How to Elevate The Quality of Research Surveys using AI
How to Elevate The Quality of Research Surveys using AI
How to Elevate The Quality of Research Surveys using AI



Research surveys are indispensable tools for collecting insights across various domains such as market research, social sciences, and customer feedback. However, traditional survey methods often come with inefficiencies that hinder the quality of collected data. Artificial Intelligence (AI) offers groundbreaking solutions to enhance the effectiveness, precision, and quality of research surveys. This blog explores the common inefficiencies in research surveys, optimization strategies to address them, how qualitative researchers gauge survey quality, and the transformative role of AI in ensuring topnotch research survey quality.
Common Inefficiencies in Research Surveys
Research surveys, while powerful, are often plagued by several inefficiencies that compromise the quality of the data and the reliability of insights derived from them. Here are some of the most common issues.
1. Survey Design Flaws
Ambiguous Questions: Poorly worded or ambiguous questions lead to misinterpretation, resulting in unreliable data.
Lengthy Surveys: Long surveys often lead to respondent fatigue, causing incomplete or rushed responses.
Lack of Relevance: Irrelevant questions frustrate respondents, leading to lower engagement and higher dropout rates.
2. Data Collection Challenges
Non-Representative Samples: Failing to obtain a representative sample skew results and limit the generalizability of the findings.
Low Response Rates: Many surveys suffer from low response rates, which affect the reliability of the data.
Inaccurate Responses: Respondents may provide inaccurate or dishonest answers due to various biases or social desirability effects.
3. Data Management Issues
Data Entry Errors: Manual data entry is prone to errors, which compromise the integrity of the dataset.
Inconsistent Coding: Inconsistent coding of responses leads to difficulties in data analysis and interpretation.
Difficulty in Real-Time Analysis: Traditional surveys often lack the capability for realtime data analysis, delaying actionable insights.
Optimization Strategies to Tackle Inefficiencies
To elevate the quality of research surveys, researchers must adopt strategies that address these inefficiencies. Here are key optimization tactics:
1. Improve Survey Design
Clear and Concise Questions: Ensure questions are clear, concise, and free from jargon to avoid misinterpretation.
Survey Length Management: Keep surveys as short as possible to minimize respondent fatigue while capturing essential data.
Relevance and Personalization: Tailor questions to the respondent's context and relevance to increase engagement and response accuracy.
2. Enhance Data Collection Techniques
Representative Sampling: Use stratified sampling methods to ensure a representative sample of the target population.
Incentives and Engagement: Provide incentives for participation and design engaging surveys to boost response rates.
Anonymity and Confidentiality: Ensure respondent anonymity to reduce biases and encourage honest responses.
3. Optimize Data Management
Automate Data Entry: Use digital survey tools to automate data collection and minimize entry errors.
Standardize Coding: Develop and adhere to consistent coding schemes for open-ended responses to facilitate analysis.
Real-Time Data Processing: Implement tools for real-time data processing to enable immediate insights and actions.
The Quality of Research Surveys: A Qualitative Researcher's Perspective
Qualitative researchers assess the quality of research surveys through several criteria.
1. Validity
Content Validity: Ensures that the survey measures the concept it intends to measure. It assesses whether the questions adequately cover the research topic.
Construct Validity: Evaluates whether the survey truly measures the theoretical construct it claims to measure.
Criterion Validity: Compares the survey results with external criteria or benchmarks to determine accuracy.
2. Reliability
Internal Consistency: Measures the consistency of responses across different items in the survey. Commonly assessed using Cronbach's alpha.
TestRetest Reliability: Assesses the stability of responses over time by administering the same survey to the same respondents at different points in time.
3. Response Rate and Representation
Response Rate: The proportion of respondents who complete the survey. A higher response rate generally indicates more reliable data.
Representativeness: Ensures that the sample accurately represents the target population, which is crucial for generalizing findings.
4. Respondent Engagement
Completion Rates: The percentage of respondents who complete the survey, indicating their engagement level.
Dropout Analysis: Examines where and why respondents drop out to identify potential survey design issues.
5. Data Integrity
Consistency Checks: Regularly review data for consistency and coherence to identify any anomalies or patterns of response bias.
Response Quality: Assess the quality of openended responses for depth and relevance.
The Role of AI in Ensuring the Quality of Research Surveys
AI is transforming the landscape of research surveys by addressing inefficiencies and enhancing quality through advanced technologies and methodologies. Here’s how AI is making a difference.
1. AI in Survey Design
Natural Language Processing (NLP): AI-powered NLP tools analyze and refine question wording to ensure clarity and relevance. They also generate dynamic, personalized questions based on respondent answers.
Survey Optimization: AI algorithms predict optimal survey length and structure to maintain respondent engagement and minimize fatigue.
2. AI in Data Collection
Automated Sampling: AI automates the sampling process to ensure a representative sample and reduce selection biases.
Chatbots and Virtual Assistants: AI-driven chatbots conduct surveys conversationally, improving engagement and response accuracy by making the process more interactive and less formal.
Adaptive Surveys: AI creates adaptive surveys that adjust questions based on previous responses, enhancing relevance and reducing survey length.
3. AI in Data Management
Real-Time Analysis: AI surveys enable real-time data analysis, providing instant insights and allowing for immediate adjustments or interventions.
Data Cleaning and Validation: AI surveys automatically clean and validate data, identifying and correcting errors or inconsistencies without manual intervention.
Sentiment Analysis: AI-driven sentiment analysis tools evaluate open-ended responses, extracting valuable insights into respondent attitudes and emotions.
4. AI in Data Analysis
Pattern Recognition: AI identifies patterns and trends in large datasets, uncovering insights that might be missed by traditional analysis methods.
Predictive Analytics: AI models predict future trends based on current and historical data, offering foresight into potential developments and outcomes.
Theme Extraction: AI-native survey builders automatically extract and categorize themes from qualitative data, providing a structured understanding of complex responses.
5. AI in Enhancing Respondent Engagement
Personalization: AI-native survey builders like Metaforms personalise the survey experience by tailoring questions and content to the individual respondent, increasing engagement and data relevance.
Gamification: AI survey builders introduce gamified elements into surveys, making the process more engaging and improving response rates.
Conclusion: The Quality of Research Surveys using AI
Elevating the quality of research surveys is imperative for obtaining reliable and actionable insights. While traditional methods face several inefficiencies, AIdriven innovations offer transformative solutions that enhance survey design, data collection, management, and analysis. By addressing common issues such as ambiguous questions, low response rates, and data inconsistencies, AI empowers researchers to conduct more effective and highquality surveys.
For qualitative researchers, understanding and leveraging AI in survey research bridges gaps in validity, reliability, and respondent engagement, ensuring that the surveys not only meet but exceed quality standards. As AI technology continues to evolve, its role in refining research methodologies and delivering precise insights will only grow, paving the way for more informed decisions and successful outcomes in the research landscape.
Research surveys are indispensable tools for collecting insights across various domains such as market research, social sciences, and customer feedback. However, traditional survey methods often come with inefficiencies that hinder the quality of collected data. Artificial Intelligence (AI) offers groundbreaking solutions to enhance the effectiveness, precision, and quality of research surveys. This blog explores the common inefficiencies in research surveys, optimization strategies to address them, how qualitative researchers gauge survey quality, and the transformative role of AI in ensuring topnotch research survey quality.
Common Inefficiencies in Research Surveys
Research surveys, while powerful, are often plagued by several inefficiencies that compromise the quality of the data and the reliability of insights derived from them. Here are some of the most common issues.
1. Survey Design Flaws
Ambiguous Questions: Poorly worded or ambiguous questions lead to misinterpretation, resulting in unreliable data.
Lengthy Surveys: Long surveys often lead to respondent fatigue, causing incomplete or rushed responses.
Lack of Relevance: Irrelevant questions frustrate respondents, leading to lower engagement and higher dropout rates.
2. Data Collection Challenges
Non-Representative Samples: Failing to obtain a representative sample skew results and limit the generalizability of the findings.
Low Response Rates: Many surveys suffer from low response rates, which affect the reliability of the data.
Inaccurate Responses: Respondents may provide inaccurate or dishonest answers due to various biases or social desirability effects.
3. Data Management Issues
Data Entry Errors: Manual data entry is prone to errors, which compromise the integrity of the dataset.
Inconsistent Coding: Inconsistent coding of responses leads to difficulties in data analysis and interpretation.
Difficulty in Real-Time Analysis: Traditional surveys often lack the capability for realtime data analysis, delaying actionable insights.
Optimization Strategies to Tackle Inefficiencies
To elevate the quality of research surveys, researchers must adopt strategies that address these inefficiencies. Here are key optimization tactics:
1. Improve Survey Design
Clear and Concise Questions: Ensure questions are clear, concise, and free from jargon to avoid misinterpretation.
Survey Length Management: Keep surveys as short as possible to minimize respondent fatigue while capturing essential data.
Relevance and Personalization: Tailor questions to the respondent's context and relevance to increase engagement and response accuracy.
2. Enhance Data Collection Techniques
Representative Sampling: Use stratified sampling methods to ensure a representative sample of the target population.
Incentives and Engagement: Provide incentives for participation and design engaging surveys to boost response rates.
Anonymity and Confidentiality: Ensure respondent anonymity to reduce biases and encourage honest responses.
3. Optimize Data Management
Automate Data Entry: Use digital survey tools to automate data collection and minimize entry errors.
Standardize Coding: Develop and adhere to consistent coding schemes for open-ended responses to facilitate analysis.
Real-Time Data Processing: Implement tools for real-time data processing to enable immediate insights and actions.
The Quality of Research Surveys: A Qualitative Researcher's Perspective
Qualitative researchers assess the quality of research surveys through several criteria.
1. Validity
Content Validity: Ensures that the survey measures the concept it intends to measure. It assesses whether the questions adequately cover the research topic.
Construct Validity: Evaluates whether the survey truly measures the theoretical construct it claims to measure.
Criterion Validity: Compares the survey results with external criteria or benchmarks to determine accuracy.
2. Reliability
Internal Consistency: Measures the consistency of responses across different items in the survey. Commonly assessed using Cronbach's alpha.
TestRetest Reliability: Assesses the stability of responses over time by administering the same survey to the same respondents at different points in time.
3. Response Rate and Representation
Response Rate: The proportion of respondents who complete the survey. A higher response rate generally indicates more reliable data.
Representativeness: Ensures that the sample accurately represents the target population, which is crucial for generalizing findings.
4. Respondent Engagement
Completion Rates: The percentage of respondents who complete the survey, indicating their engagement level.
Dropout Analysis: Examines where and why respondents drop out to identify potential survey design issues.
5. Data Integrity
Consistency Checks: Regularly review data for consistency and coherence to identify any anomalies or patterns of response bias.
Response Quality: Assess the quality of openended responses for depth and relevance.
The Role of AI in Ensuring the Quality of Research Surveys
AI is transforming the landscape of research surveys by addressing inefficiencies and enhancing quality through advanced technologies and methodologies. Here’s how AI is making a difference.
1. AI in Survey Design
Natural Language Processing (NLP): AI-powered NLP tools analyze and refine question wording to ensure clarity and relevance. They also generate dynamic, personalized questions based on respondent answers.
Survey Optimization: AI algorithms predict optimal survey length and structure to maintain respondent engagement and minimize fatigue.
2. AI in Data Collection
Automated Sampling: AI automates the sampling process to ensure a representative sample and reduce selection biases.
Chatbots and Virtual Assistants: AI-driven chatbots conduct surveys conversationally, improving engagement and response accuracy by making the process more interactive and less formal.
Adaptive Surveys: AI creates adaptive surveys that adjust questions based on previous responses, enhancing relevance and reducing survey length.
3. AI in Data Management
Real-Time Analysis: AI surveys enable real-time data analysis, providing instant insights and allowing for immediate adjustments or interventions.
Data Cleaning and Validation: AI surveys automatically clean and validate data, identifying and correcting errors or inconsistencies without manual intervention.
Sentiment Analysis: AI-driven sentiment analysis tools evaluate open-ended responses, extracting valuable insights into respondent attitudes and emotions.
4. AI in Data Analysis
Pattern Recognition: AI identifies patterns and trends in large datasets, uncovering insights that might be missed by traditional analysis methods.
Predictive Analytics: AI models predict future trends based on current and historical data, offering foresight into potential developments and outcomes.
Theme Extraction: AI-native survey builders automatically extract and categorize themes from qualitative data, providing a structured understanding of complex responses.
5. AI in Enhancing Respondent Engagement
Personalization: AI-native survey builders like Metaforms personalise the survey experience by tailoring questions and content to the individual respondent, increasing engagement and data relevance.
Gamification: AI survey builders introduce gamified elements into surveys, making the process more engaging and improving response rates.
Conclusion: The Quality of Research Surveys using AI
Elevating the quality of research surveys is imperative for obtaining reliable and actionable insights. While traditional methods face several inefficiencies, AIdriven innovations offer transformative solutions that enhance survey design, data collection, management, and analysis. By addressing common issues such as ambiguous questions, low response rates, and data inconsistencies, AI empowers researchers to conduct more effective and highquality surveys.
For qualitative researchers, understanding and leveraging AI in survey research bridges gaps in validity, reliability, and respondent engagement, ensuring that the surveys not only meet but exceed quality standards. As AI technology continues to evolve, its role in refining research methodologies and delivering precise insights will only grow, paving the way for more informed decisions and successful outcomes in the research landscape.
Research surveys are indispensable tools for collecting insights across various domains such as market research, social sciences, and customer feedback. However, traditional survey methods often come with inefficiencies that hinder the quality of collected data. Artificial Intelligence (AI) offers groundbreaking solutions to enhance the effectiveness, precision, and quality of research surveys. This blog explores the common inefficiencies in research surveys, optimization strategies to address them, how qualitative researchers gauge survey quality, and the transformative role of AI in ensuring topnotch research survey quality.
Common Inefficiencies in Research Surveys
Research surveys, while powerful, are often plagued by several inefficiencies that compromise the quality of the data and the reliability of insights derived from them. Here are some of the most common issues.
1. Survey Design Flaws
Ambiguous Questions: Poorly worded or ambiguous questions lead to misinterpretation, resulting in unreliable data.
Lengthy Surveys: Long surveys often lead to respondent fatigue, causing incomplete or rushed responses.
Lack of Relevance: Irrelevant questions frustrate respondents, leading to lower engagement and higher dropout rates.
2. Data Collection Challenges
Non-Representative Samples: Failing to obtain a representative sample skew results and limit the generalizability of the findings.
Low Response Rates: Many surveys suffer from low response rates, which affect the reliability of the data.
Inaccurate Responses: Respondents may provide inaccurate or dishonest answers due to various biases or social desirability effects.
3. Data Management Issues
Data Entry Errors: Manual data entry is prone to errors, which compromise the integrity of the dataset.
Inconsistent Coding: Inconsistent coding of responses leads to difficulties in data analysis and interpretation.
Difficulty in Real-Time Analysis: Traditional surveys often lack the capability for realtime data analysis, delaying actionable insights.
Optimization Strategies to Tackle Inefficiencies
To elevate the quality of research surveys, researchers must adopt strategies that address these inefficiencies. Here are key optimization tactics:
1. Improve Survey Design
Clear and Concise Questions: Ensure questions are clear, concise, and free from jargon to avoid misinterpretation.
Survey Length Management: Keep surveys as short as possible to minimize respondent fatigue while capturing essential data.
Relevance and Personalization: Tailor questions to the respondent's context and relevance to increase engagement and response accuracy.
2. Enhance Data Collection Techniques
Representative Sampling: Use stratified sampling methods to ensure a representative sample of the target population.
Incentives and Engagement: Provide incentives for participation and design engaging surveys to boost response rates.
Anonymity and Confidentiality: Ensure respondent anonymity to reduce biases and encourage honest responses.
3. Optimize Data Management
Automate Data Entry: Use digital survey tools to automate data collection and minimize entry errors.
Standardize Coding: Develop and adhere to consistent coding schemes for open-ended responses to facilitate analysis.
Real-Time Data Processing: Implement tools for real-time data processing to enable immediate insights and actions.
The Quality of Research Surveys: A Qualitative Researcher's Perspective
Qualitative researchers assess the quality of research surveys through several criteria.
1. Validity
Content Validity: Ensures that the survey measures the concept it intends to measure. It assesses whether the questions adequately cover the research topic.
Construct Validity: Evaluates whether the survey truly measures the theoretical construct it claims to measure.
Criterion Validity: Compares the survey results with external criteria or benchmarks to determine accuracy.
2. Reliability
Internal Consistency: Measures the consistency of responses across different items in the survey. Commonly assessed using Cronbach's alpha.
TestRetest Reliability: Assesses the stability of responses over time by administering the same survey to the same respondents at different points in time.
3. Response Rate and Representation
Response Rate: The proportion of respondents who complete the survey. A higher response rate generally indicates more reliable data.
Representativeness: Ensures that the sample accurately represents the target population, which is crucial for generalizing findings.
4. Respondent Engagement
Completion Rates: The percentage of respondents who complete the survey, indicating their engagement level.
Dropout Analysis: Examines where and why respondents drop out to identify potential survey design issues.
5. Data Integrity
Consistency Checks: Regularly review data for consistency and coherence to identify any anomalies or patterns of response bias.
Response Quality: Assess the quality of openended responses for depth and relevance.
The Role of AI in Ensuring the Quality of Research Surveys
AI is transforming the landscape of research surveys by addressing inefficiencies and enhancing quality through advanced technologies and methodologies. Here’s how AI is making a difference.
1. AI in Survey Design
Natural Language Processing (NLP): AI-powered NLP tools analyze and refine question wording to ensure clarity and relevance. They also generate dynamic, personalized questions based on respondent answers.
Survey Optimization: AI algorithms predict optimal survey length and structure to maintain respondent engagement and minimize fatigue.
2. AI in Data Collection
Automated Sampling: AI automates the sampling process to ensure a representative sample and reduce selection biases.
Chatbots and Virtual Assistants: AI-driven chatbots conduct surveys conversationally, improving engagement and response accuracy by making the process more interactive and less formal.
Adaptive Surveys: AI creates adaptive surveys that adjust questions based on previous responses, enhancing relevance and reducing survey length.
3. AI in Data Management
Real-Time Analysis: AI surveys enable real-time data analysis, providing instant insights and allowing for immediate adjustments or interventions.
Data Cleaning and Validation: AI surveys automatically clean and validate data, identifying and correcting errors or inconsistencies without manual intervention.
Sentiment Analysis: AI-driven sentiment analysis tools evaluate open-ended responses, extracting valuable insights into respondent attitudes and emotions.
4. AI in Data Analysis
Pattern Recognition: AI identifies patterns and trends in large datasets, uncovering insights that might be missed by traditional analysis methods.
Predictive Analytics: AI models predict future trends based on current and historical data, offering foresight into potential developments and outcomes.
Theme Extraction: AI-native survey builders automatically extract and categorize themes from qualitative data, providing a structured understanding of complex responses.
5. AI in Enhancing Respondent Engagement
Personalization: AI-native survey builders like Metaforms personalise the survey experience by tailoring questions and content to the individual respondent, increasing engagement and data relevance.
Gamification: AI survey builders introduce gamified elements into surveys, making the process more engaging and improving response rates.
Conclusion: The Quality of Research Surveys using AI
Elevating the quality of research surveys is imperative for obtaining reliable and actionable insights. While traditional methods face several inefficiencies, AIdriven innovations offer transformative solutions that enhance survey design, data collection, management, and analysis. By addressing common issues such as ambiguous questions, low response rates, and data inconsistencies, AI empowers researchers to conduct more effective and highquality surveys.
For qualitative researchers, understanding and leveraging AI in survey research bridges gaps in validity, reliability, and respondent engagement, ensuring that the surveys not only meet but exceed quality standards. As AI technology continues to evolve, its role in refining research methodologies and delivering precise insights will only grow, paving the way for more informed decisions and successful outcomes in the research landscape.
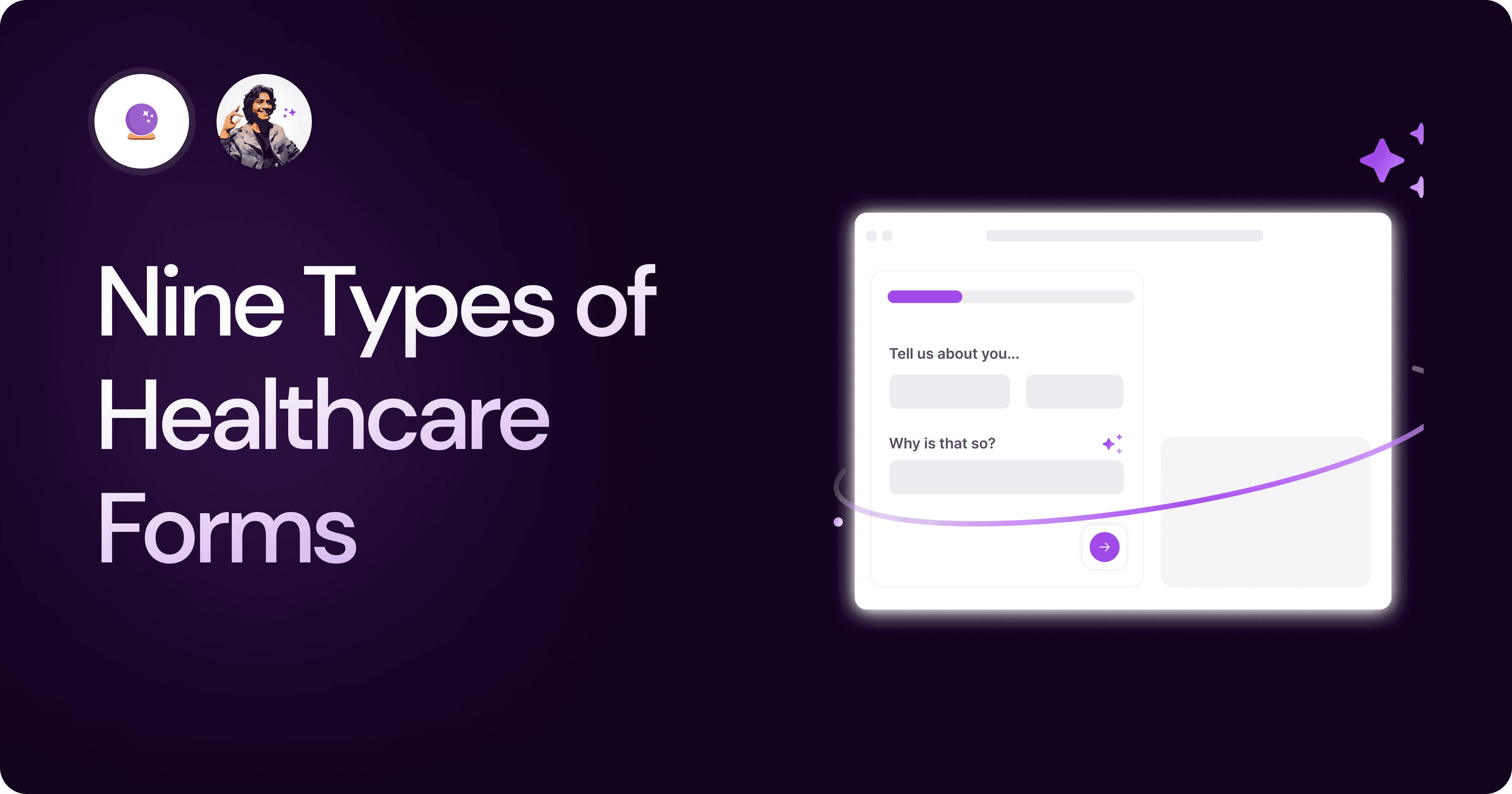
Nine Types of Healthcare and Medical Forms.
Medical forms are a must-have for any healthcare business or practitioner. Learn about the different kinds of medical and healthcare forms.
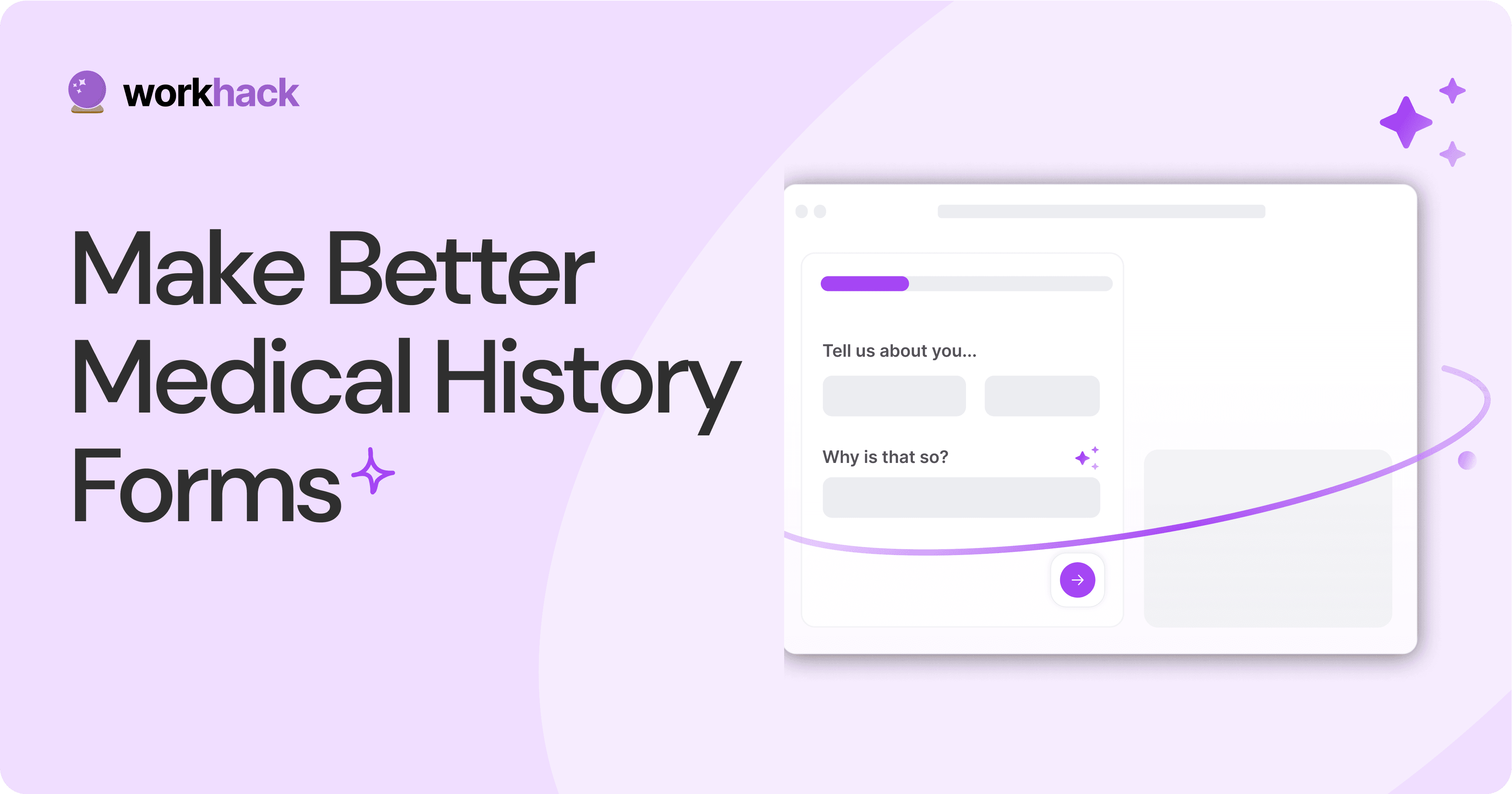
4 Tips for Better Medical History Forms.
Medical history forms are central to patient care, onboarding, and medical administration records. Learn how to make them easier to fill.
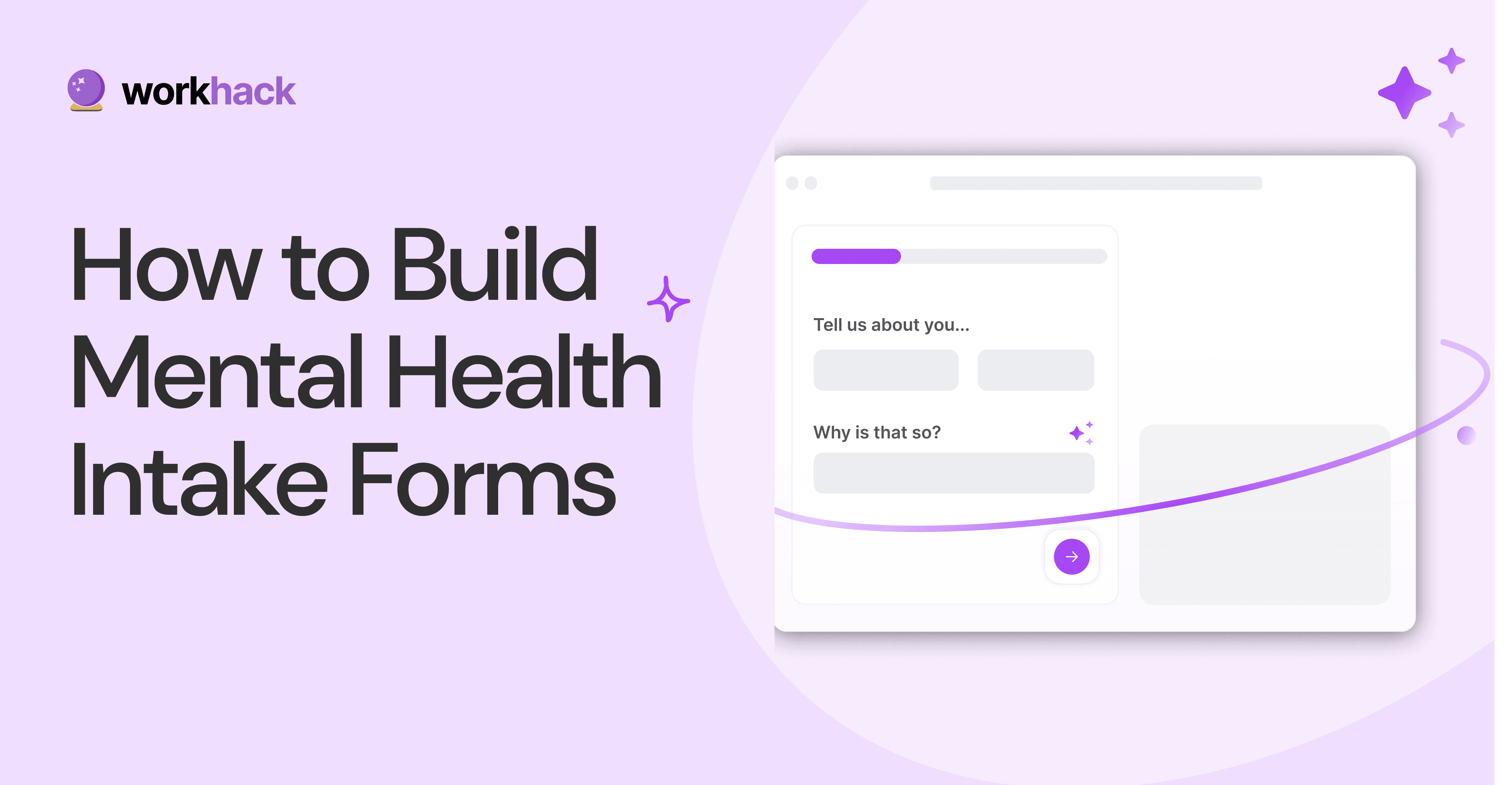
How to Build Mental Health Intake Forms?
Mental health intake forms are not like patient intake forms. Mental health intake forms deal with far more sensitive data and have specific design methods.
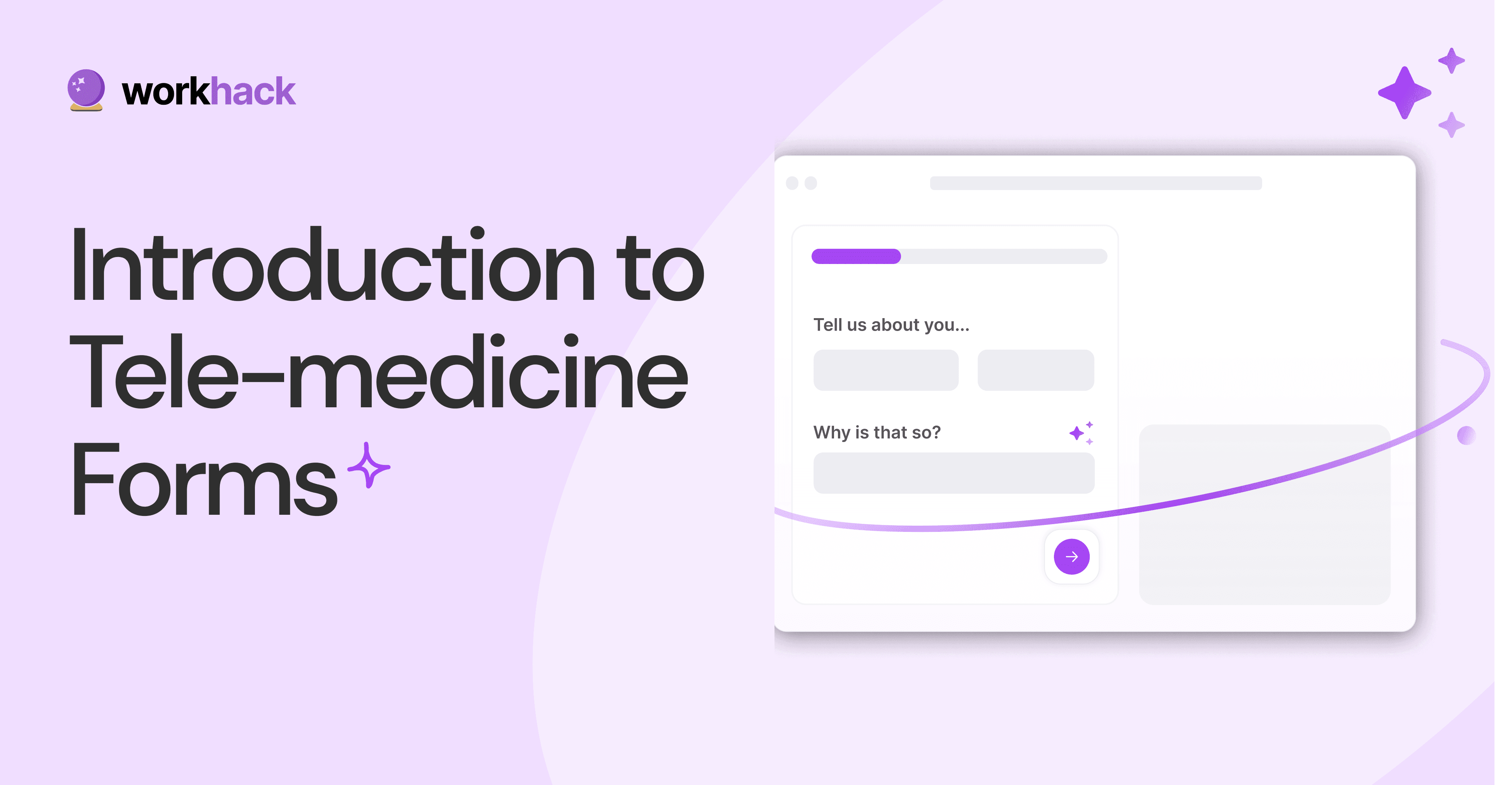
What, Why and How of Telemedicine Forms.
Telemedicine is on the rise and with different form builders out there, which one best suits your needs as a healthcare services provider?
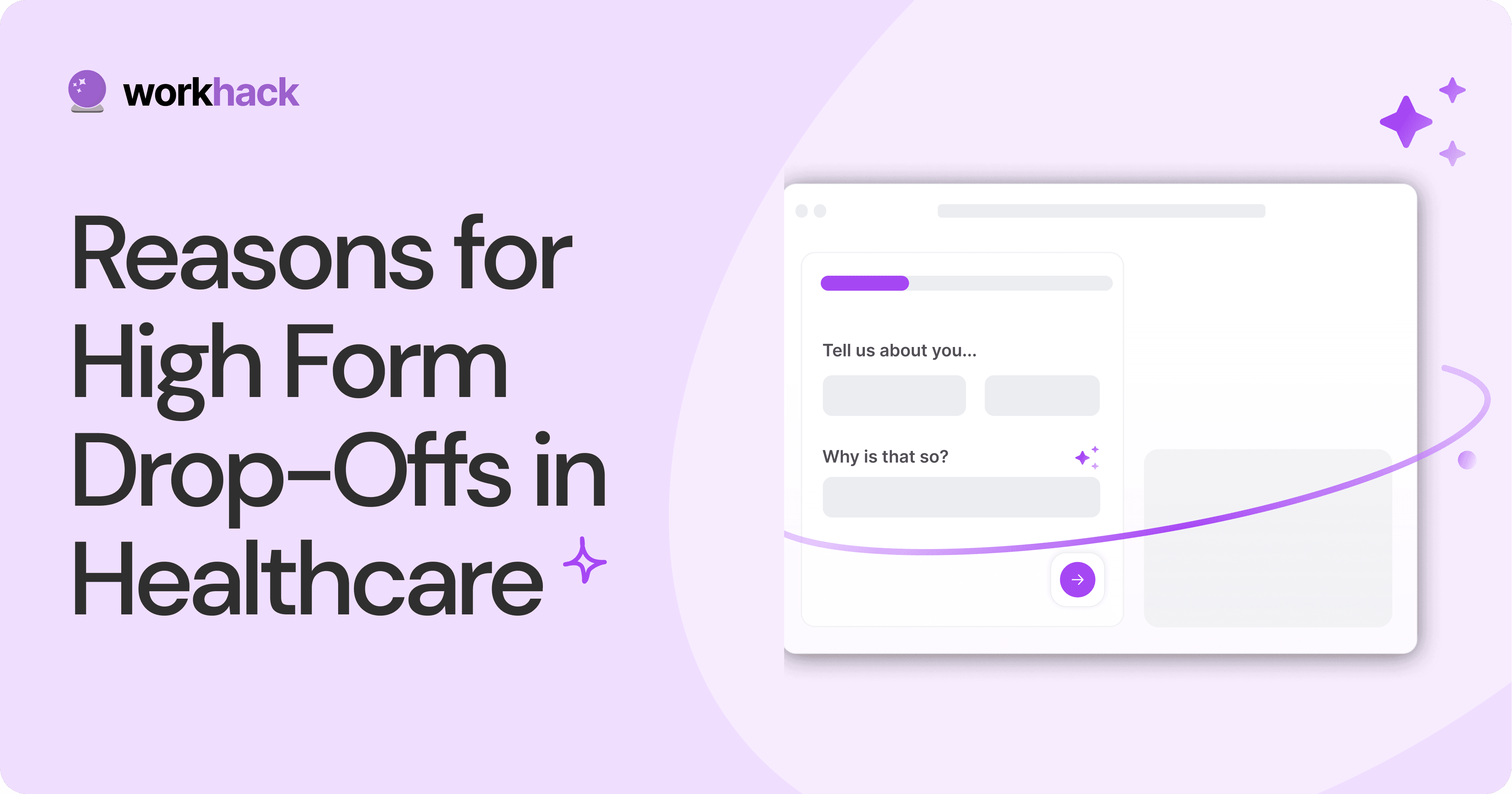
3 Reasons for Major Drop-Offs in Medical Forms.
No matter which healthcare form we pick, there are major drop-off reasons. We shall dive into the top 3 and learn how to resolve them in your next form.
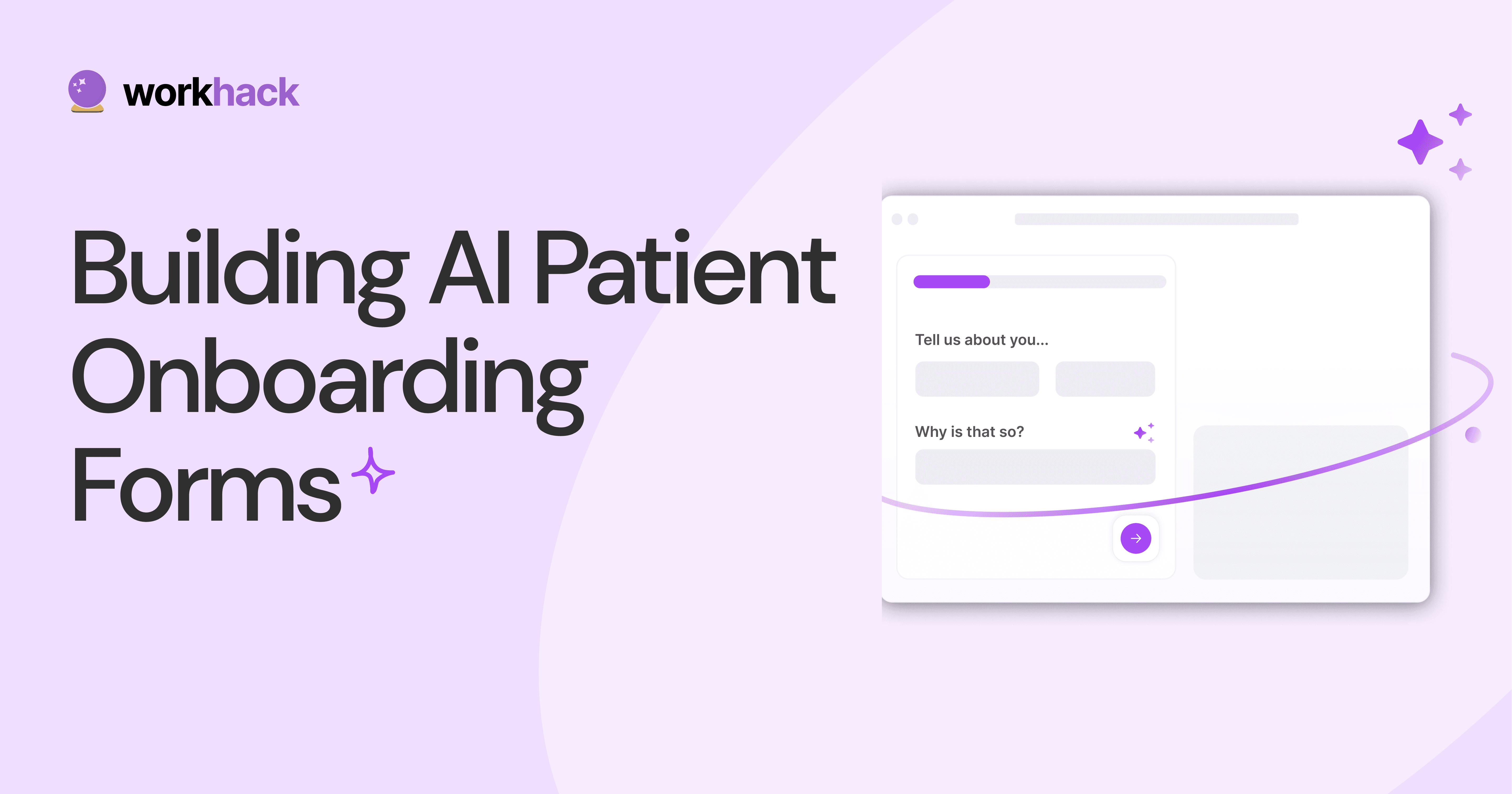
Patient Onboarding Forms - From Click to Clinic.
Patient onboarding forms are the first touchpoint for patients; getting this right for higher conversion rates is a must-have. Learn how to perfect them now.

5 Key Parts of a Good Patient Satisfaction Form.
The goal of patient satisfaction surveys is to course-correct the services of a healthcare provider. Patient feedback leads to a culture of patient-centric care.
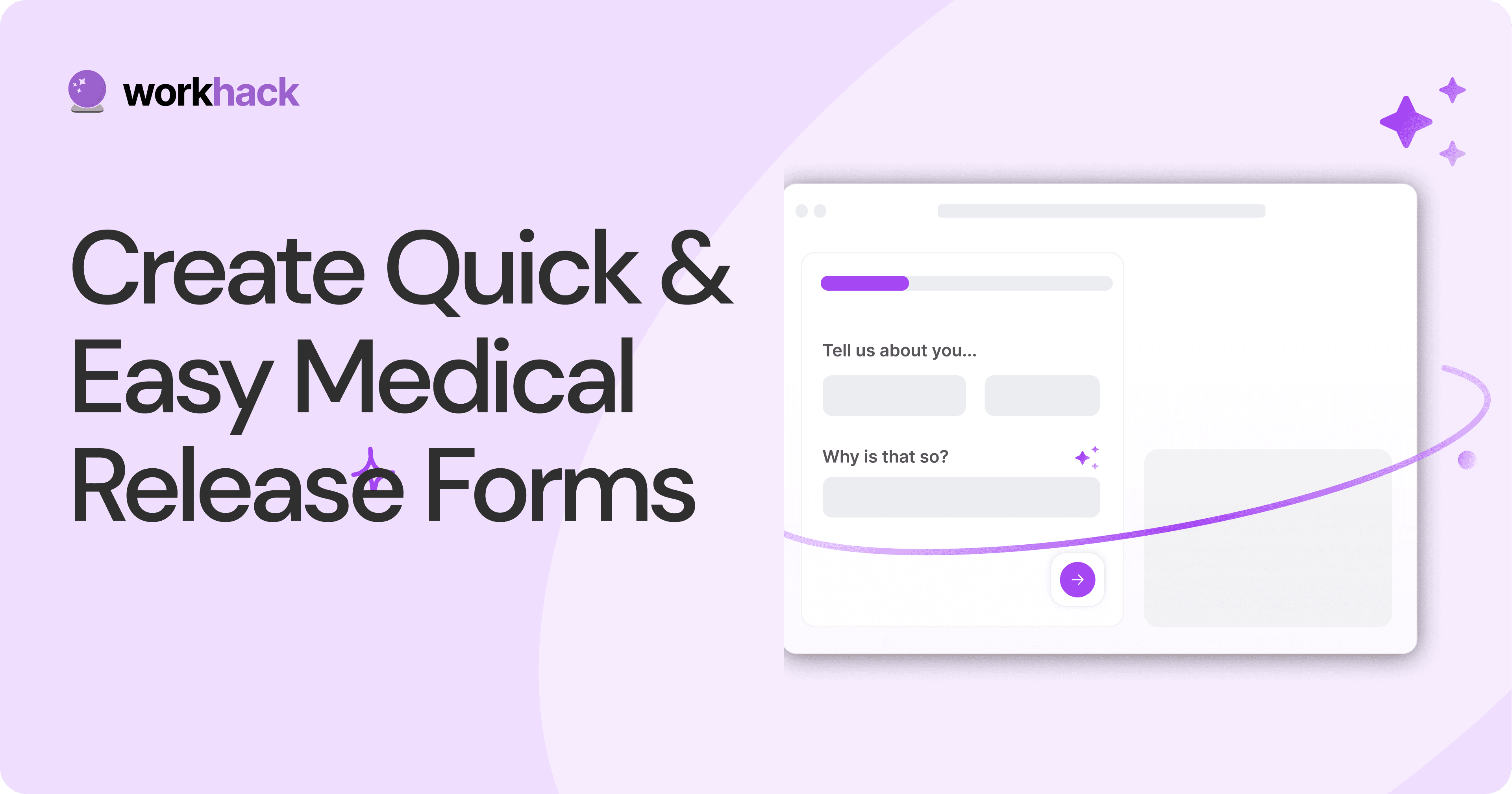
Build Quick and Easy Medical Release Forms.
Every HIPAA-compliant healthcare provider comes across medical release forms that involve details from medical history forms. Can they be shipped fast? Yes.
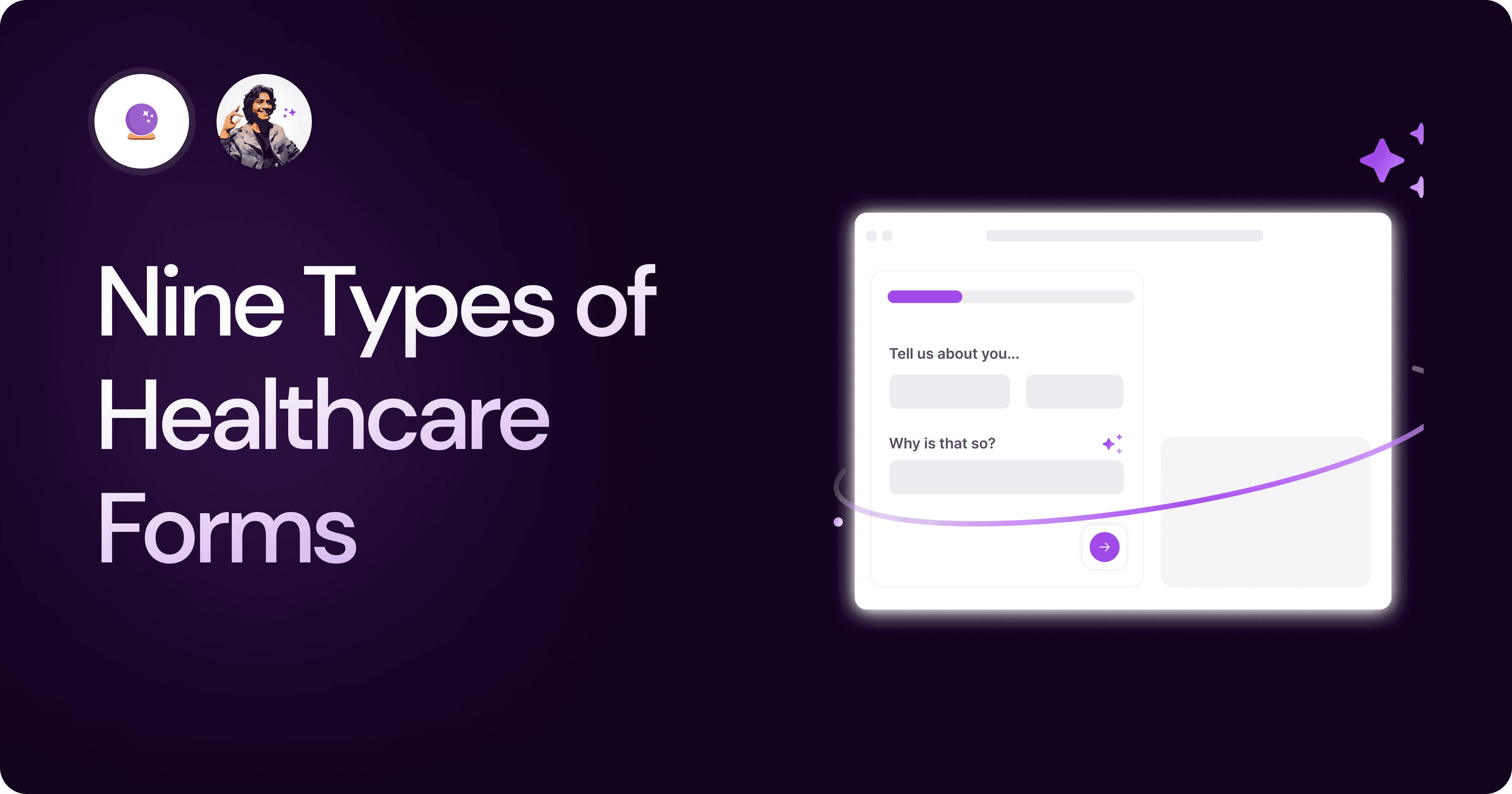
Nine Types of Healthcare and Medical Forms.
Medical forms are a must-have for any healthcare business or practitioner. Learn about the different kinds of medical and healthcare forms.
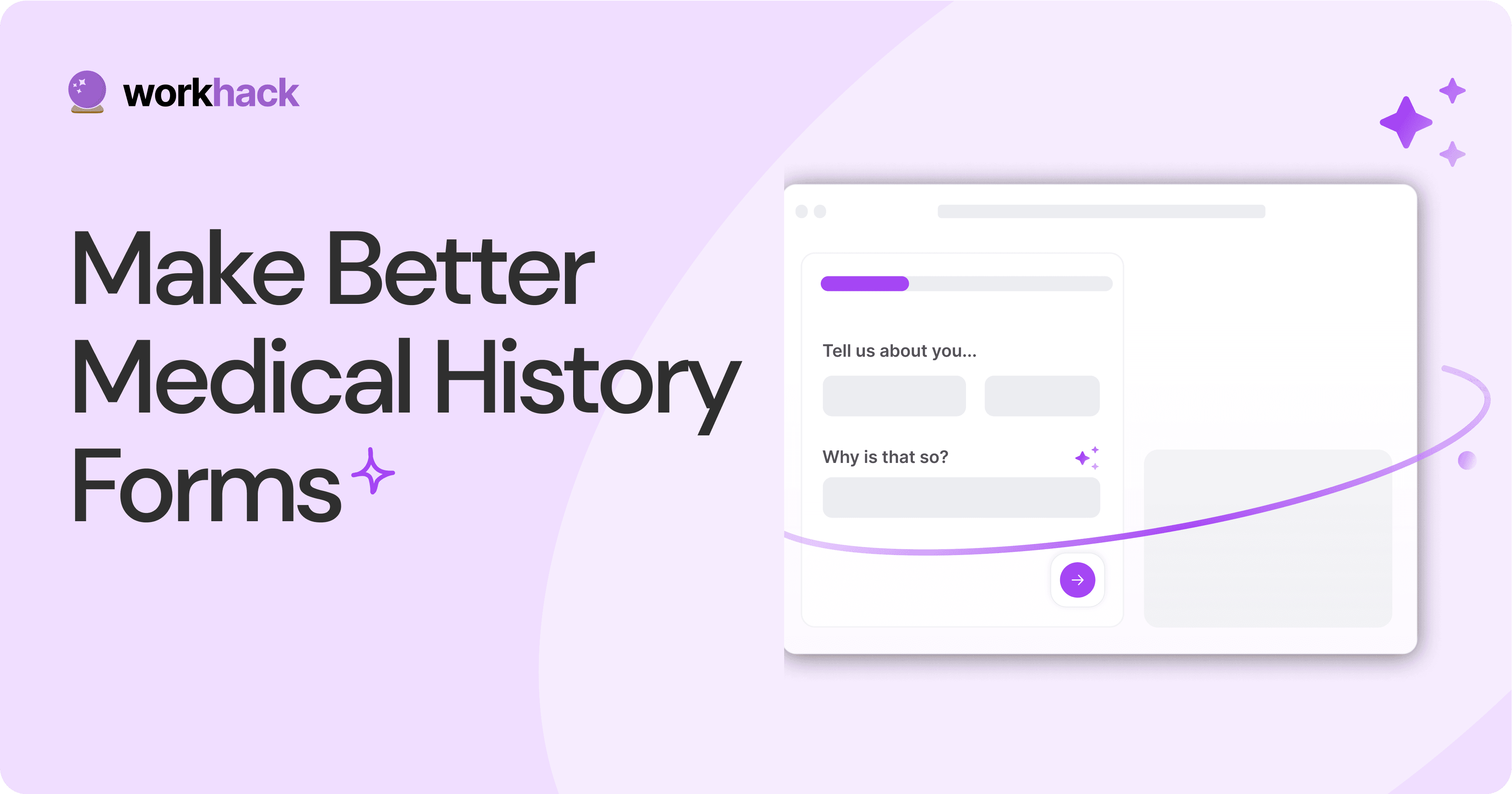
4 Tips for Better Medical History Forms.
Medical history forms are central to patient care, onboarding, and medical administration records. Learn how to make them easier to fill.
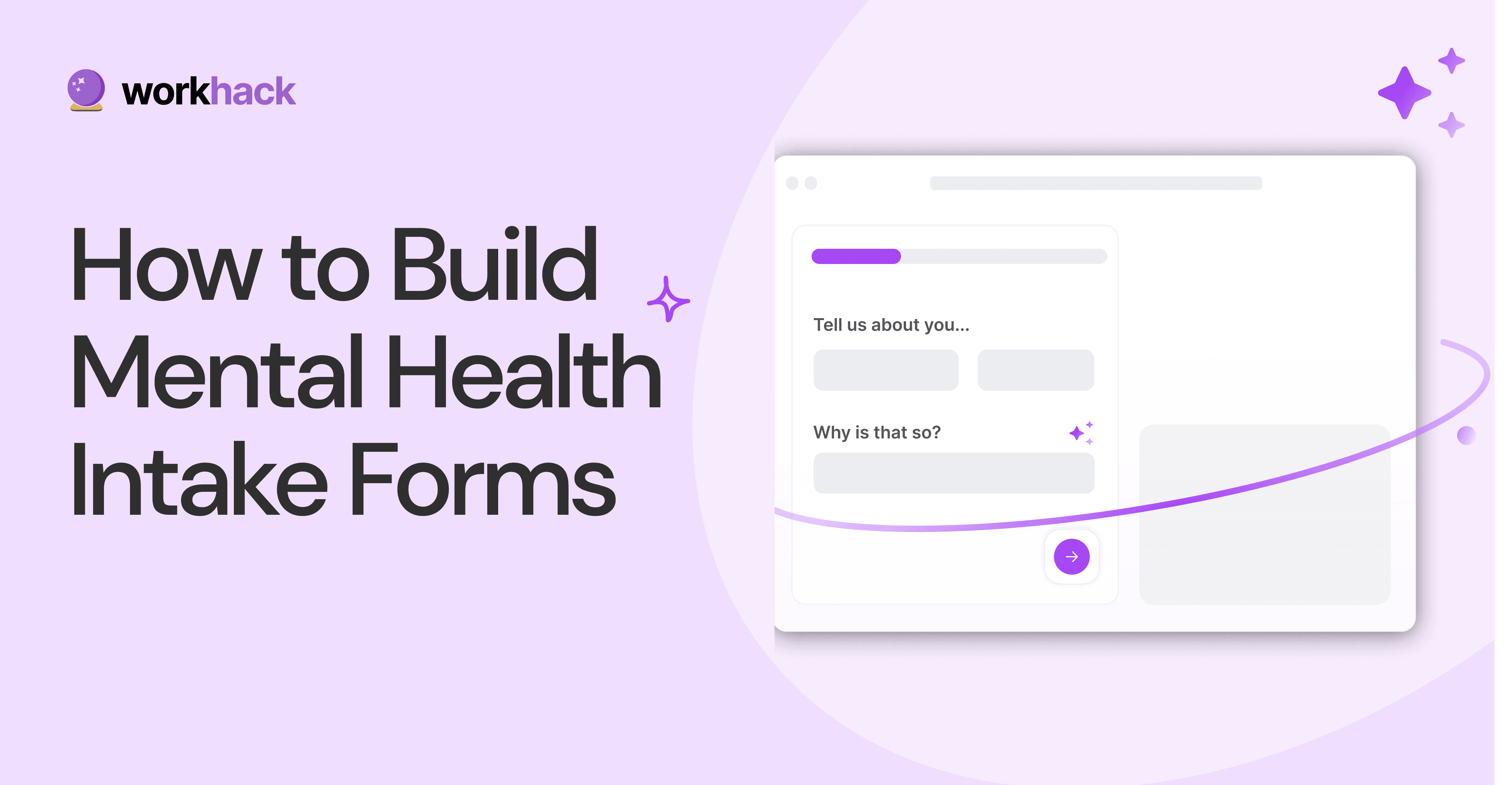
How to Build Mental Health Intake Forms?
Mental health intake forms are not like patient intake forms. Mental health intake forms deal with far more sensitive data and have specific design methods.
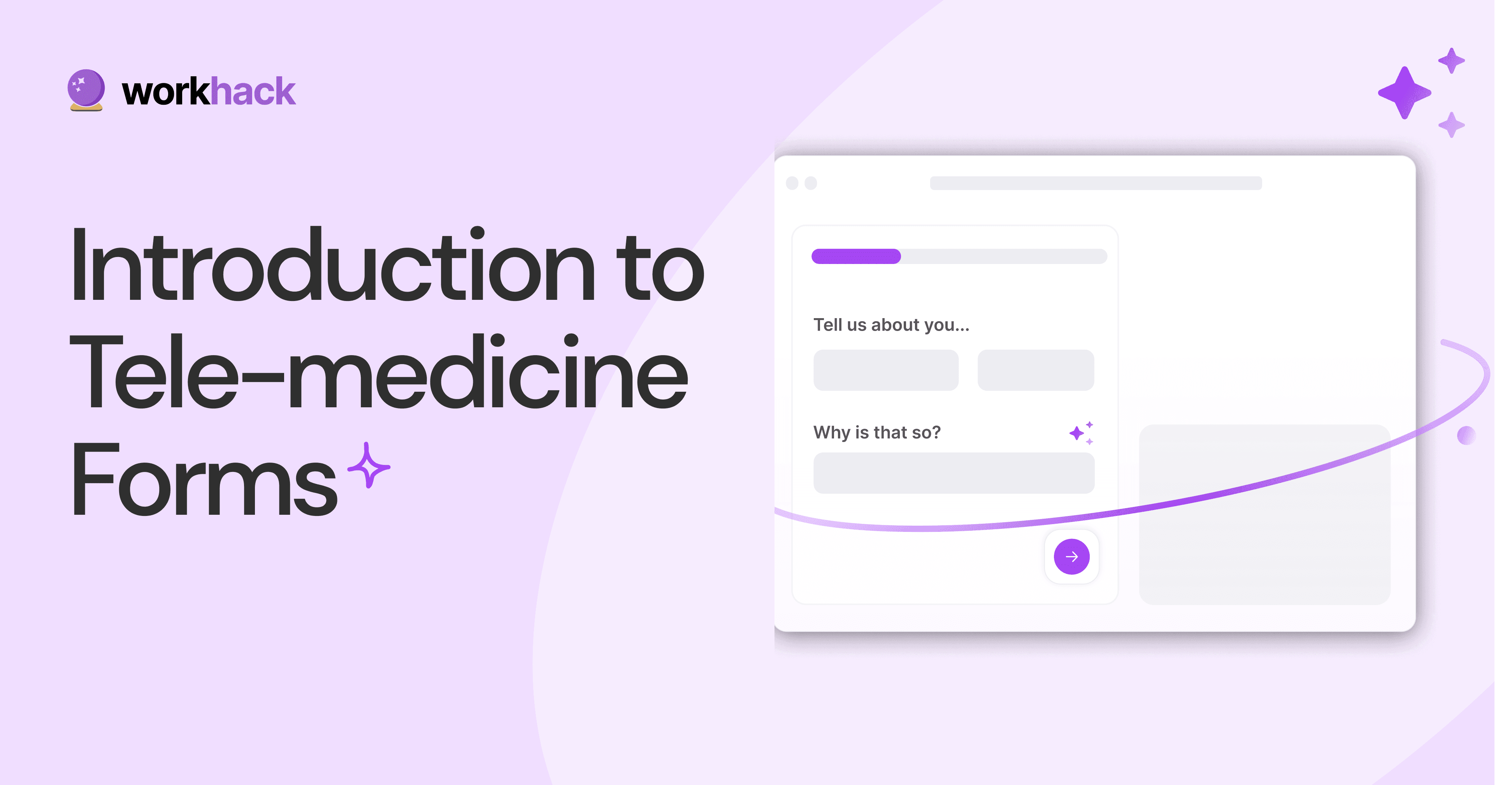
What, Why and How of Telemedicine Forms.
Telemedicine is on the rise and with different form builders out there, which one best suits your needs as a healthcare services provider?
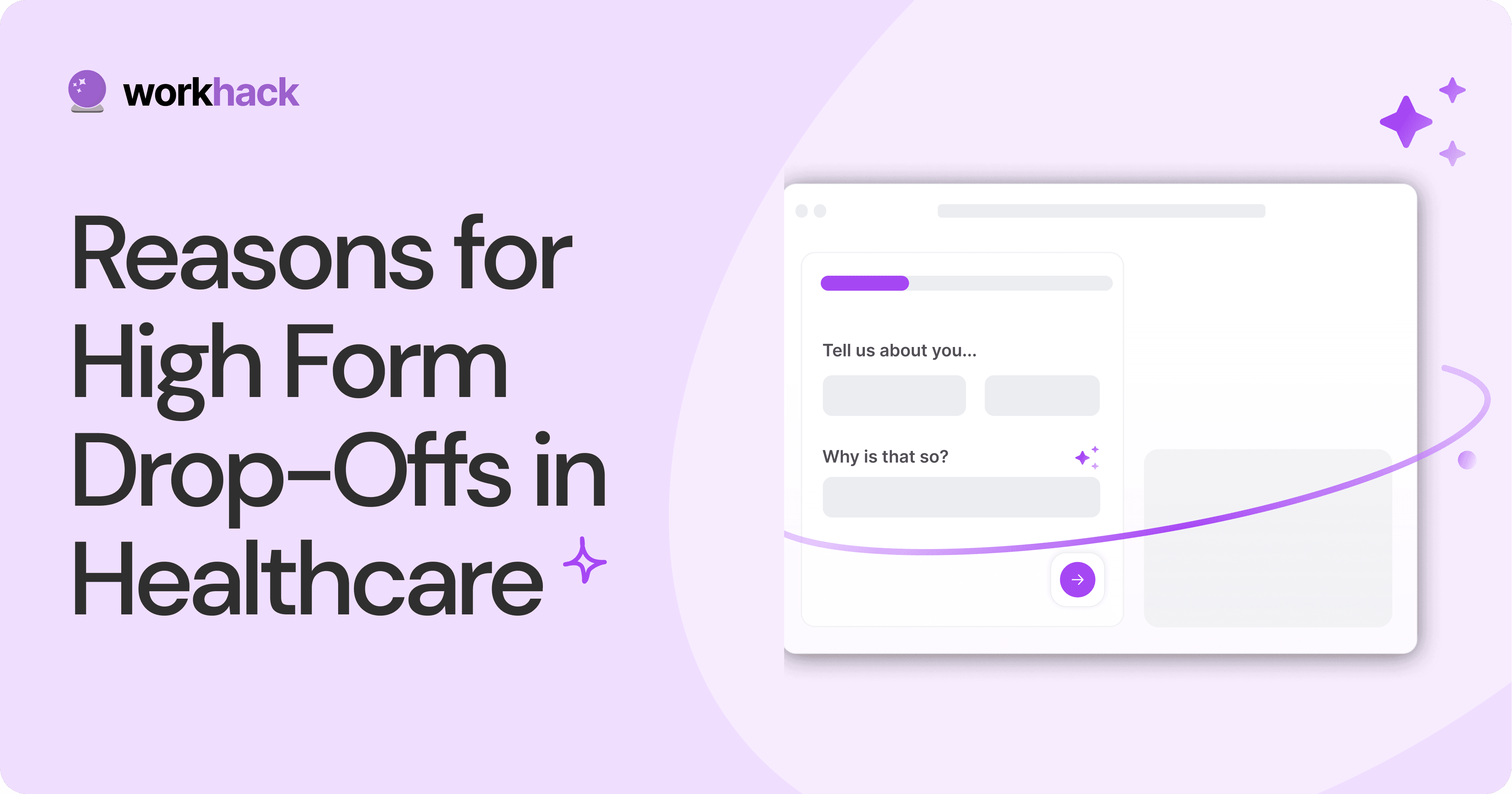
3 Reasons for Major Drop-Offs in Medical Forms.
No matter which healthcare form we pick, there are major drop-off reasons. We shall dive into the top 3 and learn how to resolve them in your next form.
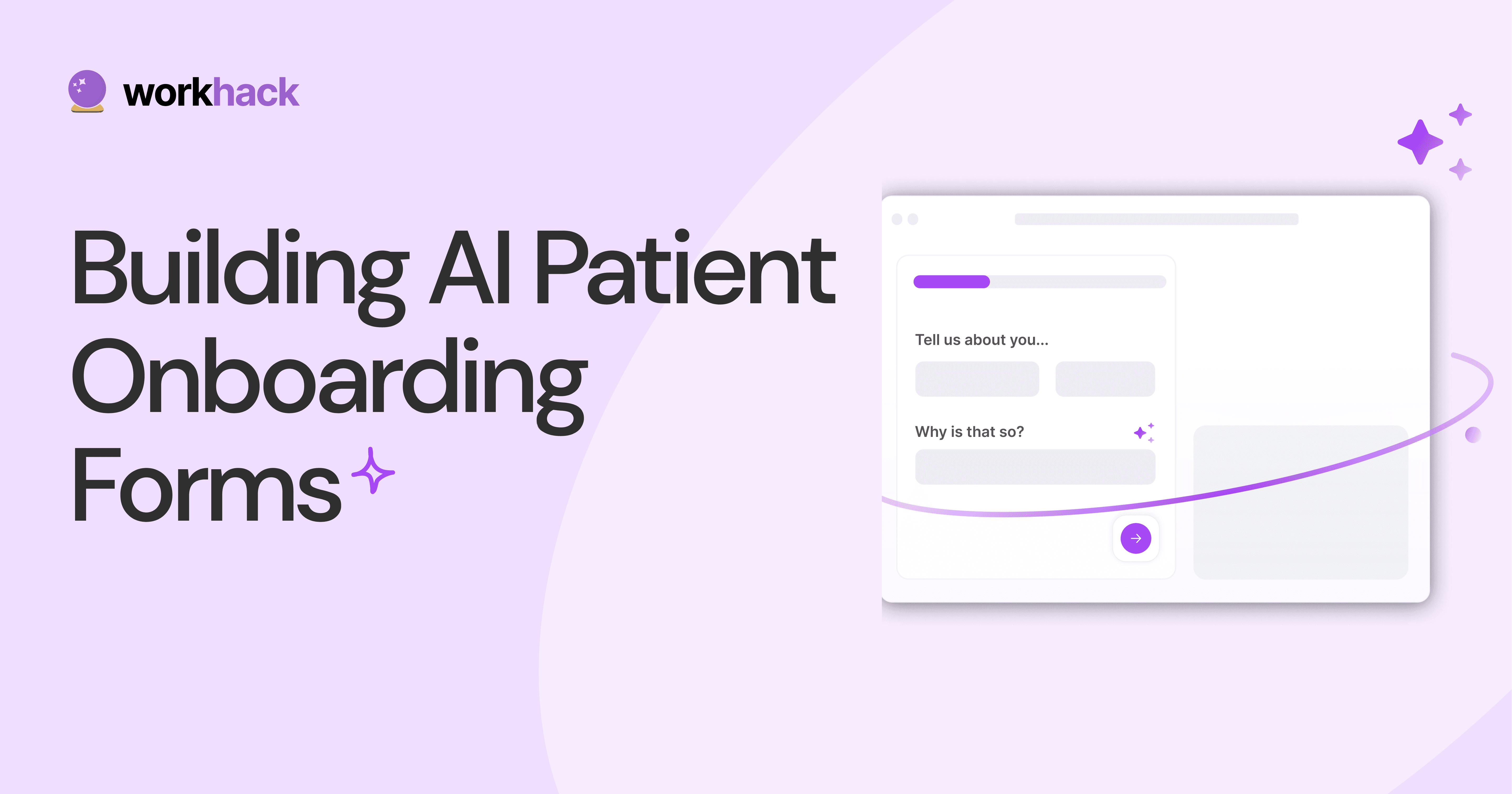
Patient Onboarding Forms - From Click to Clinic.
Patient onboarding forms are the first touchpoint for patients; getting this right for higher conversion rates is a must-have. Learn how to perfect them now.

5 Key Parts of a Good Patient Satisfaction Form.
The goal of patient satisfaction surveys is to course-correct the services of a healthcare provider. Patient feedback leads to a culture of patient-centric care.
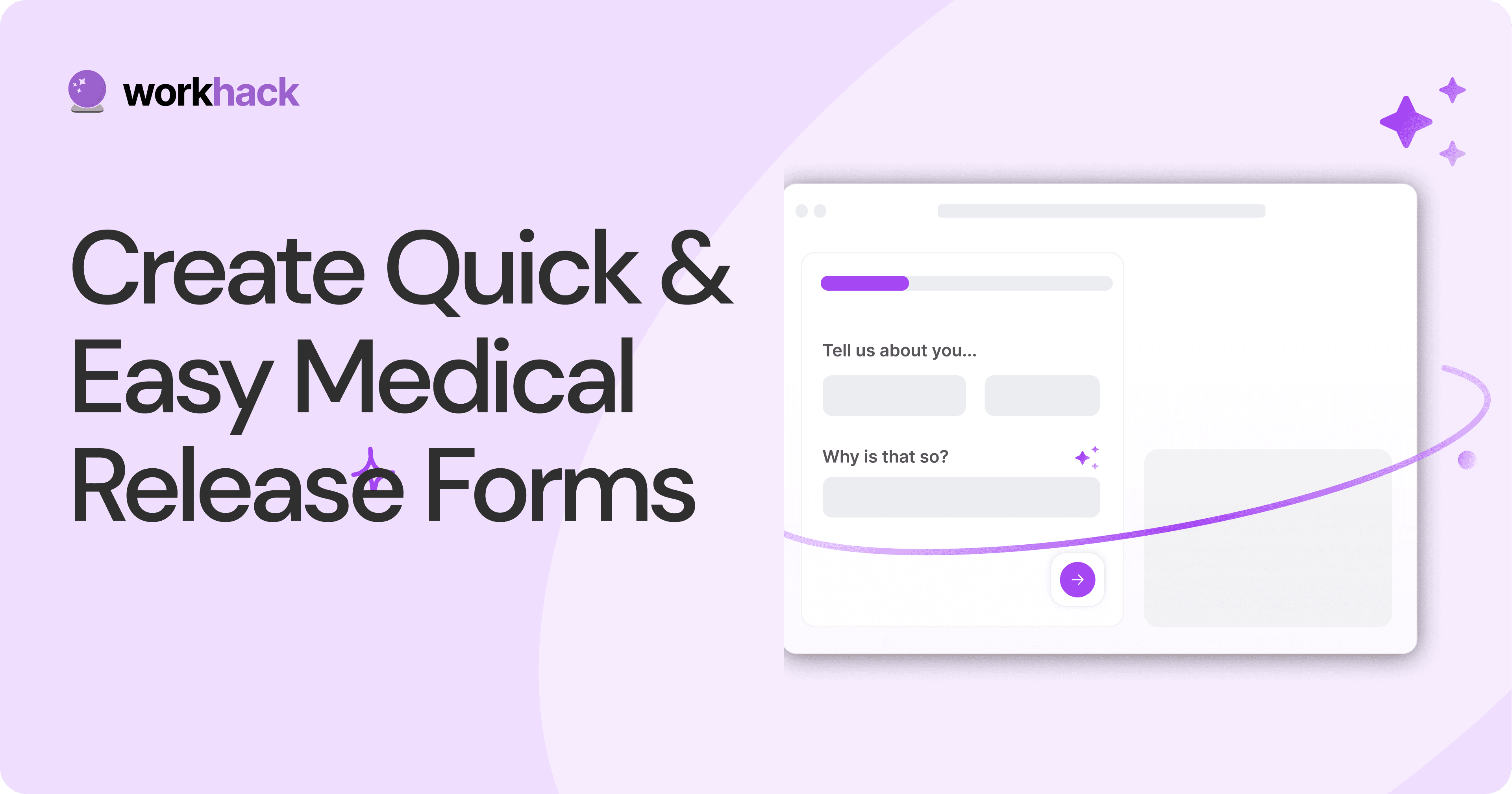
Build Quick and Easy Medical Release Forms.
Every HIPAA-compliant healthcare provider comes across medical release forms that involve details from medical history forms. Can they be shipped fast? Yes.
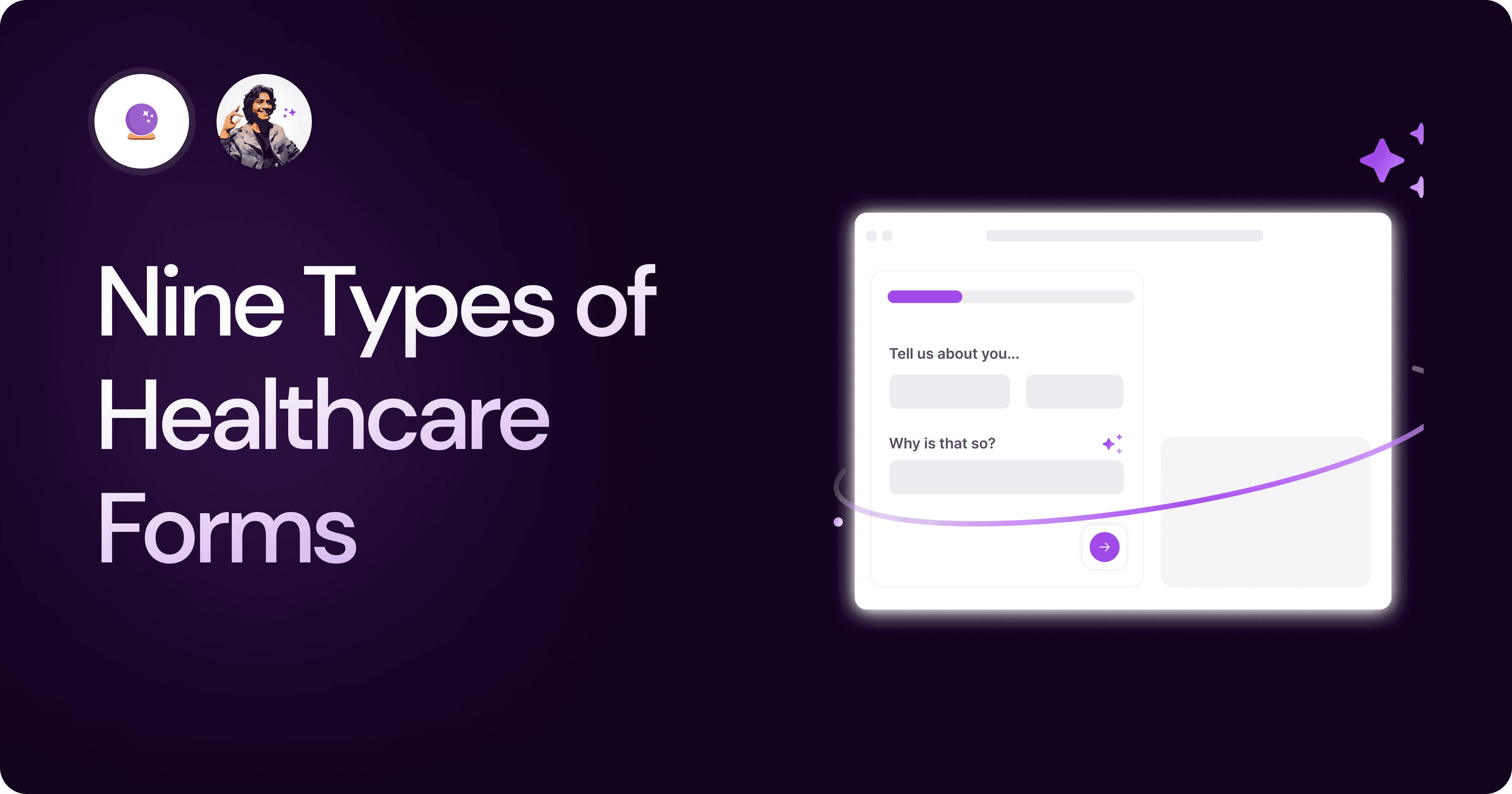
Nine Types of Healthcare and Medical Forms.
Medical forms are a must-have for any healthcare business or practitioner. Learn about the different kinds of medical and healthcare forms.
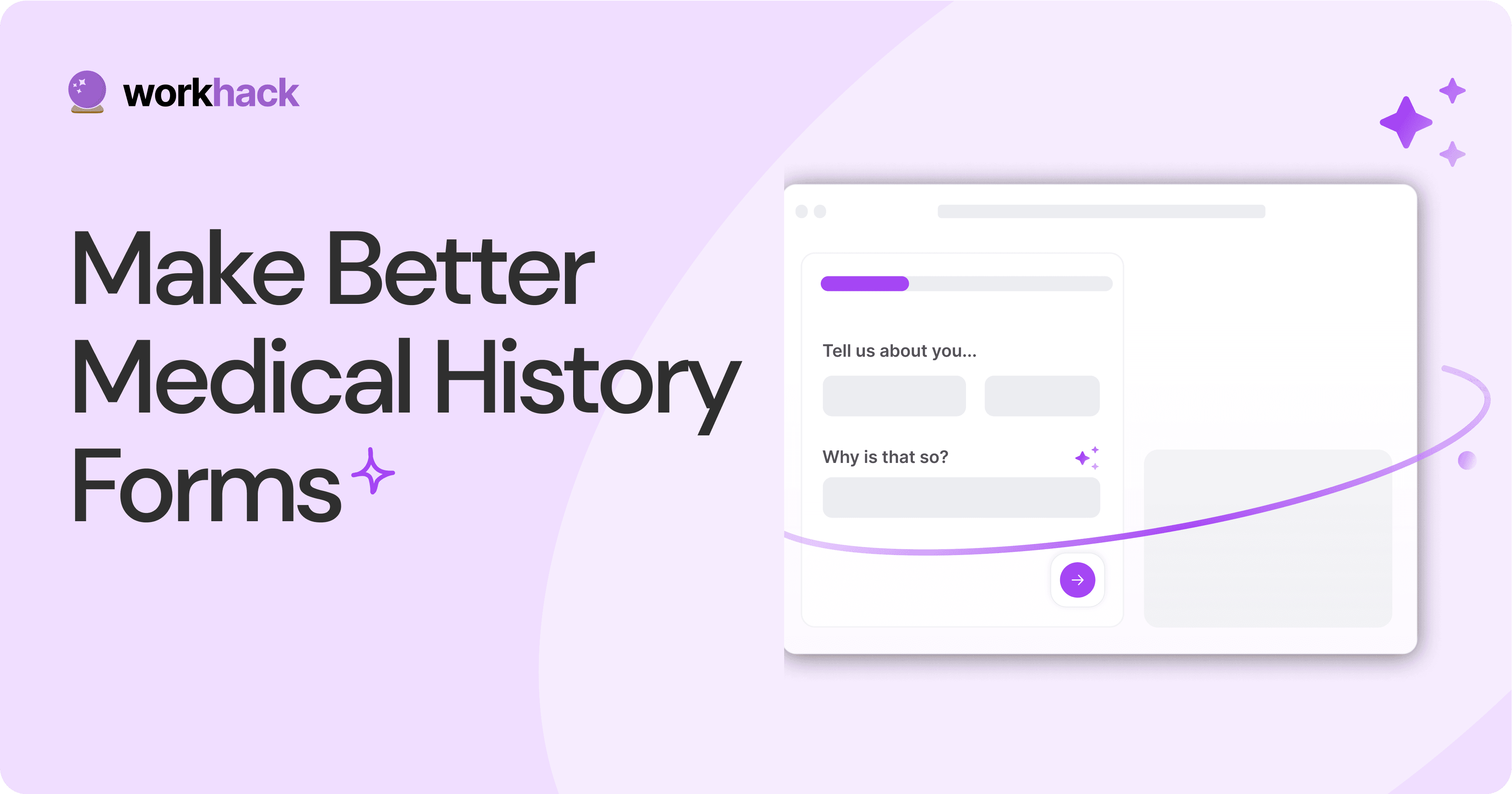
4 Tips for Better Medical History Forms.
Medical history forms are central to patient care, onboarding, and medical administration records. Learn how to make them easier to fill.
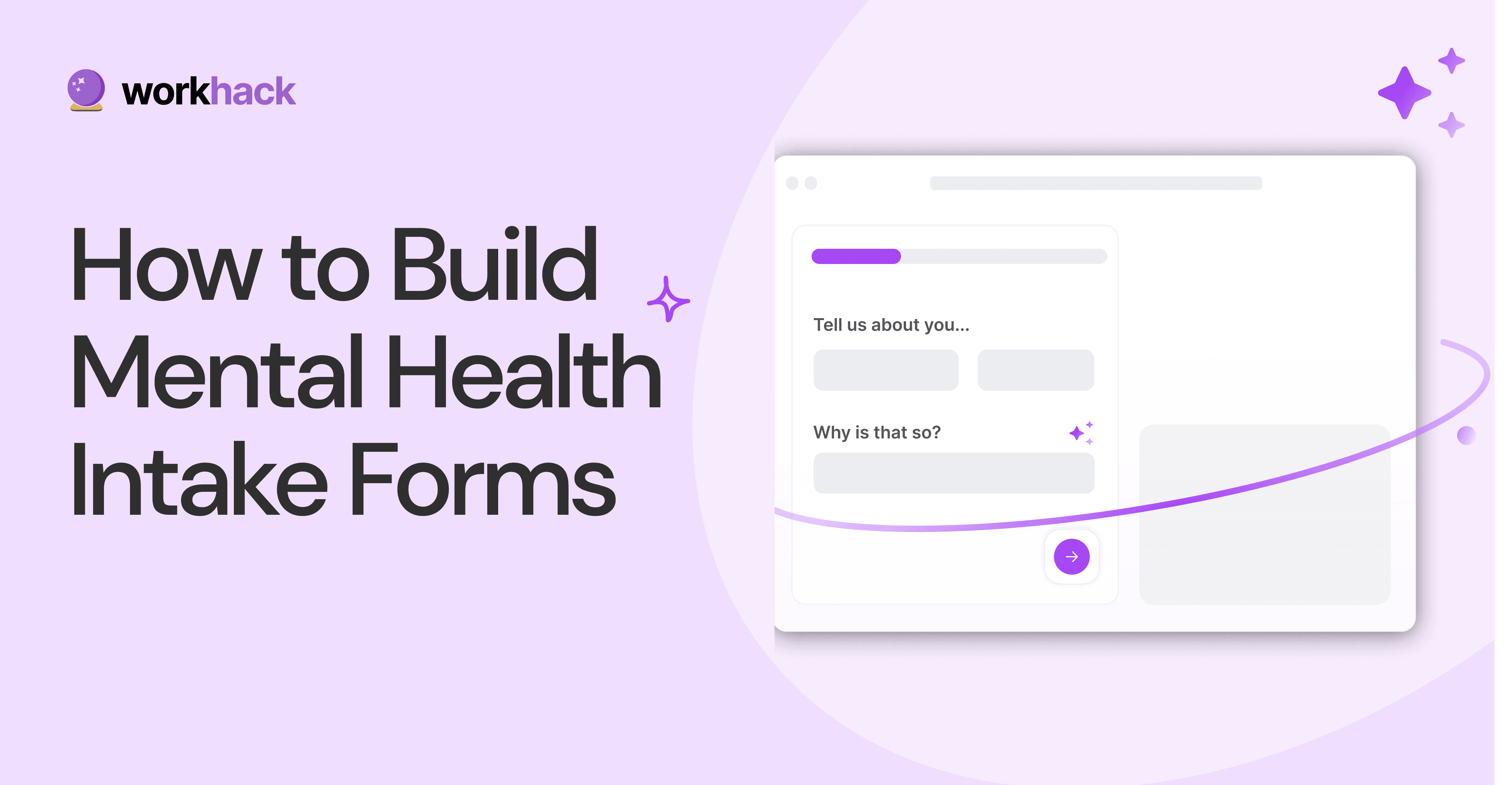
How to Build Mental Health Intake Forms?
Mental health intake forms are not like patient intake forms. Mental health intake forms deal with far more sensitive data and have specific design methods.
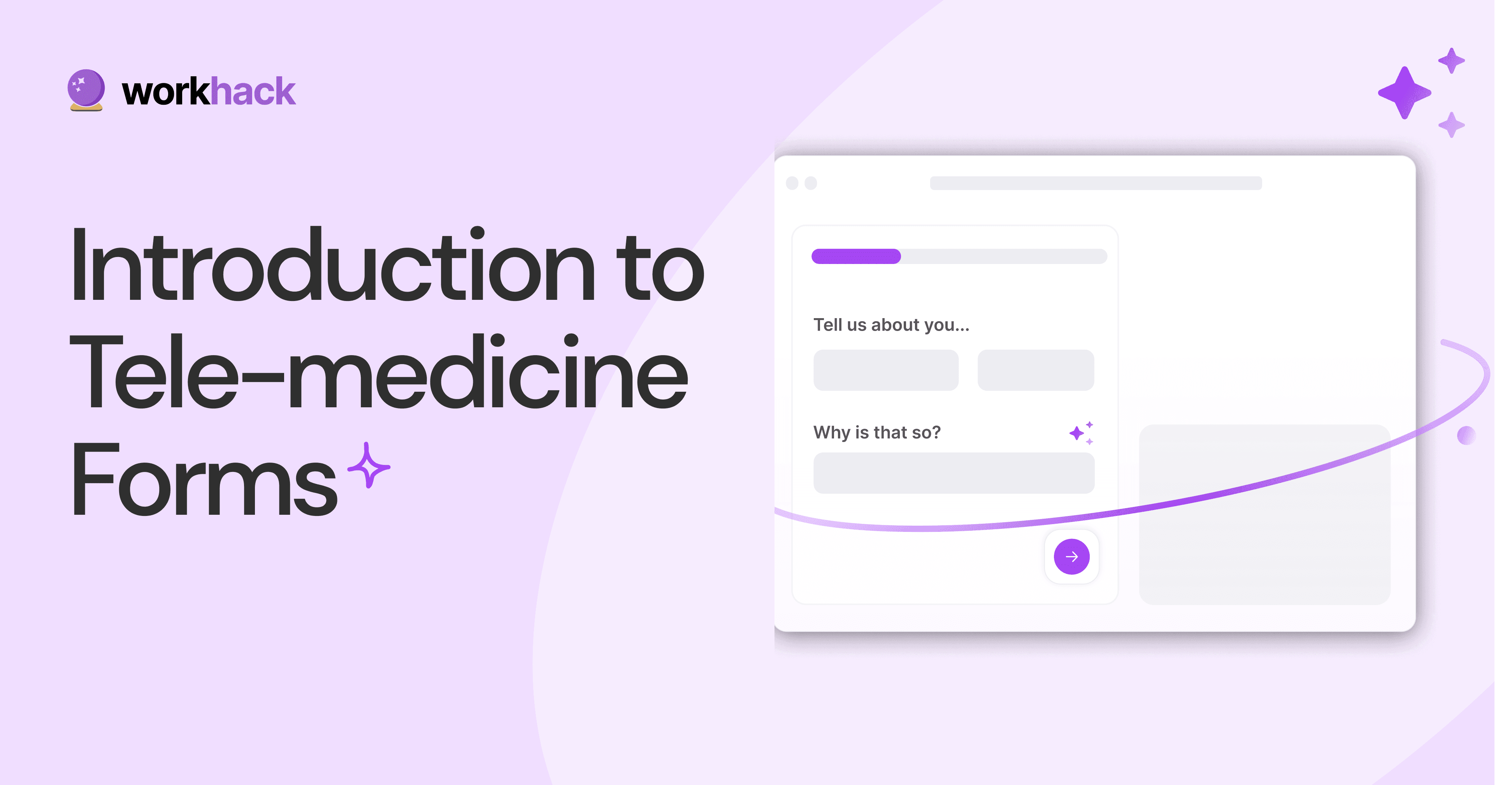
What, Why and How of Telemedicine Forms.
Telemedicine is on the rise and with different form builders out there, which one best suits your needs as a healthcare services provider?
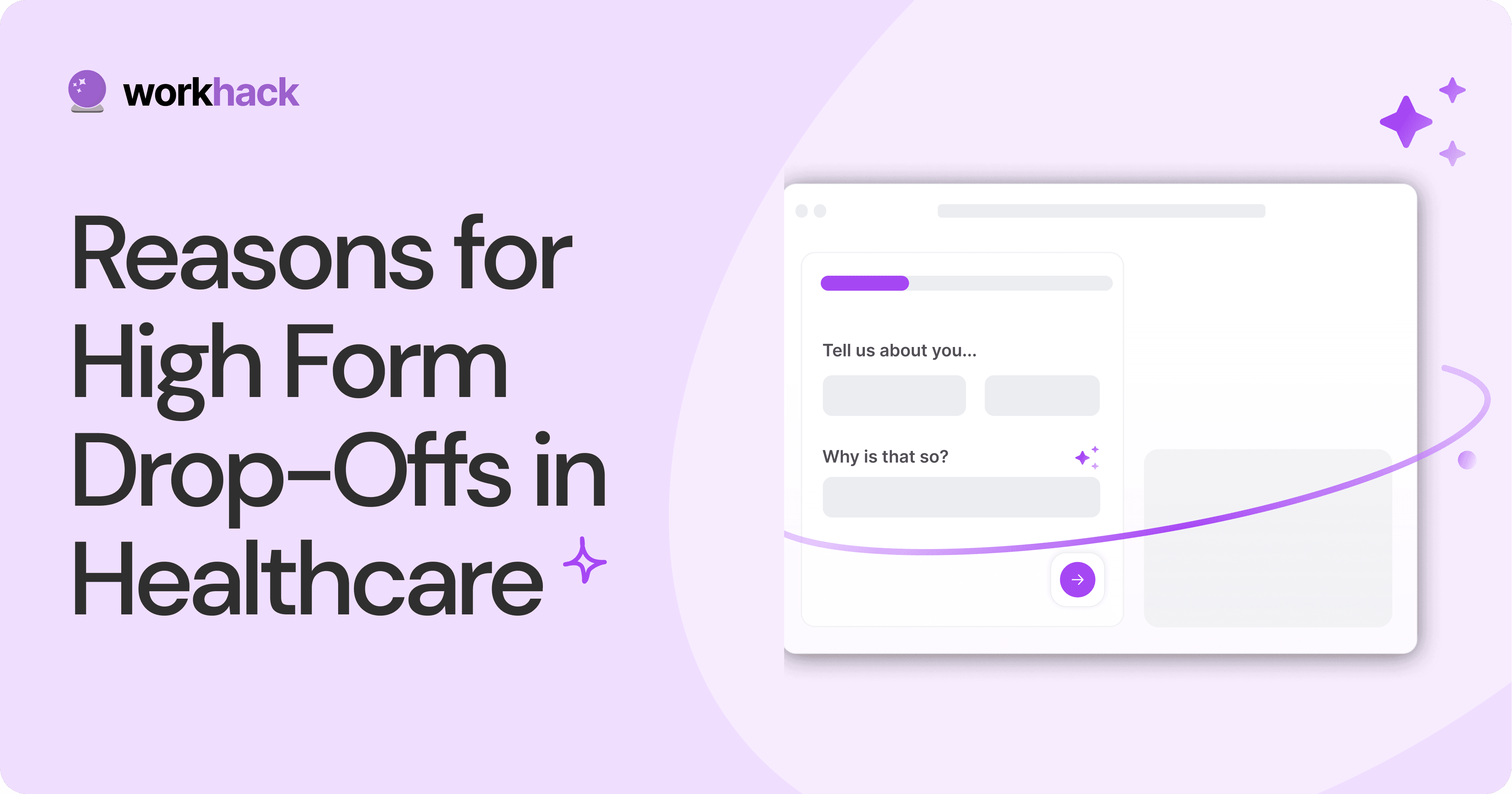
3 Reasons for Major Drop-Offs in Medical Forms.
No matter which healthcare form we pick, there are major drop-off reasons. We shall dive into the top 3 and learn how to resolve them in your next form.
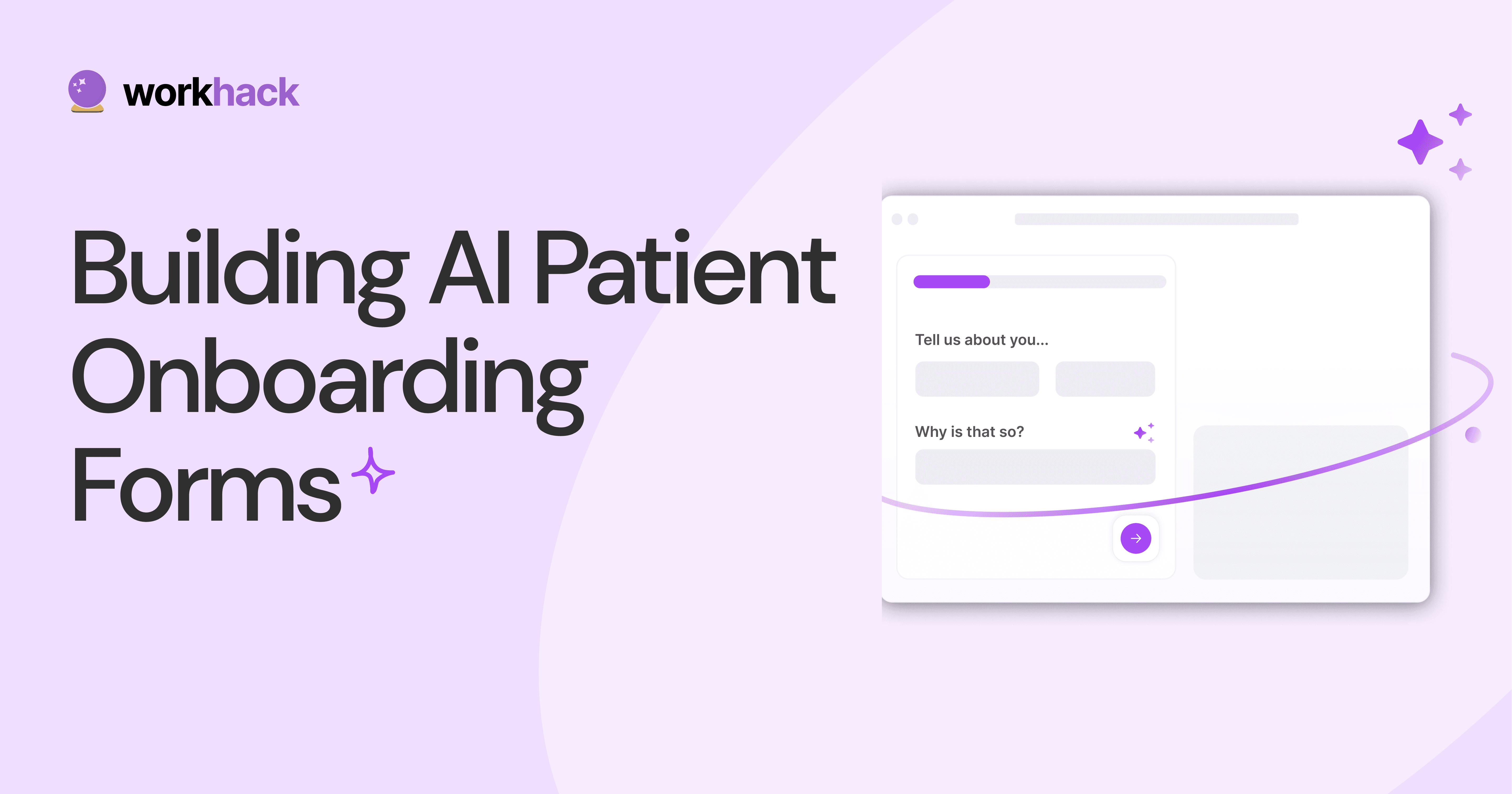
Patient Onboarding Forms - From Click to Clinic.
Patient onboarding forms are the first touchpoint for patients; getting this right for higher conversion rates is a must-have. Learn how to perfect them now.

5 Key Parts of a Good Patient Satisfaction Form.
The goal of patient satisfaction surveys is to course-correct the services of a healthcare provider. Patient feedback leads to a culture of patient-centric care.
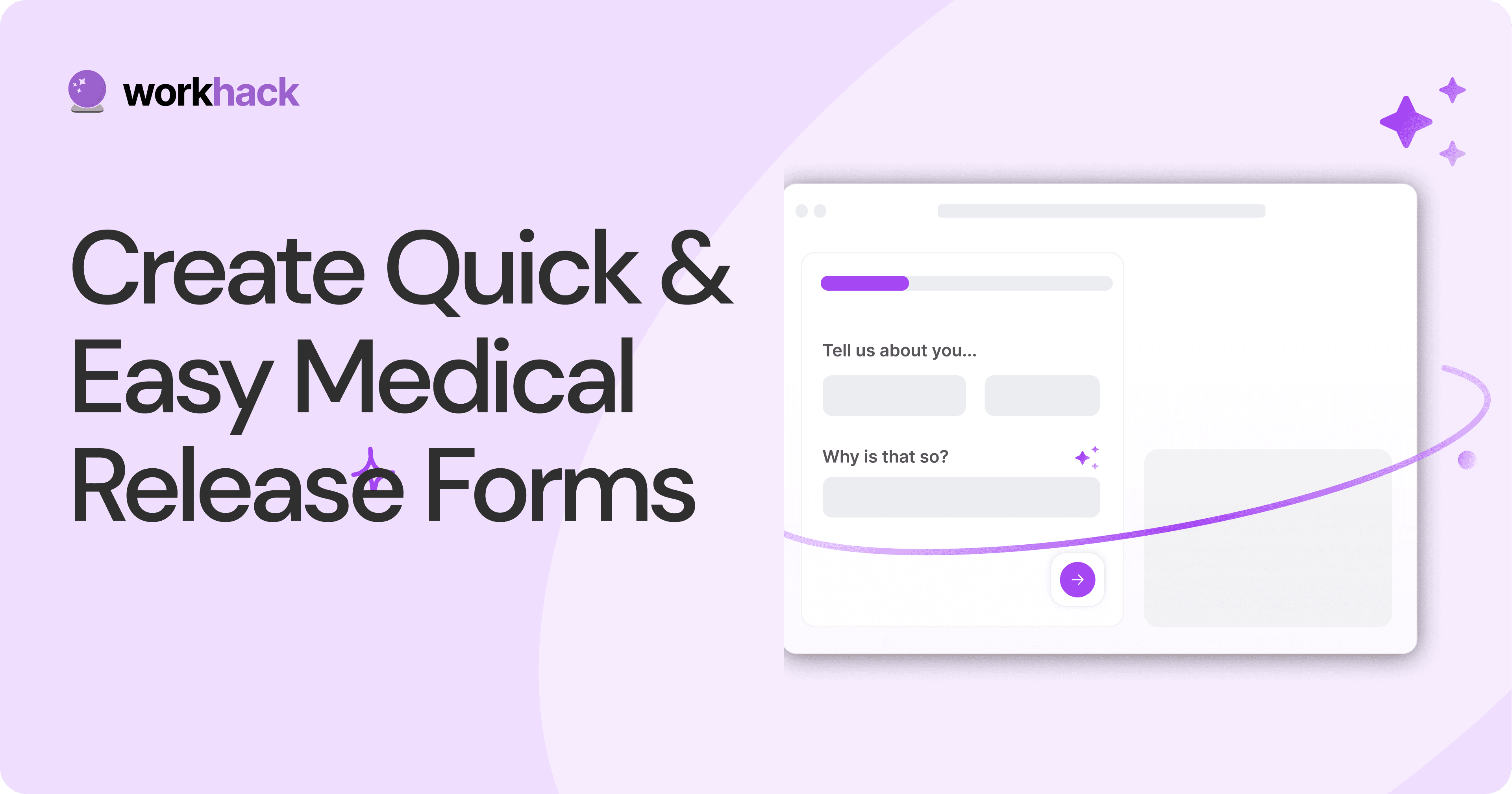
Build Quick and Easy Medical Release Forms.
Every HIPAA-compliant healthcare provider comes across medical release forms that involve details from medical history forms. Can they be shipped fast? Yes.
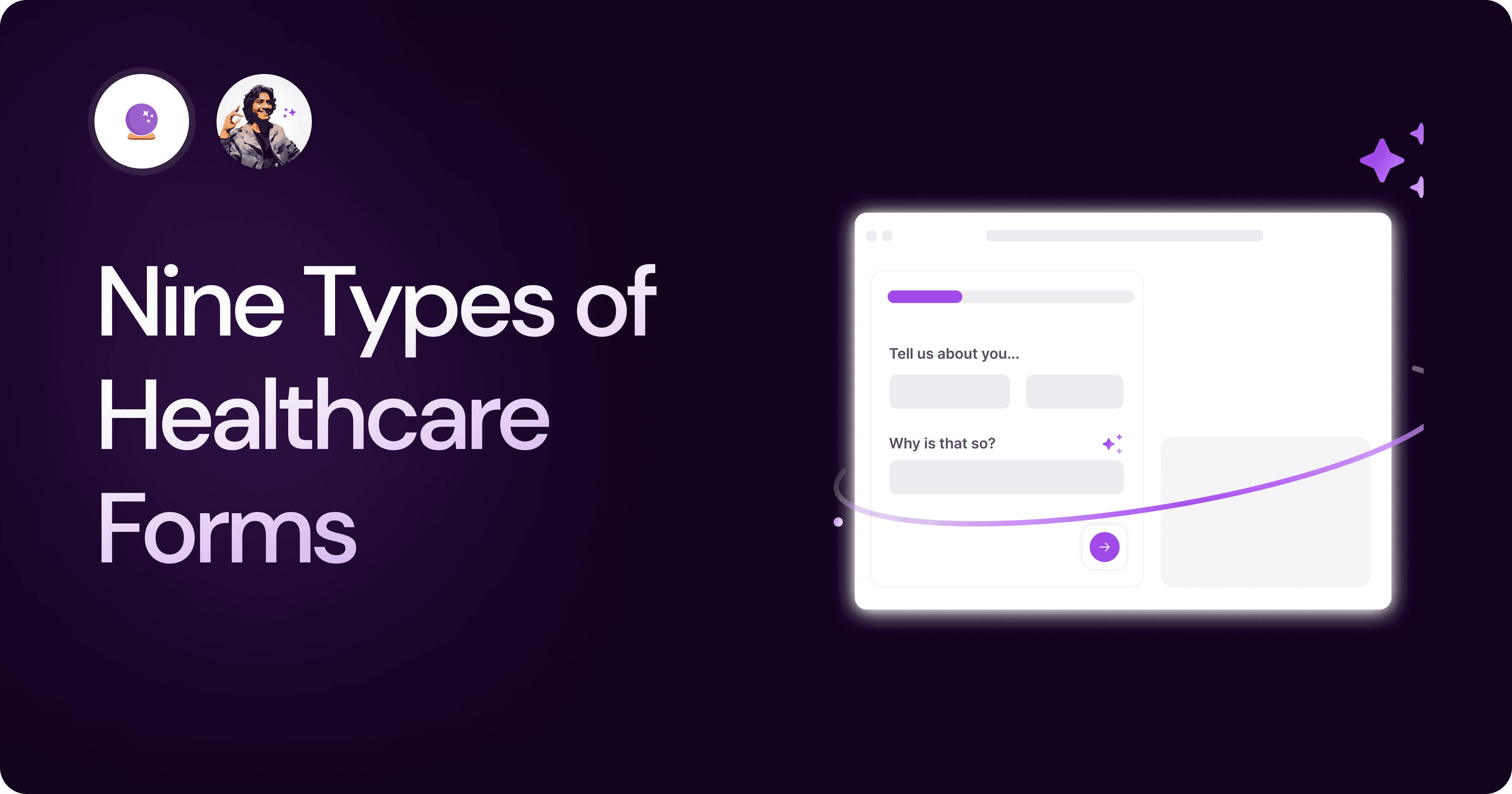
Nine Types of Healthcare and Medical Forms.
Medical forms are a must-have for any healthcare business or practitioner. Learn about the different kinds of medical and healthcare forms.
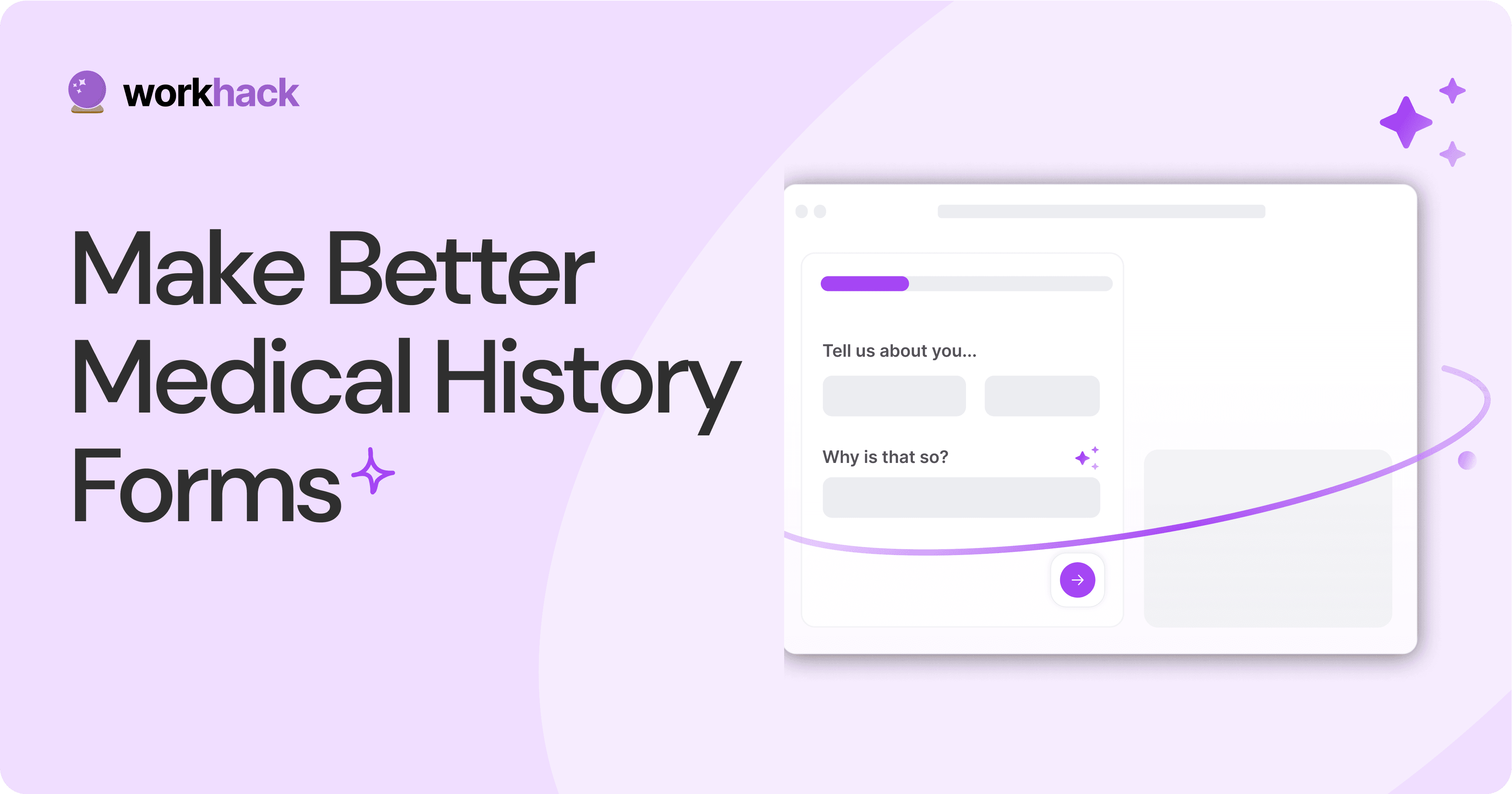
4 Tips for Better Medical History Forms.
Medical history forms are central to patient care, onboarding, and medical administration records. Learn how to make them easier to fill.
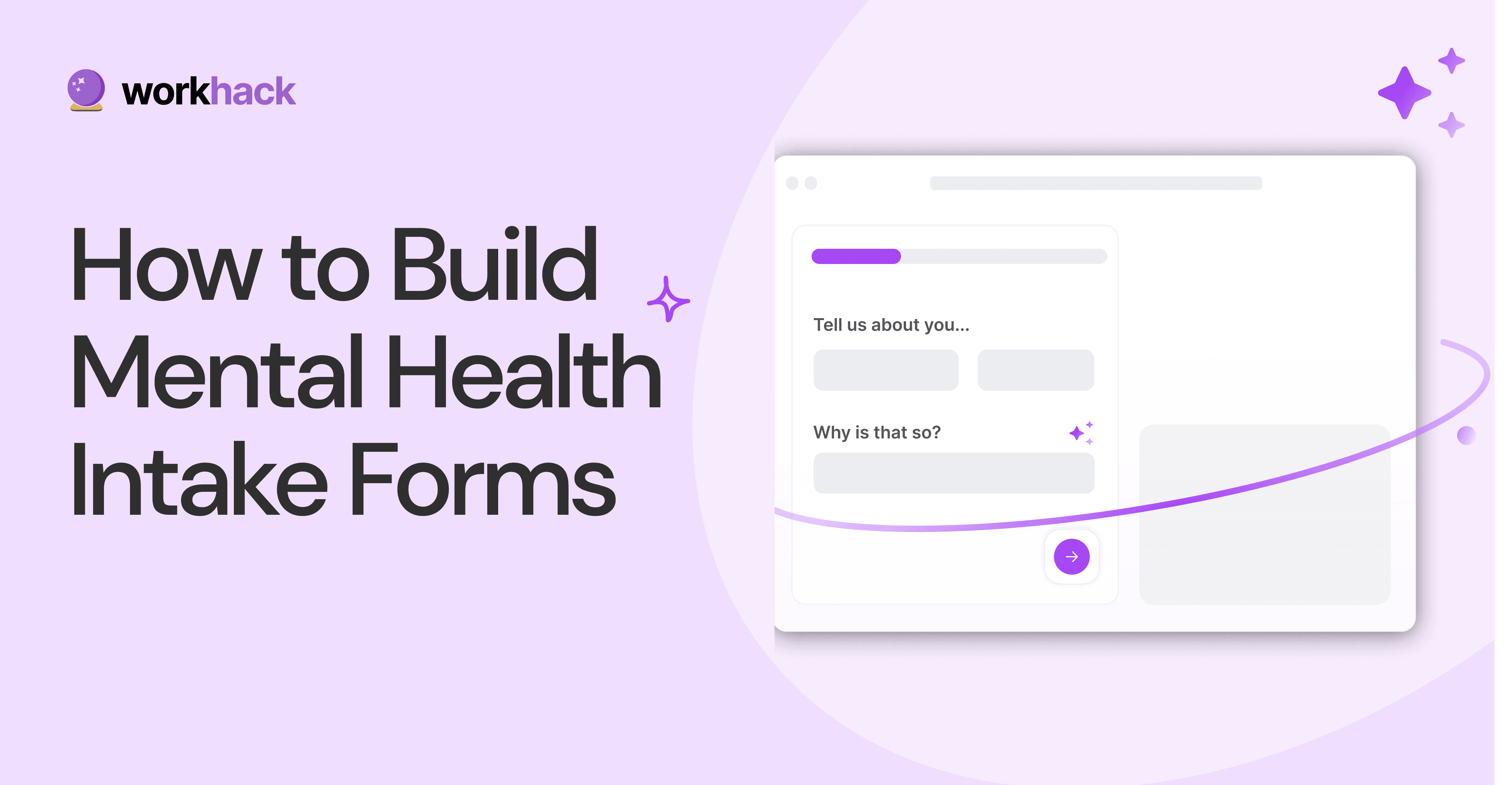
How to Build Mental Health Intake Forms?
Mental health intake forms are not like patient intake forms. Mental health intake forms deal with far more sensitive data and have specific design methods.
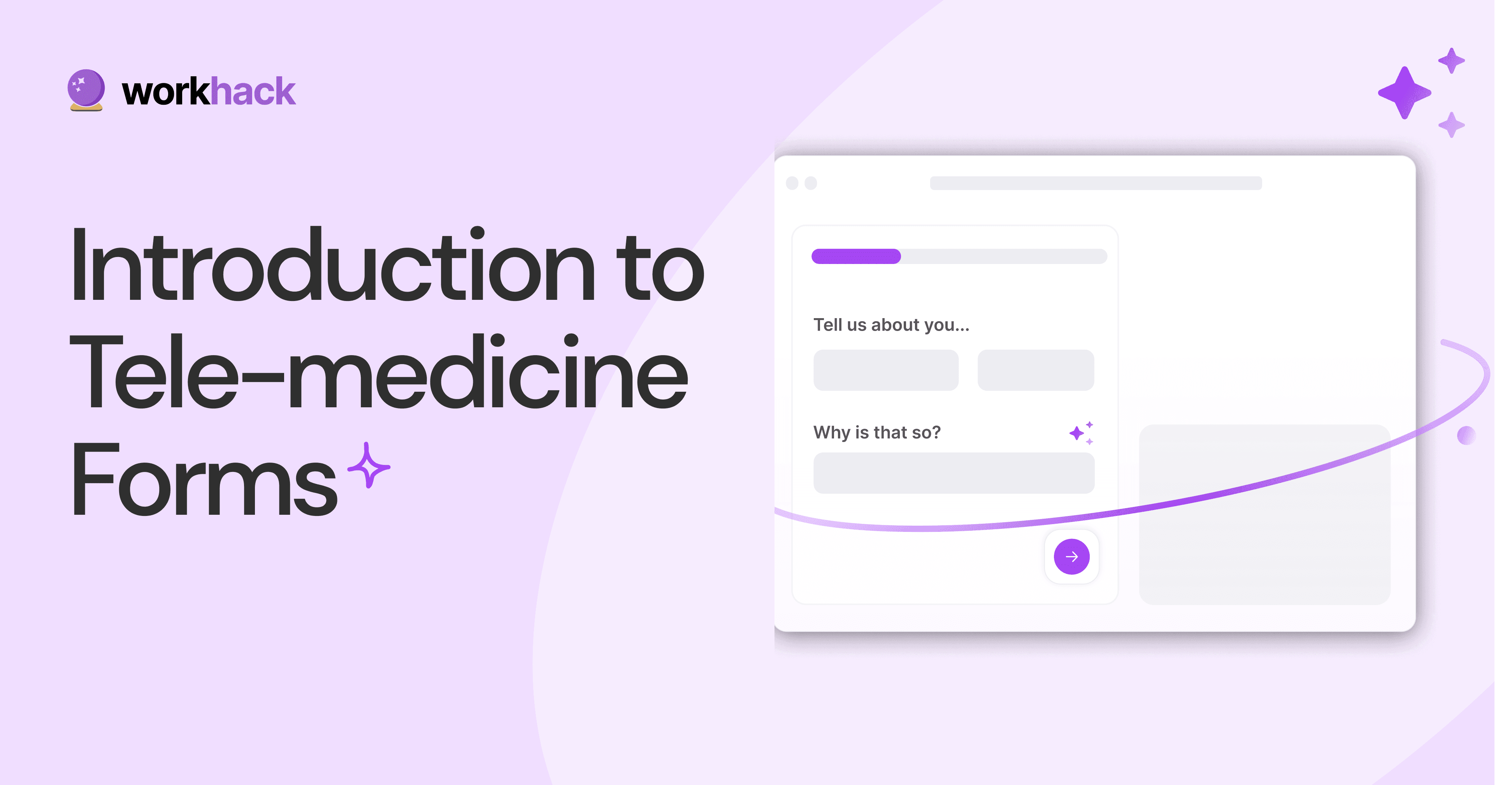
What, Why and How of Telemedicine Forms.
Telemedicine is on the rise and with different form builders out there, which one best suits your needs as a healthcare services provider?
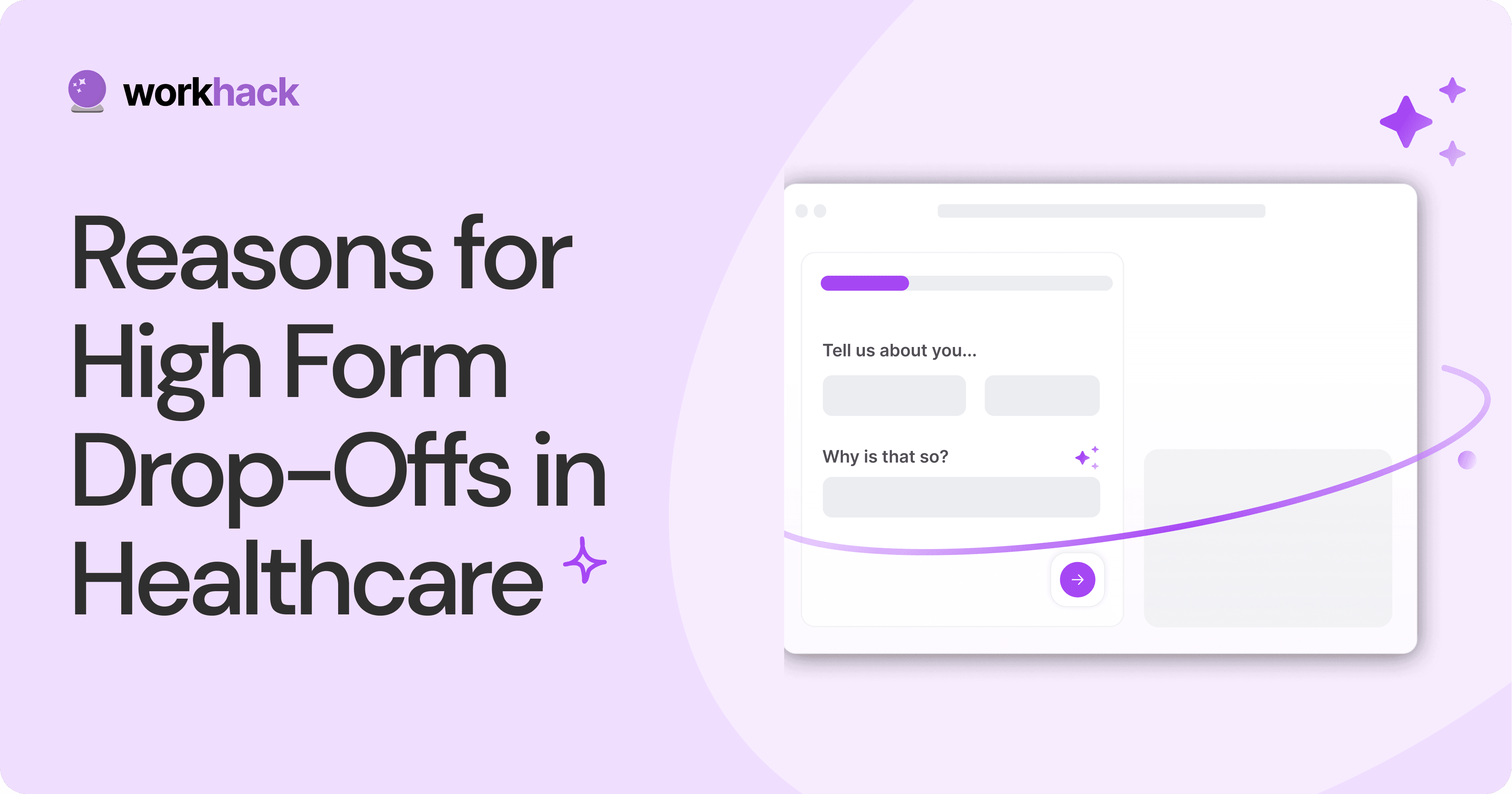
3 Reasons for Major Drop-Offs in Medical Forms.
No matter which healthcare form we pick, there are major drop-off reasons. We shall dive into the top 3 and learn how to resolve them in your next form.
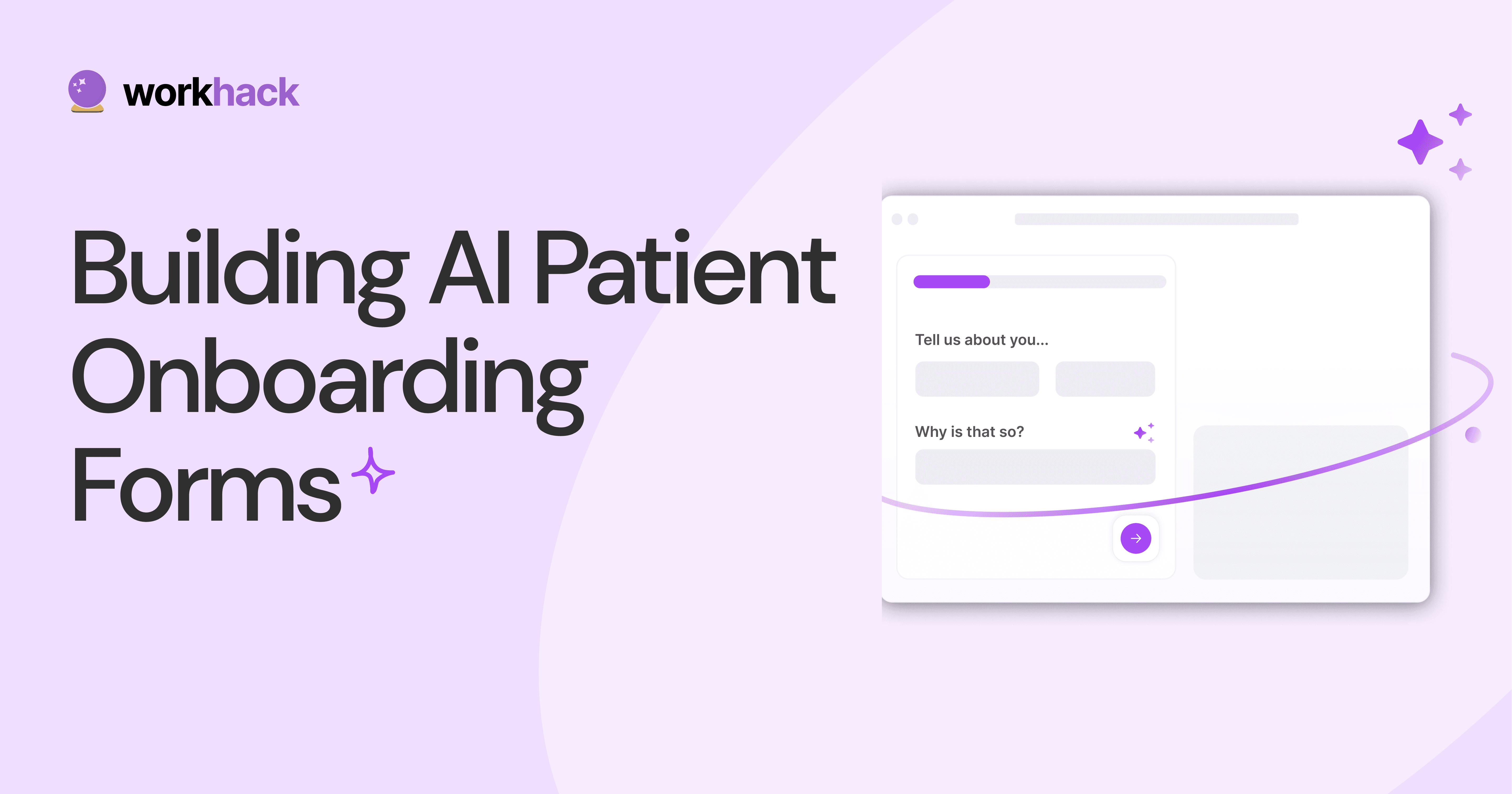
Patient Onboarding Forms - From Click to Clinic.
Patient onboarding forms are the first touchpoint for patients; getting this right for higher conversion rates is a must-have. Learn how to perfect them now.

5 Key Parts of a Good Patient Satisfaction Form.
The goal of patient satisfaction surveys is to course-correct the services of a healthcare provider. Patient feedback leads to a culture of patient-centric care.
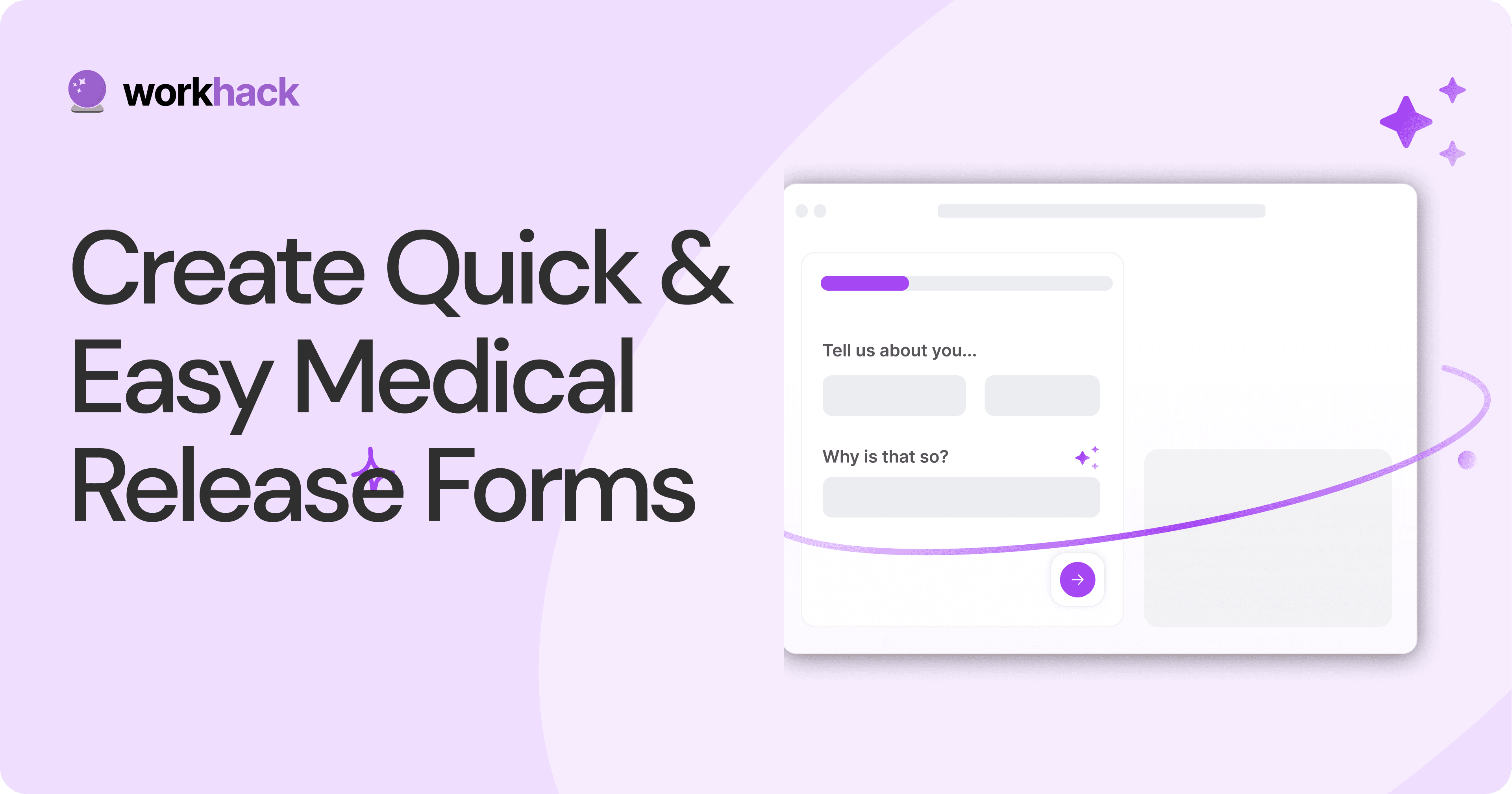
Build Quick and Easy Medical Release Forms.
Every HIPAA-compliant healthcare provider comes across medical release forms that involve details from medical history forms. Can they be shipped fast? Yes.
Subscribe to stay updated.
Subscribe to stay updated.
Subscribe to stay updated.
HC
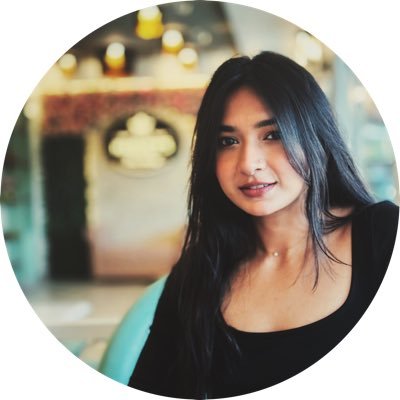
HC
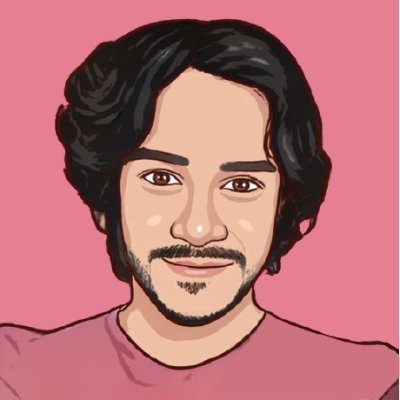
HC
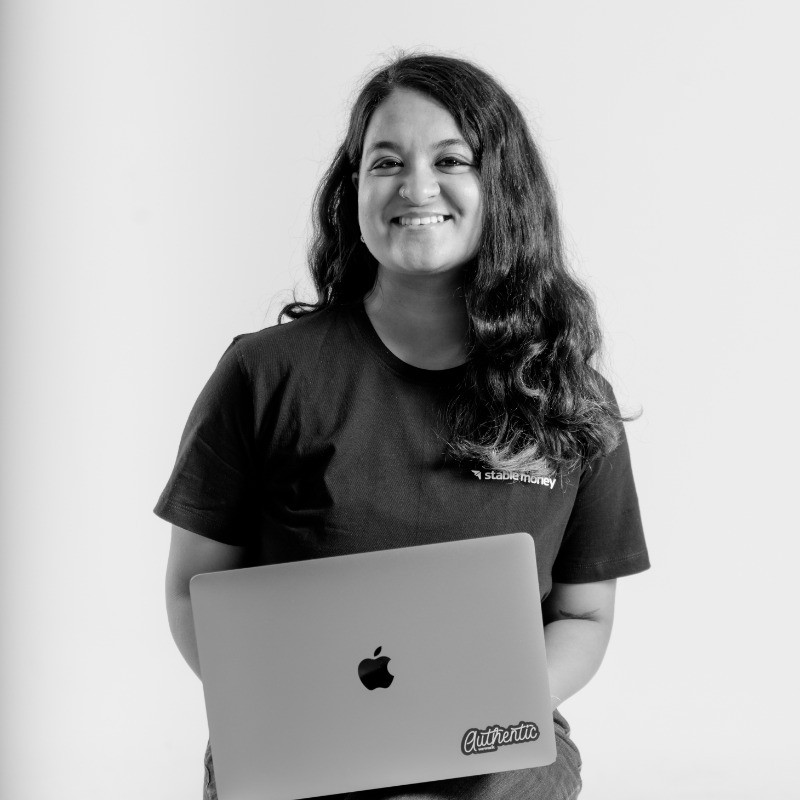
HC
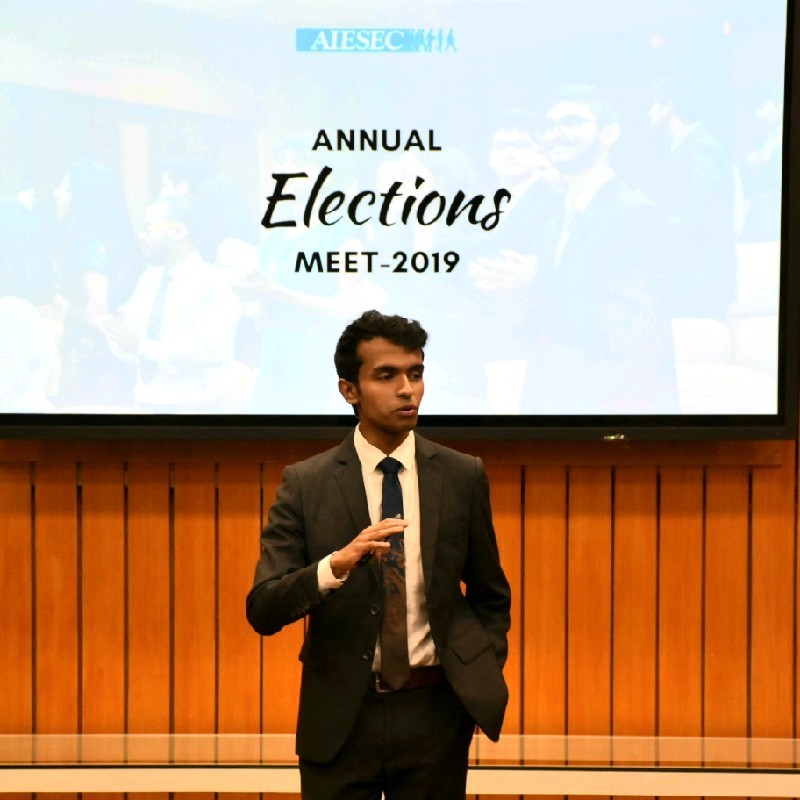
70+ people from across industries read our emails.
HC
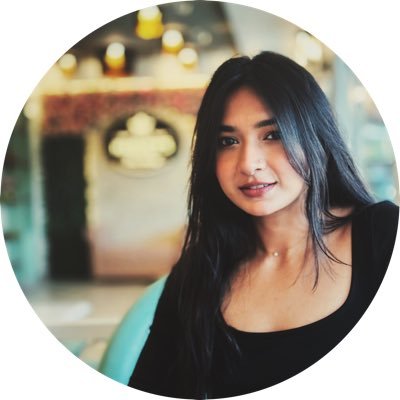
HC
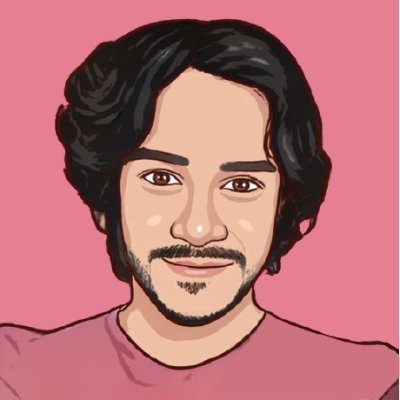
70+ people from across industries read our emails.
HC
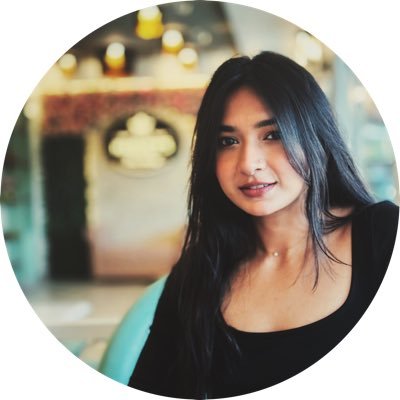
HC
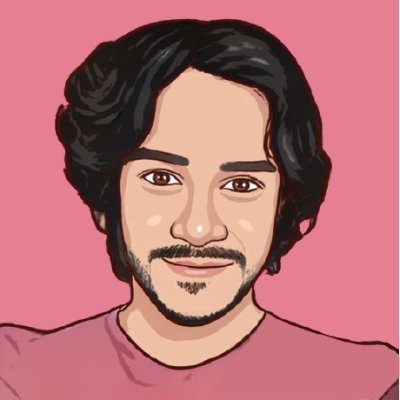
HC
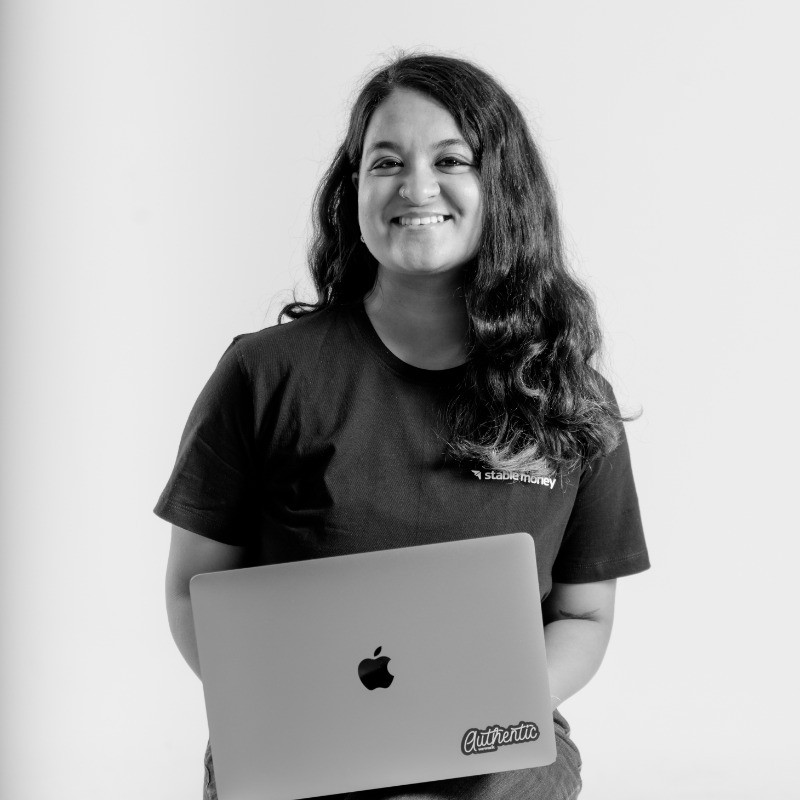
70+ people from across industries read our emails.
Bangalore, India / San Francisco, US
WorkHack Inc. 2023
Bangalore, India
San Francisco, US
WorkHack Inc. 2023
WorkHack Inc. 2023
Bangalore, India / San Francisco, US
WorkHack Inc. 2023
Bangalore, India / San Francisco, US