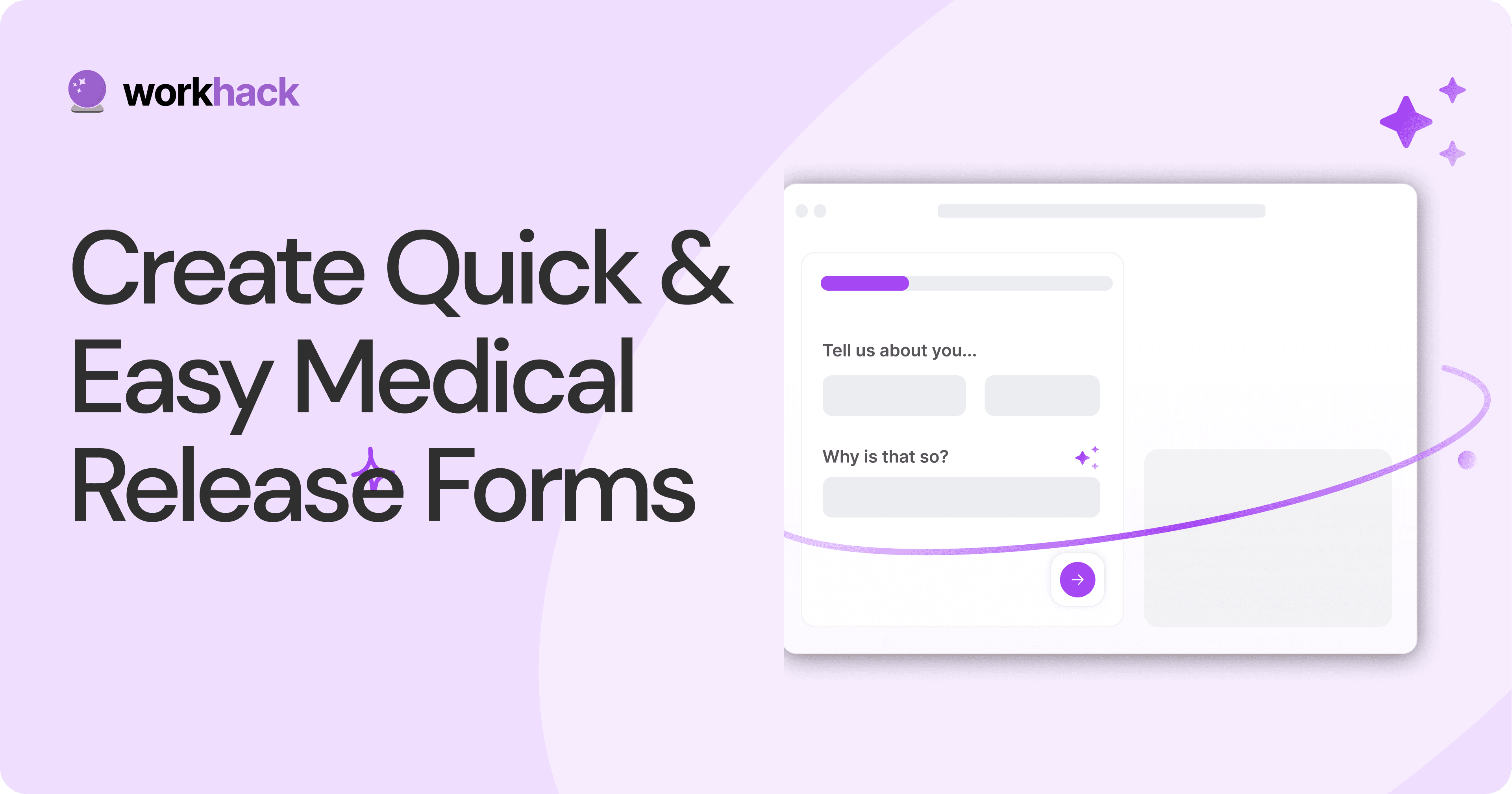
Build Quick and Easy Medical Release Forms.
Every HIPAA-compliant healthcare provider comes across medical release forms that involve details from medical history forms. Can they be shipped fast? Yes.
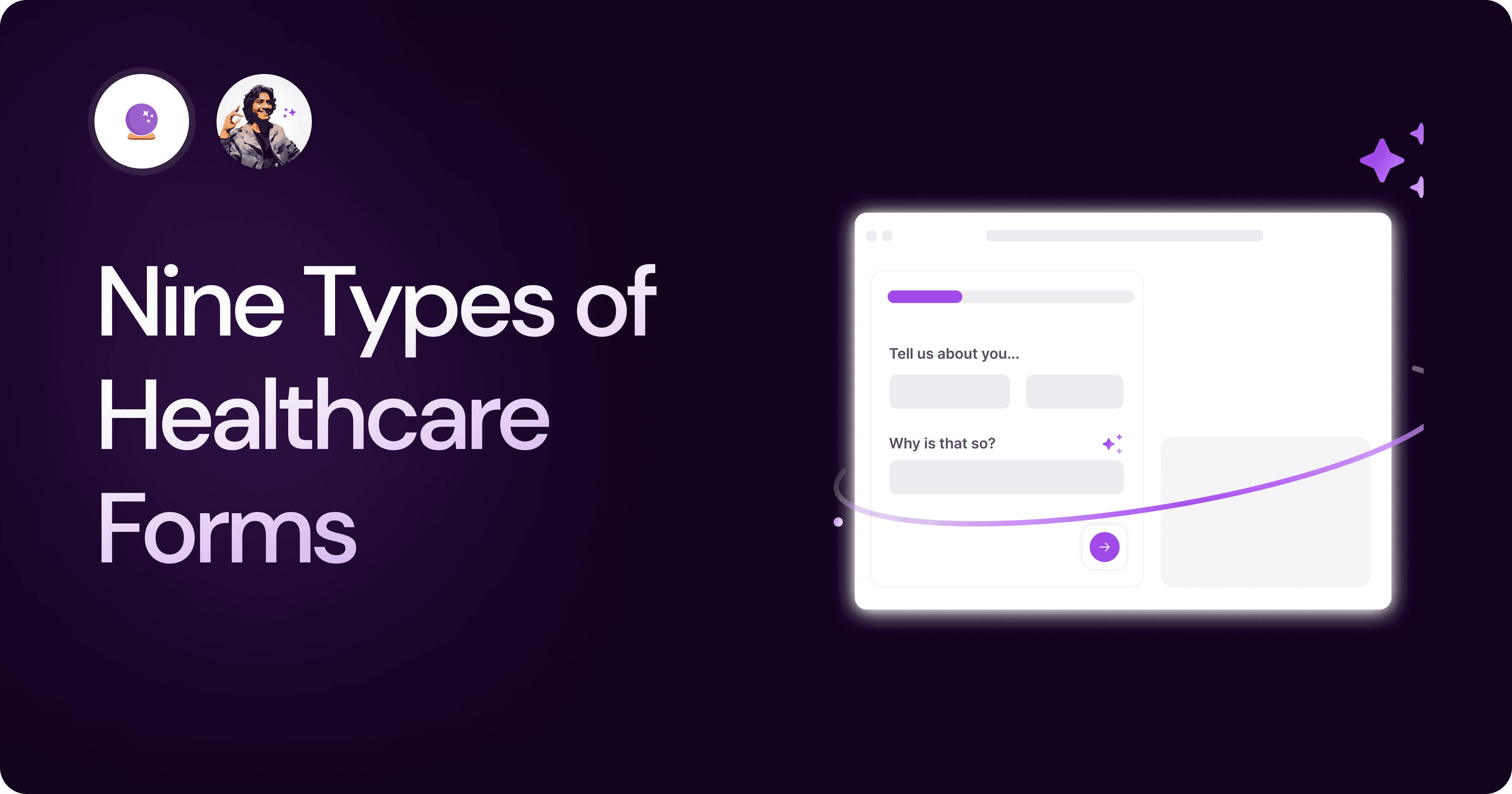
Nine Types of Healthcare and Medical Forms.
Medical forms are a must-have for any healthcare business or practitioner. Learn about the different kinds of medical and healthcare forms.
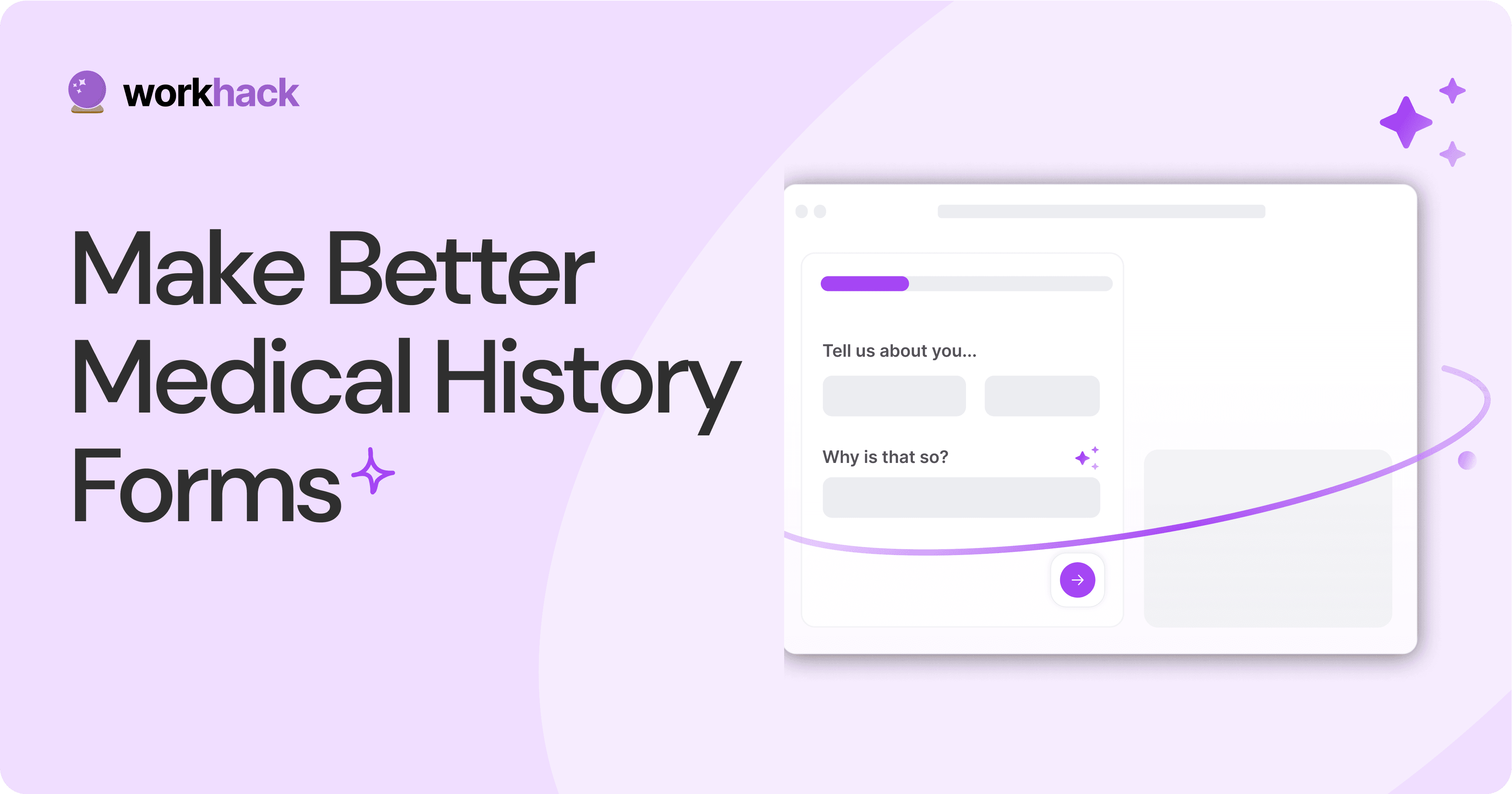
4 Tips for Better Medical History Forms.
Medical history forms are central to patient care, onboarding, and medical administration records. Learn how to make them easier to fill.
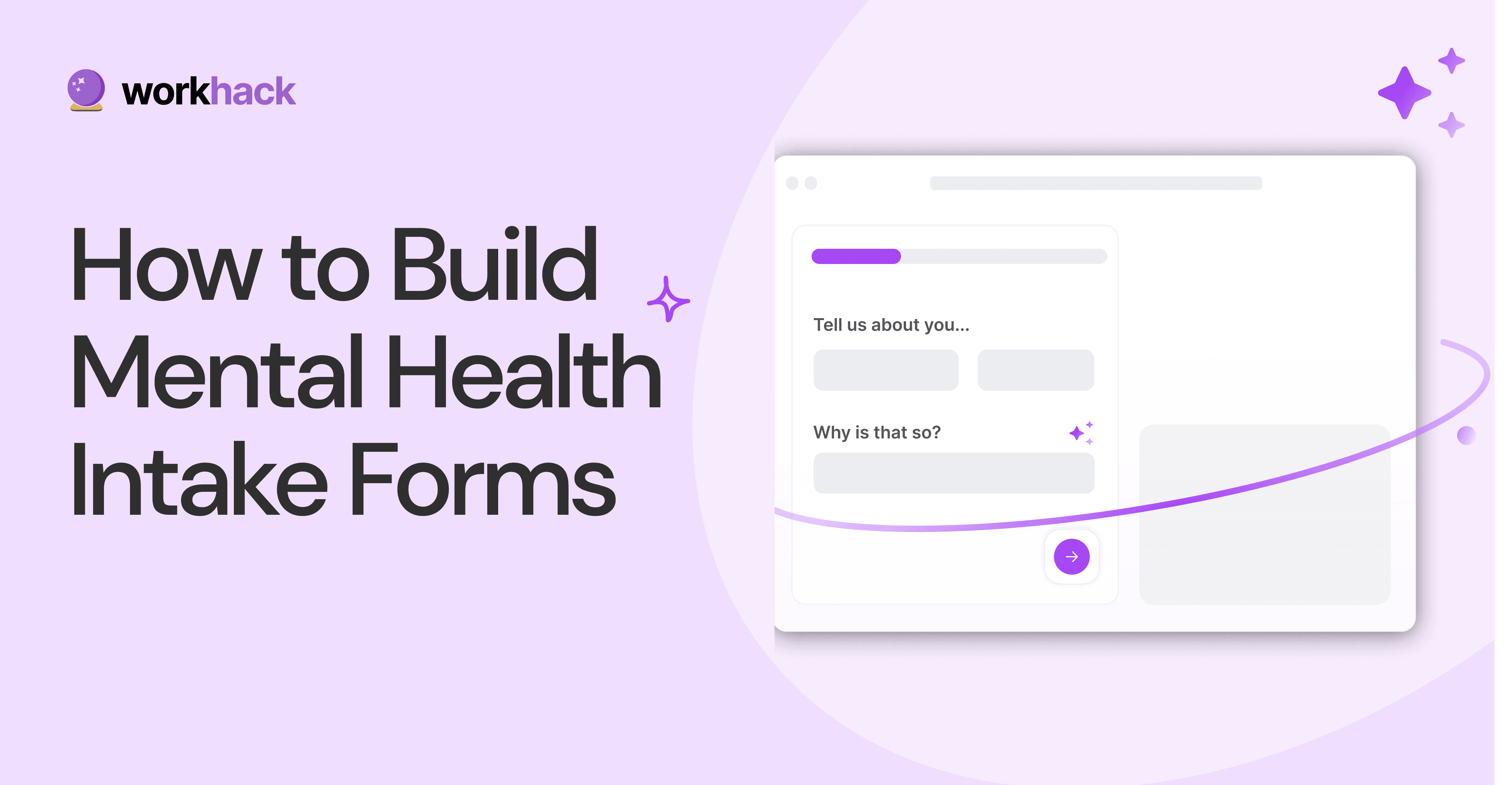
How to Build Mental Health Intake Forms?
Mental health intake forms are not like patient intake forms. Mental health intake forms deal with far more sensitive data and have specific design methods.
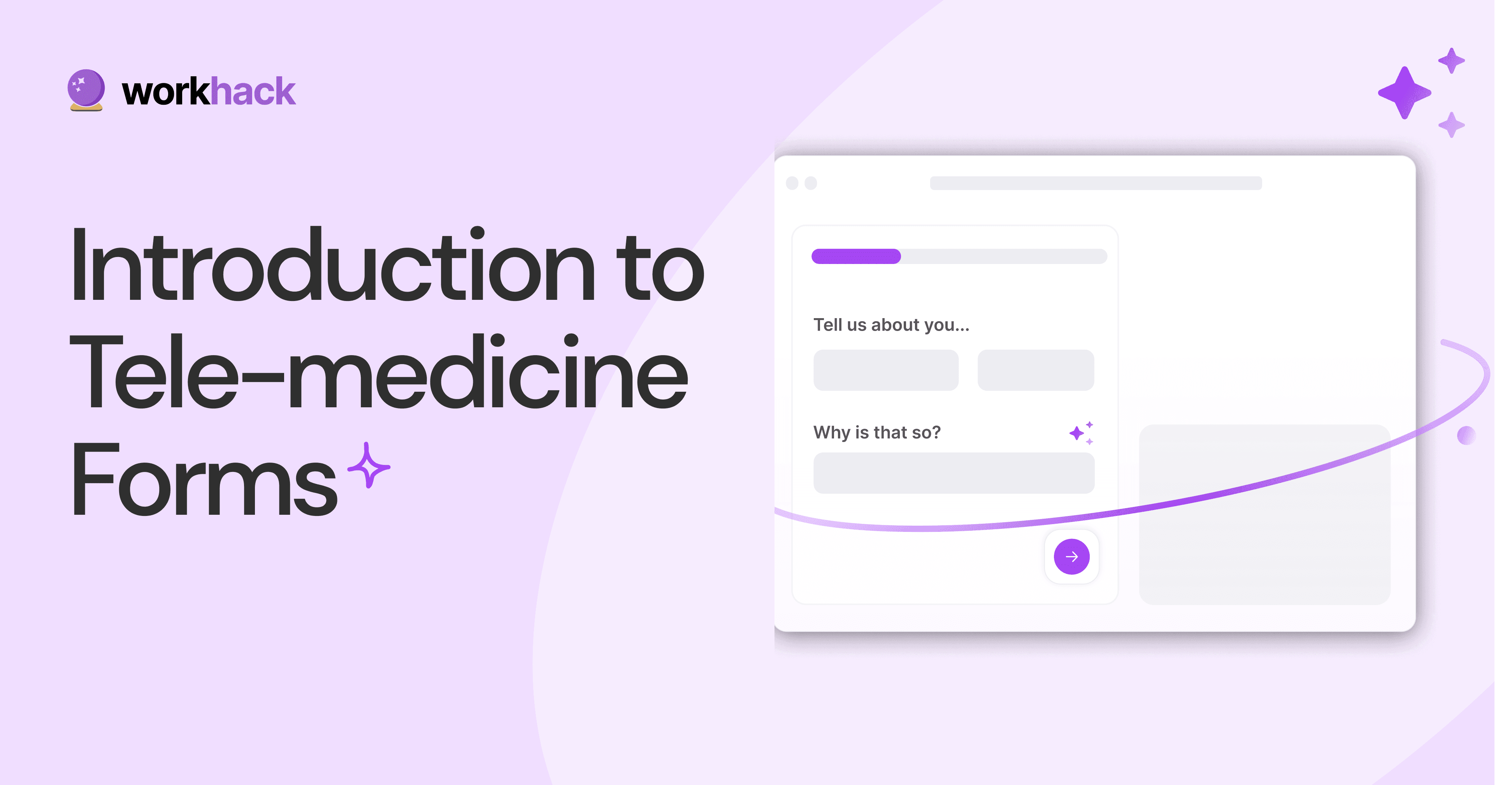
What, Why and How of Telemedicine Forms.
Telemedicine is on the rise and with different form builders out there, which one best suits your needs as a healthcare services provider?
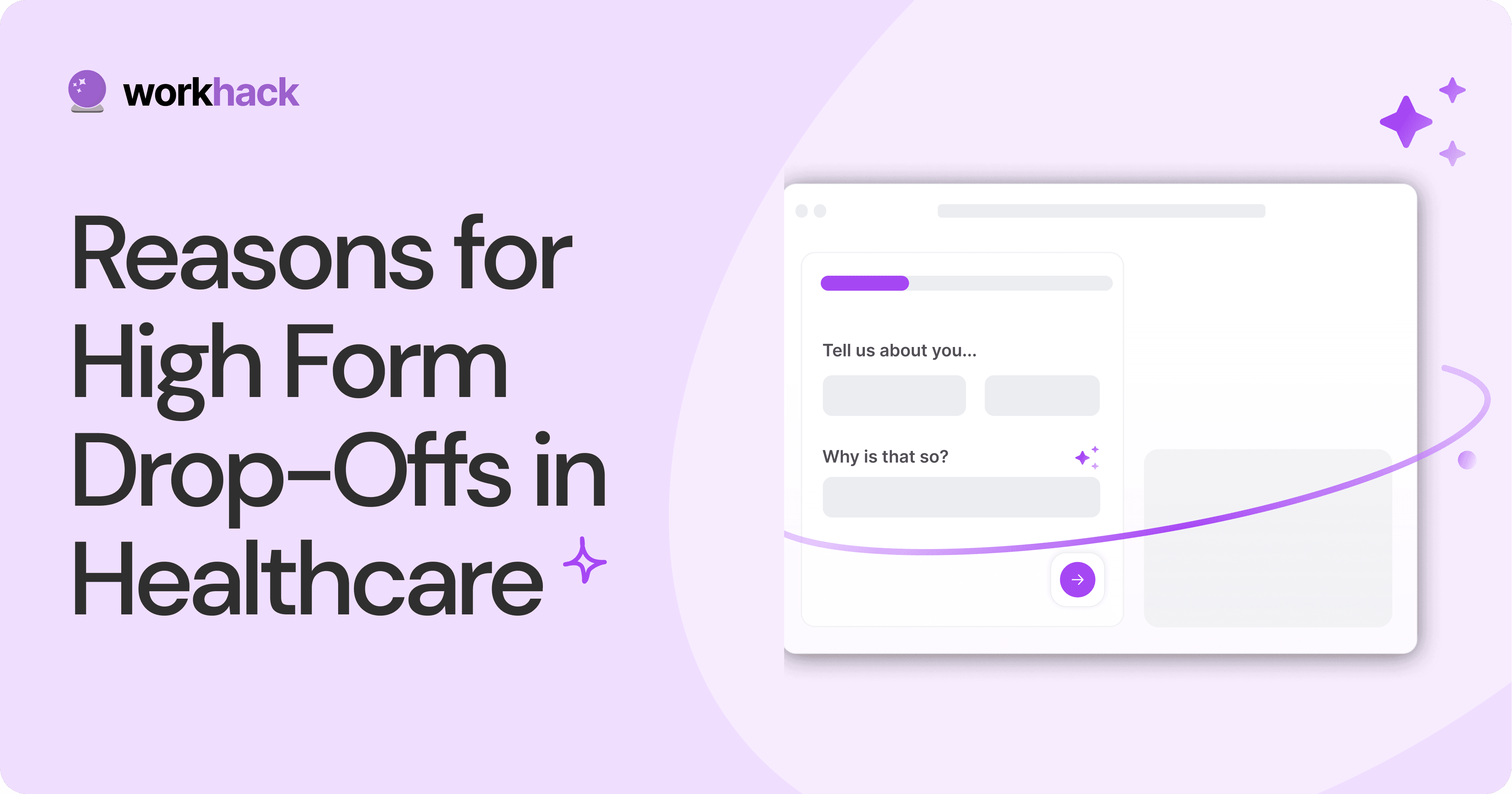
3 Reasons for Major Drop-Offs in Medical Forms.
No matter which healthcare form we pick, there are major drop-off reasons. We shall dive into the top 3 and learn how to resolve them in your next form.
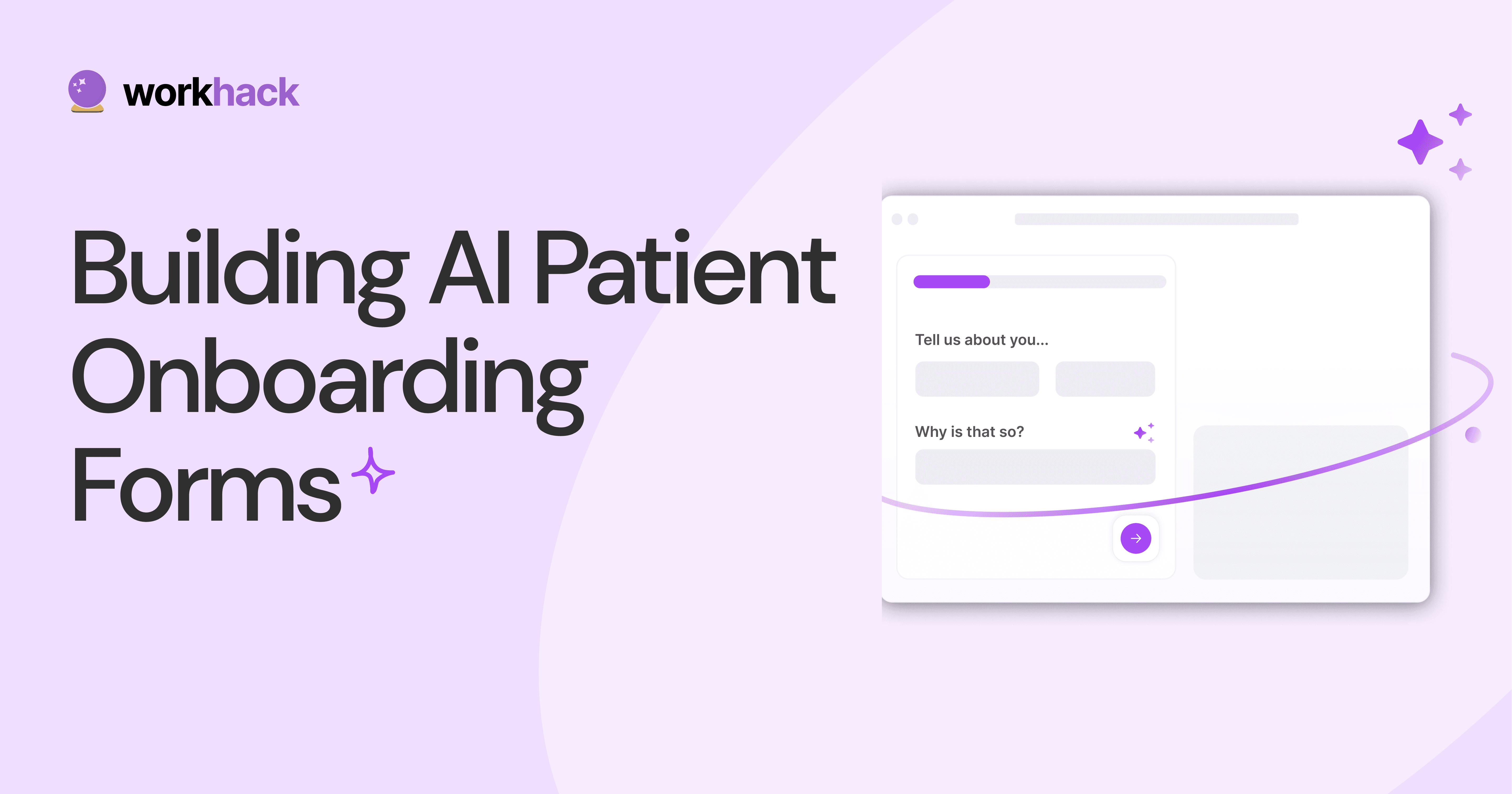
Patient Onboarding Forms - From Click to Clinic.
Patient onboarding forms are the first touchpoint for patients; getting this right for higher conversion rates is a must-have. Learn how to perfect them now.

5 Key Parts of a Good Patient Satisfaction Form.
The goal of patient satisfaction surveys is to course-correct the services of a healthcare provider. Patient feedback leads to a culture of patient-centric care.
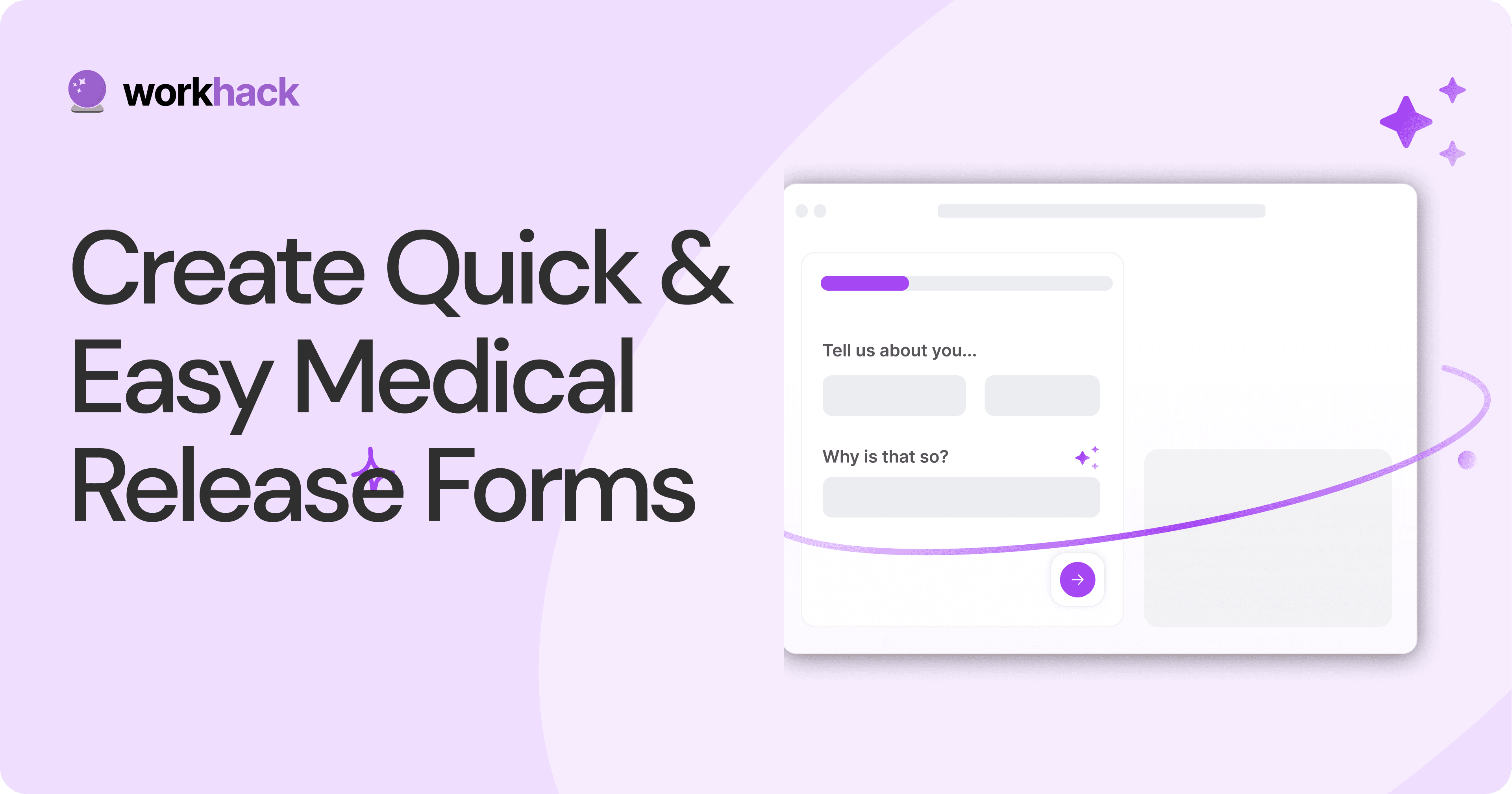
Build Quick and Easy Medical Release Forms.
Every HIPAA-compliant healthcare provider comes across medical release forms that involve details from medical history forms. Can they be shipped fast? Yes.
Subscribe to stay updated.
Subscribe to stay updated.
Subscribe to stay updated.
HC
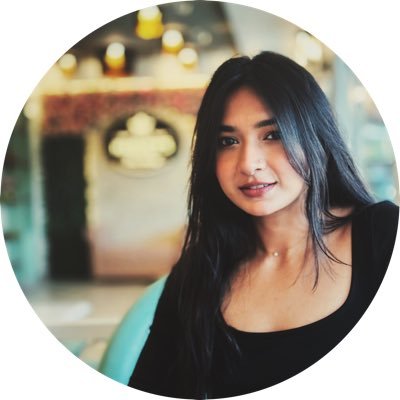
HC
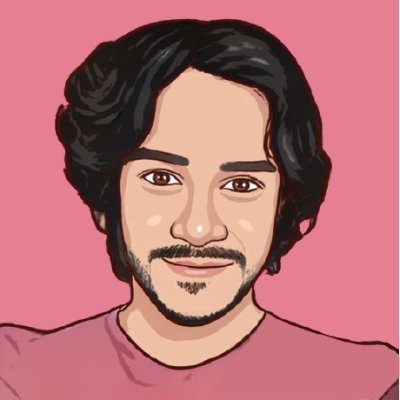
HC
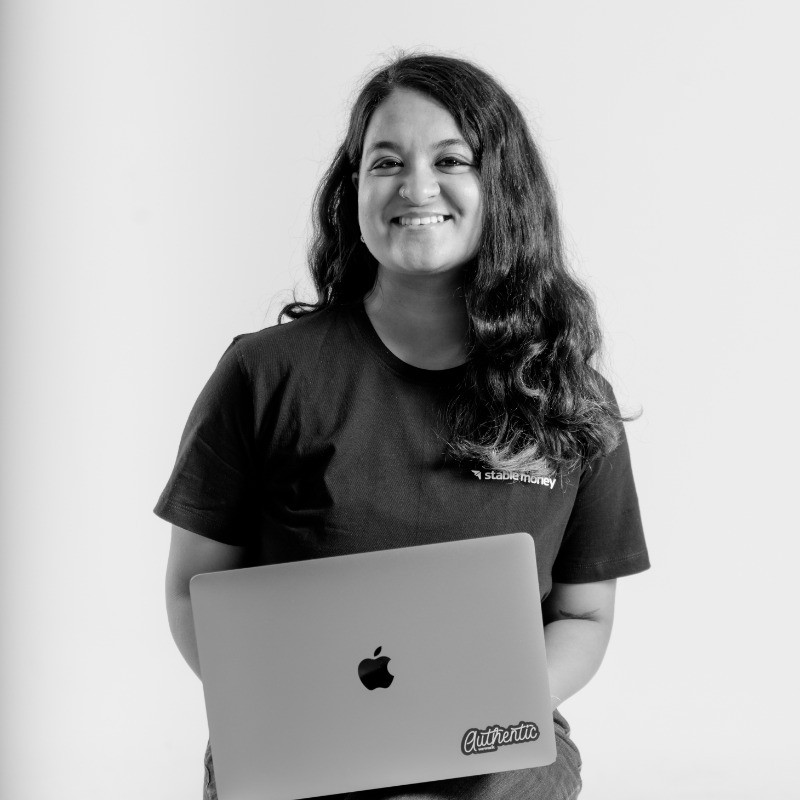
HC
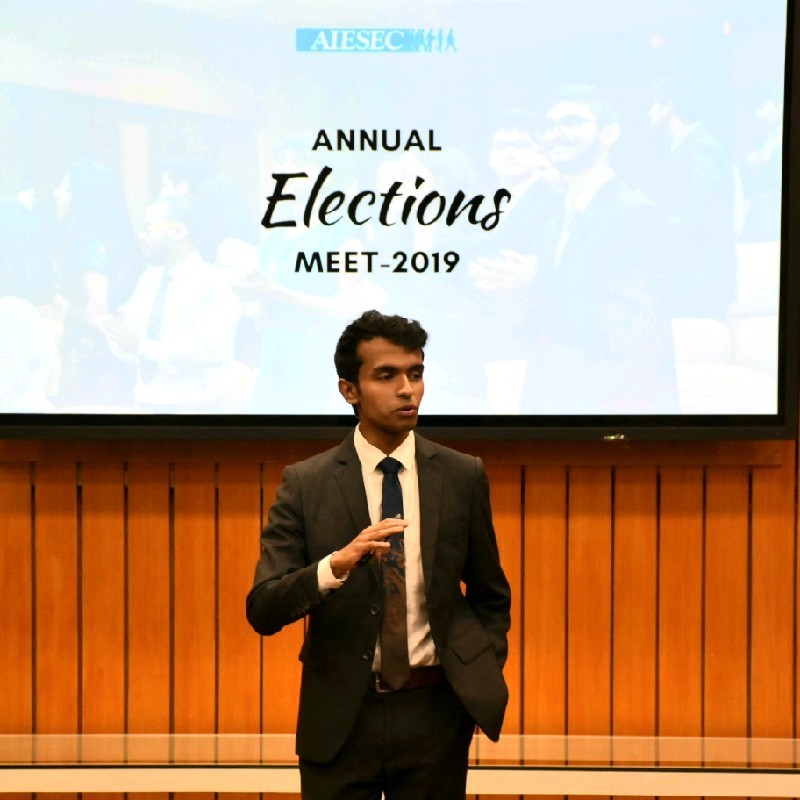
70+ people from across industries read our emails.
HC
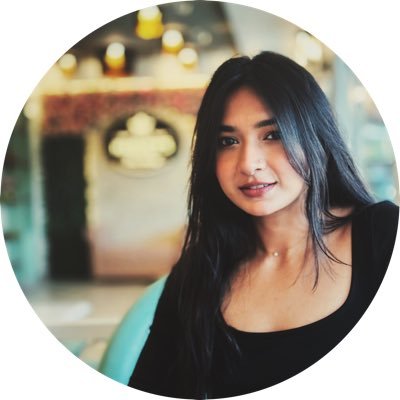
HC
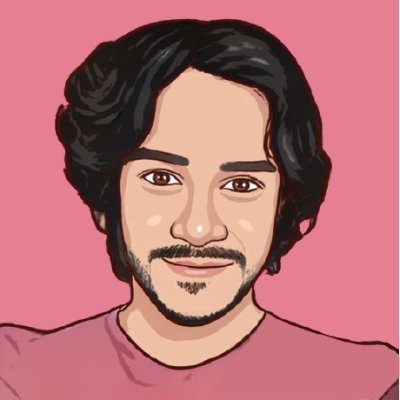
70+ people from across industries read our emails.
HC
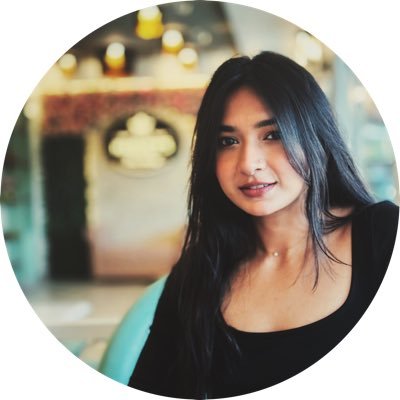
HC
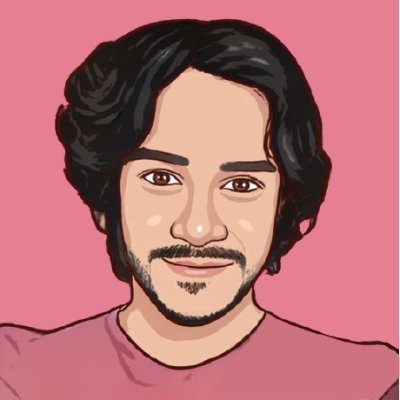
HC
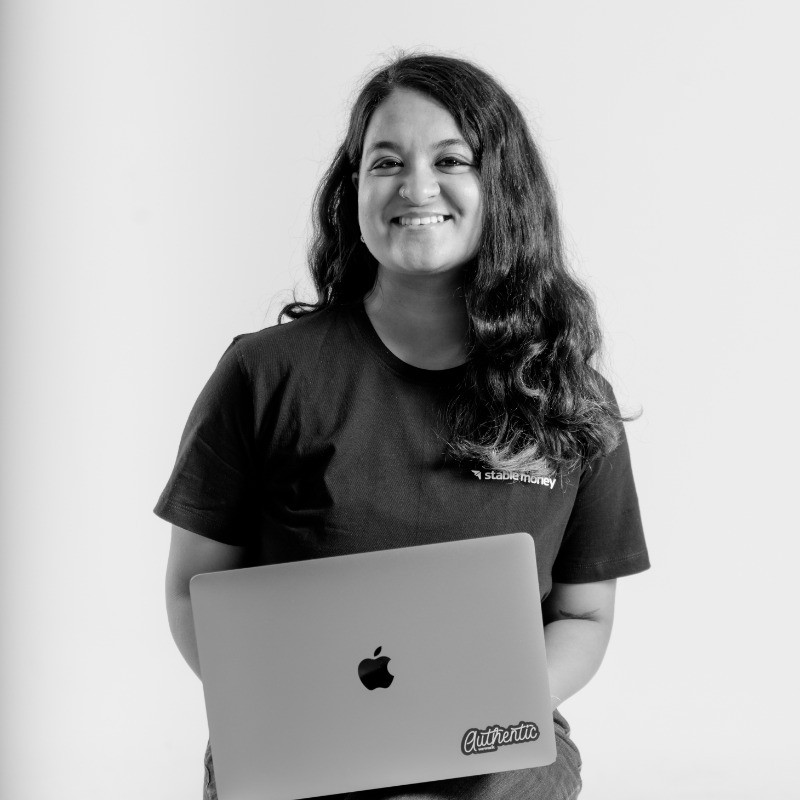
70+ people from across industries read our emails.
Bangalore, India / San Francisco, US
WorkHack Inc. 2023
Bangalore, India
San Francisco, US
WorkHack Inc. 2023
WorkHack Inc. 2023
Bangalore, India / San Francisco, US
WorkHack Inc. 2023
Bangalore, India / San Francisco, US