How To Use AI To Improve Product Research With Qualitative Data
How To Use AI To Improve Product Research With Qualitative Data
How To Use AI To Improve Product Research With Qualitative Data



Qualitative data—the stuff of dreams for product researchers and the stuff of nightmares for anyone who’s ever tried to manually sift through a mountain of open-ended survey responses. It provides the rich, nuanced insights that numbers alone can’t deliver. Fortunately, AI is here to save the day, or at least make it a lot less daunting.
Qualitative data refers to non-numerical information that captures the characteristics, attributes, and subjective experiences of individuals or phenomena. It includes descriptive details such as opinions, behaviors, feelings, and observations, often collected through interviews, surveys, focus groups, and open-ended responses. It provides depth and context to understand the underlying reasons, motivations, and patterns in human behavior, complementing quantitative data by offering rich, narrative insights.
In this blog post, we'll explore how AI elevates your qualitative data game, making product research more insightful, efficient, and just plain fun. We’ll also delve into some practical use cases and best practices. So, buckle up and get ready to transform your approach to qualitative data!
The Charm and Challenge of Qualitative Data
Before diving into the AI magic, let’s appreciate the allure of qualitative data. While quantitative data tells you what is happening, qualitative data answers the why behind it. It gives voice to the user's thoughts, emotions, and motivations, often revealing insights that numbers can't express.
However, analyzing qualitative data traditionally involves hours of coding responses, tagging themes, and finding patterns—a labor-intensive process that’s as exciting as watching paint dry. This is where AI steps in, promising to cut through the noise and deliver actionable insights faster than you can say “Natural Language Processing.”
How AI Transforms Qualitative Data Analysis
1. Automated Text Analysis: The AI Detective
Imagine having an assistant who tirelessly reads through thousands of survey responses, interview transcripts, or social media comments and summarizes them without missing a beat. That's AI-powered text analysis for you. Using Natural Language Processing (NLP), AI identifies themes, sentiment, and key phrases, transforming unstructured text into structured data.
Use Case: Customer Feedback Analysis
Suppose you’ve launched a new app feature and have collected a sea of user feedback. AI scans through this feedback to detect common complaints, frequently requested features, or general sentiment towards the update. This allows you to prioritize your development roadmap based on actual user needs and emotions, not just gut feelings.
2. Sentiment Analysis: The Mood Ring of Data
Sentiment analysis, another NLP capability, gauges the emotional tone behind user comments. You can differentiate between users who are frustrated (and likely to churn) and those who are delighted (and potential advocates).
Use Case: Social Media Monitoring
Imagine monitoring social media buzz after a product launch. Sentiment analysis helps you quickly identify whether the conversation is positive, negative, or neutral, enabling you to respond to issues in real-time or capitalize on positive buzz.
3. Topic Modeling: The AI Librarian
AI automatically categorizes vast amounts of qualitative data into topics or themes, making it easier to understand the broader context. It's particularly useful when dealing with open-ended survey responses or interview transcripts.
Use Case: User Interviews
After conducting a series of user interviews about their experience with your product, AI helps identify common topics discussed, such as “ease of use,” “feature requests,” or “technical issues.” This not only saves time but also ensures that no key themes are overlooked.
4. Trend Analysis: The Crystal Ball
AI doesn’t just see what’s happening now; it predicts trends by analyzing patterns over time. This allows you to anticipate shifts in user sentiment or emerging themes before they become widespread issues or opportunities.
Use Case: Product Lifecycle Management
By continuously analyzing qualitative feedback, AI helps you spot declining user satisfaction with a particular feature before it becomes a significant problem. This enables proactive improvements, enhancing user retention and satisfaction.
5. Automated Reporting: The AI Secretary
Gone are the days of manually crafting lengthy reports. AI generates summaries, dashboards, and insights from qualitative data, making it easy to communicate findings to stakeholders and integrate them into your decision-making process.
Use Case: Executive Briefings
When preparing a report for your leadership team, AI helps create a concise summary of key insights, complete with visualizations. This ensures that your data-driven story is both compelling and easy to digest, aiding strategic decision-making.
Best Practices for Using AI in Qualitative Research
While AI offers powerful tools for qualitative data analysis, using them effectively requires some finesse. Here are a few best practices to keep in mind:
1. Choose the Right Tool for the Job
Not all AI tools are created equal. Some excel at text analysis, while others shine in sentiment or trend analysis. Identify your research needs and select tools that align with your goals. For example, tools like MonkeyLearn and Lexalytics are great for text analysis, whereas Brandwatch and Talkwalker excel in sentiment analysis.
2. Train Your AI with High-Quality Data
AI learns from the data it’s fed. Ensure that your training data is representative of the issues you're exploring. This might mean curating a diverse set of responses or including a range of emotional tones in sentiment analysis models.
3. Combine AI Insights with Human Judgment
AI will crunch numbers and identify patterns, but human intuition and domain knowledge are crucial for interpreting these insights. Use AI to do the heavy lifting but validate findings through expert review and context.
4. Monitor and Update Your AI Models
AI models degrade over time as new language patterns emerge or as your product evolves. Regularly update your models to ensure they remain accurate and relevant. This is especially important in fast-changing industries where trends and user language shift rapidly.
5. Focus on Actionable Insights
It’s easy to get overwhelmed by the volume of data AI processes. Focus on insights that directly inform product decisions or strategy. Prioritize findings that are actionable and have a clear impact on user experience or business goals.
Conclusion
AI-driven market research automation is revolutionizing the way marketers gather, analyze, and utilize data. By integrating advanced AI technologies and AI-native tools like Metaforms, businesses streamline research processes, extract deeper insights, and respond to market changes with greater agility. Unlike traditional methods, AI automation offers scalability, efficiency, and real-time analysis, making it essential for modern marketing strategies.
Key steps include data collection, analysis, and reporting, supported by tools like natural language processing (NLP) and machine learning algorithms. Best practices involve maintaining data quality, ensuring ethical AI use, and leveraging AI tools such as predictive analytics and sentiment analysis to inform decision-making.
By embracing AI-driven market research, businesses enhance their understanding of consumer behavior, optimize marketing efforts, and stay competitive in a rapidly evolving market landscape.
Qualitative data—the stuff of dreams for product researchers and the stuff of nightmares for anyone who’s ever tried to manually sift through a mountain of open-ended survey responses. It provides the rich, nuanced insights that numbers alone can’t deliver. Fortunately, AI is here to save the day, or at least make it a lot less daunting.
Qualitative data refers to non-numerical information that captures the characteristics, attributes, and subjective experiences of individuals or phenomena. It includes descriptive details such as opinions, behaviors, feelings, and observations, often collected through interviews, surveys, focus groups, and open-ended responses. It provides depth and context to understand the underlying reasons, motivations, and patterns in human behavior, complementing quantitative data by offering rich, narrative insights.
In this blog post, we'll explore how AI elevates your qualitative data game, making product research more insightful, efficient, and just plain fun. We’ll also delve into some practical use cases and best practices. So, buckle up and get ready to transform your approach to qualitative data!
The Charm and Challenge of Qualitative Data
Before diving into the AI magic, let’s appreciate the allure of qualitative data. While quantitative data tells you what is happening, qualitative data answers the why behind it. It gives voice to the user's thoughts, emotions, and motivations, often revealing insights that numbers can't express.
However, analyzing qualitative data traditionally involves hours of coding responses, tagging themes, and finding patterns—a labor-intensive process that’s as exciting as watching paint dry. This is where AI steps in, promising to cut through the noise and deliver actionable insights faster than you can say “Natural Language Processing.”
How AI Transforms Qualitative Data Analysis
1. Automated Text Analysis: The AI Detective
Imagine having an assistant who tirelessly reads through thousands of survey responses, interview transcripts, or social media comments and summarizes them without missing a beat. That's AI-powered text analysis for you. Using Natural Language Processing (NLP), AI identifies themes, sentiment, and key phrases, transforming unstructured text into structured data.
Use Case: Customer Feedback Analysis
Suppose you’ve launched a new app feature and have collected a sea of user feedback. AI scans through this feedback to detect common complaints, frequently requested features, or general sentiment towards the update. This allows you to prioritize your development roadmap based on actual user needs and emotions, not just gut feelings.
2. Sentiment Analysis: The Mood Ring of Data
Sentiment analysis, another NLP capability, gauges the emotional tone behind user comments. You can differentiate between users who are frustrated (and likely to churn) and those who are delighted (and potential advocates).
Use Case: Social Media Monitoring
Imagine monitoring social media buzz after a product launch. Sentiment analysis helps you quickly identify whether the conversation is positive, negative, or neutral, enabling you to respond to issues in real-time or capitalize on positive buzz.
3. Topic Modeling: The AI Librarian
AI automatically categorizes vast amounts of qualitative data into topics or themes, making it easier to understand the broader context. It's particularly useful when dealing with open-ended survey responses or interview transcripts.
Use Case: User Interviews
After conducting a series of user interviews about their experience with your product, AI helps identify common topics discussed, such as “ease of use,” “feature requests,” or “technical issues.” This not only saves time but also ensures that no key themes are overlooked.
4. Trend Analysis: The Crystal Ball
AI doesn’t just see what’s happening now; it predicts trends by analyzing patterns over time. This allows you to anticipate shifts in user sentiment or emerging themes before they become widespread issues or opportunities.
Use Case: Product Lifecycle Management
By continuously analyzing qualitative feedback, AI helps you spot declining user satisfaction with a particular feature before it becomes a significant problem. This enables proactive improvements, enhancing user retention and satisfaction.
5. Automated Reporting: The AI Secretary
Gone are the days of manually crafting lengthy reports. AI generates summaries, dashboards, and insights from qualitative data, making it easy to communicate findings to stakeholders and integrate them into your decision-making process.
Use Case: Executive Briefings
When preparing a report for your leadership team, AI helps create a concise summary of key insights, complete with visualizations. This ensures that your data-driven story is both compelling and easy to digest, aiding strategic decision-making.
Best Practices for Using AI in Qualitative Research
While AI offers powerful tools for qualitative data analysis, using them effectively requires some finesse. Here are a few best practices to keep in mind:
1. Choose the Right Tool for the Job
Not all AI tools are created equal. Some excel at text analysis, while others shine in sentiment or trend analysis. Identify your research needs and select tools that align with your goals. For example, tools like MonkeyLearn and Lexalytics are great for text analysis, whereas Brandwatch and Talkwalker excel in sentiment analysis.
2. Train Your AI with High-Quality Data
AI learns from the data it’s fed. Ensure that your training data is representative of the issues you're exploring. This might mean curating a diverse set of responses or including a range of emotional tones in sentiment analysis models.
3. Combine AI Insights with Human Judgment
AI will crunch numbers and identify patterns, but human intuition and domain knowledge are crucial for interpreting these insights. Use AI to do the heavy lifting but validate findings through expert review and context.
4. Monitor and Update Your AI Models
AI models degrade over time as new language patterns emerge or as your product evolves. Regularly update your models to ensure they remain accurate and relevant. This is especially important in fast-changing industries where trends and user language shift rapidly.
5. Focus on Actionable Insights
It’s easy to get overwhelmed by the volume of data AI processes. Focus on insights that directly inform product decisions or strategy. Prioritize findings that are actionable and have a clear impact on user experience or business goals.
Conclusion
AI-driven market research automation is revolutionizing the way marketers gather, analyze, and utilize data. By integrating advanced AI technologies and AI-native tools like Metaforms, businesses streamline research processes, extract deeper insights, and respond to market changes with greater agility. Unlike traditional methods, AI automation offers scalability, efficiency, and real-time analysis, making it essential for modern marketing strategies.
Key steps include data collection, analysis, and reporting, supported by tools like natural language processing (NLP) and machine learning algorithms. Best practices involve maintaining data quality, ensuring ethical AI use, and leveraging AI tools such as predictive analytics and sentiment analysis to inform decision-making.
By embracing AI-driven market research, businesses enhance their understanding of consumer behavior, optimize marketing efforts, and stay competitive in a rapidly evolving market landscape.
Qualitative data—the stuff of dreams for product researchers and the stuff of nightmares for anyone who’s ever tried to manually sift through a mountain of open-ended survey responses. It provides the rich, nuanced insights that numbers alone can’t deliver. Fortunately, AI is here to save the day, or at least make it a lot less daunting.
Qualitative data refers to non-numerical information that captures the characteristics, attributes, and subjective experiences of individuals or phenomena. It includes descriptive details such as opinions, behaviors, feelings, and observations, often collected through interviews, surveys, focus groups, and open-ended responses. It provides depth and context to understand the underlying reasons, motivations, and patterns in human behavior, complementing quantitative data by offering rich, narrative insights.
In this blog post, we'll explore how AI elevates your qualitative data game, making product research more insightful, efficient, and just plain fun. We’ll also delve into some practical use cases and best practices. So, buckle up and get ready to transform your approach to qualitative data!
The Charm and Challenge of Qualitative Data
Before diving into the AI magic, let’s appreciate the allure of qualitative data. While quantitative data tells you what is happening, qualitative data answers the why behind it. It gives voice to the user's thoughts, emotions, and motivations, often revealing insights that numbers can't express.
However, analyzing qualitative data traditionally involves hours of coding responses, tagging themes, and finding patterns—a labor-intensive process that’s as exciting as watching paint dry. This is where AI steps in, promising to cut through the noise and deliver actionable insights faster than you can say “Natural Language Processing.”
How AI Transforms Qualitative Data Analysis
1. Automated Text Analysis: The AI Detective
Imagine having an assistant who tirelessly reads through thousands of survey responses, interview transcripts, or social media comments and summarizes them without missing a beat. That's AI-powered text analysis for you. Using Natural Language Processing (NLP), AI identifies themes, sentiment, and key phrases, transforming unstructured text into structured data.
Use Case: Customer Feedback Analysis
Suppose you’ve launched a new app feature and have collected a sea of user feedback. AI scans through this feedback to detect common complaints, frequently requested features, or general sentiment towards the update. This allows you to prioritize your development roadmap based on actual user needs and emotions, not just gut feelings.
2. Sentiment Analysis: The Mood Ring of Data
Sentiment analysis, another NLP capability, gauges the emotional tone behind user comments. You can differentiate between users who are frustrated (and likely to churn) and those who are delighted (and potential advocates).
Use Case: Social Media Monitoring
Imagine monitoring social media buzz after a product launch. Sentiment analysis helps you quickly identify whether the conversation is positive, negative, or neutral, enabling you to respond to issues in real-time or capitalize on positive buzz.
3. Topic Modeling: The AI Librarian
AI automatically categorizes vast amounts of qualitative data into topics or themes, making it easier to understand the broader context. It's particularly useful when dealing with open-ended survey responses or interview transcripts.
Use Case: User Interviews
After conducting a series of user interviews about their experience with your product, AI helps identify common topics discussed, such as “ease of use,” “feature requests,” or “technical issues.” This not only saves time but also ensures that no key themes are overlooked.
4. Trend Analysis: The Crystal Ball
AI doesn’t just see what’s happening now; it predicts trends by analyzing patterns over time. This allows you to anticipate shifts in user sentiment or emerging themes before they become widespread issues or opportunities.
Use Case: Product Lifecycle Management
By continuously analyzing qualitative feedback, AI helps you spot declining user satisfaction with a particular feature before it becomes a significant problem. This enables proactive improvements, enhancing user retention and satisfaction.
5. Automated Reporting: The AI Secretary
Gone are the days of manually crafting lengthy reports. AI generates summaries, dashboards, and insights from qualitative data, making it easy to communicate findings to stakeholders and integrate them into your decision-making process.
Use Case: Executive Briefings
When preparing a report for your leadership team, AI helps create a concise summary of key insights, complete with visualizations. This ensures that your data-driven story is both compelling and easy to digest, aiding strategic decision-making.
Best Practices for Using AI in Qualitative Research
While AI offers powerful tools for qualitative data analysis, using them effectively requires some finesse. Here are a few best practices to keep in mind:
1. Choose the Right Tool for the Job
Not all AI tools are created equal. Some excel at text analysis, while others shine in sentiment or trend analysis. Identify your research needs and select tools that align with your goals. For example, tools like MonkeyLearn and Lexalytics are great for text analysis, whereas Brandwatch and Talkwalker excel in sentiment analysis.
2. Train Your AI with High-Quality Data
AI learns from the data it’s fed. Ensure that your training data is representative of the issues you're exploring. This might mean curating a diverse set of responses or including a range of emotional tones in sentiment analysis models.
3. Combine AI Insights with Human Judgment
AI will crunch numbers and identify patterns, but human intuition and domain knowledge are crucial for interpreting these insights. Use AI to do the heavy lifting but validate findings through expert review and context.
4. Monitor and Update Your AI Models
AI models degrade over time as new language patterns emerge or as your product evolves. Regularly update your models to ensure they remain accurate and relevant. This is especially important in fast-changing industries where trends and user language shift rapidly.
5. Focus on Actionable Insights
It’s easy to get overwhelmed by the volume of data AI processes. Focus on insights that directly inform product decisions or strategy. Prioritize findings that are actionable and have a clear impact on user experience or business goals.
Conclusion
AI-driven market research automation is revolutionizing the way marketers gather, analyze, and utilize data. By integrating advanced AI technologies and AI-native tools like Metaforms, businesses streamline research processes, extract deeper insights, and respond to market changes with greater agility. Unlike traditional methods, AI automation offers scalability, efficiency, and real-time analysis, making it essential for modern marketing strategies.
Key steps include data collection, analysis, and reporting, supported by tools like natural language processing (NLP) and machine learning algorithms. Best practices involve maintaining data quality, ensuring ethical AI use, and leveraging AI tools such as predictive analytics and sentiment analysis to inform decision-making.
By embracing AI-driven market research, businesses enhance their understanding of consumer behavior, optimize marketing efforts, and stay competitive in a rapidly evolving market landscape.
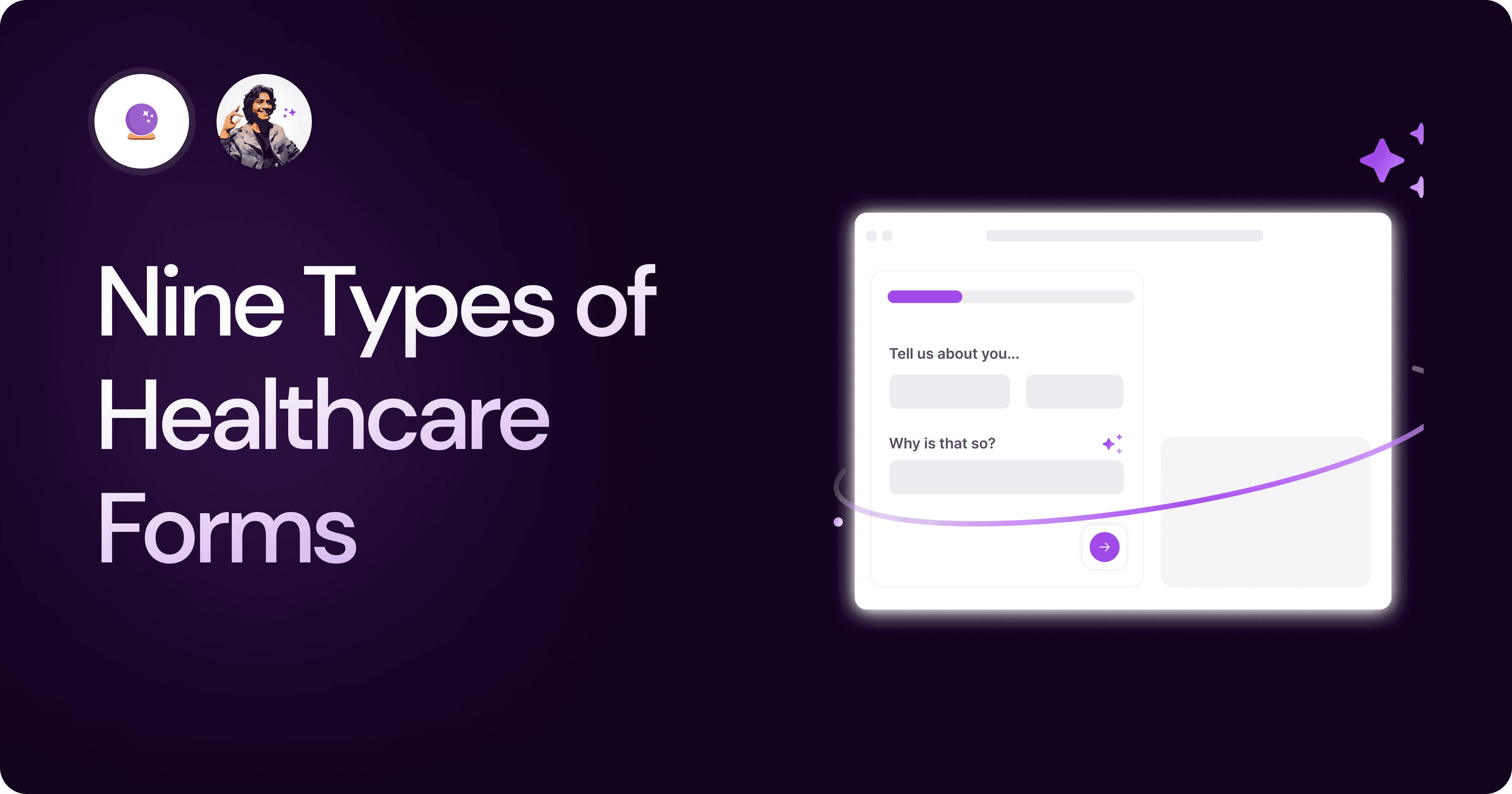
Nine Types of Healthcare and Medical Forms.
Medical forms are a must-have for any healthcare business or practitioner. Learn about the different kinds of medical and healthcare forms.
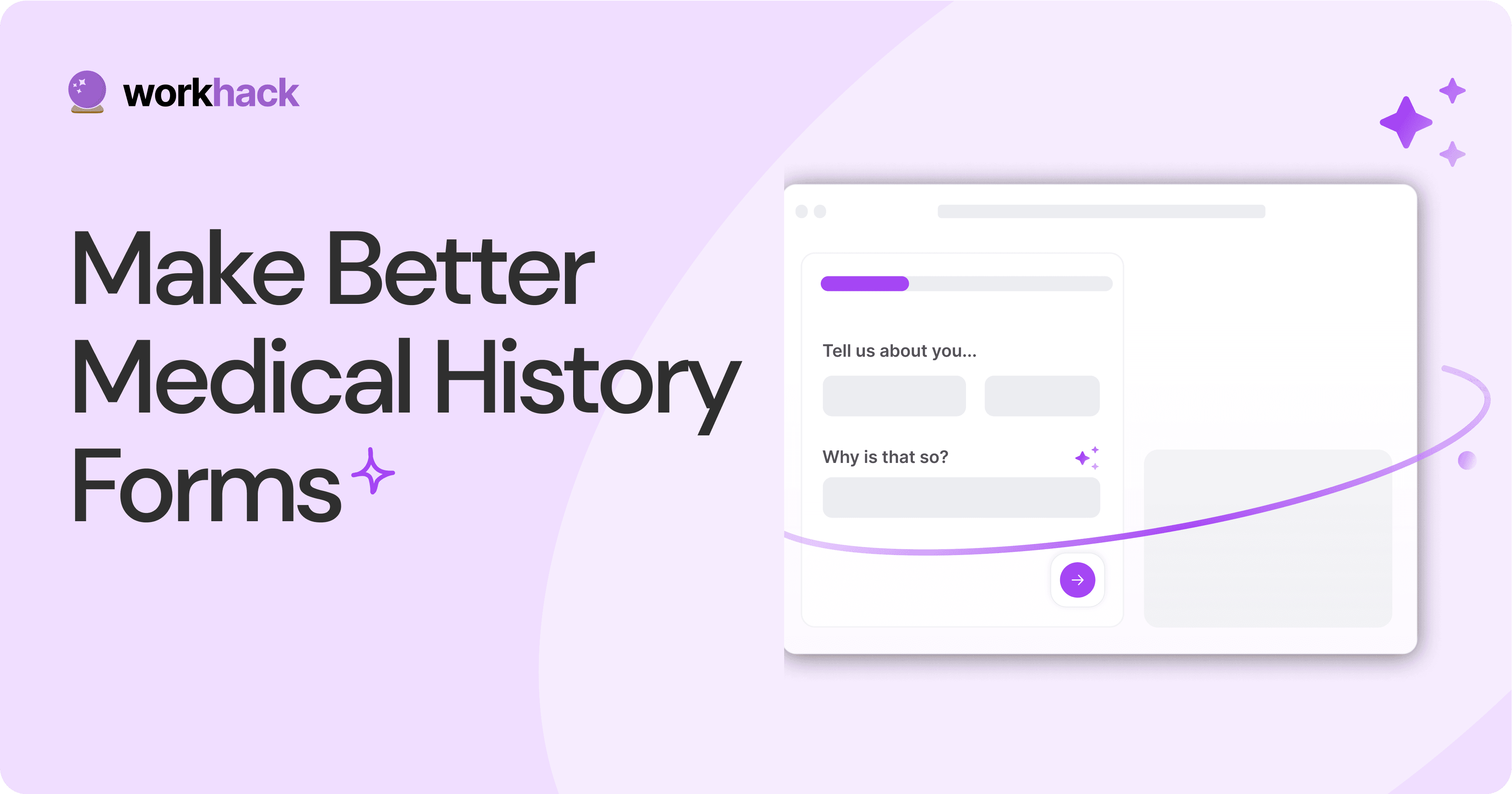
4 Tips for Better Medical History Forms.
Medical history forms are central to patient care, onboarding, and medical administration records. Learn how to make them easier to fill.
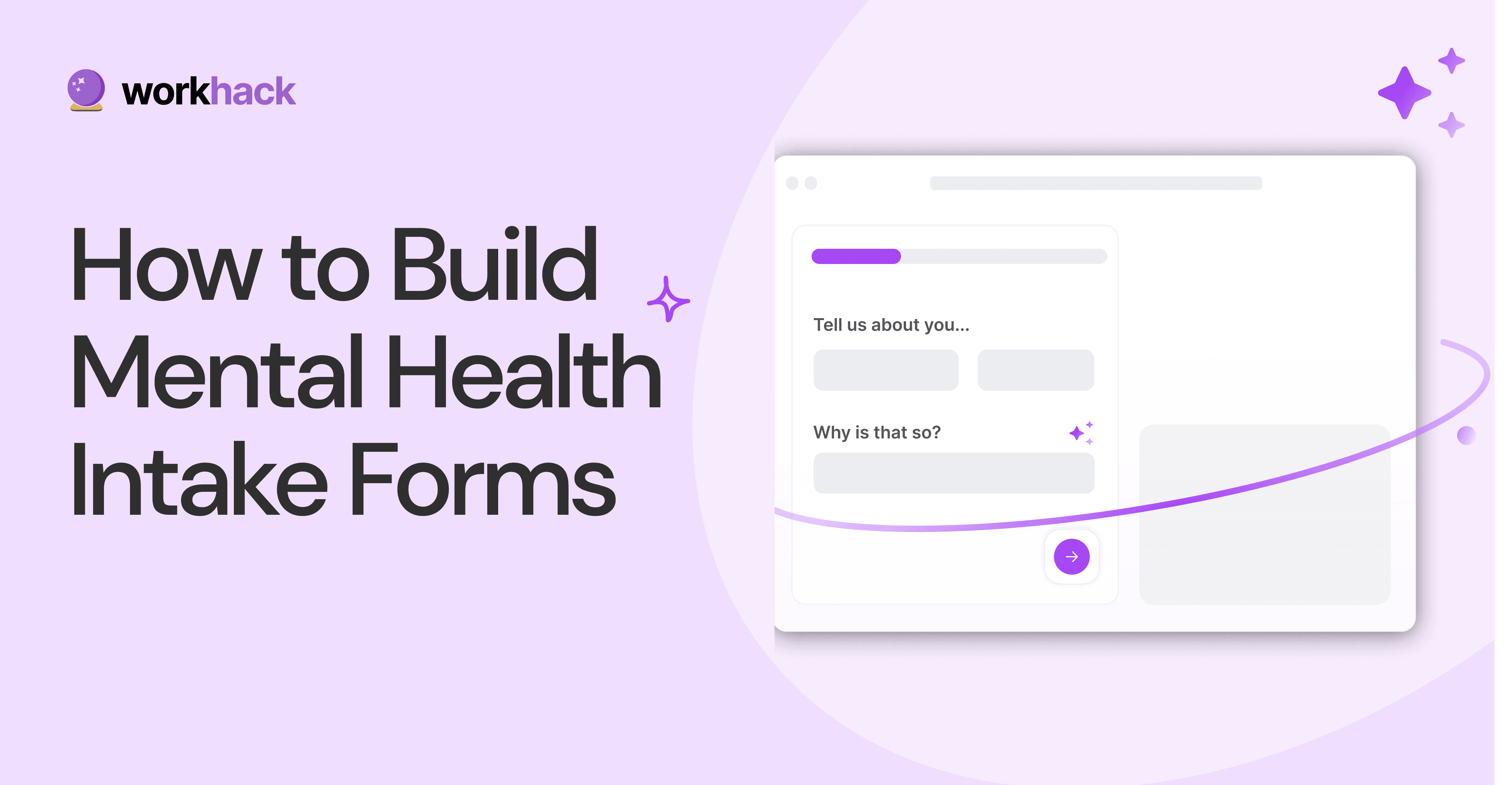
How to Build Mental Health Intake Forms?
Mental health intake forms are not like patient intake forms. Mental health intake forms deal with far more sensitive data and have specific design methods.
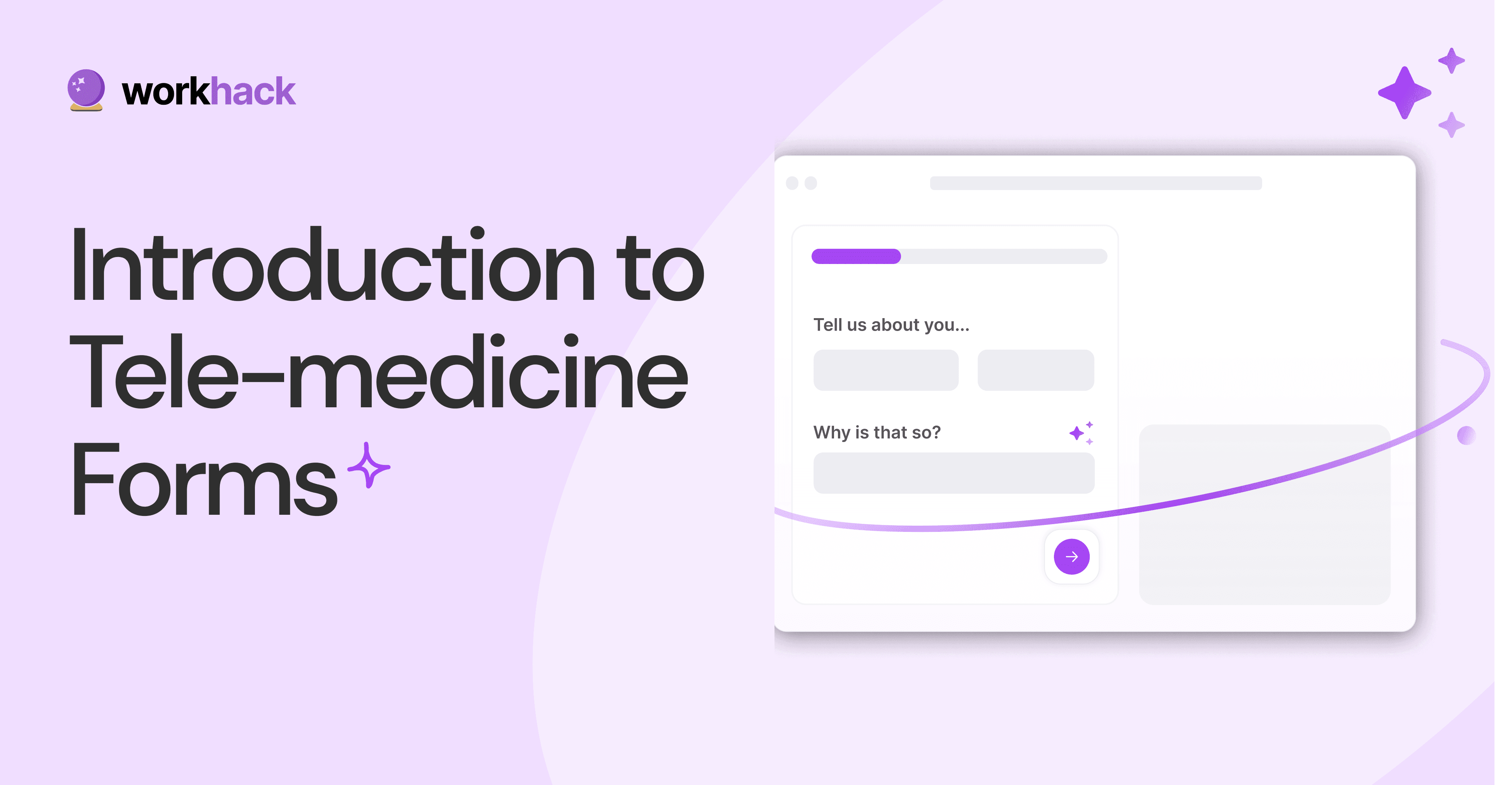
What, Why and How of Telemedicine Forms.
Telemedicine is on the rise and with different form builders out there, which one best suits your needs as a healthcare services provider?
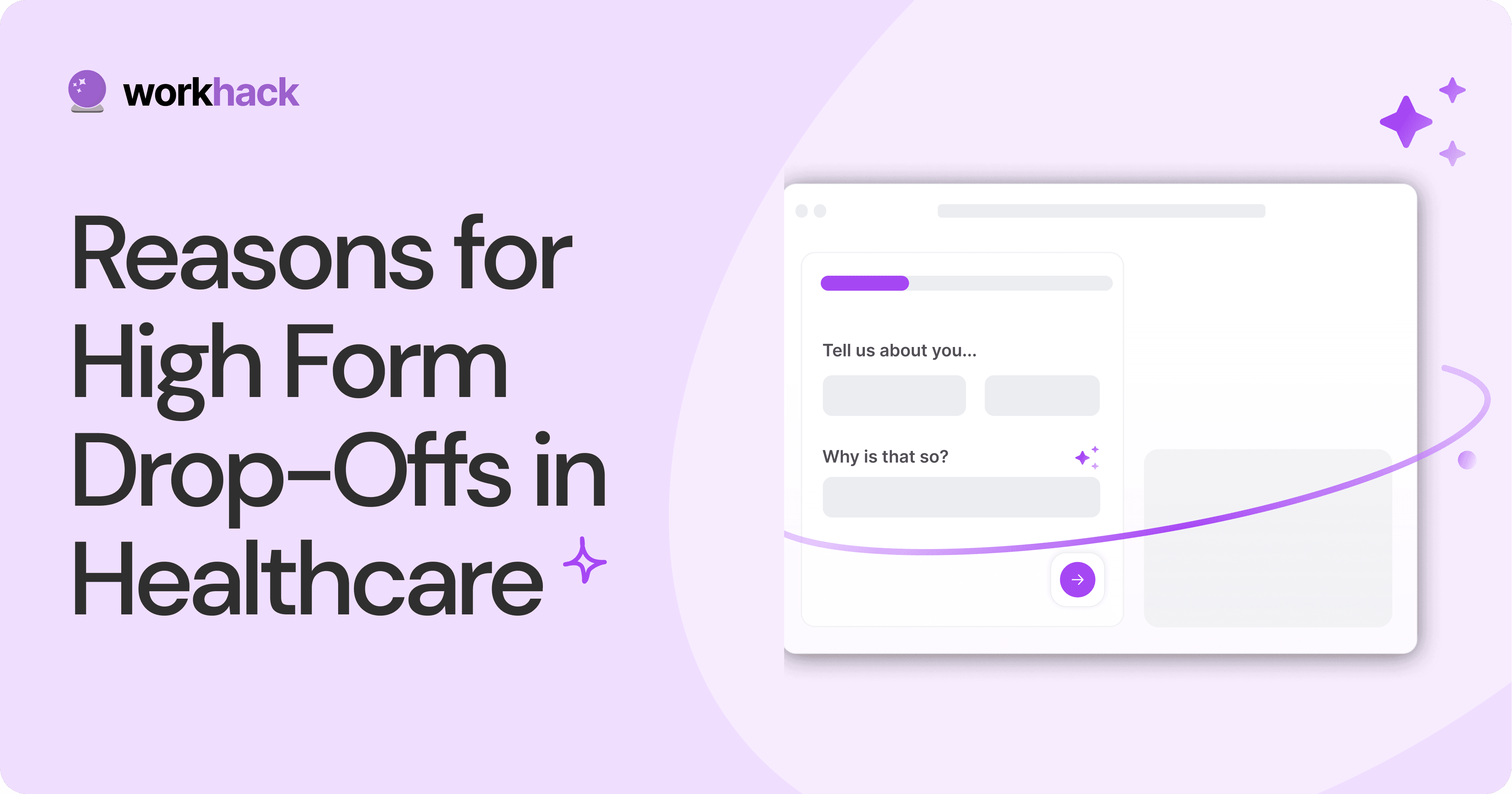
3 Reasons for Major Drop-Offs in Medical Forms.
No matter which healthcare form we pick, there are major drop-off reasons. We shall dive into the top 3 and learn how to resolve them in your next form.
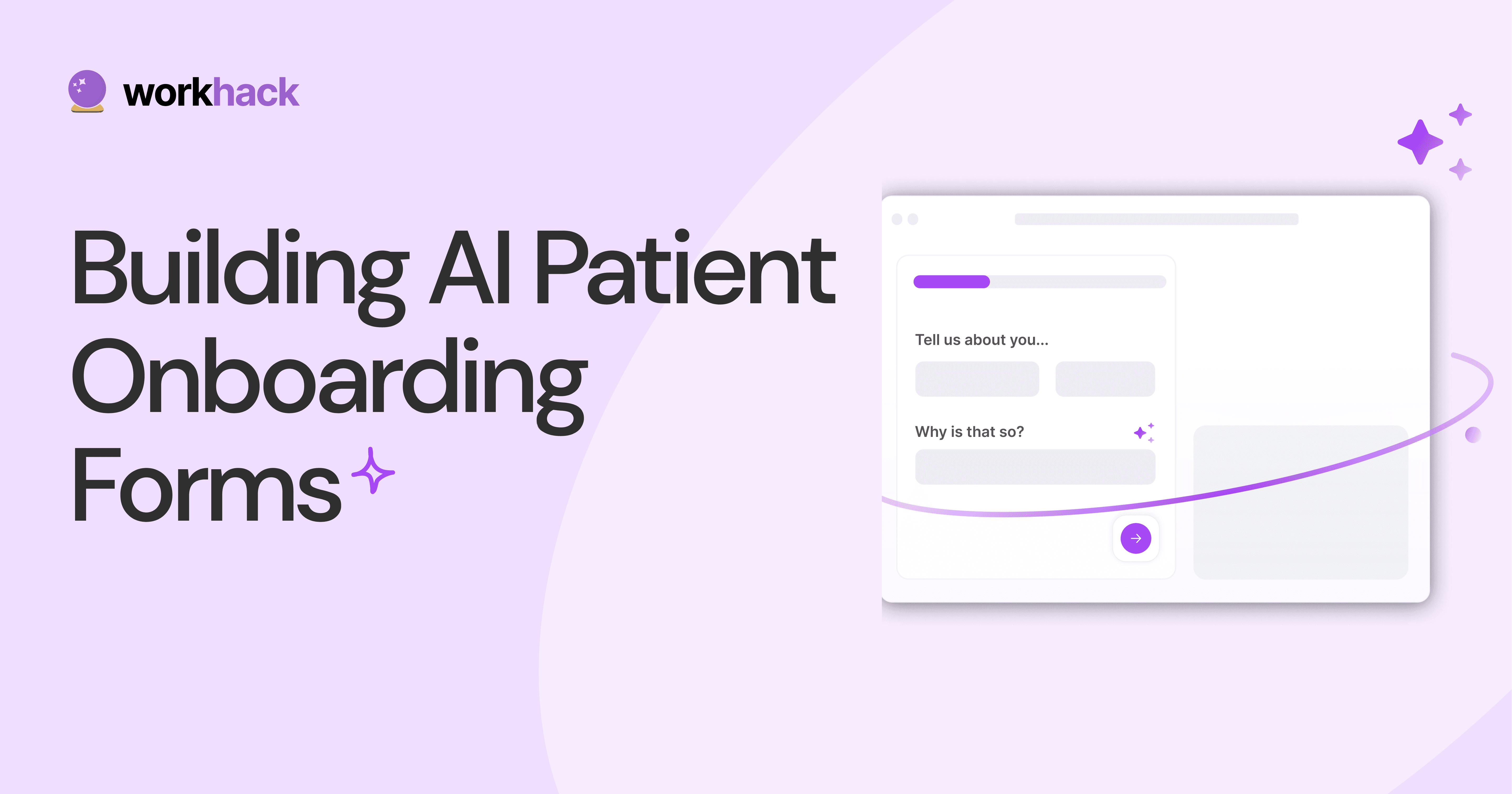
Patient Onboarding Forms - From Click to Clinic.
Patient onboarding forms are the first touchpoint for patients; getting this right for higher conversion rates is a must-have. Learn how to perfect them now.

5 Key Parts of a Good Patient Satisfaction Form.
The goal of patient satisfaction surveys is to course-correct the services of a healthcare provider. Patient feedback leads to a culture of patient-centric care.
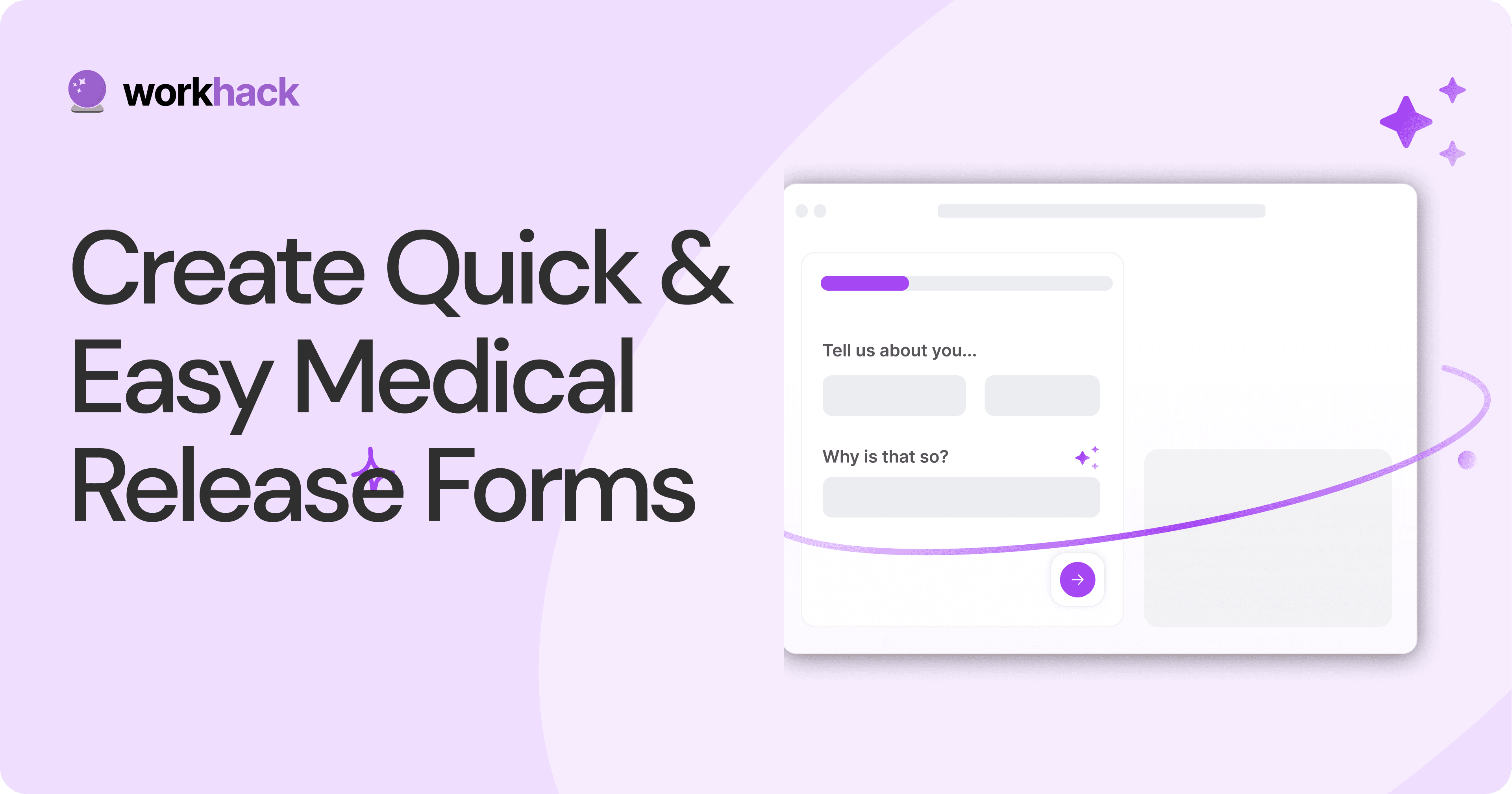
Build Quick and Easy Medical Release Forms.
Every HIPAA-compliant healthcare provider comes across medical release forms that involve details from medical history forms. Can they be shipped fast? Yes.
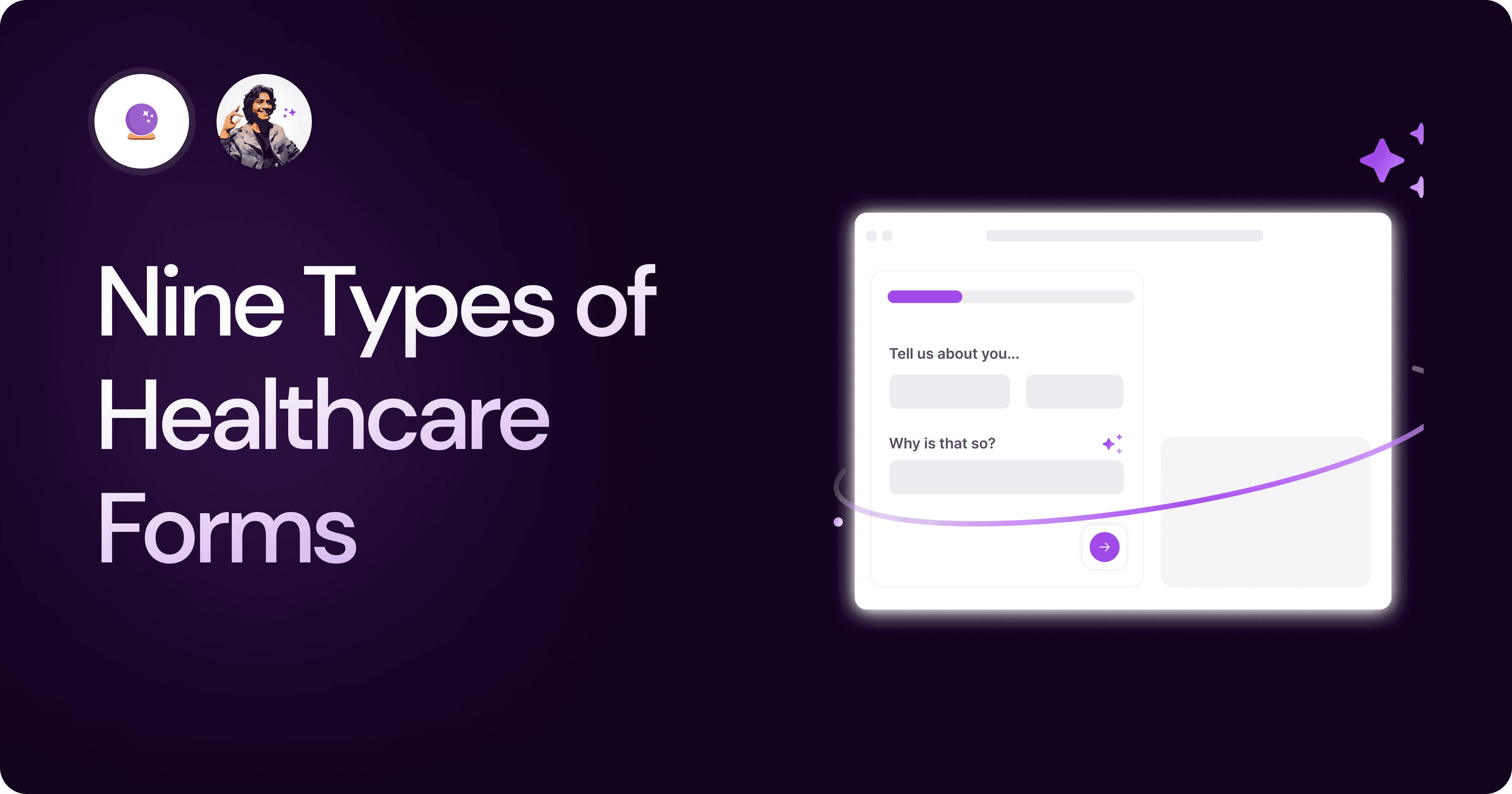
Nine Types of Healthcare and Medical Forms.
Medical forms are a must-have for any healthcare business or practitioner. Learn about the different kinds of medical and healthcare forms.
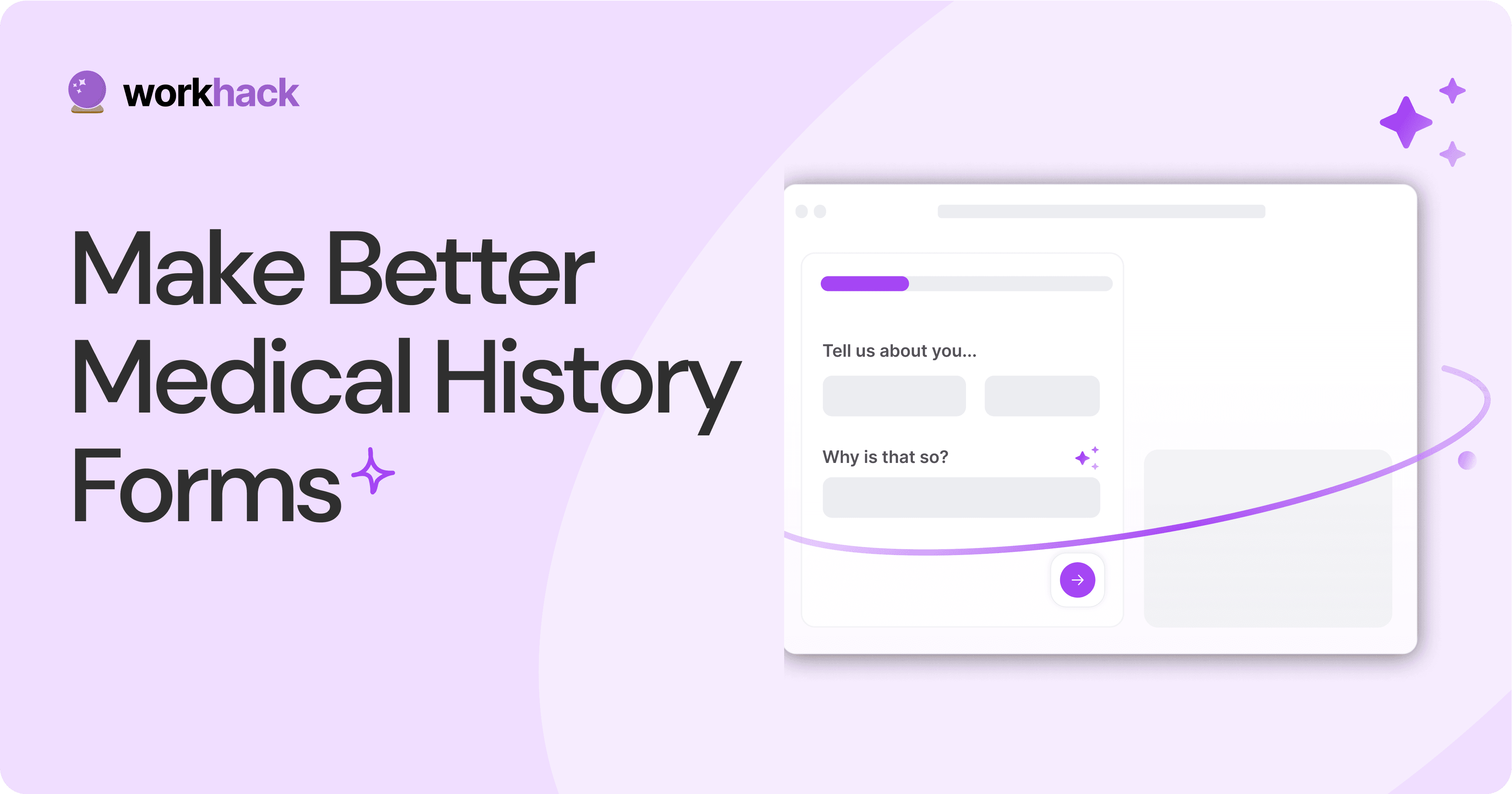
4 Tips for Better Medical History Forms.
Medical history forms are central to patient care, onboarding, and medical administration records. Learn how to make them easier to fill.
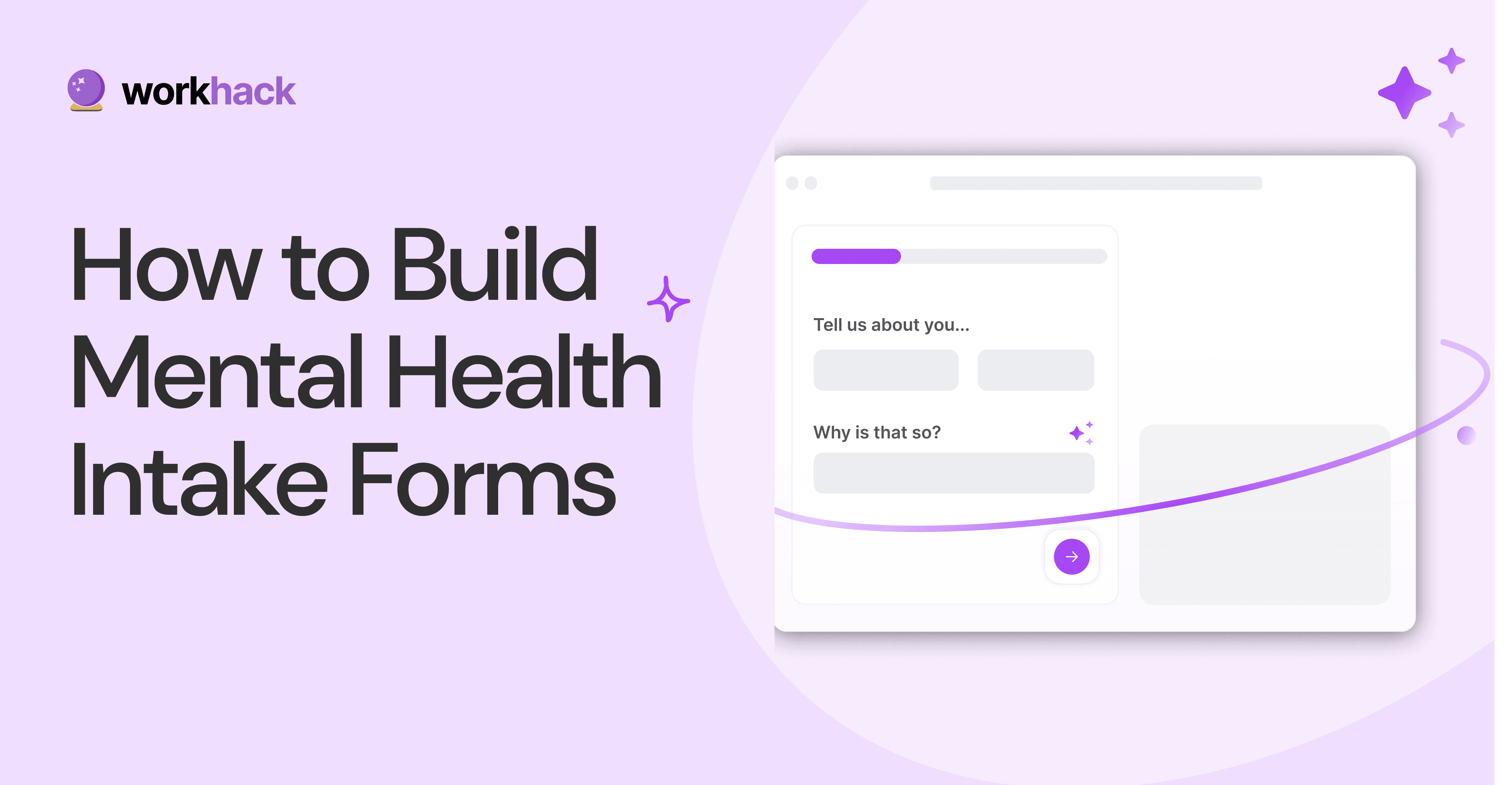
How to Build Mental Health Intake Forms?
Mental health intake forms are not like patient intake forms. Mental health intake forms deal with far more sensitive data and have specific design methods.
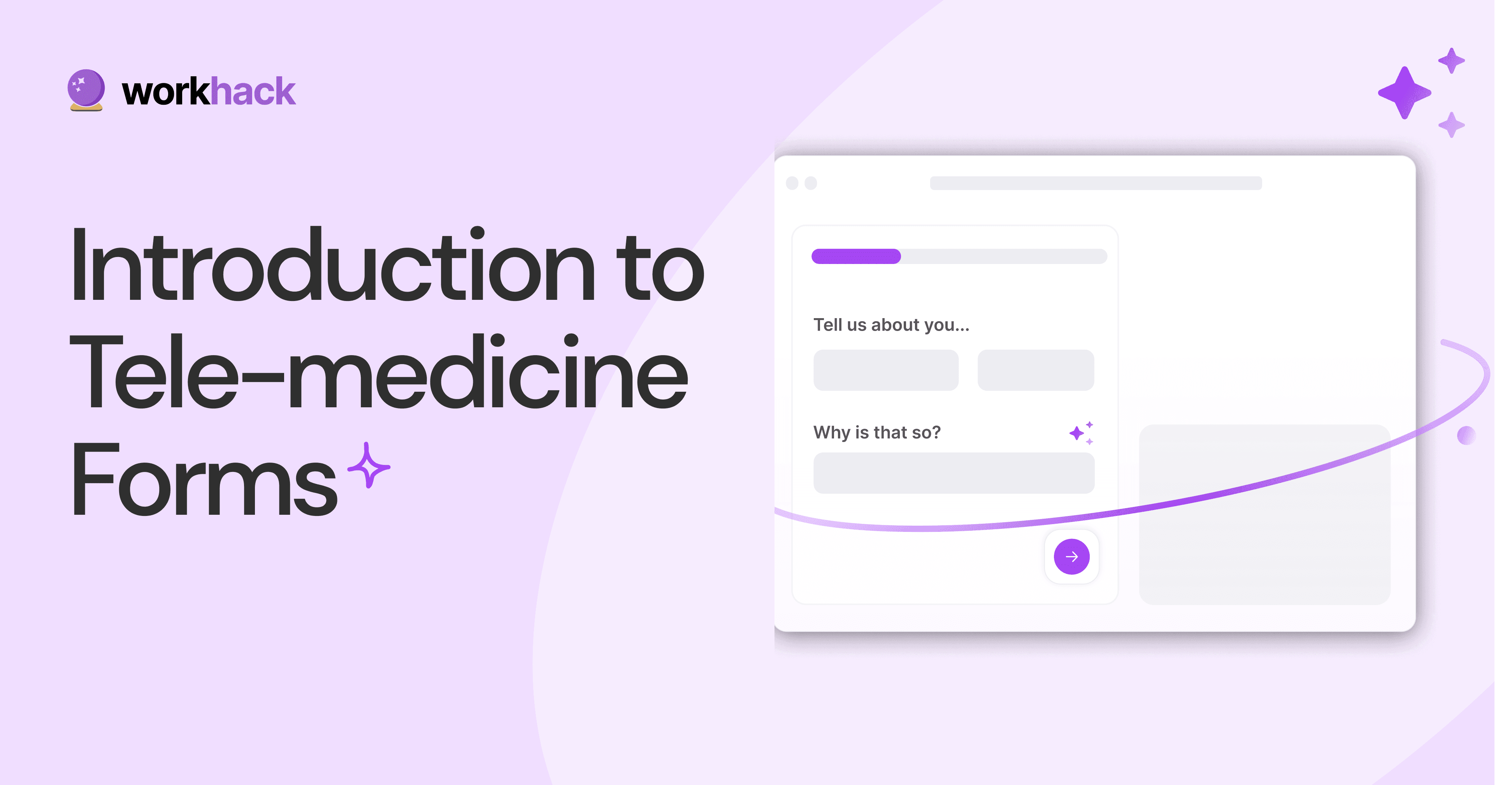
What, Why and How of Telemedicine Forms.
Telemedicine is on the rise and with different form builders out there, which one best suits your needs as a healthcare services provider?
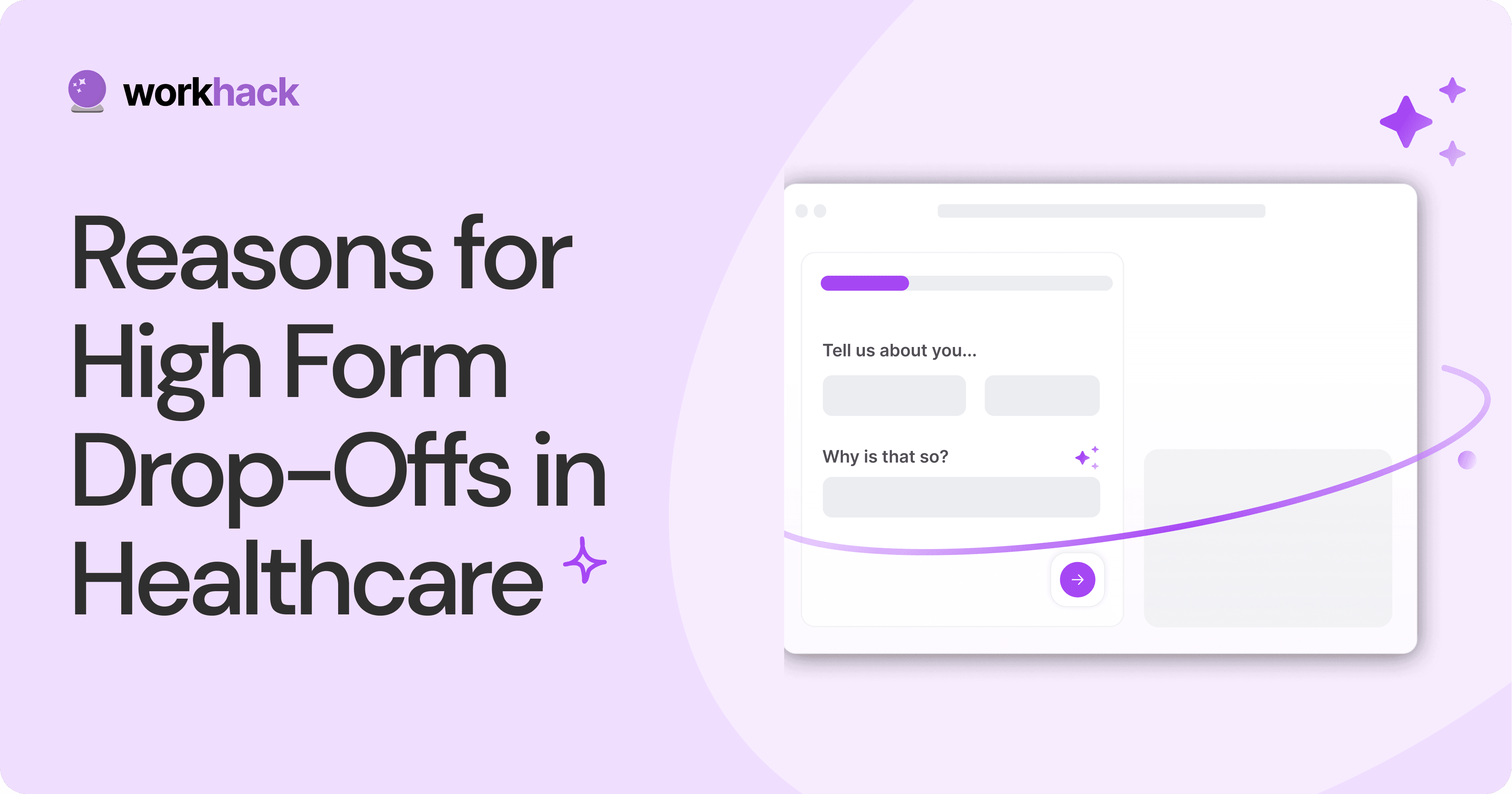
3 Reasons for Major Drop-Offs in Medical Forms.
No matter which healthcare form we pick, there are major drop-off reasons. We shall dive into the top 3 and learn how to resolve them in your next form.
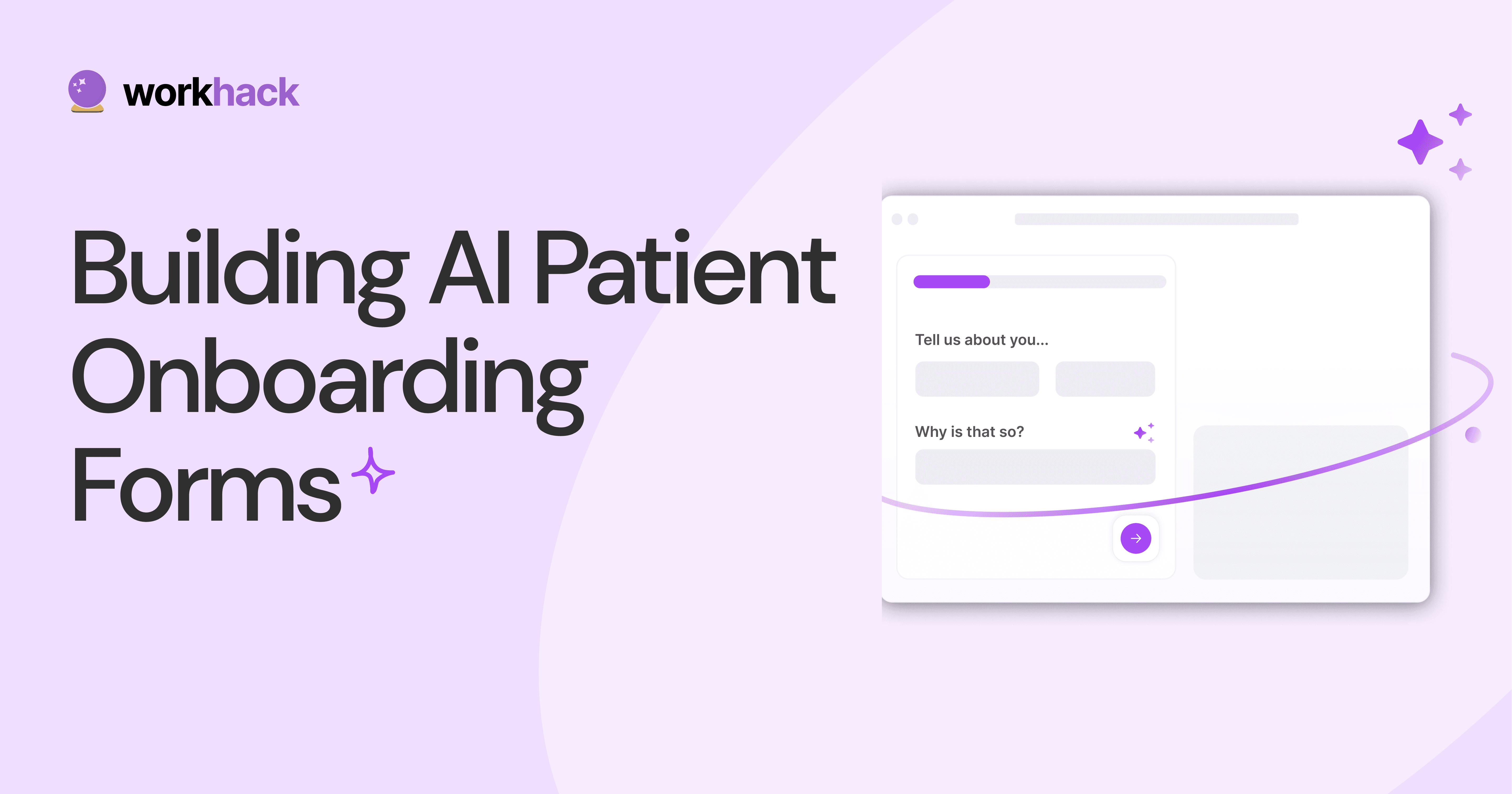
Patient Onboarding Forms - From Click to Clinic.
Patient onboarding forms are the first touchpoint for patients; getting this right for higher conversion rates is a must-have. Learn how to perfect them now.

5 Key Parts of a Good Patient Satisfaction Form.
The goal of patient satisfaction surveys is to course-correct the services of a healthcare provider. Patient feedback leads to a culture of patient-centric care.
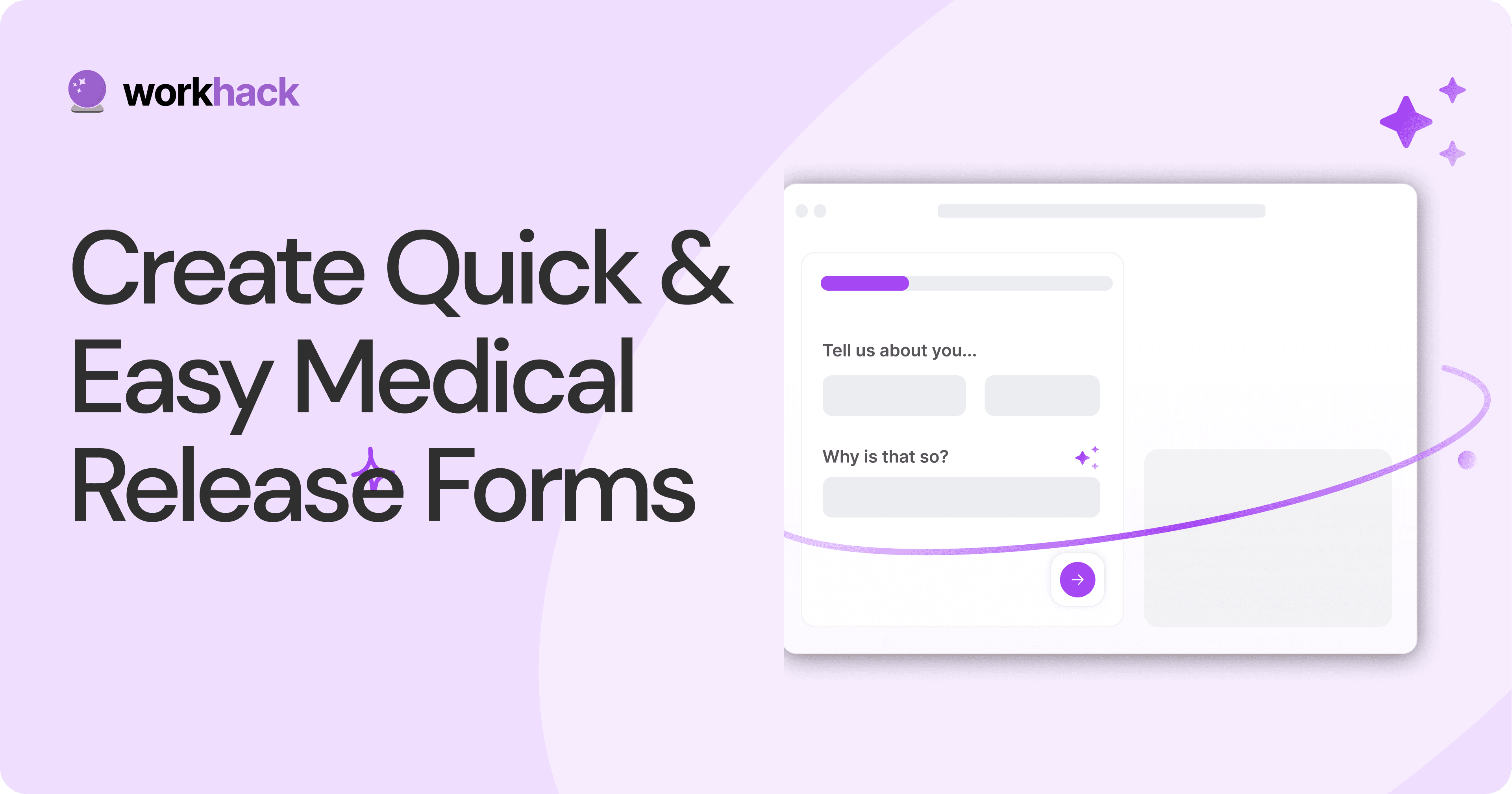
Build Quick and Easy Medical Release Forms.
Every HIPAA-compliant healthcare provider comes across medical release forms that involve details from medical history forms. Can they be shipped fast? Yes.
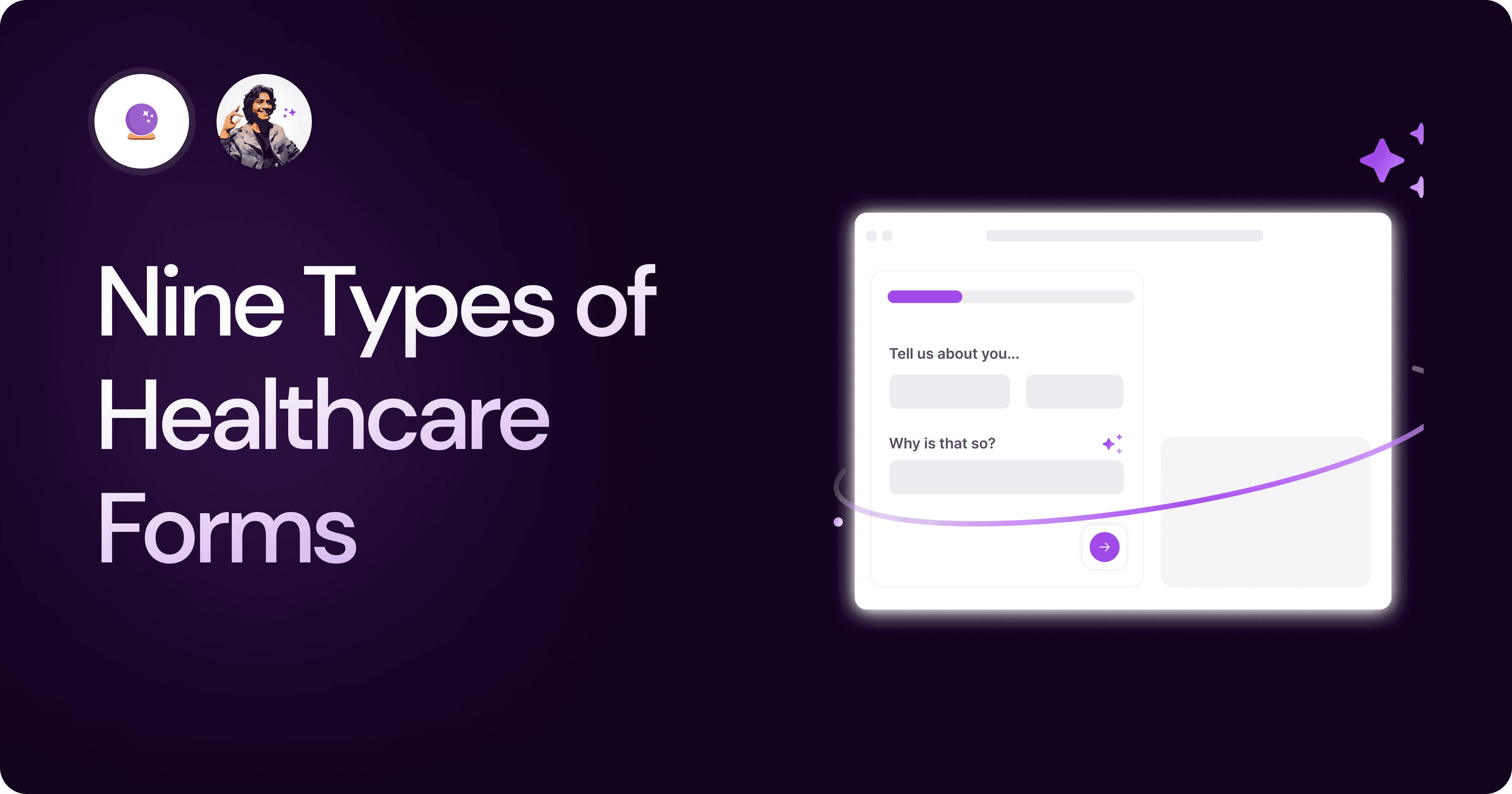
Nine Types of Healthcare and Medical Forms.
Medical forms are a must-have for any healthcare business or practitioner. Learn about the different kinds of medical and healthcare forms.
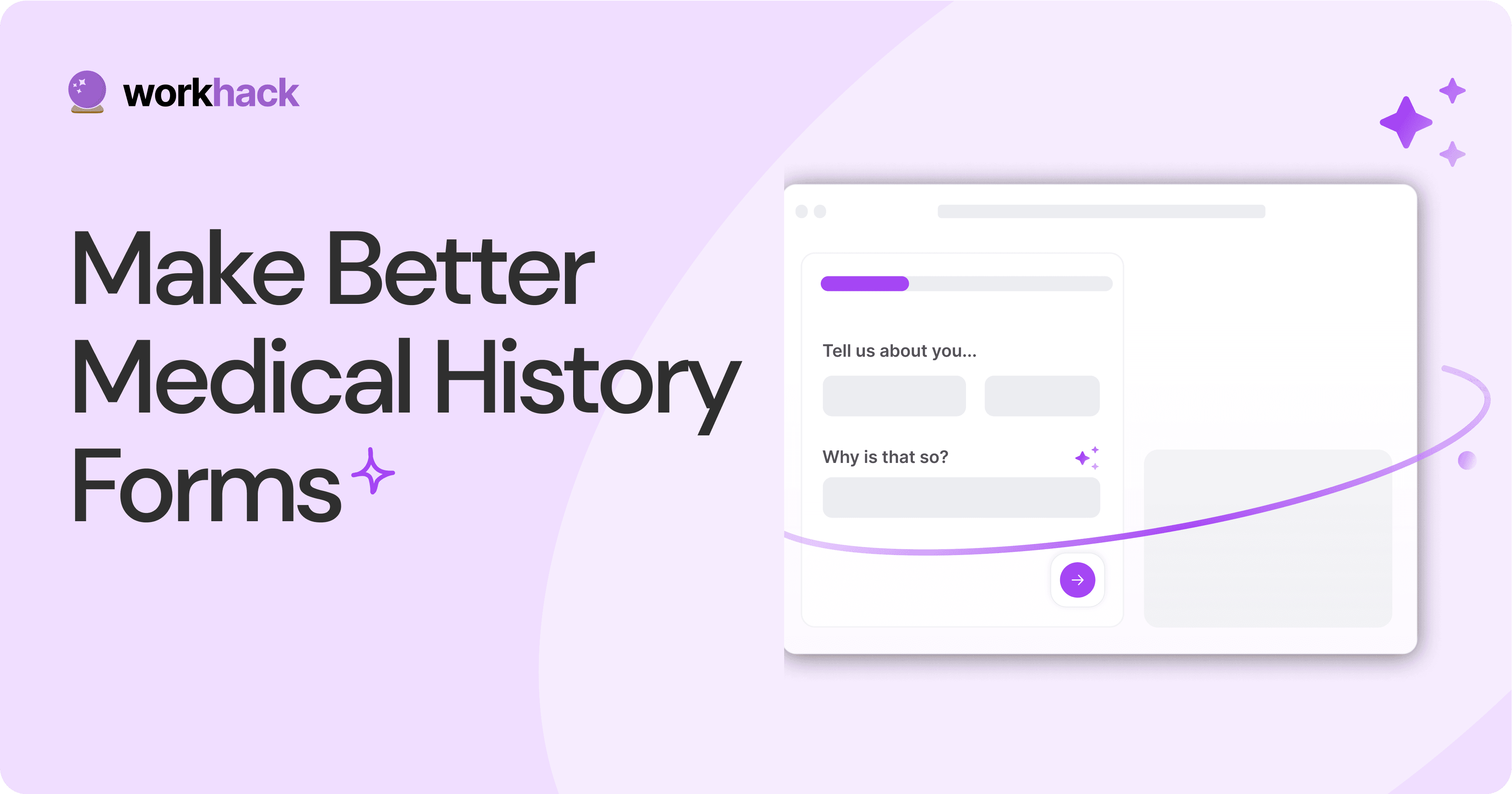
4 Tips for Better Medical History Forms.
Medical history forms are central to patient care, onboarding, and medical administration records. Learn how to make them easier to fill.
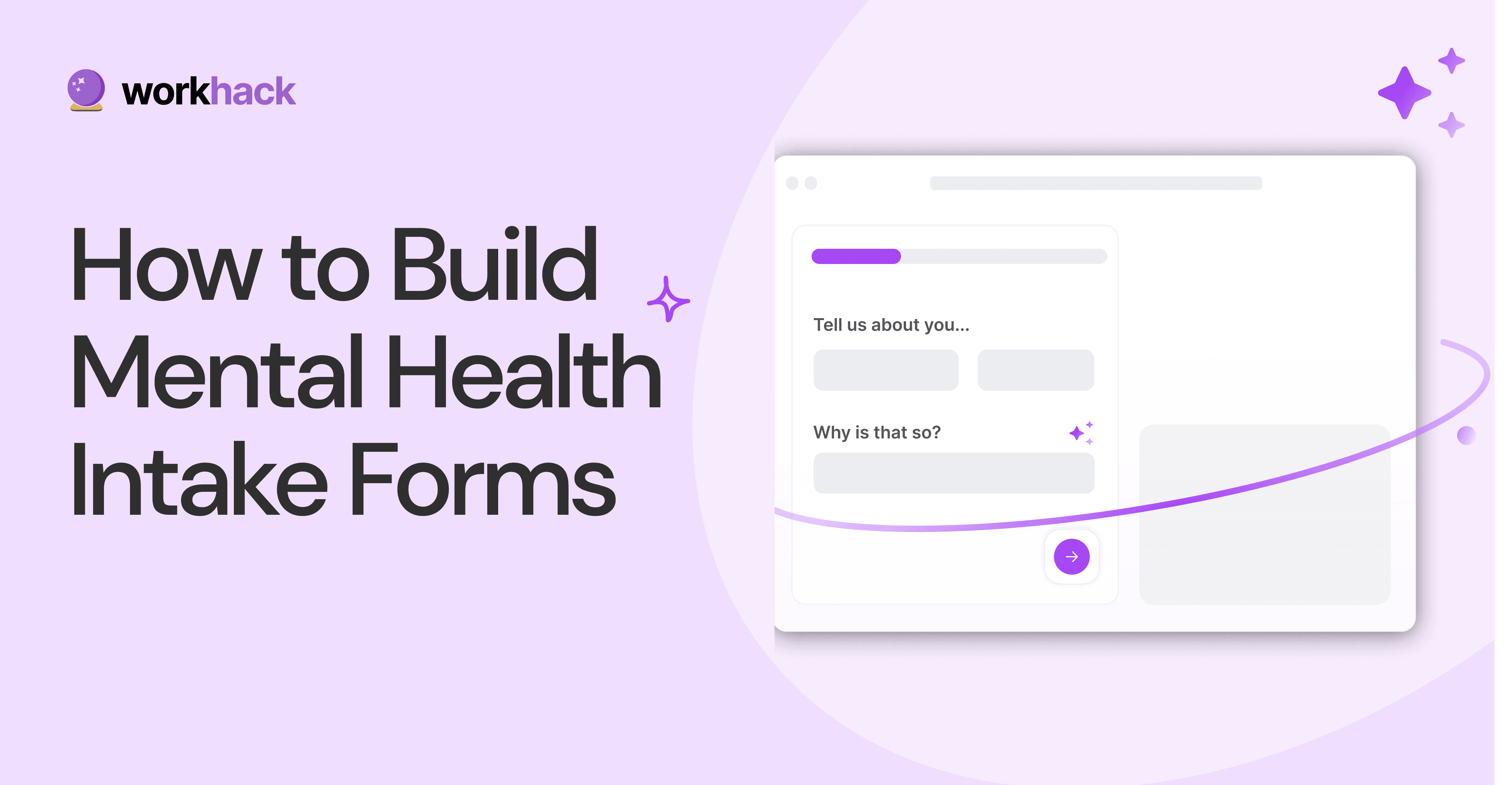
How to Build Mental Health Intake Forms?
Mental health intake forms are not like patient intake forms. Mental health intake forms deal with far more sensitive data and have specific design methods.
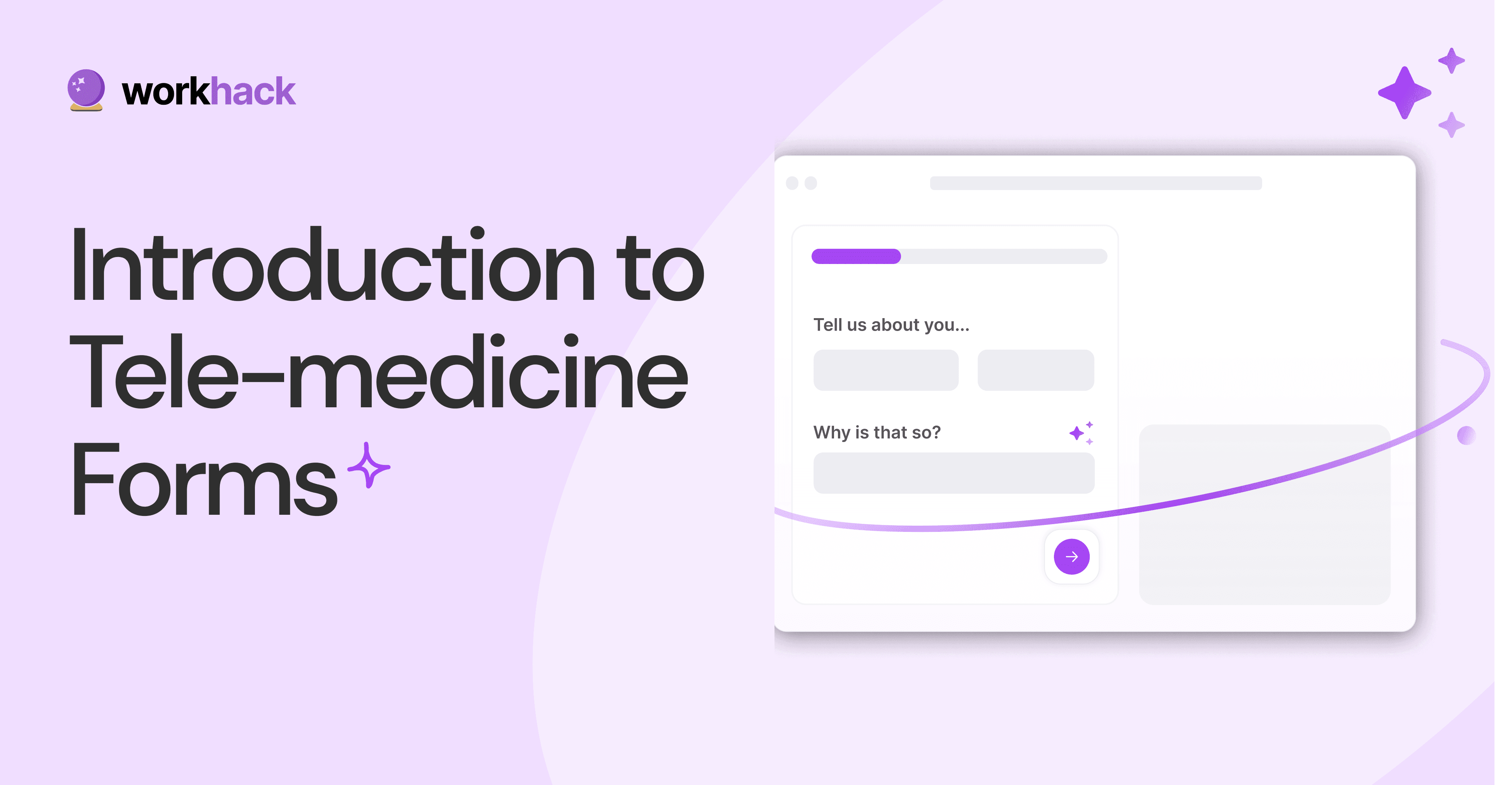
What, Why and How of Telemedicine Forms.
Telemedicine is on the rise and with different form builders out there, which one best suits your needs as a healthcare services provider?
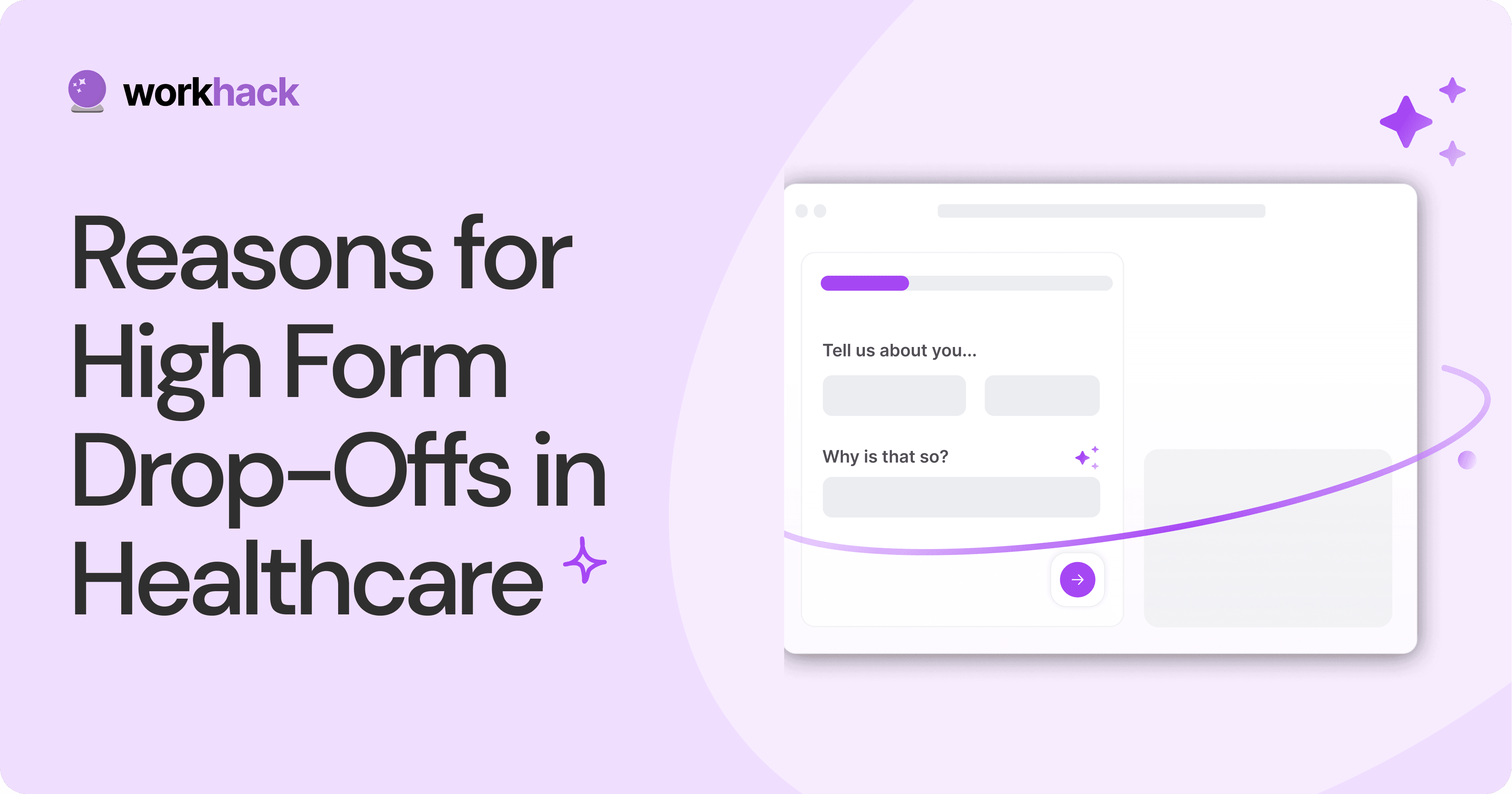
3 Reasons for Major Drop-Offs in Medical Forms.
No matter which healthcare form we pick, there are major drop-off reasons. We shall dive into the top 3 and learn how to resolve them in your next form.
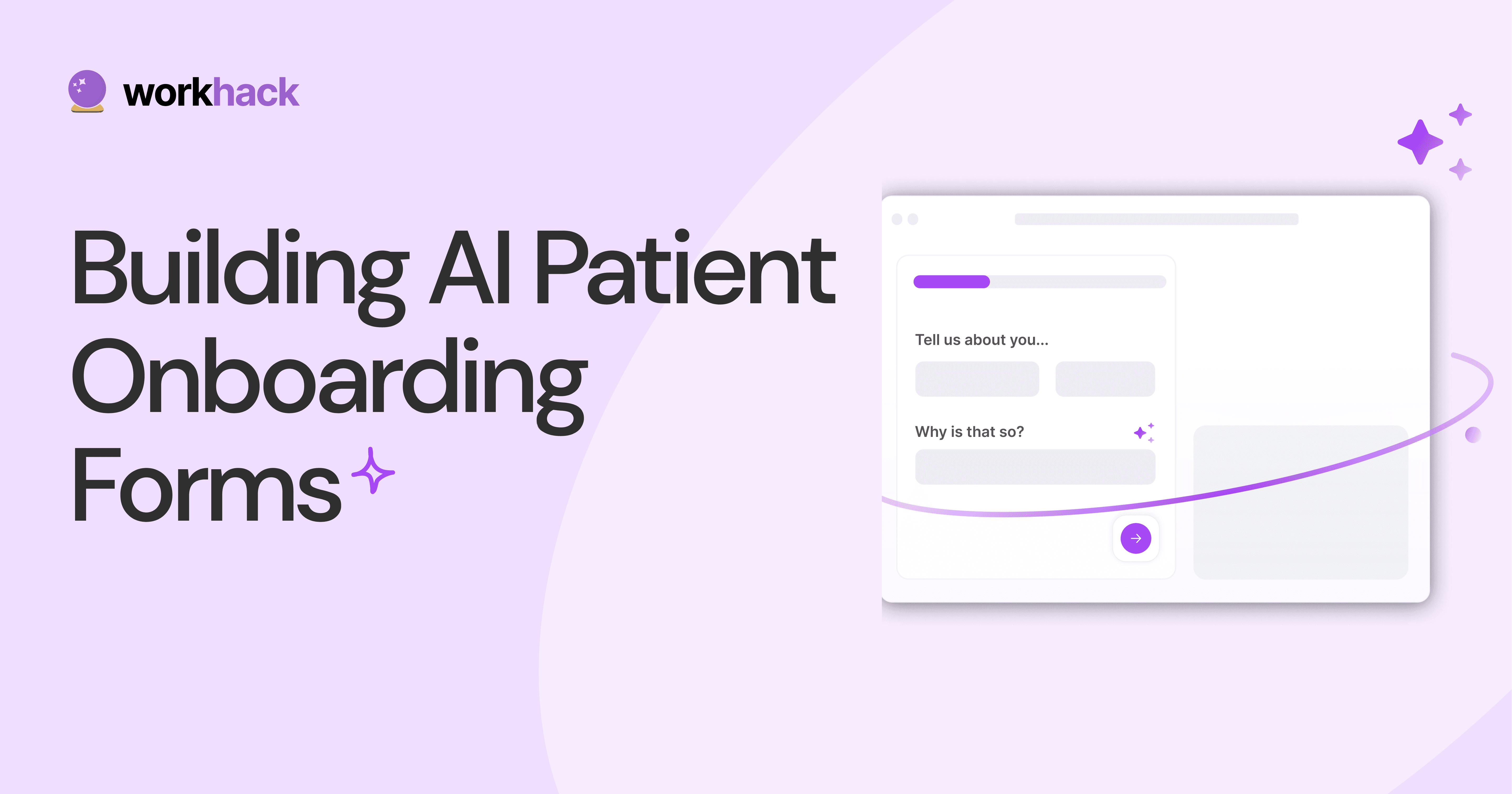
Patient Onboarding Forms - From Click to Clinic.
Patient onboarding forms are the first touchpoint for patients; getting this right for higher conversion rates is a must-have. Learn how to perfect them now.

5 Key Parts of a Good Patient Satisfaction Form.
The goal of patient satisfaction surveys is to course-correct the services of a healthcare provider. Patient feedback leads to a culture of patient-centric care.
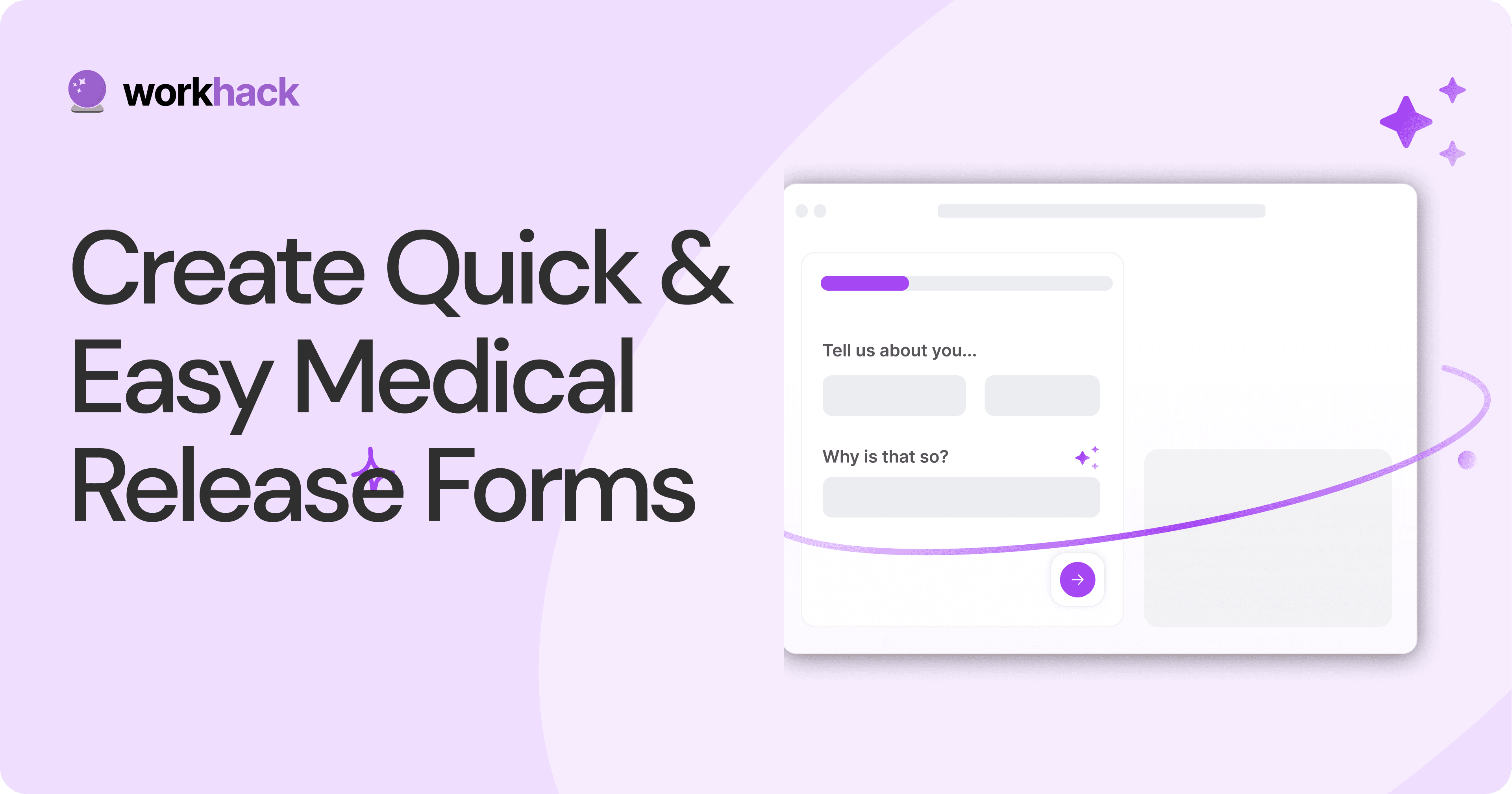
Build Quick and Easy Medical Release Forms.
Every HIPAA-compliant healthcare provider comes across medical release forms that involve details from medical history forms. Can they be shipped fast? Yes.
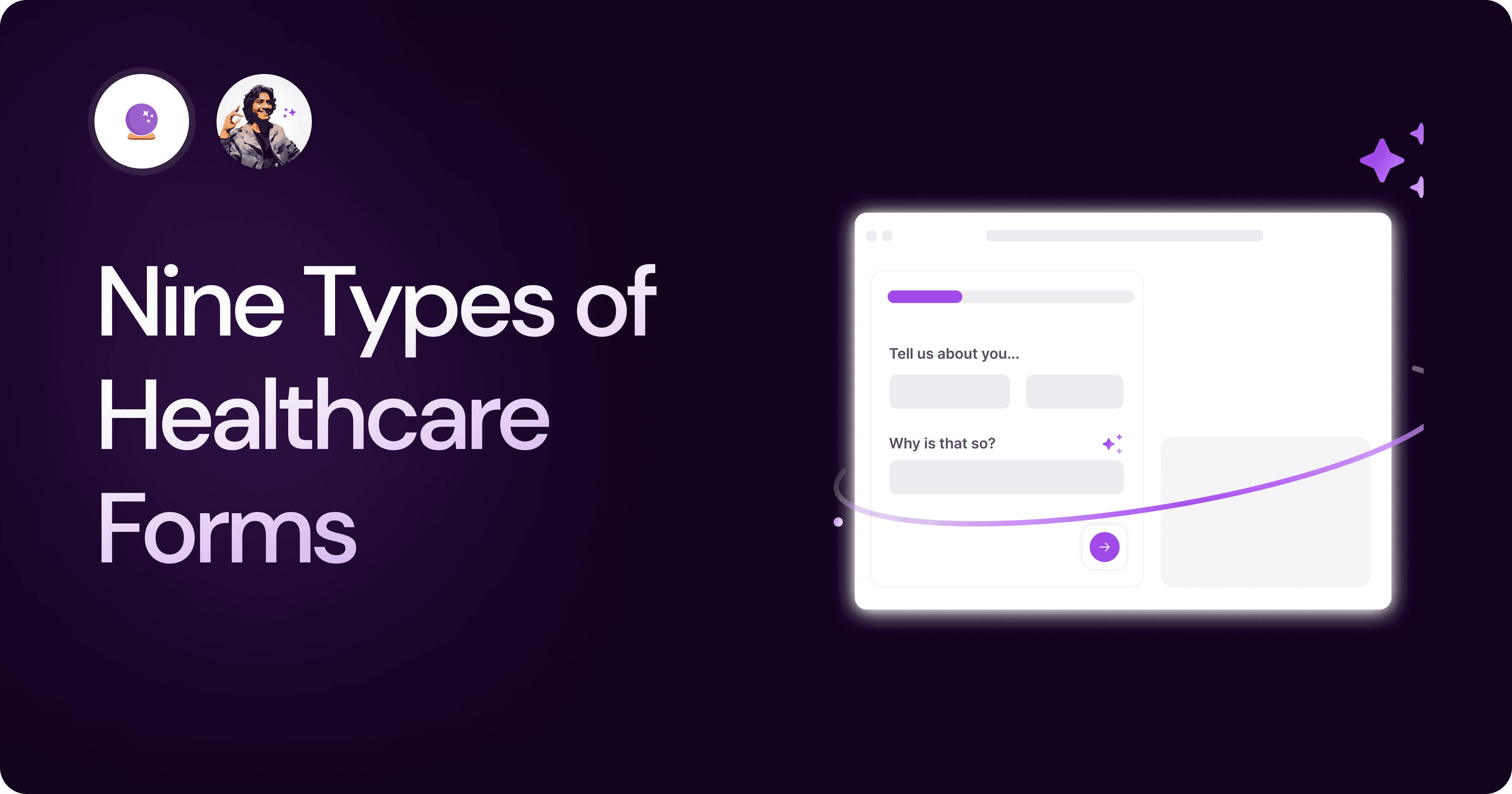
Nine Types of Healthcare and Medical Forms.
Medical forms are a must-have for any healthcare business or practitioner. Learn about the different kinds of medical and healthcare forms.
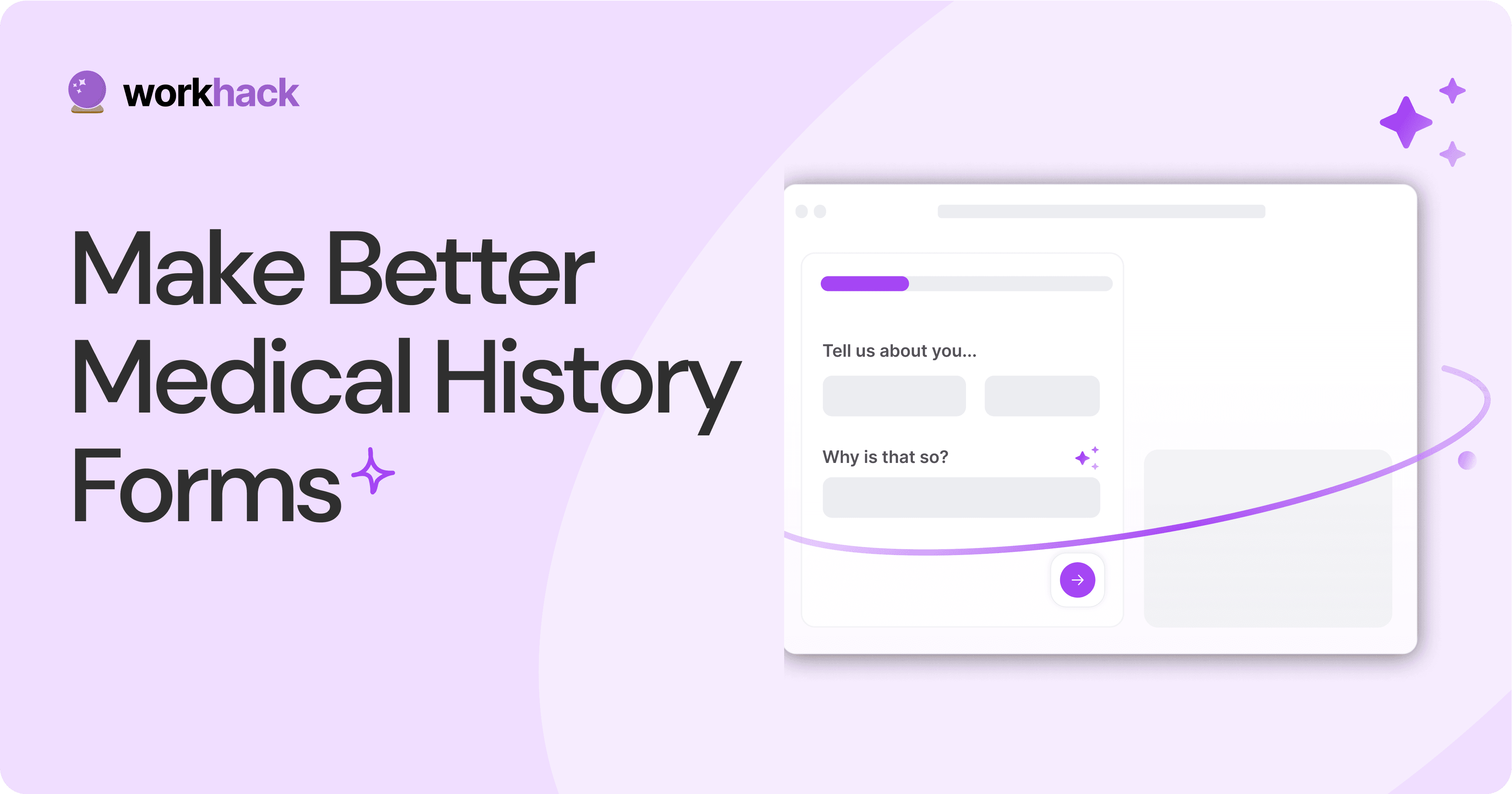
4 Tips for Better Medical History Forms.
Medical history forms are central to patient care, onboarding, and medical administration records. Learn how to make them easier to fill.
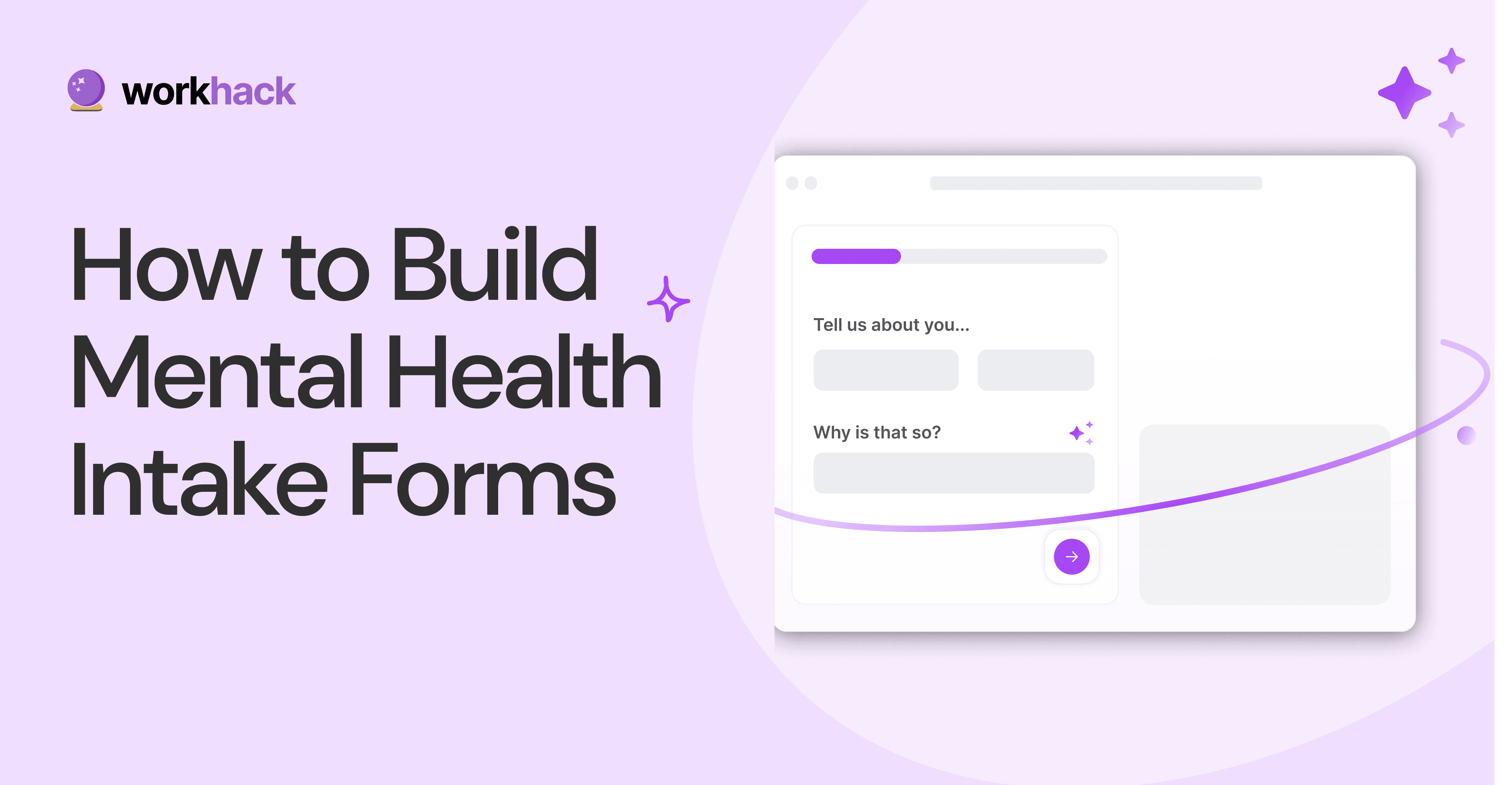
How to Build Mental Health Intake Forms?
Mental health intake forms are not like patient intake forms. Mental health intake forms deal with far more sensitive data and have specific design methods.
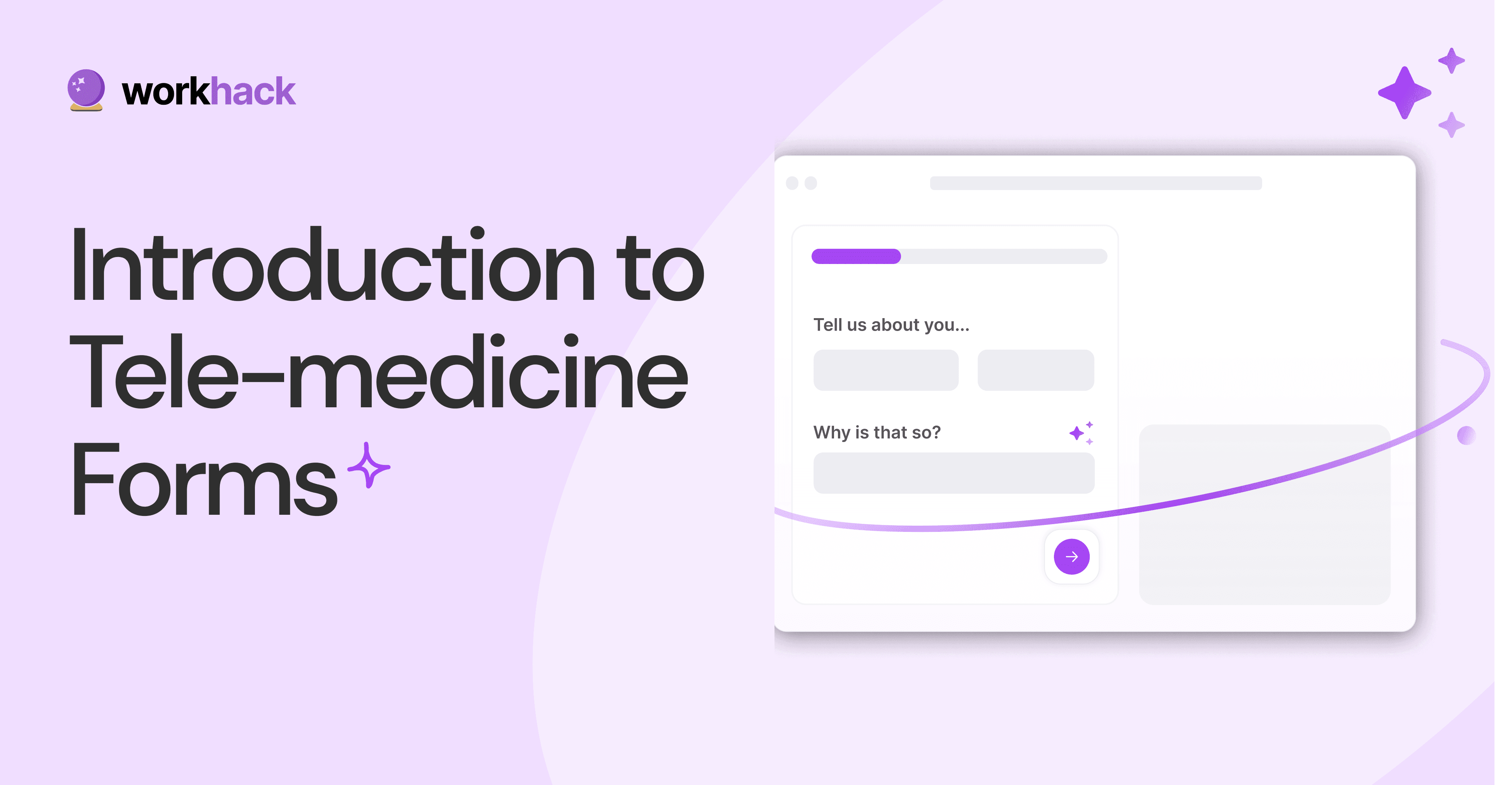
What, Why and How of Telemedicine Forms.
Telemedicine is on the rise and with different form builders out there, which one best suits your needs as a healthcare services provider?
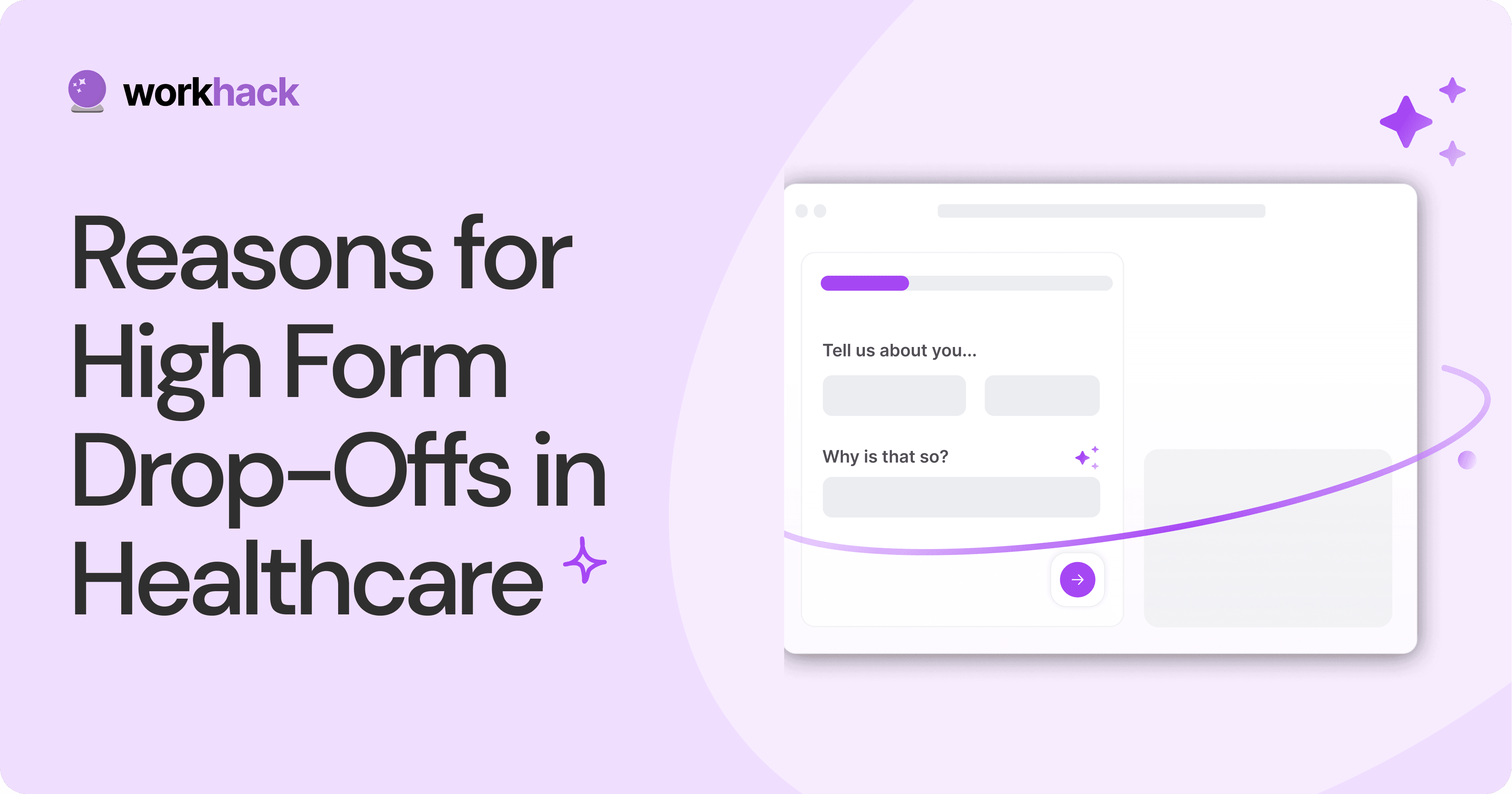
3 Reasons for Major Drop-Offs in Medical Forms.
No matter which healthcare form we pick, there are major drop-off reasons. We shall dive into the top 3 and learn how to resolve them in your next form.
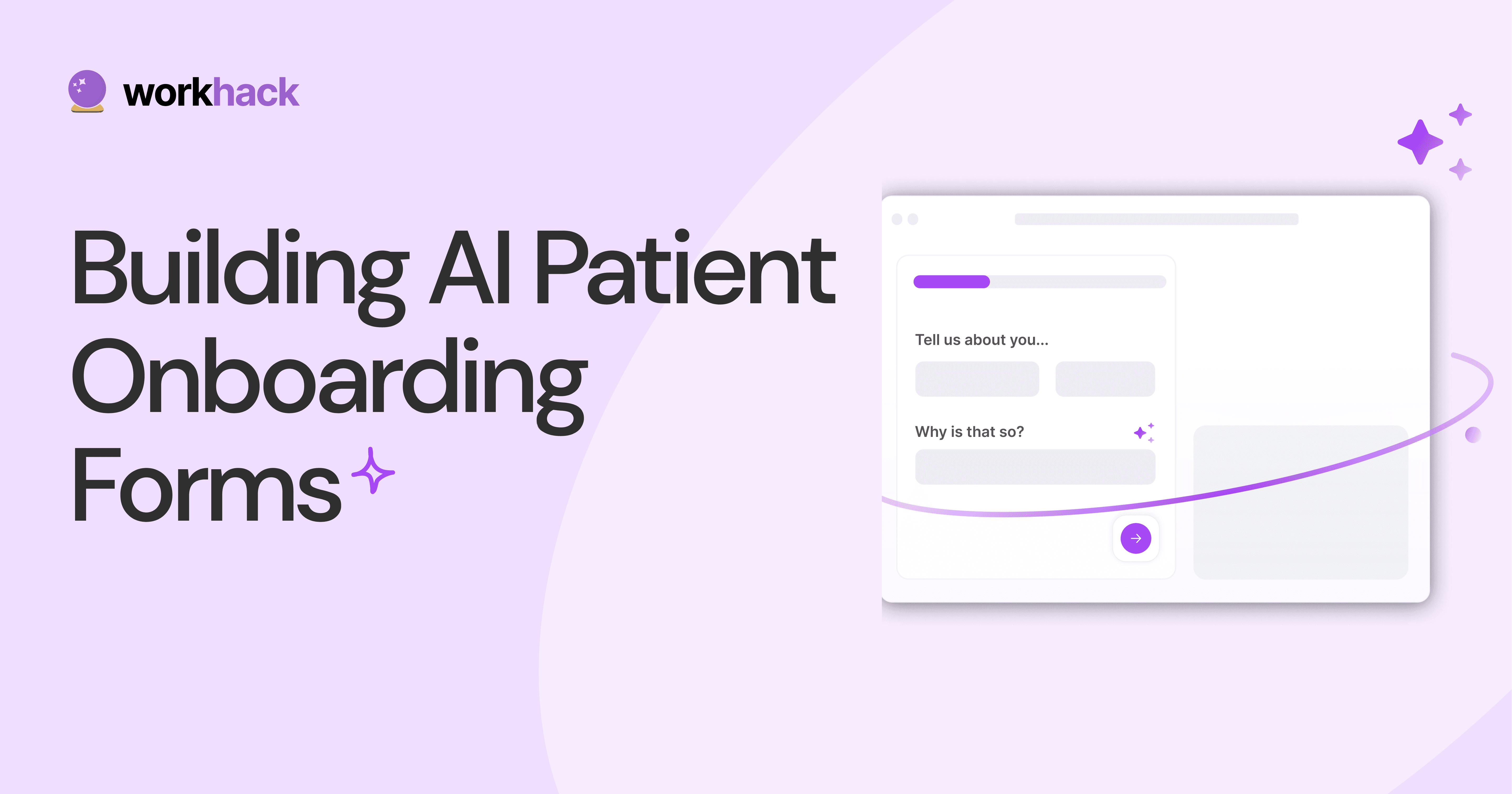
Patient Onboarding Forms - From Click to Clinic.
Patient onboarding forms are the first touchpoint for patients; getting this right for higher conversion rates is a must-have. Learn how to perfect them now.

5 Key Parts of a Good Patient Satisfaction Form.
The goal of patient satisfaction surveys is to course-correct the services of a healthcare provider. Patient feedback leads to a culture of patient-centric care.
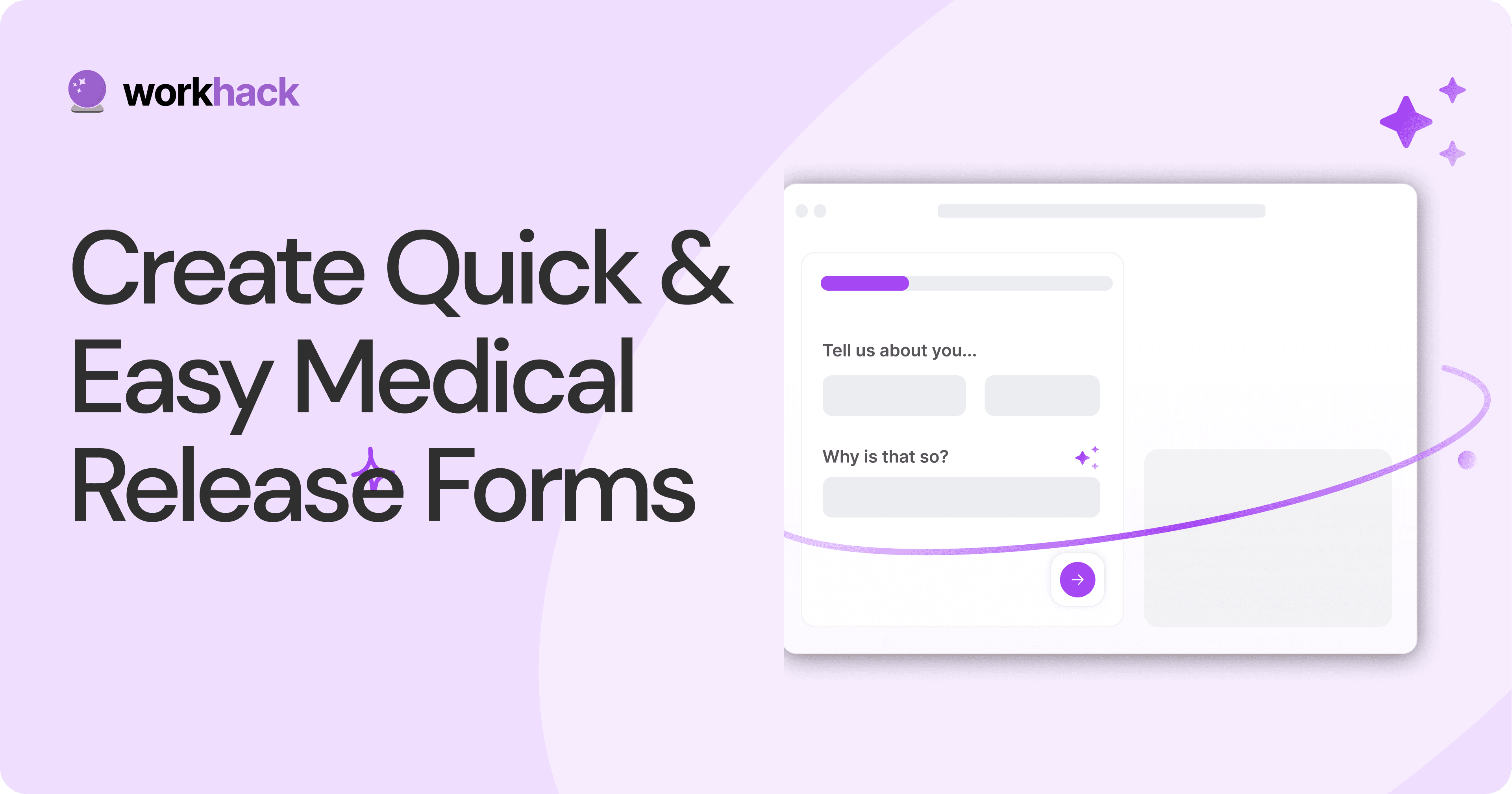
Build Quick and Easy Medical Release Forms.
Every HIPAA-compliant healthcare provider comes across medical release forms that involve details from medical history forms. Can they be shipped fast? Yes.
Subscribe to stay updated.
Subscribe to stay updated.
Subscribe to stay updated.
HC
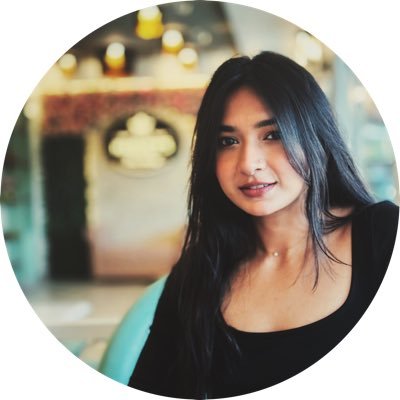
HC
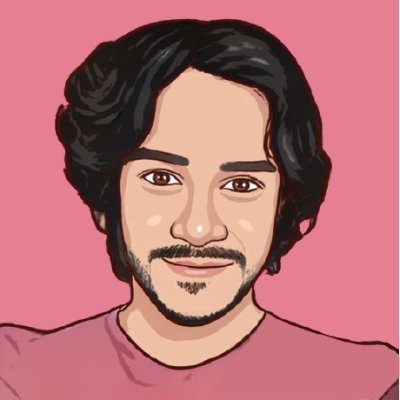
HC
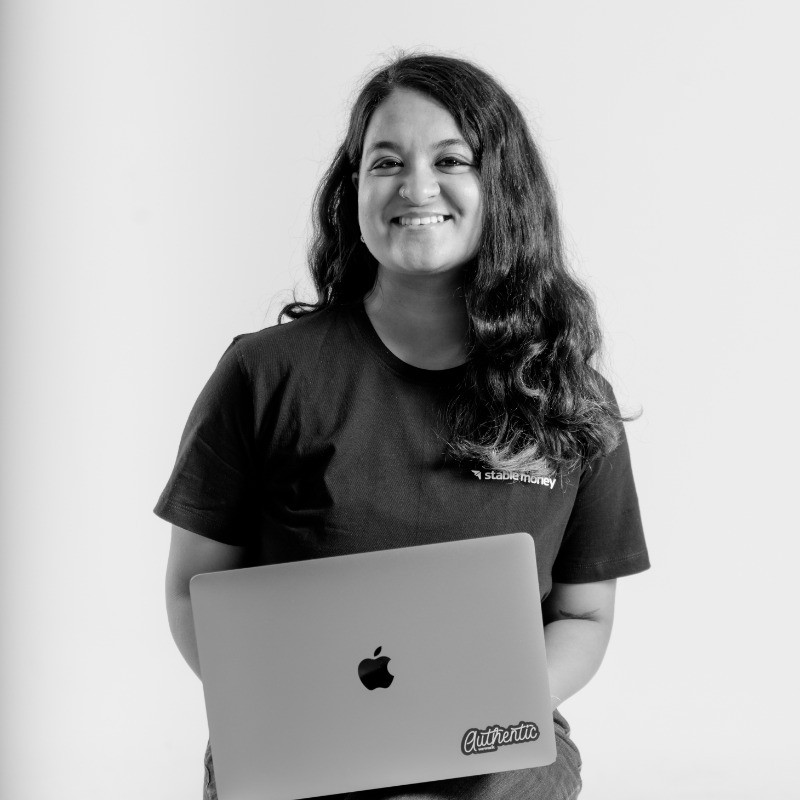
HC
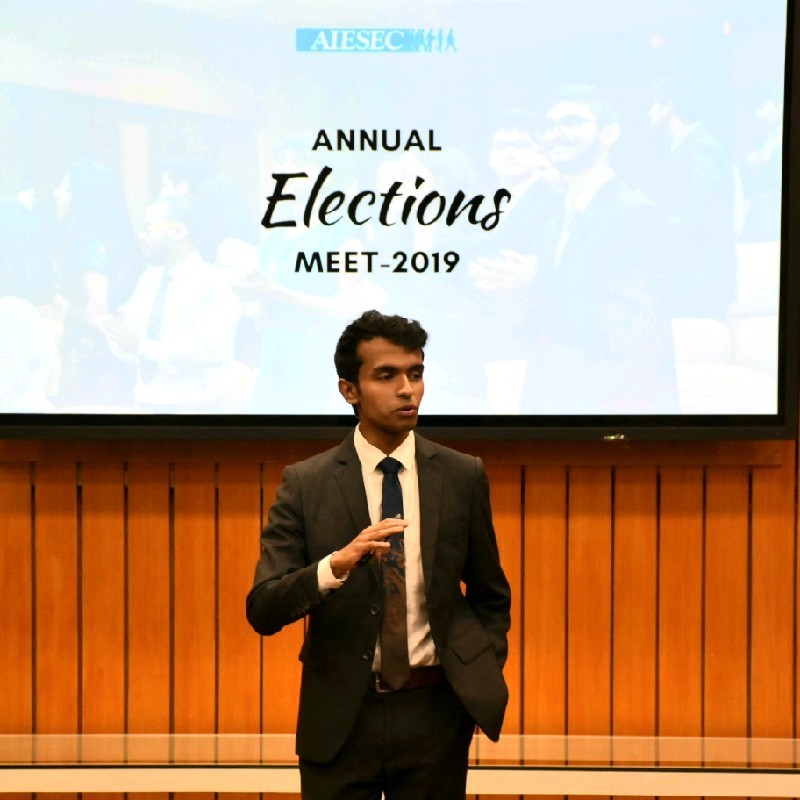
70+ people from across industries read our emails.
HC
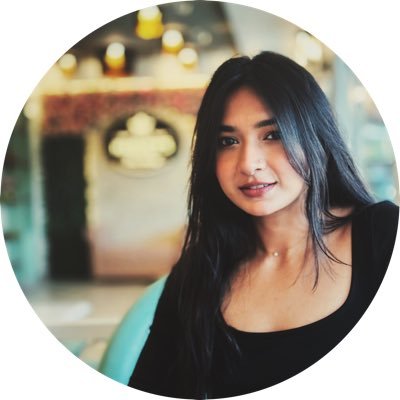
HC
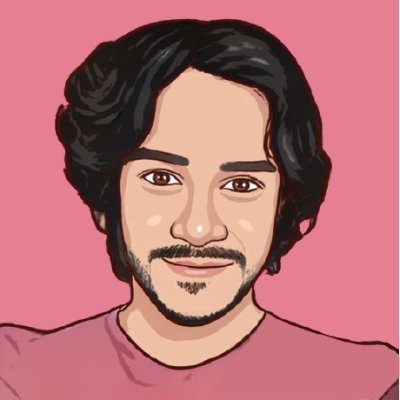
70+ people from across industries read our emails.
HC
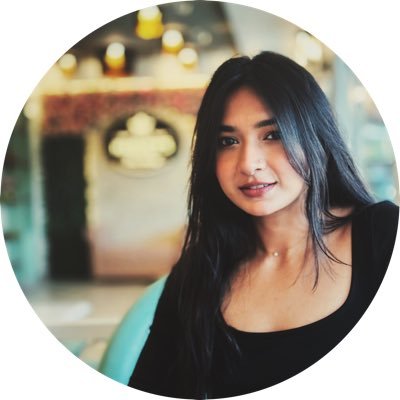
HC
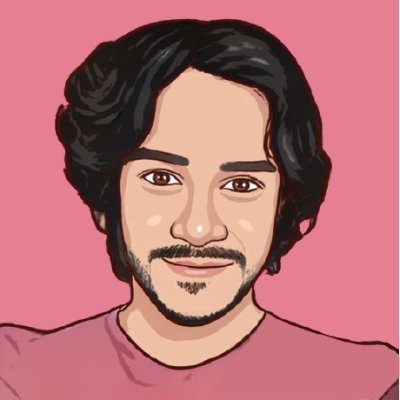
HC
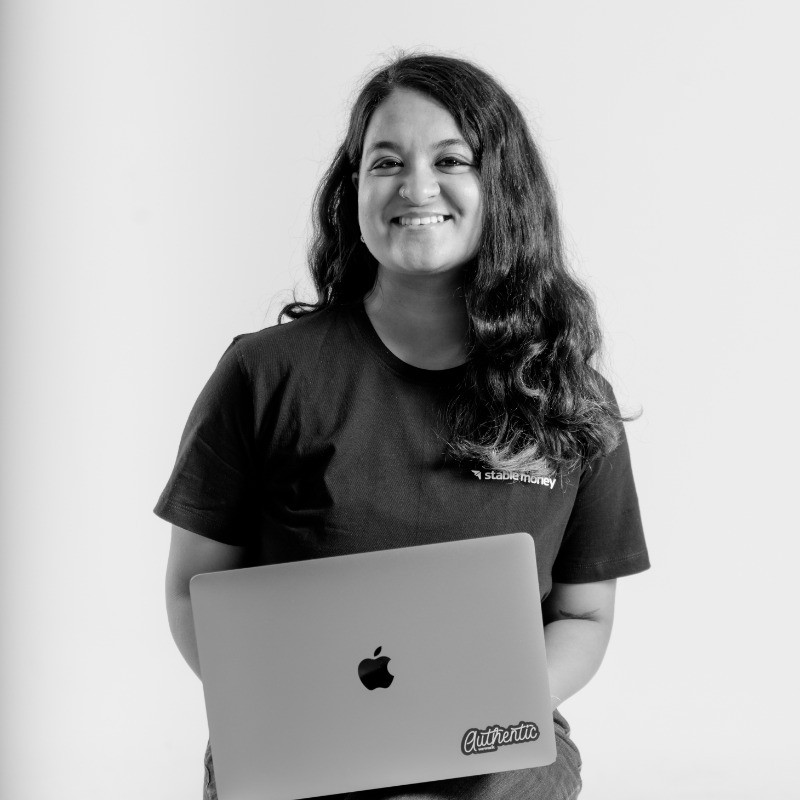
70+ people from across industries read our emails.
Bangalore, India / San Francisco, US
WorkHack Inc. 2023
Bangalore, India
San Francisco, US
WorkHack Inc. 2023
WorkHack Inc. 2023
Bangalore, India / San Francisco, US
WorkHack Inc. 2023
Bangalore, India / San Francisco, US