Market Researchers' Perception and Adoption of AI-Driven Technologies
Market Researchers' Perception and Adoption of AI-Driven Technologies
Market Researchers' Perception and Adoption of AI-Driven Technologies



Artificial Intelligence (AI) has transformed nearly every industry, and market research is no exception. For market researchers, AI represents both a beacon of innovation and a source of cautious contemplation. The potential benefits are tantalizing: faster data analysis, deeper insights, and streamlined processes. Yet, alongside these promises come concerns about data privacy, algorithmic biases, and the future role of traditional research methods.
Perception: Balancing Excitement with Caution
Market researchers are intrigued by AI's ability to transform their field. The prospect of automating repetitive tasks like data collection and analysis frees up valuable time for strategic thinking. AI-driven tools such as sentiment analysis and predictive analytics offer the promise of uncovering patterns and trends that human analysts might miss. However, skepticism remains regarding AI's reliability, ethical implications, and the potential to replace human intuition in interpreting complex data.
Adoption: Integrating AI into the Research Workflow
Despite reservations, market research agencies are increasingly integrating AI into their operations. Practical applications abound:
Sentiment Analysis: AI algorithms sift through vast amounts of textual data—from social media posts to customer reviews—extracting nuanced sentiments and trends.
Predictive Analytics: By analyzing historical data, AI models forecast market trends, consumer behavior shifts, and product performance with greater accuracy.
Automation: Routine tasks such as data cleaning, report generation, and survey design are streamlined, enhancing efficiency and scalability.
How Qualitative Market Researchers Leverage AI-Native Tools
Qualitative market research, traditionally reliant on human interpretation and empathy, is also being transformed by AI. Here’s how AI-native tools are reshaping qualitative research practices:
Enhanced Data Collection Efficiency
AI streamlines data collection by automating processes such as transcription and sentiment coding. This accelerates the gathering of insights from qualitative methods like interviews, focus groups, and open-ended surveys, enabling researchers to handle larger volumes of data more effectively.
Deep Analysis and Insights Unveiled
Natural Language Processing (NLP) algorithms decode the subtleties of language, revealing underlying sentiments and emerging themes in qualitative data. AI-driven analytics complement human intuition by identifying patterns and connections across large datasets, offering a more comprehensive understanding of consumer perceptions and behaviors.
Practical Use Cases in Qualitative Research
Theme Identification: AI algorithms categorize and prioritize themes within qualitative data, guiding researchers to focus on key findings and actionable insights.
Personalization: During interviews or surveys, AI suggests personalized follow-up questions based on respondents' previous answers, fostering deeper engagement and richer data.
Real-time Feedback: AI-powered surveys provide instant feedback loops, allowing researchers to adapt strategies and refine research methodologies on the fly, ensuring relevance and responsiveness.
Practical Integration Example
Imagine a market research firm launching a new product:
AI-Powered Sentiment Analysis: The firm uses AI to analyze social media conversations and customer reviews about the product. AI identifies positive sentiments towards specific features and uncovers areas for improvement.
Qualitative Insights: Researchers conduct in-depth interviews and focus groups. AI aids in transcribing and analyzing these sessions, pinpointing emerging trends and consumer preferences.
Continuous Improvement: AI-powered surveys collect ongoing feedback post-launch, enabling the firm to iteratively refine the product based on real-time consumer insights.
Practical Challenges in AI Adoption and Integration in Qualitative Market Research
Adopting AI in qualitative market research holds tremendous promise, but it also presents several practical challenges that researchers and agencies must navigate effectively. These challenges stem from technological limitations, ethical considerations, and the need to balance automation with human expertise.
1. Data Privacy and Ethical Concerns
Challenge: AI tools rely on vast amounts of data, often including sensitive information from interviews, focus groups, and social media. Ensuring participant privacy, obtaining informed consent, and safeguarding data integrity are critical ethical considerations.
AI Solution: AI-native tools incorporate robust data anonymization techniques and encryption protocols to protect participant identities. Automated data anonymization algorithms ensure that personally identifiable information (PII) is masked or removed, preserving confidentiality while allowing for meaningful analysis.
2. Algorithmic Bias and Interpretation
Challenge: AI algorithms can inherit biases from training data, potentially leading to skewed results or reinforcing existing stereotypes. Ensuring fairness and transparency in algorithmic decision-making is essential to maintain credibility in research outcomes.
AI Solution: AI-native tools employ bias detection algorithms and fairness metrics during model development and deployment. Techniques such as algorithmic auditing and diverse training datasets help mitigate biases by promoting balanced representations and reducing unintended discrimination.
3. Integration with Human Expertise
Challenge: Qualitative research often requires nuanced interpretation and contextual understanding that AI may struggle to replicate. Balancing the automation of repetitive tasks with the expertise of human researchers is crucial for generating actionable insights.
AI Solution: AI-native tools augment human expertise by automating time-consuming tasks like transcription, sentiment analysis, and theme identification. Natural Language Processing (NLP) algorithms enhance data interpretation by detecting subtle linguistic cues and sentiment nuances, enabling researchers to focus on higher-level analysis and strategic decision-making.
4. Complexity of Qualitative Data Analysis
Challenge: Qualitative data is inherently complex, characterized by unstructured formats, diverse linguistic styles, and subjective interpretations. Extracting meaningful insights requires sophisticated analytical tools capable of handling variability and ambiguity.
AI Solution: AI-powered analytics platforms leverage advanced machine learning algorithms to process and categorize qualitative data efficiently. Text mining techniques, topic modeling, and sentiment analysis algorithms streamline data interpretation, revealing patterns and trends that might otherwise go unnoticed. Interactive visualization tools further enhance researchers' ability to explore and communicate findings effectively.
5. Cost and Resource Allocation
Challenge: Implementing AI technologies involves initial investment in infrastructure, software licenses, and training. Smaller agencies or research teams with limited budgets may face barriers to adopting AI-driven solutions effectively.
AI Solution: AI-native tools offer scalable solutions tailored to diverse budgetary constraints. Cloud-based platforms and subscription models reduce upfront costs and provide flexibility in resource allocation. Moreover, AI's efficiency in automating repetitive tasks minimizes operational expenses over time, allowing agencies to reallocate resources towards strategic initiatives and client engagement.
Conclusion: Embracing Innovation while Navigating Challenges
Qualitative market researchers are at a pivotal moment, embracing AI's transformative potential while navigating its complexities. As AI-native tools continue to evolve, researchers must remain vigilant about ethical considerations, data privacy safeguards, and the symbiotic relationship between human judgment and machine intelligence.
The integration of AI into qualitative market research represents a paradigm shift, empowering researchers to glean deeper insights and enhance decision-making capabilities. It’s critical to address practical challenges through ethical frameworks, algorithmic transparency, and synergistic collaboration between AI and human expertise.
Market research agencies leverage AI-native tools like Metaforms.ai to unlock new dimensions of understanding in consumer behavior and market dynamics. Embracing innovation while mitigating risks ensures that AI adoption in qualitative research is not just a technological advancement but a strategic imperative for staying competitive in a rapidly evolving industry landscape. Sign-up for a demo today!
Artificial Intelligence (AI) has transformed nearly every industry, and market research is no exception. For market researchers, AI represents both a beacon of innovation and a source of cautious contemplation. The potential benefits are tantalizing: faster data analysis, deeper insights, and streamlined processes. Yet, alongside these promises come concerns about data privacy, algorithmic biases, and the future role of traditional research methods.
Perception: Balancing Excitement with Caution
Market researchers are intrigued by AI's ability to transform their field. The prospect of automating repetitive tasks like data collection and analysis frees up valuable time for strategic thinking. AI-driven tools such as sentiment analysis and predictive analytics offer the promise of uncovering patterns and trends that human analysts might miss. However, skepticism remains regarding AI's reliability, ethical implications, and the potential to replace human intuition in interpreting complex data.
Adoption: Integrating AI into the Research Workflow
Despite reservations, market research agencies are increasingly integrating AI into their operations. Practical applications abound:
Sentiment Analysis: AI algorithms sift through vast amounts of textual data—from social media posts to customer reviews—extracting nuanced sentiments and trends.
Predictive Analytics: By analyzing historical data, AI models forecast market trends, consumer behavior shifts, and product performance with greater accuracy.
Automation: Routine tasks such as data cleaning, report generation, and survey design are streamlined, enhancing efficiency and scalability.
How Qualitative Market Researchers Leverage AI-Native Tools
Qualitative market research, traditionally reliant on human interpretation and empathy, is also being transformed by AI. Here’s how AI-native tools are reshaping qualitative research practices:
Enhanced Data Collection Efficiency
AI streamlines data collection by automating processes such as transcription and sentiment coding. This accelerates the gathering of insights from qualitative methods like interviews, focus groups, and open-ended surveys, enabling researchers to handle larger volumes of data more effectively.
Deep Analysis and Insights Unveiled
Natural Language Processing (NLP) algorithms decode the subtleties of language, revealing underlying sentiments and emerging themes in qualitative data. AI-driven analytics complement human intuition by identifying patterns and connections across large datasets, offering a more comprehensive understanding of consumer perceptions and behaviors.
Practical Use Cases in Qualitative Research
Theme Identification: AI algorithms categorize and prioritize themes within qualitative data, guiding researchers to focus on key findings and actionable insights.
Personalization: During interviews or surveys, AI suggests personalized follow-up questions based on respondents' previous answers, fostering deeper engagement and richer data.
Real-time Feedback: AI-powered surveys provide instant feedback loops, allowing researchers to adapt strategies and refine research methodologies on the fly, ensuring relevance and responsiveness.
Practical Integration Example
Imagine a market research firm launching a new product:
AI-Powered Sentiment Analysis: The firm uses AI to analyze social media conversations and customer reviews about the product. AI identifies positive sentiments towards specific features and uncovers areas for improvement.
Qualitative Insights: Researchers conduct in-depth interviews and focus groups. AI aids in transcribing and analyzing these sessions, pinpointing emerging trends and consumer preferences.
Continuous Improvement: AI-powered surveys collect ongoing feedback post-launch, enabling the firm to iteratively refine the product based on real-time consumer insights.
Practical Challenges in AI Adoption and Integration in Qualitative Market Research
Adopting AI in qualitative market research holds tremendous promise, but it also presents several practical challenges that researchers and agencies must navigate effectively. These challenges stem from technological limitations, ethical considerations, and the need to balance automation with human expertise.
1. Data Privacy and Ethical Concerns
Challenge: AI tools rely on vast amounts of data, often including sensitive information from interviews, focus groups, and social media. Ensuring participant privacy, obtaining informed consent, and safeguarding data integrity are critical ethical considerations.
AI Solution: AI-native tools incorporate robust data anonymization techniques and encryption protocols to protect participant identities. Automated data anonymization algorithms ensure that personally identifiable information (PII) is masked or removed, preserving confidentiality while allowing for meaningful analysis.
2. Algorithmic Bias and Interpretation
Challenge: AI algorithms can inherit biases from training data, potentially leading to skewed results or reinforcing existing stereotypes. Ensuring fairness and transparency in algorithmic decision-making is essential to maintain credibility in research outcomes.
AI Solution: AI-native tools employ bias detection algorithms and fairness metrics during model development and deployment. Techniques such as algorithmic auditing and diverse training datasets help mitigate biases by promoting balanced representations and reducing unintended discrimination.
3. Integration with Human Expertise
Challenge: Qualitative research often requires nuanced interpretation and contextual understanding that AI may struggle to replicate. Balancing the automation of repetitive tasks with the expertise of human researchers is crucial for generating actionable insights.
AI Solution: AI-native tools augment human expertise by automating time-consuming tasks like transcription, sentiment analysis, and theme identification. Natural Language Processing (NLP) algorithms enhance data interpretation by detecting subtle linguistic cues and sentiment nuances, enabling researchers to focus on higher-level analysis and strategic decision-making.
4. Complexity of Qualitative Data Analysis
Challenge: Qualitative data is inherently complex, characterized by unstructured formats, diverse linguistic styles, and subjective interpretations. Extracting meaningful insights requires sophisticated analytical tools capable of handling variability and ambiguity.
AI Solution: AI-powered analytics platforms leverage advanced machine learning algorithms to process and categorize qualitative data efficiently. Text mining techniques, topic modeling, and sentiment analysis algorithms streamline data interpretation, revealing patterns and trends that might otherwise go unnoticed. Interactive visualization tools further enhance researchers' ability to explore and communicate findings effectively.
5. Cost and Resource Allocation
Challenge: Implementing AI technologies involves initial investment in infrastructure, software licenses, and training. Smaller agencies or research teams with limited budgets may face barriers to adopting AI-driven solutions effectively.
AI Solution: AI-native tools offer scalable solutions tailored to diverse budgetary constraints. Cloud-based platforms and subscription models reduce upfront costs and provide flexibility in resource allocation. Moreover, AI's efficiency in automating repetitive tasks minimizes operational expenses over time, allowing agencies to reallocate resources towards strategic initiatives and client engagement.
Conclusion: Embracing Innovation while Navigating Challenges
Qualitative market researchers are at a pivotal moment, embracing AI's transformative potential while navigating its complexities. As AI-native tools continue to evolve, researchers must remain vigilant about ethical considerations, data privacy safeguards, and the symbiotic relationship between human judgment and machine intelligence.
The integration of AI into qualitative market research represents a paradigm shift, empowering researchers to glean deeper insights and enhance decision-making capabilities. It’s critical to address practical challenges through ethical frameworks, algorithmic transparency, and synergistic collaboration between AI and human expertise.
Market research agencies leverage AI-native tools like Metaforms.ai to unlock new dimensions of understanding in consumer behavior and market dynamics. Embracing innovation while mitigating risks ensures that AI adoption in qualitative research is not just a technological advancement but a strategic imperative for staying competitive in a rapidly evolving industry landscape. Sign-up for a demo today!
Artificial Intelligence (AI) has transformed nearly every industry, and market research is no exception. For market researchers, AI represents both a beacon of innovation and a source of cautious contemplation. The potential benefits are tantalizing: faster data analysis, deeper insights, and streamlined processes. Yet, alongside these promises come concerns about data privacy, algorithmic biases, and the future role of traditional research methods.
Perception: Balancing Excitement with Caution
Market researchers are intrigued by AI's ability to transform their field. The prospect of automating repetitive tasks like data collection and analysis frees up valuable time for strategic thinking. AI-driven tools such as sentiment analysis and predictive analytics offer the promise of uncovering patterns and trends that human analysts might miss. However, skepticism remains regarding AI's reliability, ethical implications, and the potential to replace human intuition in interpreting complex data.
Adoption: Integrating AI into the Research Workflow
Despite reservations, market research agencies are increasingly integrating AI into their operations. Practical applications abound:
Sentiment Analysis: AI algorithms sift through vast amounts of textual data—from social media posts to customer reviews—extracting nuanced sentiments and trends.
Predictive Analytics: By analyzing historical data, AI models forecast market trends, consumer behavior shifts, and product performance with greater accuracy.
Automation: Routine tasks such as data cleaning, report generation, and survey design are streamlined, enhancing efficiency and scalability.
How Qualitative Market Researchers Leverage AI-Native Tools
Qualitative market research, traditionally reliant on human interpretation and empathy, is also being transformed by AI. Here’s how AI-native tools are reshaping qualitative research practices:
Enhanced Data Collection Efficiency
AI streamlines data collection by automating processes such as transcription and sentiment coding. This accelerates the gathering of insights from qualitative methods like interviews, focus groups, and open-ended surveys, enabling researchers to handle larger volumes of data more effectively.
Deep Analysis and Insights Unveiled
Natural Language Processing (NLP) algorithms decode the subtleties of language, revealing underlying sentiments and emerging themes in qualitative data. AI-driven analytics complement human intuition by identifying patterns and connections across large datasets, offering a more comprehensive understanding of consumer perceptions and behaviors.
Practical Use Cases in Qualitative Research
Theme Identification: AI algorithms categorize and prioritize themes within qualitative data, guiding researchers to focus on key findings and actionable insights.
Personalization: During interviews or surveys, AI suggests personalized follow-up questions based on respondents' previous answers, fostering deeper engagement and richer data.
Real-time Feedback: AI-powered surveys provide instant feedback loops, allowing researchers to adapt strategies and refine research methodologies on the fly, ensuring relevance and responsiveness.
Practical Integration Example
Imagine a market research firm launching a new product:
AI-Powered Sentiment Analysis: The firm uses AI to analyze social media conversations and customer reviews about the product. AI identifies positive sentiments towards specific features and uncovers areas for improvement.
Qualitative Insights: Researchers conduct in-depth interviews and focus groups. AI aids in transcribing and analyzing these sessions, pinpointing emerging trends and consumer preferences.
Continuous Improvement: AI-powered surveys collect ongoing feedback post-launch, enabling the firm to iteratively refine the product based on real-time consumer insights.
Practical Challenges in AI Adoption and Integration in Qualitative Market Research
Adopting AI in qualitative market research holds tremendous promise, but it also presents several practical challenges that researchers and agencies must navigate effectively. These challenges stem from technological limitations, ethical considerations, and the need to balance automation with human expertise.
1. Data Privacy and Ethical Concerns
Challenge: AI tools rely on vast amounts of data, often including sensitive information from interviews, focus groups, and social media. Ensuring participant privacy, obtaining informed consent, and safeguarding data integrity are critical ethical considerations.
AI Solution: AI-native tools incorporate robust data anonymization techniques and encryption protocols to protect participant identities. Automated data anonymization algorithms ensure that personally identifiable information (PII) is masked or removed, preserving confidentiality while allowing for meaningful analysis.
2. Algorithmic Bias and Interpretation
Challenge: AI algorithms can inherit biases from training data, potentially leading to skewed results or reinforcing existing stereotypes. Ensuring fairness and transparency in algorithmic decision-making is essential to maintain credibility in research outcomes.
AI Solution: AI-native tools employ bias detection algorithms and fairness metrics during model development and deployment. Techniques such as algorithmic auditing and diverse training datasets help mitigate biases by promoting balanced representations and reducing unintended discrimination.
3. Integration with Human Expertise
Challenge: Qualitative research often requires nuanced interpretation and contextual understanding that AI may struggle to replicate. Balancing the automation of repetitive tasks with the expertise of human researchers is crucial for generating actionable insights.
AI Solution: AI-native tools augment human expertise by automating time-consuming tasks like transcription, sentiment analysis, and theme identification. Natural Language Processing (NLP) algorithms enhance data interpretation by detecting subtle linguistic cues and sentiment nuances, enabling researchers to focus on higher-level analysis and strategic decision-making.
4. Complexity of Qualitative Data Analysis
Challenge: Qualitative data is inherently complex, characterized by unstructured formats, diverse linguistic styles, and subjective interpretations. Extracting meaningful insights requires sophisticated analytical tools capable of handling variability and ambiguity.
AI Solution: AI-powered analytics platforms leverage advanced machine learning algorithms to process and categorize qualitative data efficiently. Text mining techniques, topic modeling, and sentiment analysis algorithms streamline data interpretation, revealing patterns and trends that might otherwise go unnoticed. Interactive visualization tools further enhance researchers' ability to explore and communicate findings effectively.
5. Cost and Resource Allocation
Challenge: Implementing AI technologies involves initial investment in infrastructure, software licenses, and training. Smaller agencies or research teams with limited budgets may face barriers to adopting AI-driven solutions effectively.
AI Solution: AI-native tools offer scalable solutions tailored to diverse budgetary constraints. Cloud-based platforms and subscription models reduce upfront costs and provide flexibility in resource allocation. Moreover, AI's efficiency in automating repetitive tasks minimizes operational expenses over time, allowing agencies to reallocate resources towards strategic initiatives and client engagement.
Conclusion: Embracing Innovation while Navigating Challenges
Qualitative market researchers are at a pivotal moment, embracing AI's transformative potential while navigating its complexities. As AI-native tools continue to evolve, researchers must remain vigilant about ethical considerations, data privacy safeguards, and the symbiotic relationship between human judgment and machine intelligence.
The integration of AI into qualitative market research represents a paradigm shift, empowering researchers to glean deeper insights and enhance decision-making capabilities. It’s critical to address practical challenges through ethical frameworks, algorithmic transparency, and synergistic collaboration between AI and human expertise.
Market research agencies leverage AI-native tools like Metaforms.ai to unlock new dimensions of understanding in consumer behavior and market dynamics. Embracing innovation while mitigating risks ensures that AI adoption in qualitative research is not just a technological advancement but a strategic imperative for staying competitive in a rapidly evolving industry landscape. Sign-up for a demo today!
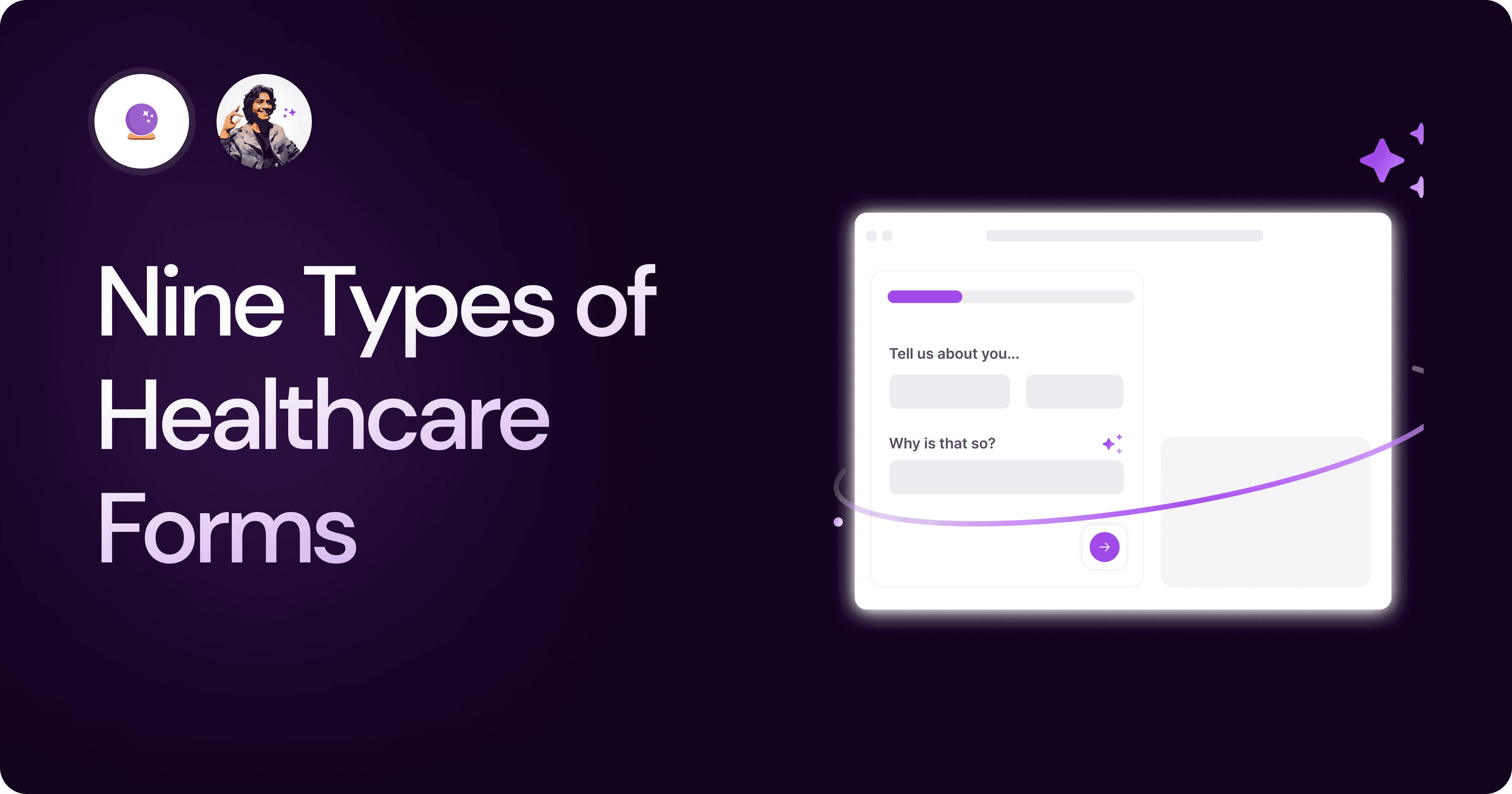
Nine Types of Healthcare and Medical Forms.
Medical forms are a must-have for any healthcare business or practitioner. Learn about the different kinds of medical and healthcare forms.
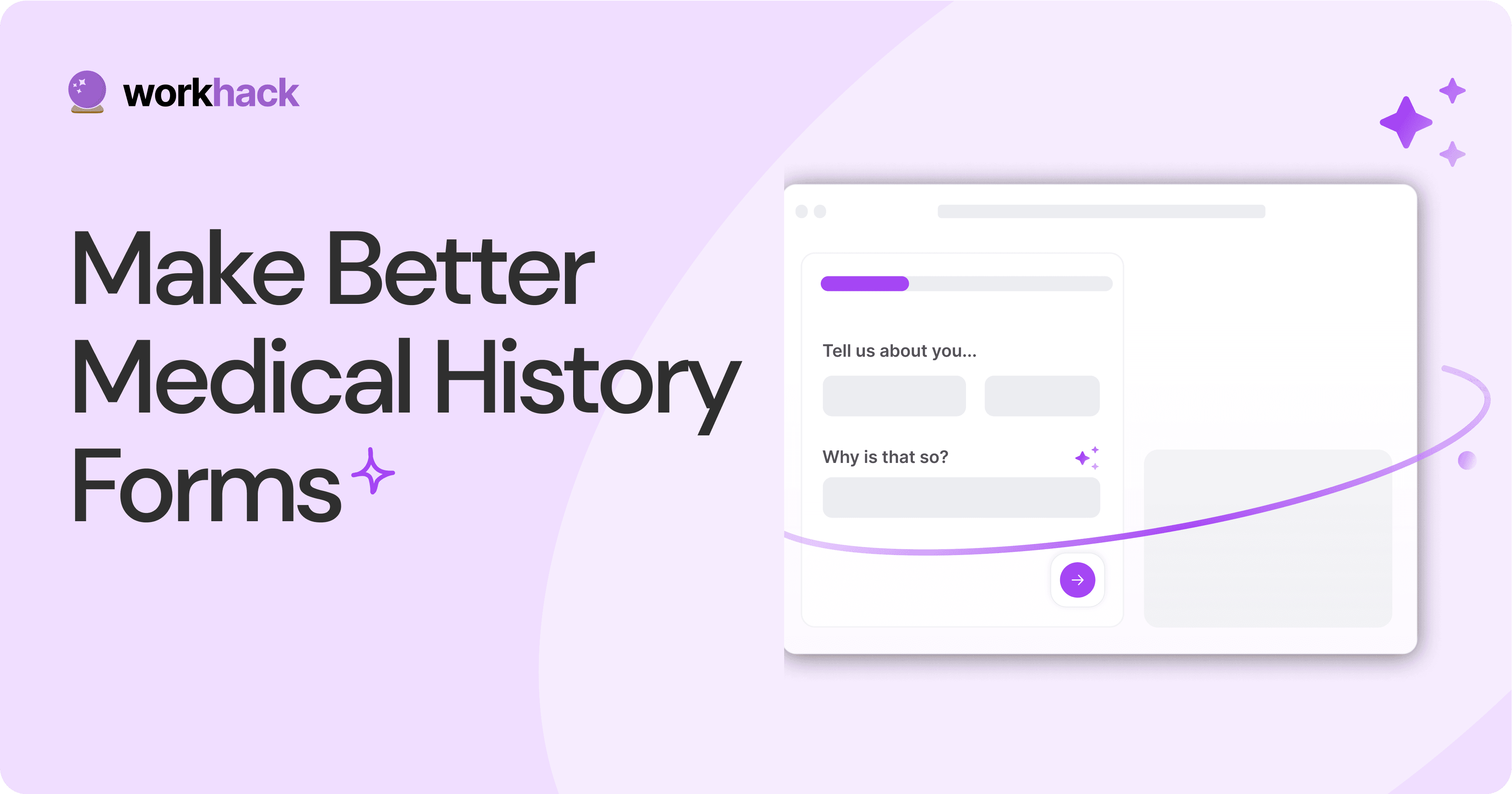
4 Tips for Better Medical History Forms.
Medical history forms are central to patient care, onboarding, and medical administration records. Learn how to make them easier to fill.
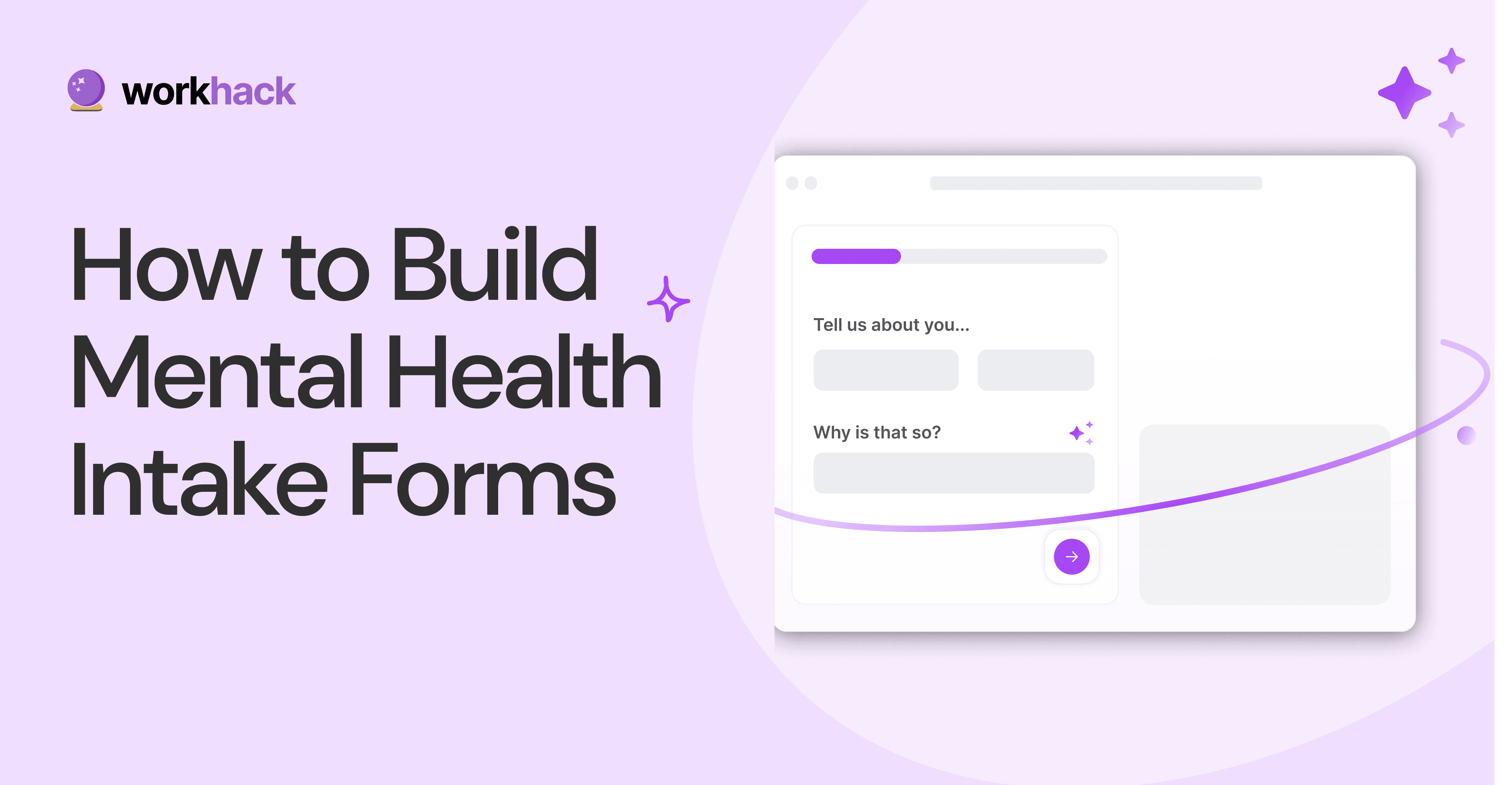
How to Build Mental Health Intake Forms?
Mental health intake forms are not like patient intake forms. Mental health intake forms deal with far more sensitive data and have specific design methods.
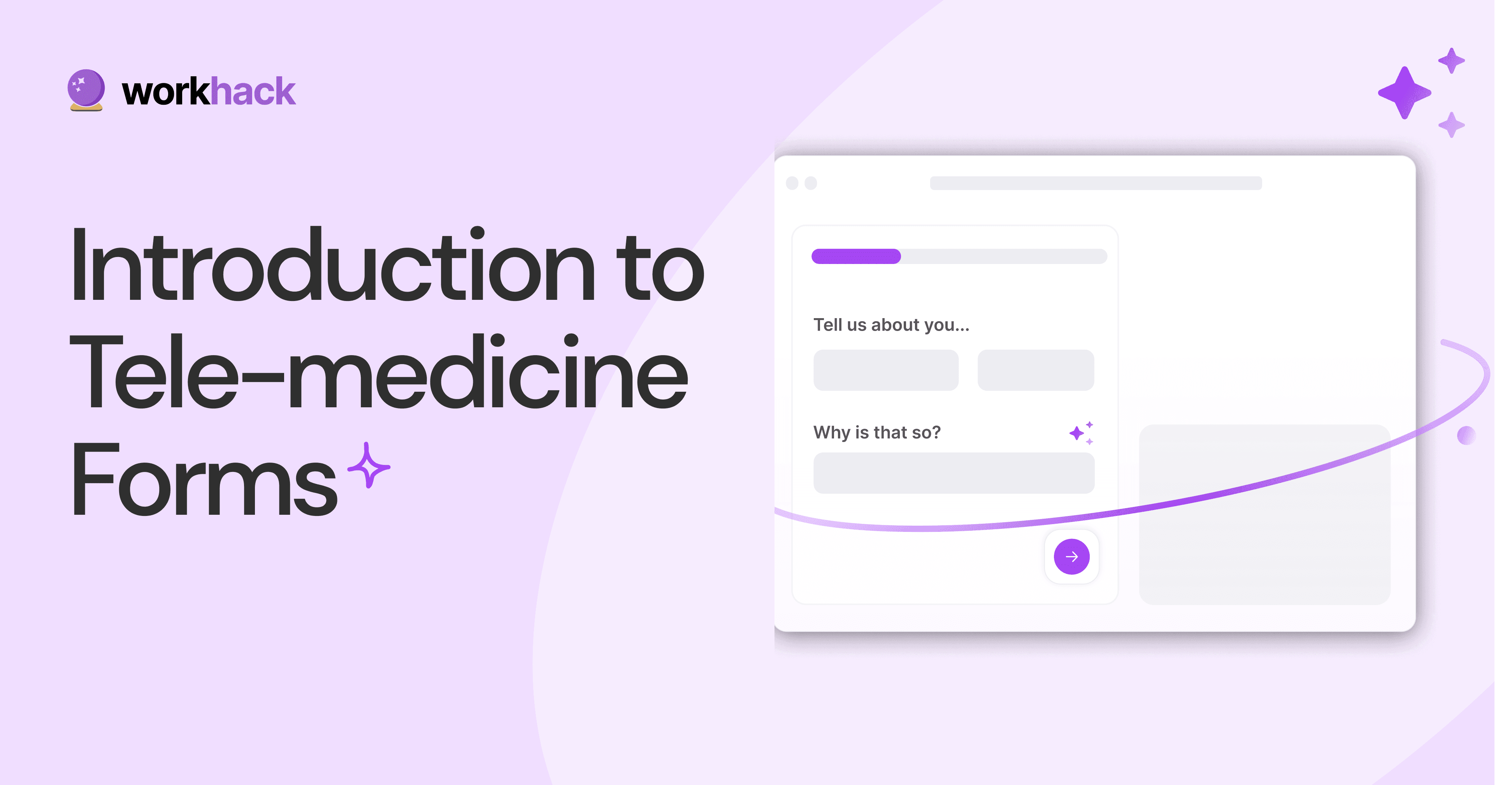
What, Why and How of Telemedicine Forms.
Telemedicine is on the rise and with different form builders out there, which one best suits your needs as a healthcare services provider?
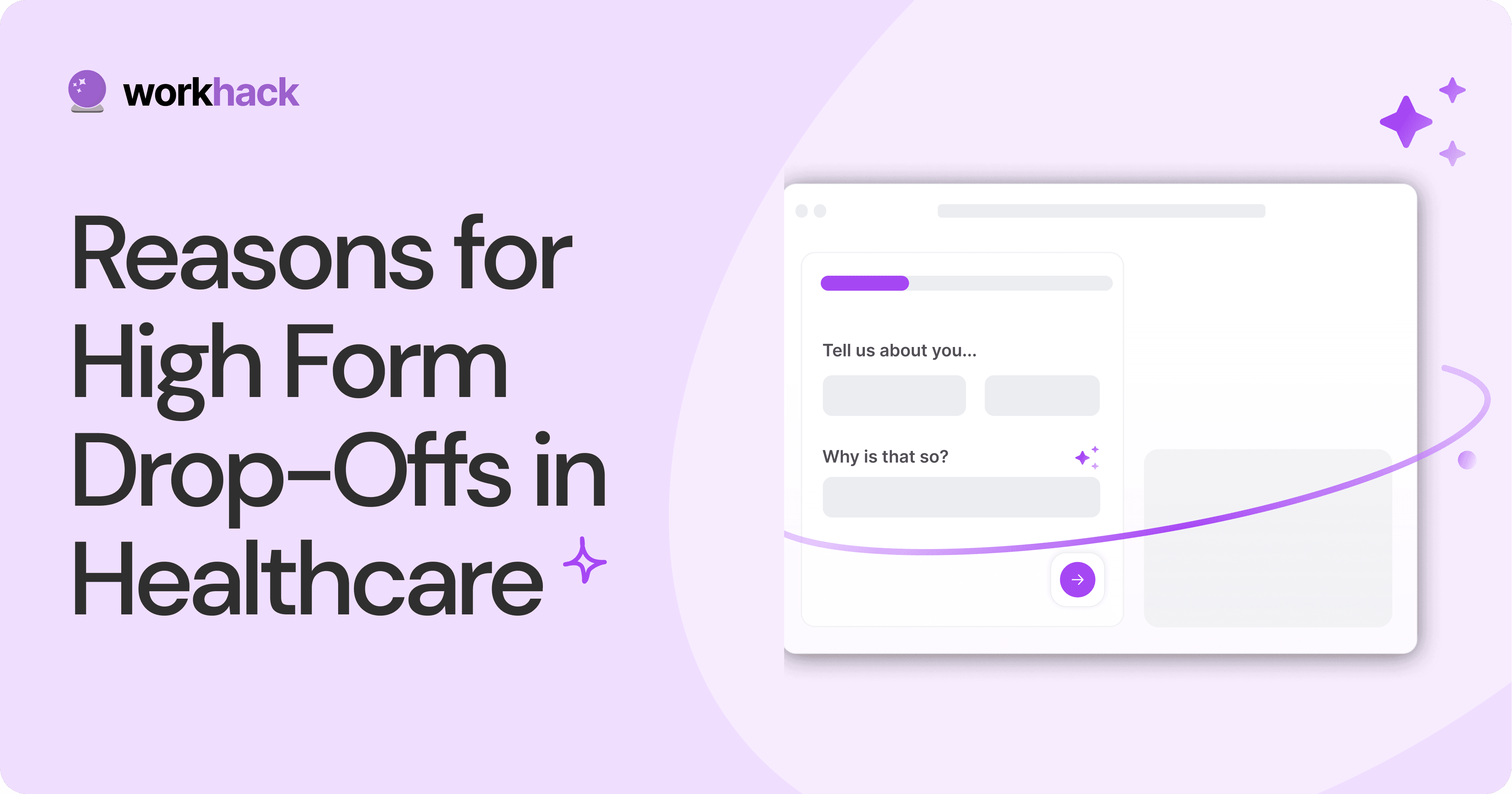
3 Reasons for Major Drop-Offs in Medical Forms.
No matter which healthcare form we pick, there are major drop-off reasons. We shall dive into the top 3 and learn how to resolve them in your next form.
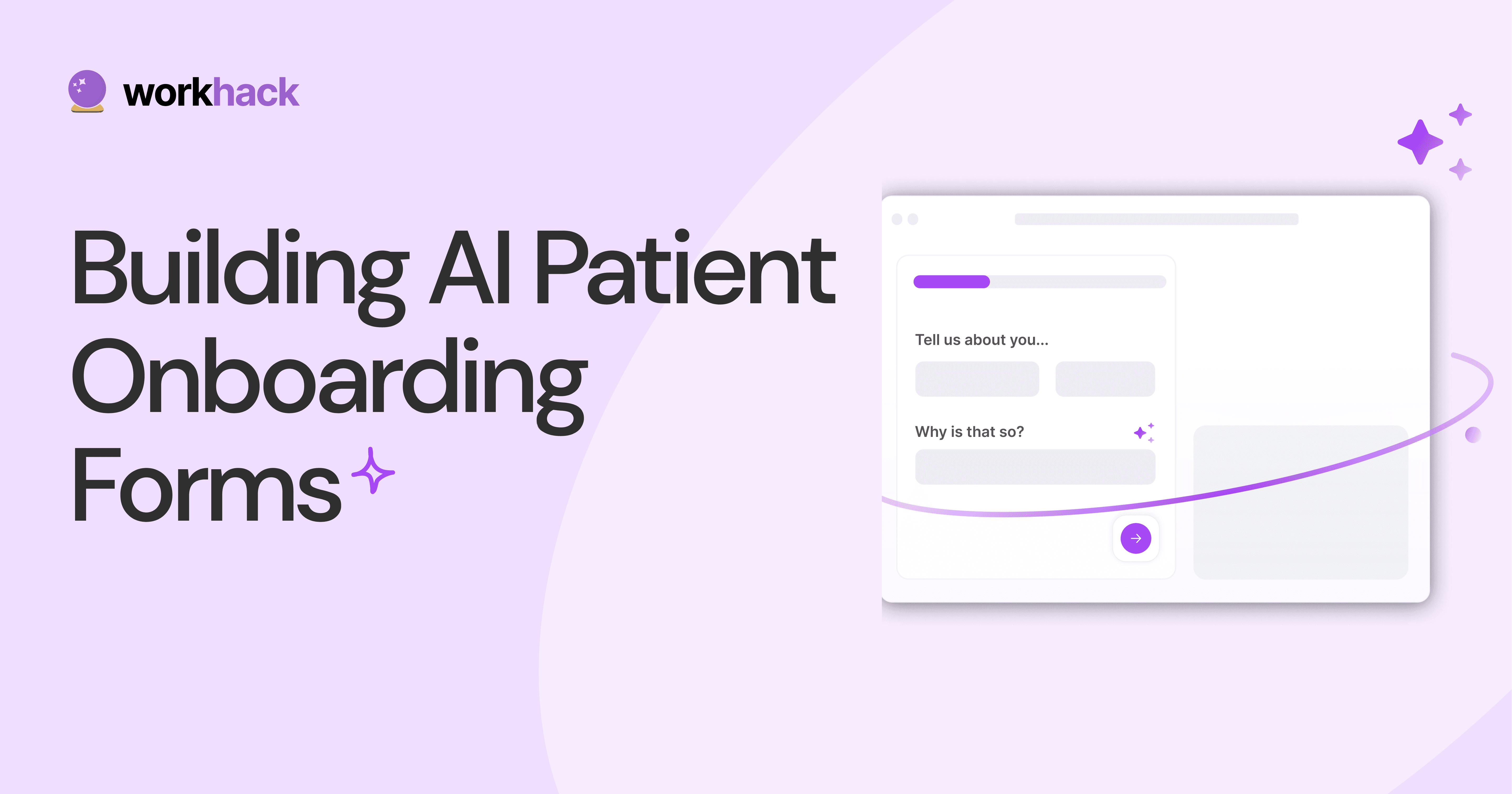
Patient Onboarding Forms - From Click to Clinic.
Patient onboarding forms are the first touchpoint for patients; getting this right for higher conversion rates is a must-have. Learn how to perfect them now.

5 Key Parts of a Good Patient Satisfaction Form.
The goal of patient satisfaction surveys is to course-correct the services of a healthcare provider. Patient feedback leads to a culture of patient-centric care.
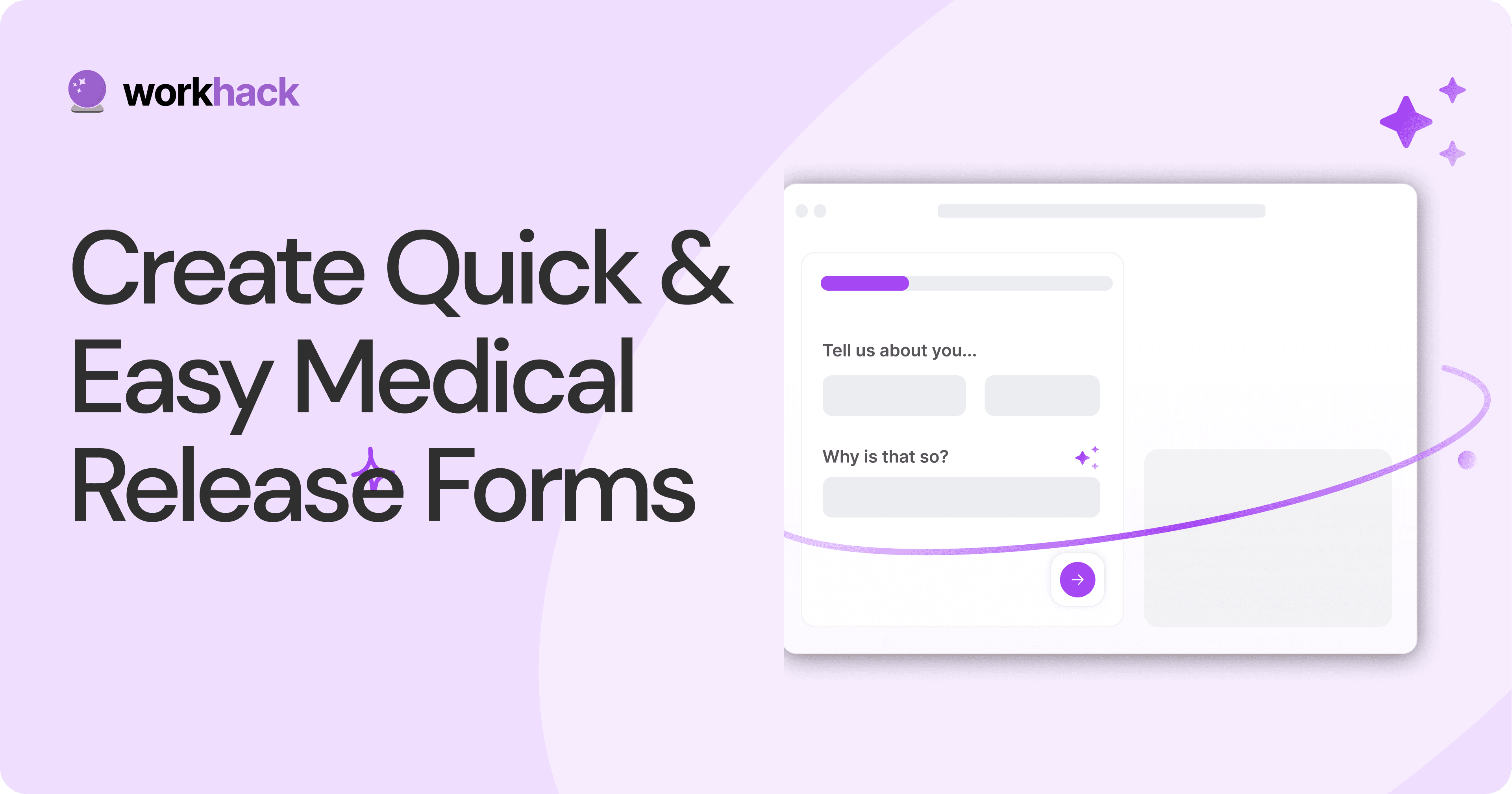
Build Quick and Easy Medical Release Forms.
Every HIPAA-compliant healthcare provider comes across medical release forms that involve details from medical history forms. Can they be shipped fast? Yes.
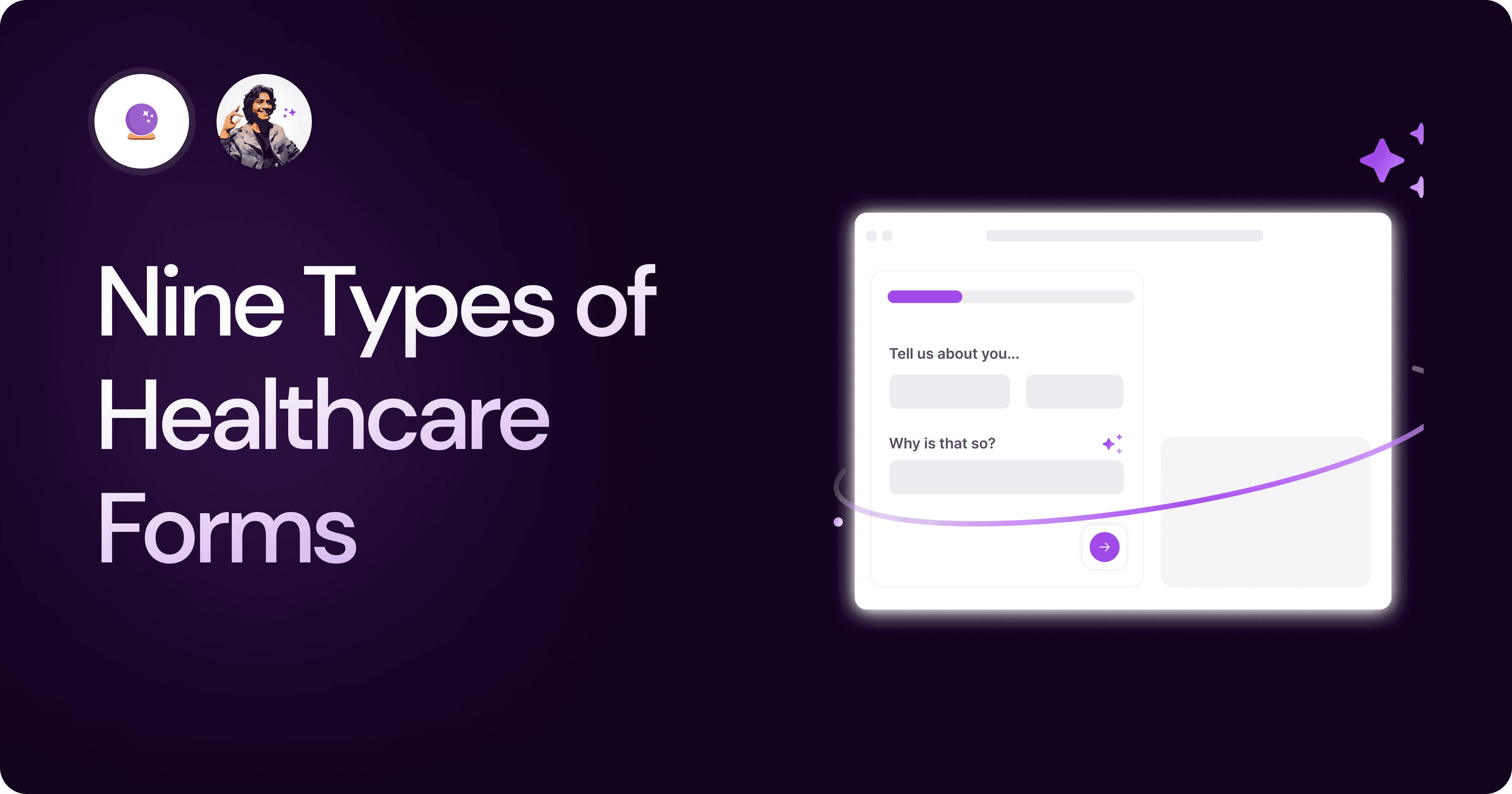
Nine Types of Healthcare and Medical Forms.
Medical forms are a must-have for any healthcare business or practitioner. Learn about the different kinds of medical and healthcare forms.
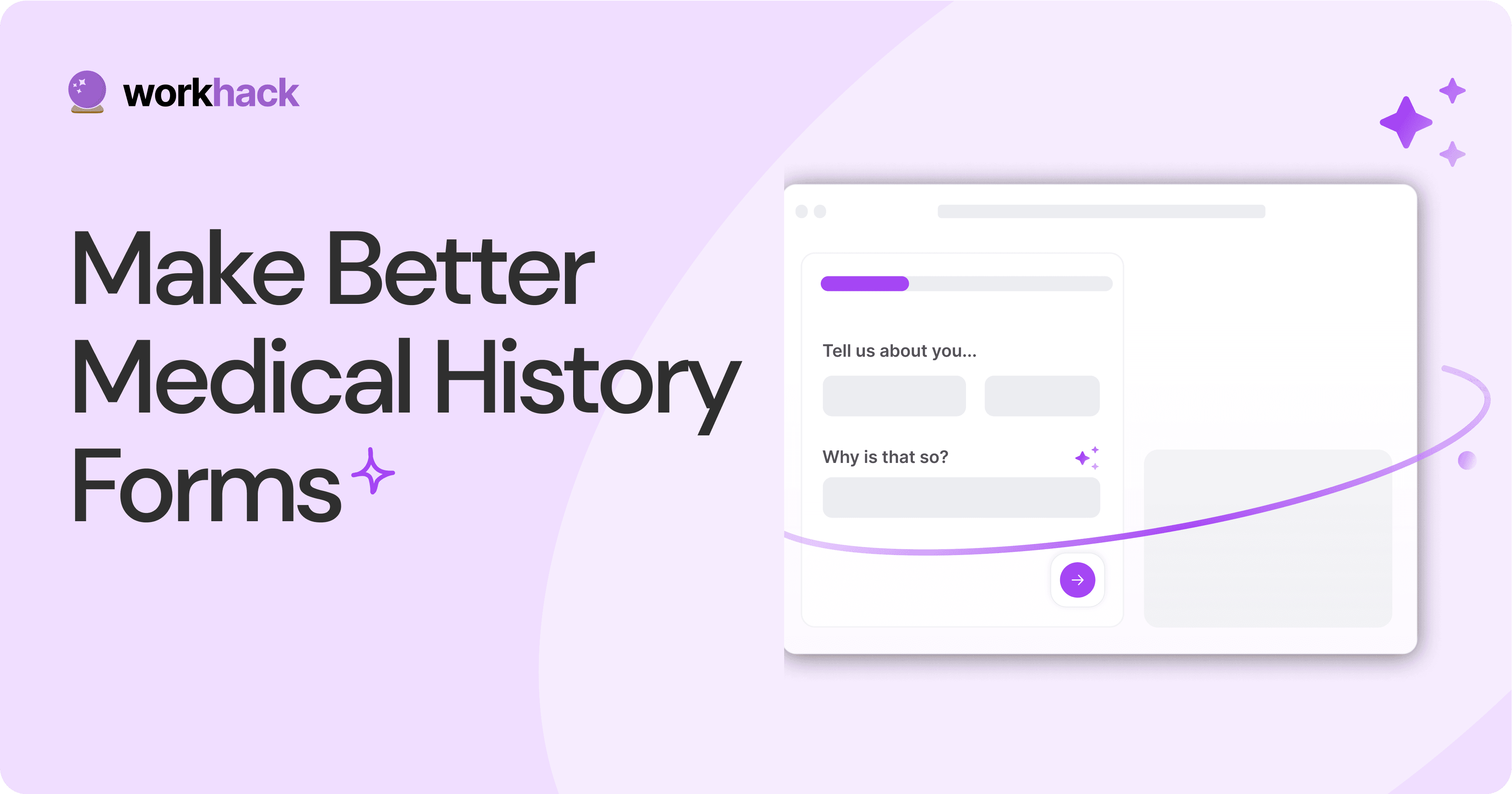
4 Tips for Better Medical History Forms.
Medical history forms are central to patient care, onboarding, and medical administration records. Learn how to make them easier to fill.
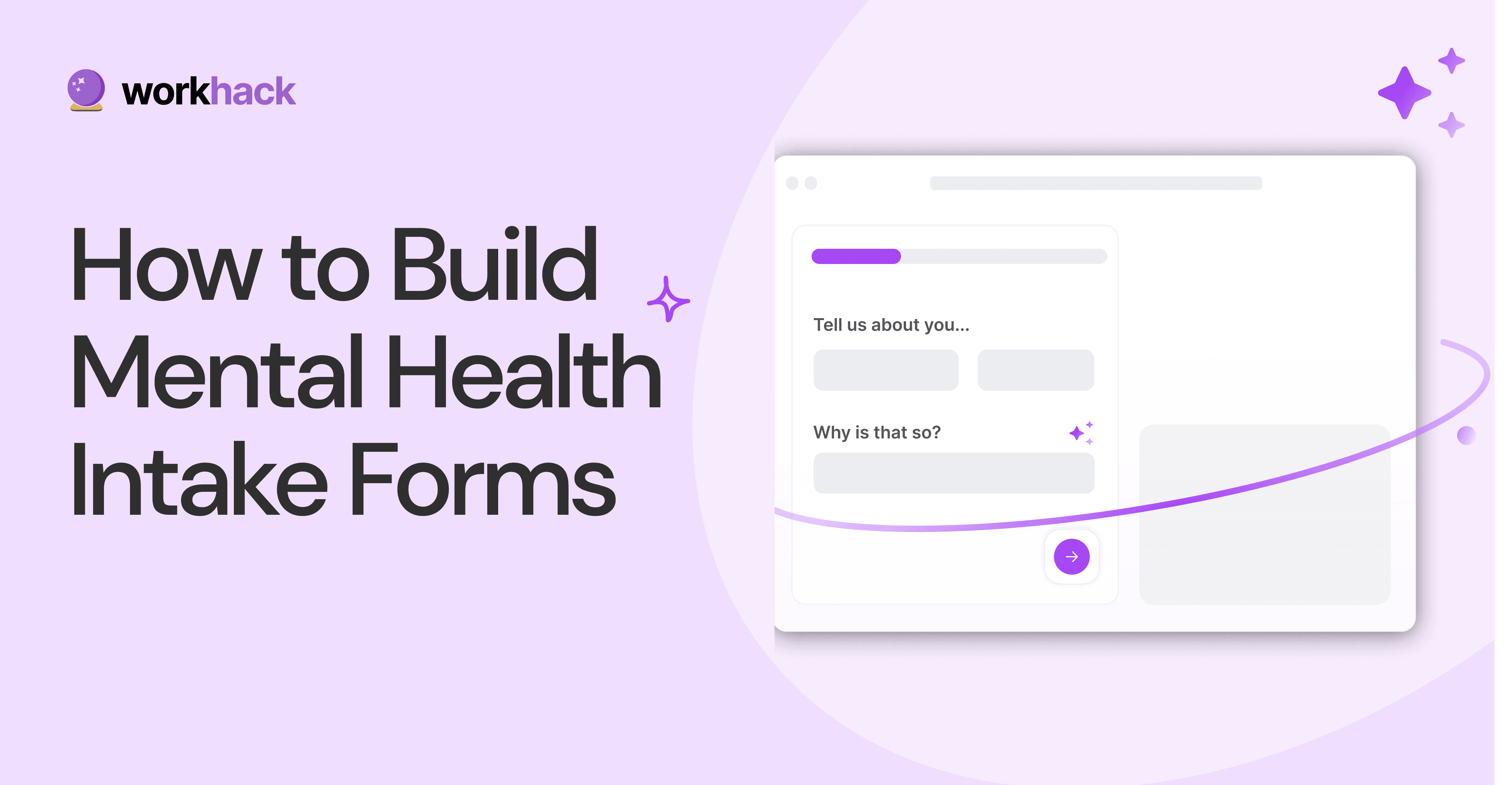
How to Build Mental Health Intake Forms?
Mental health intake forms are not like patient intake forms. Mental health intake forms deal with far more sensitive data and have specific design methods.
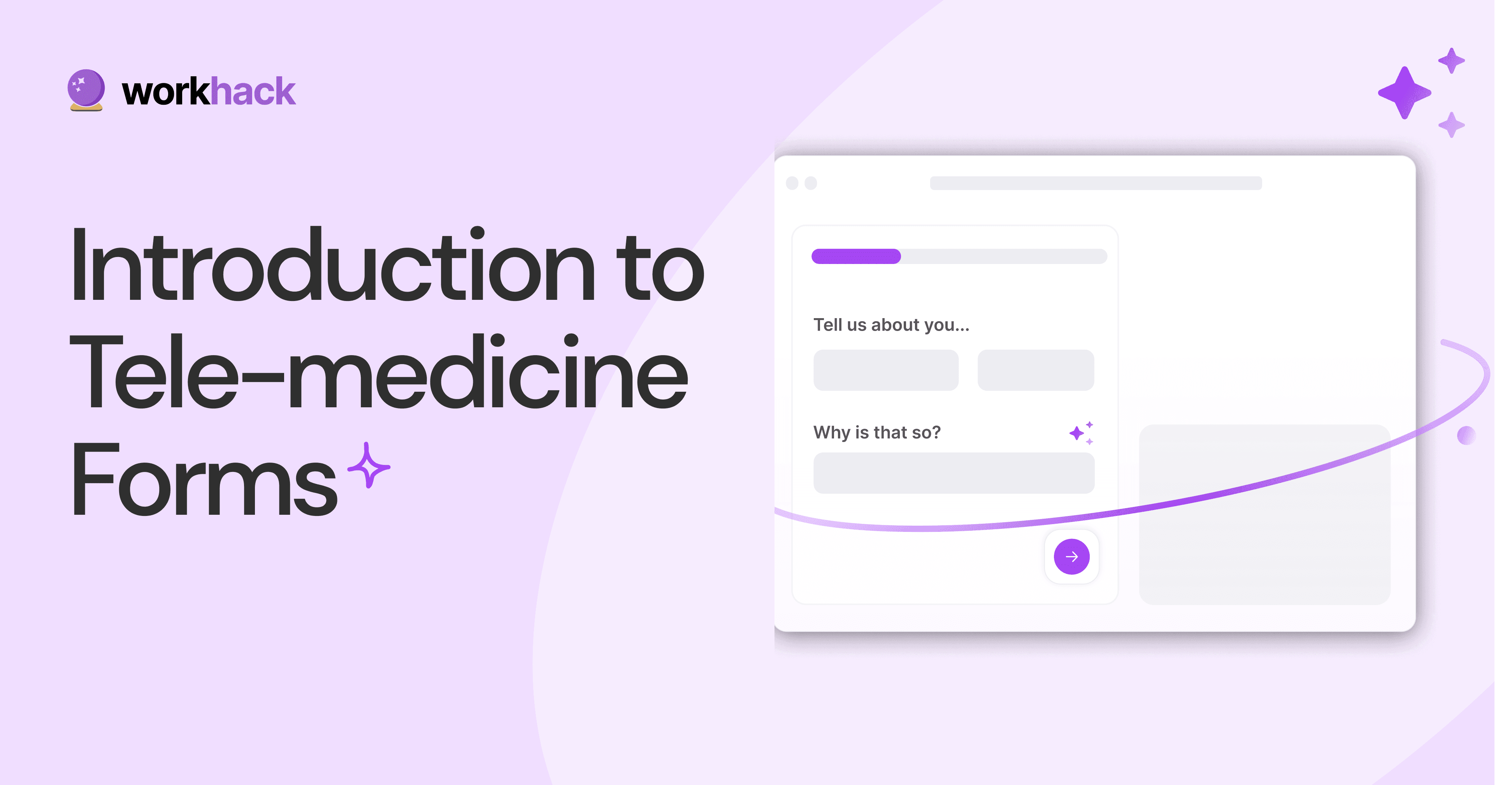
What, Why and How of Telemedicine Forms.
Telemedicine is on the rise and with different form builders out there, which one best suits your needs as a healthcare services provider?
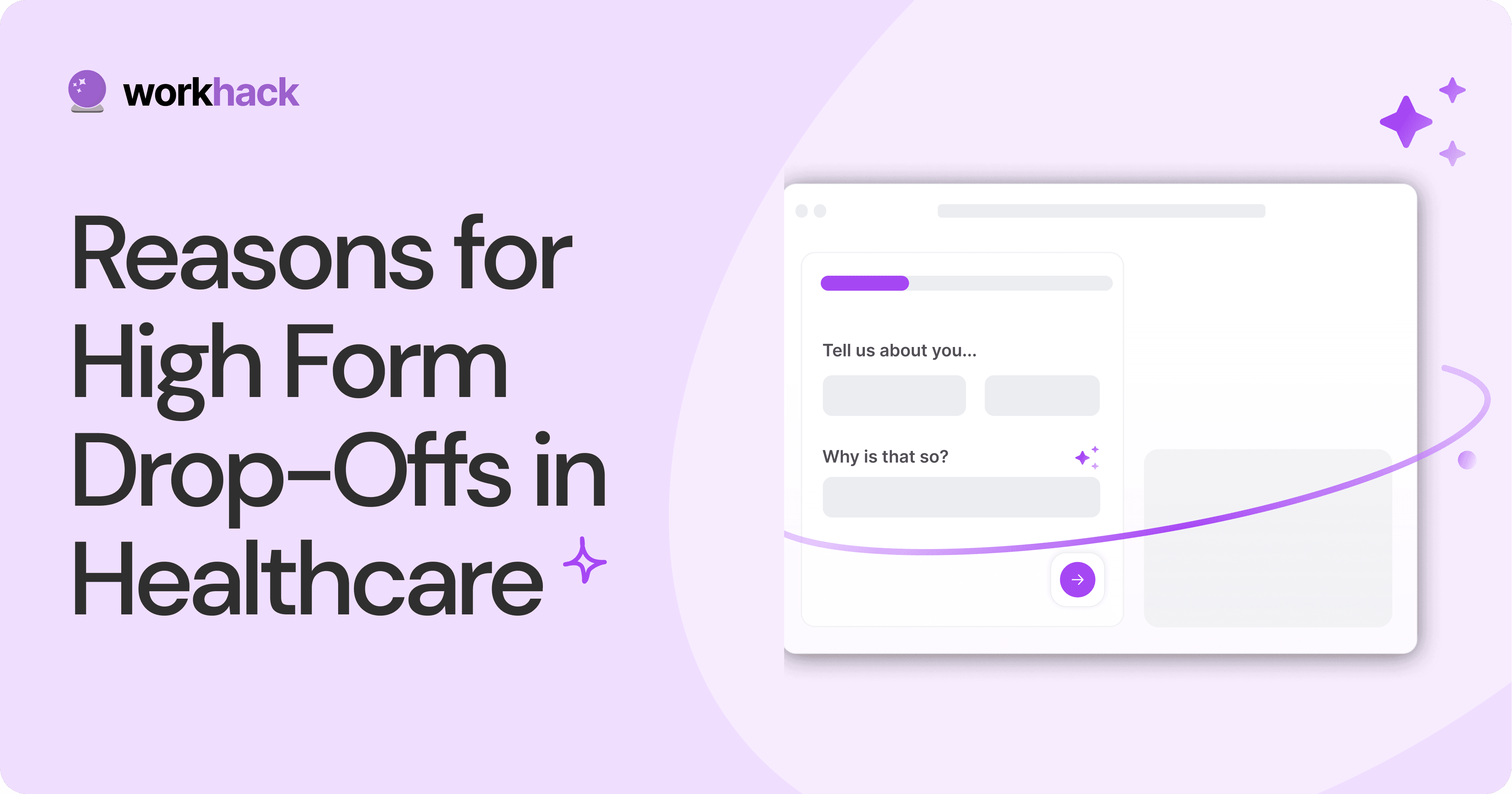
3 Reasons for Major Drop-Offs in Medical Forms.
No matter which healthcare form we pick, there are major drop-off reasons. We shall dive into the top 3 and learn how to resolve them in your next form.
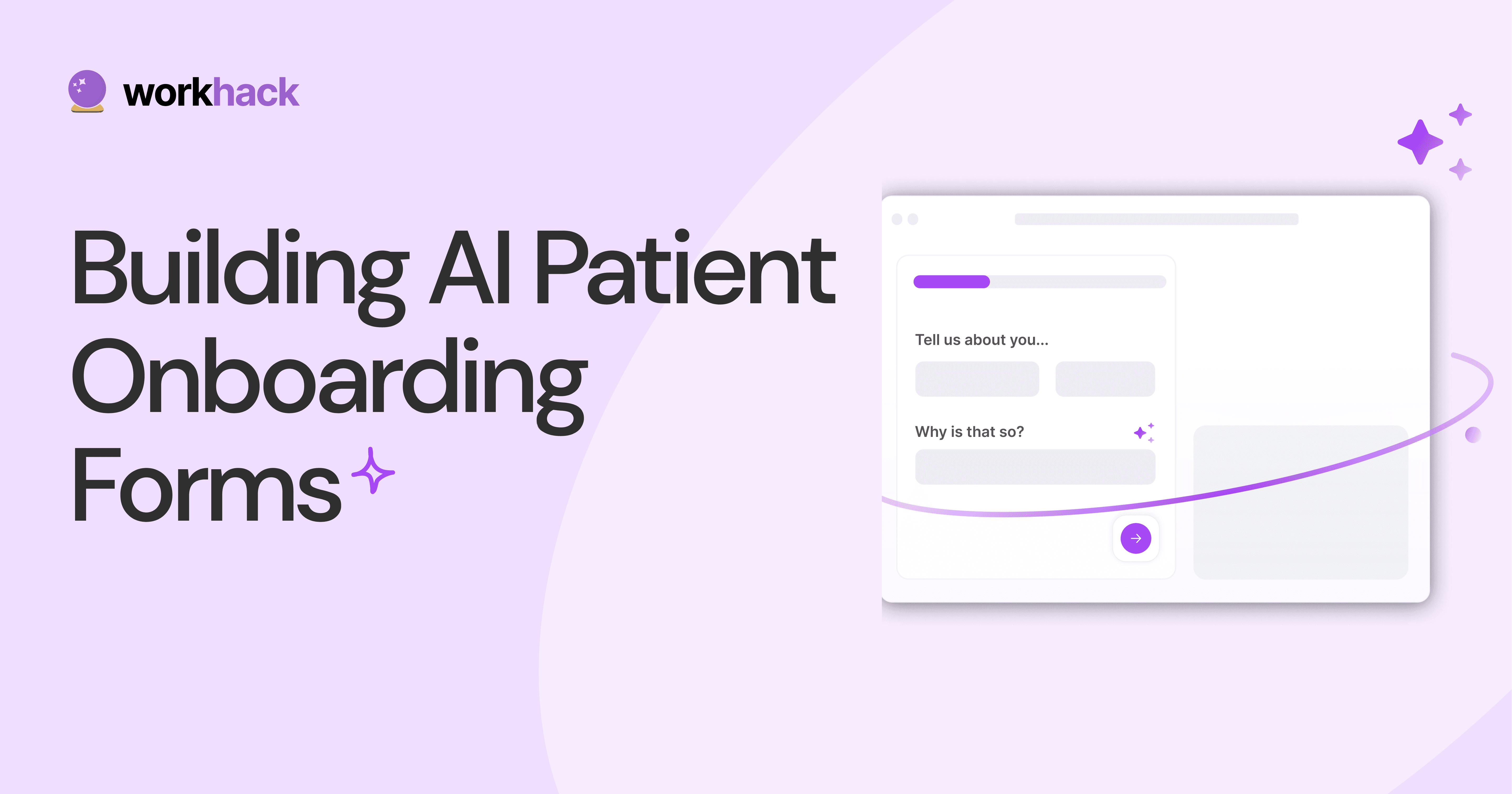
Patient Onboarding Forms - From Click to Clinic.
Patient onboarding forms are the first touchpoint for patients; getting this right for higher conversion rates is a must-have. Learn how to perfect them now.

5 Key Parts of a Good Patient Satisfaction Form.
The goal of patient satisfaction surveys is to course-correct the services of a healthcare provider. Patient feedback leads to a culture of patient-centric care.
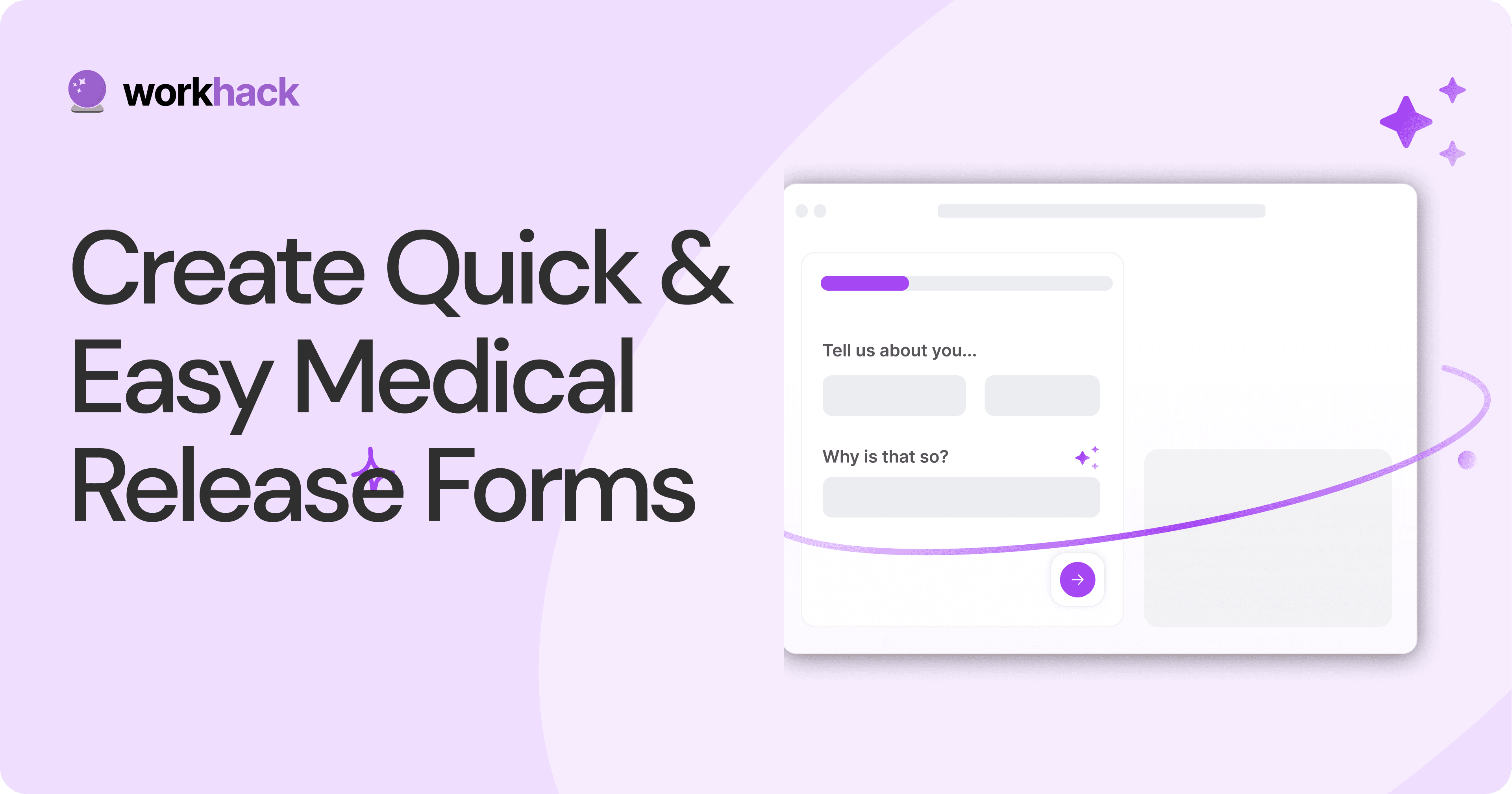
Build Quick and Easy Medical Release Forms.
Every HIPAA-compliant healthcare provider comes across medical release forms that involve details from medical history forms. Can they be shipped fast? Yes.
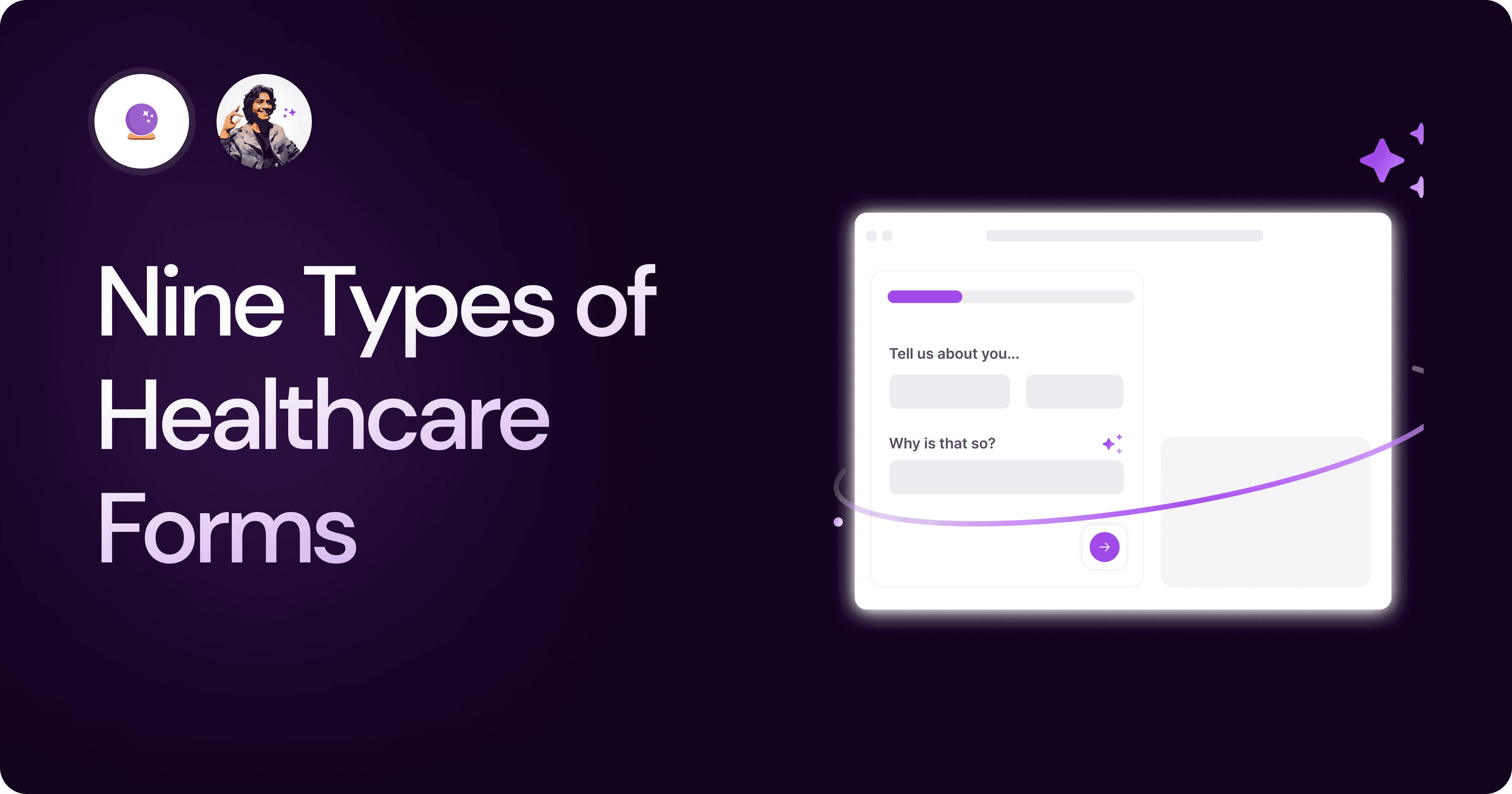
Nine Types of Healthcare and Medical Forms.
Medical forms are a must-have for any healthcare business or practitioner. Learn about the different kinds of medical and healthcare forms.
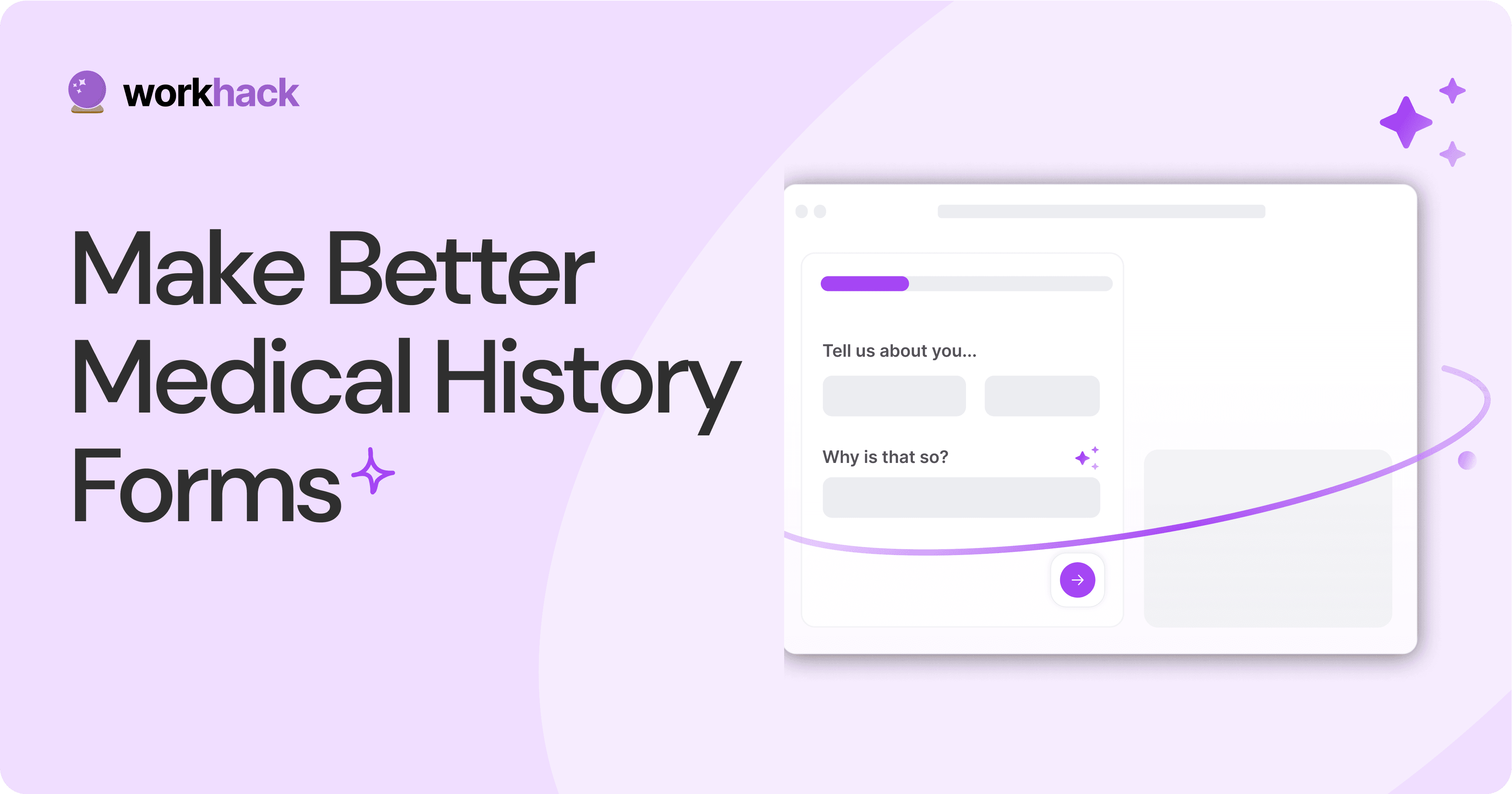
4 Tips for Better Medical History Forms.
Medical history forms are central to patient care, onboarding, and medical administration records. Learn how to make them easier to fill.
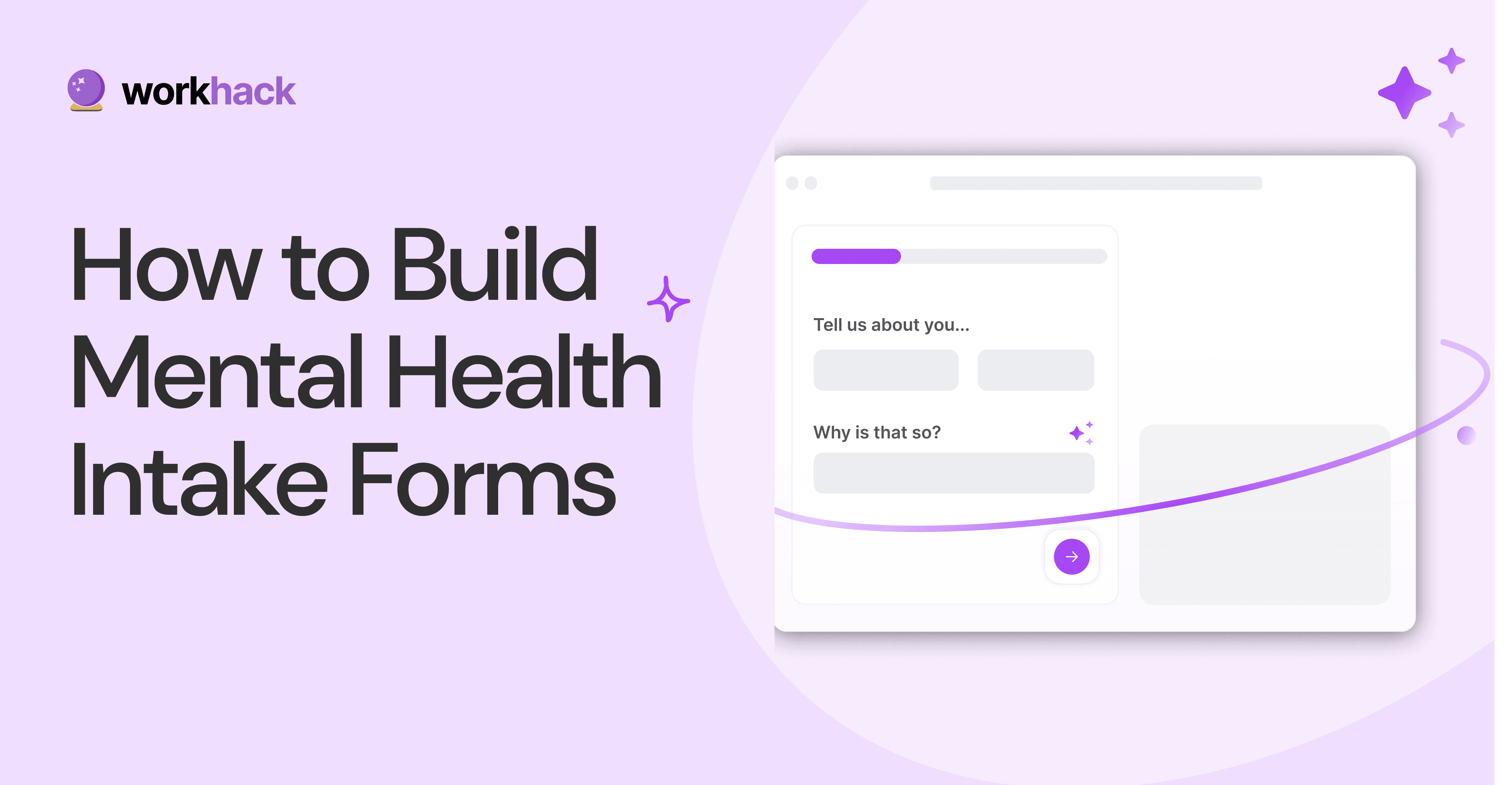
How to Build Mental Health Intake Forms?
Mental health intake forms are not like patient intake forms. Mental health intake forms deal with far more sensitive data and have specific design methods.
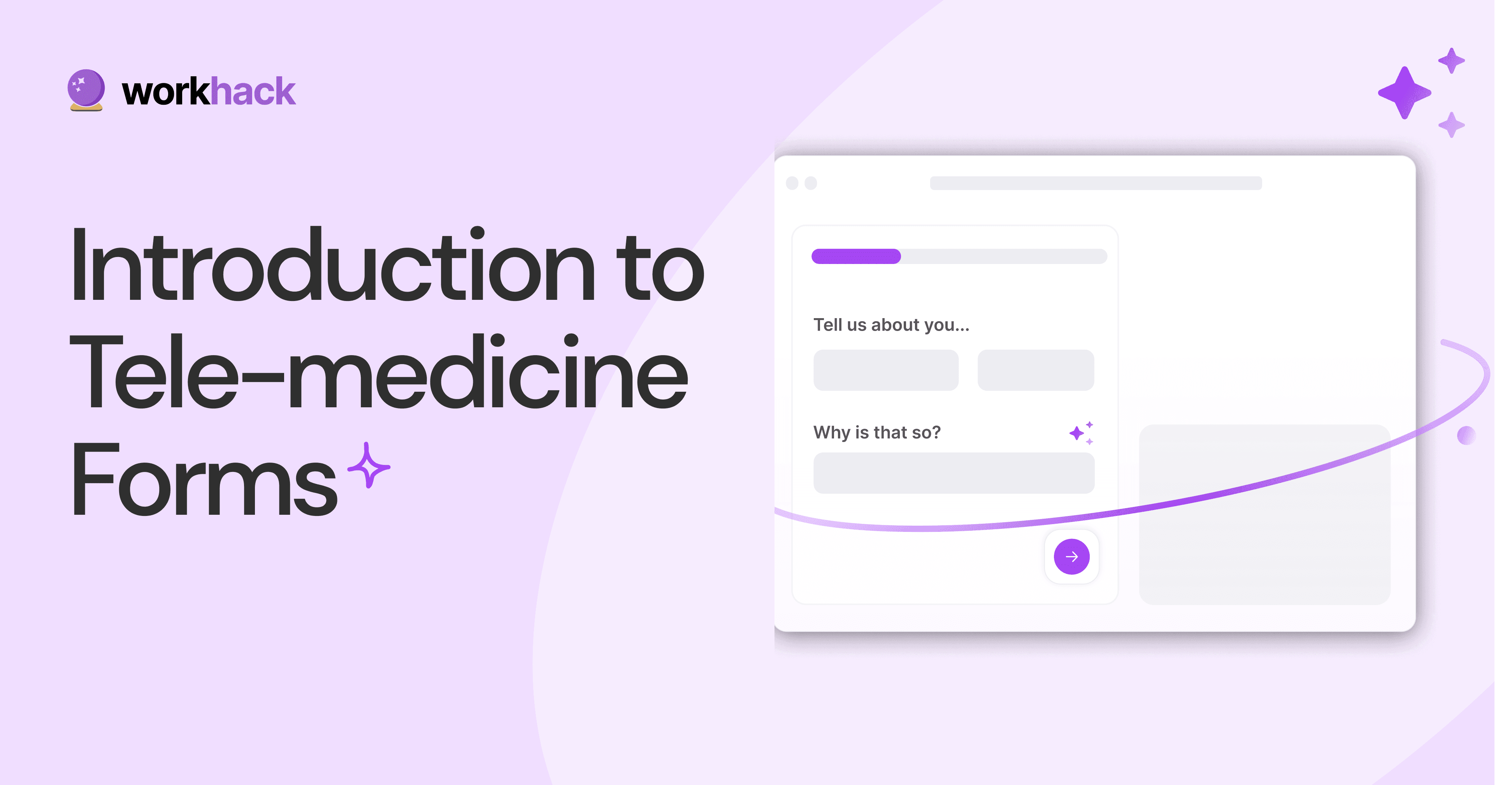
What, Why and How of Telemedicine Forms.
Telemedicine is on the rise and with different form builders out there, which one best suits your needs as a healthcare services provider?
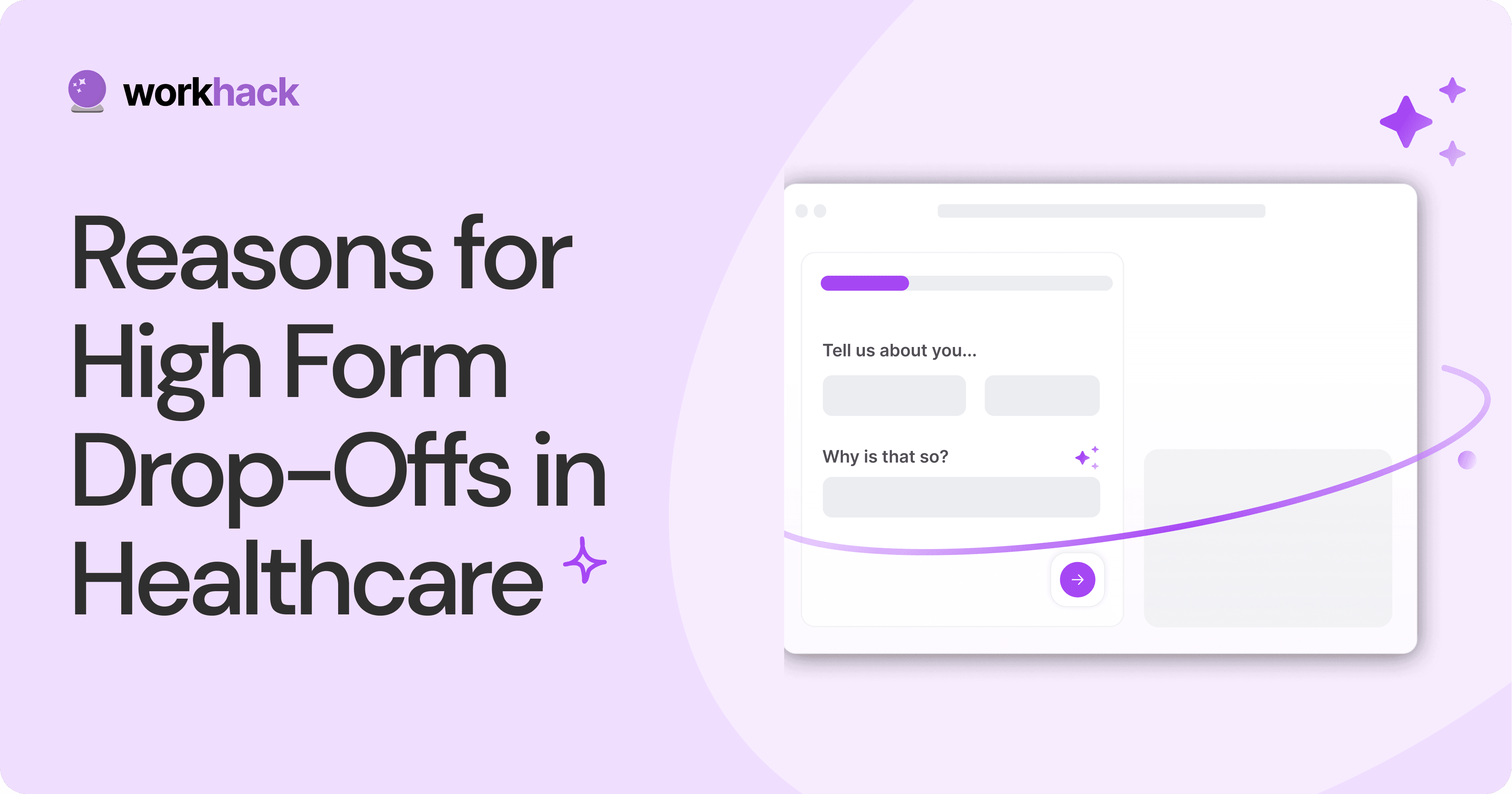
3 Reasons for Major Drop-Offs in Medical Forms.
No matter which healthcare form we pick, there are major drop-off reasons. We shall dive into the top 3 and learn how to resolve them in your next form.
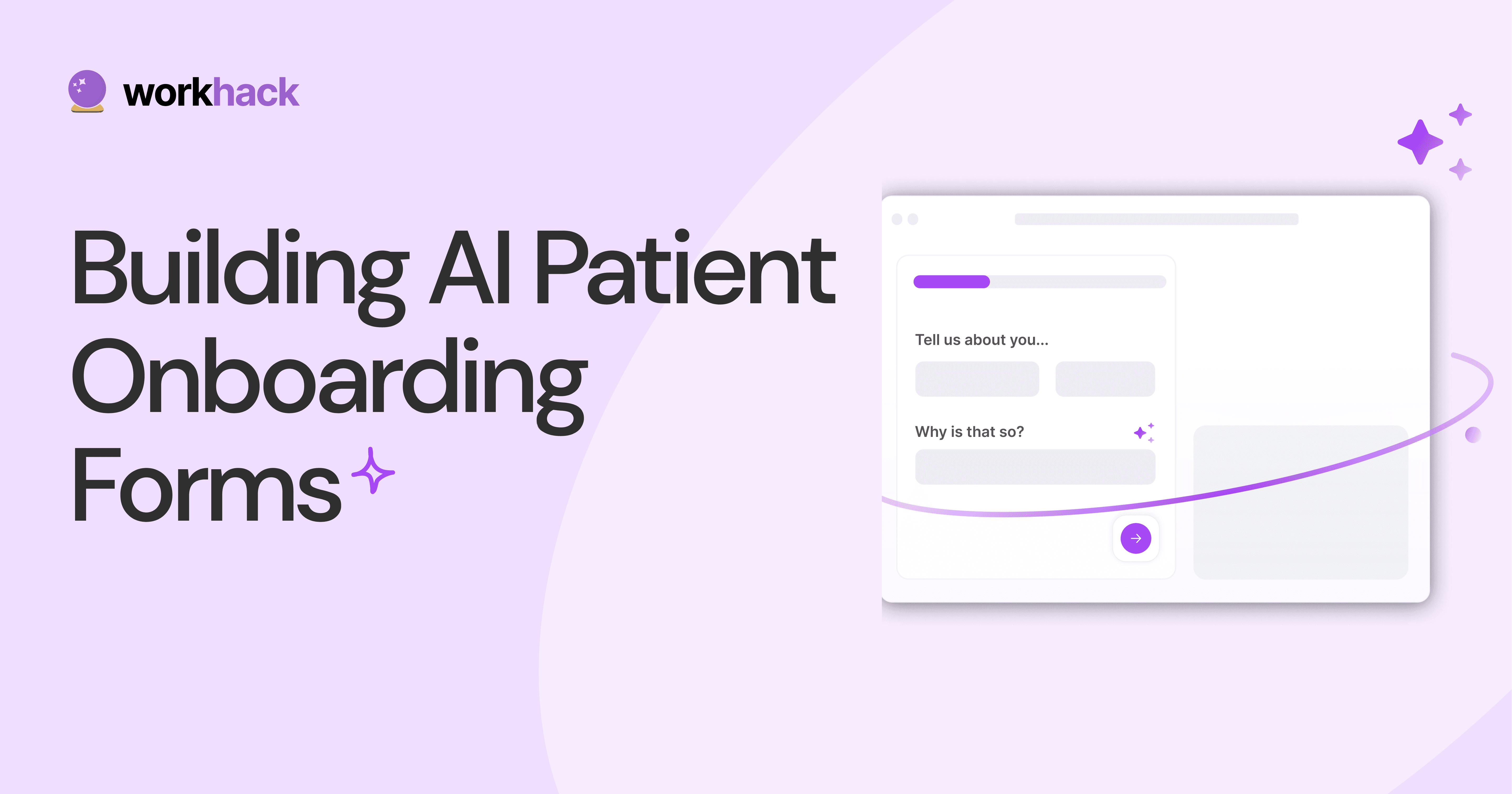
Patient Onboarding Forms - From Click to Clinic.
Patient onboarding forms are the first touchpoint for patients; getting this right for higher conversion rates is a must-have. Learn how to perfect them now.

5 Key Parts of a Good Patient Satisfaction Form.
The goal of patient satisfaction surveys is to course-correct the services of a healthcare provider. Patient feedback leads to a culture of patient-centric care.
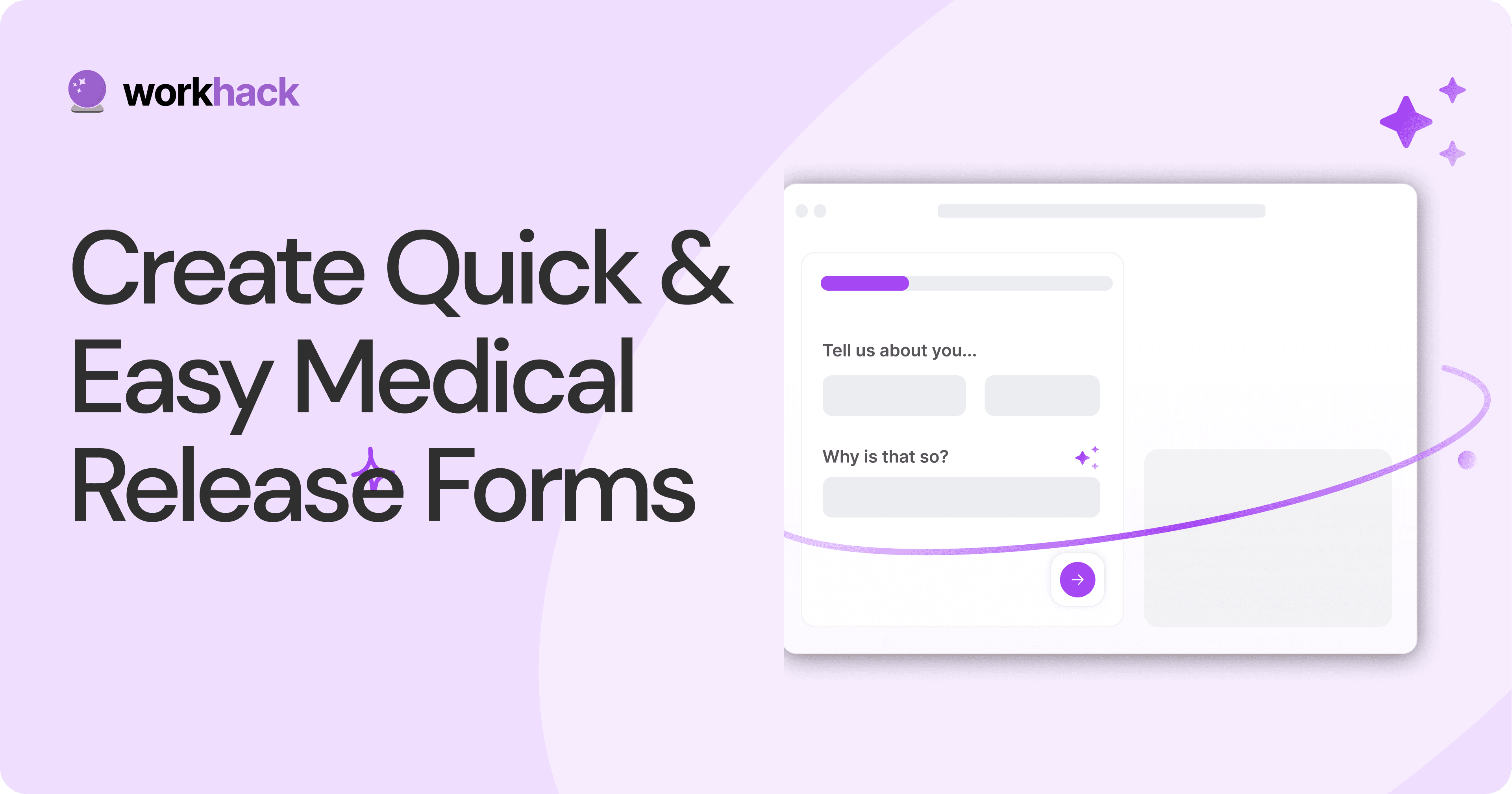
Build Quick and Easy Medical Release Forms.
Every HIPAA-compliant healthcare provider comes across medical release forms that involve details from medical history forms. Can they be shipped fast? Yes.
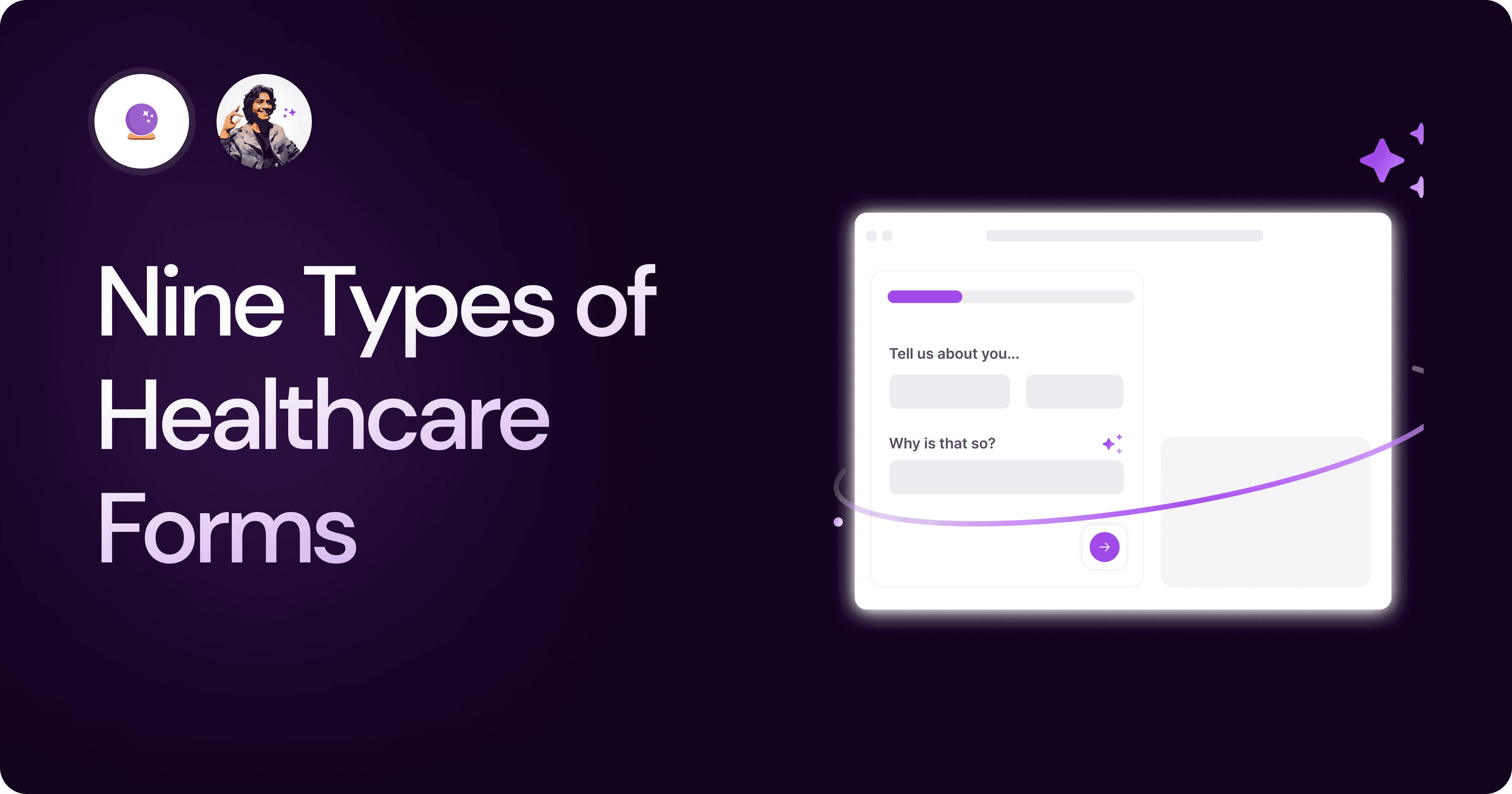
Nine Types of Healthcare and Medical Forms.
Medical forms are a must-have for any healthcare business or practitioner. Learn about the different kinds of medical and healthcare forms.
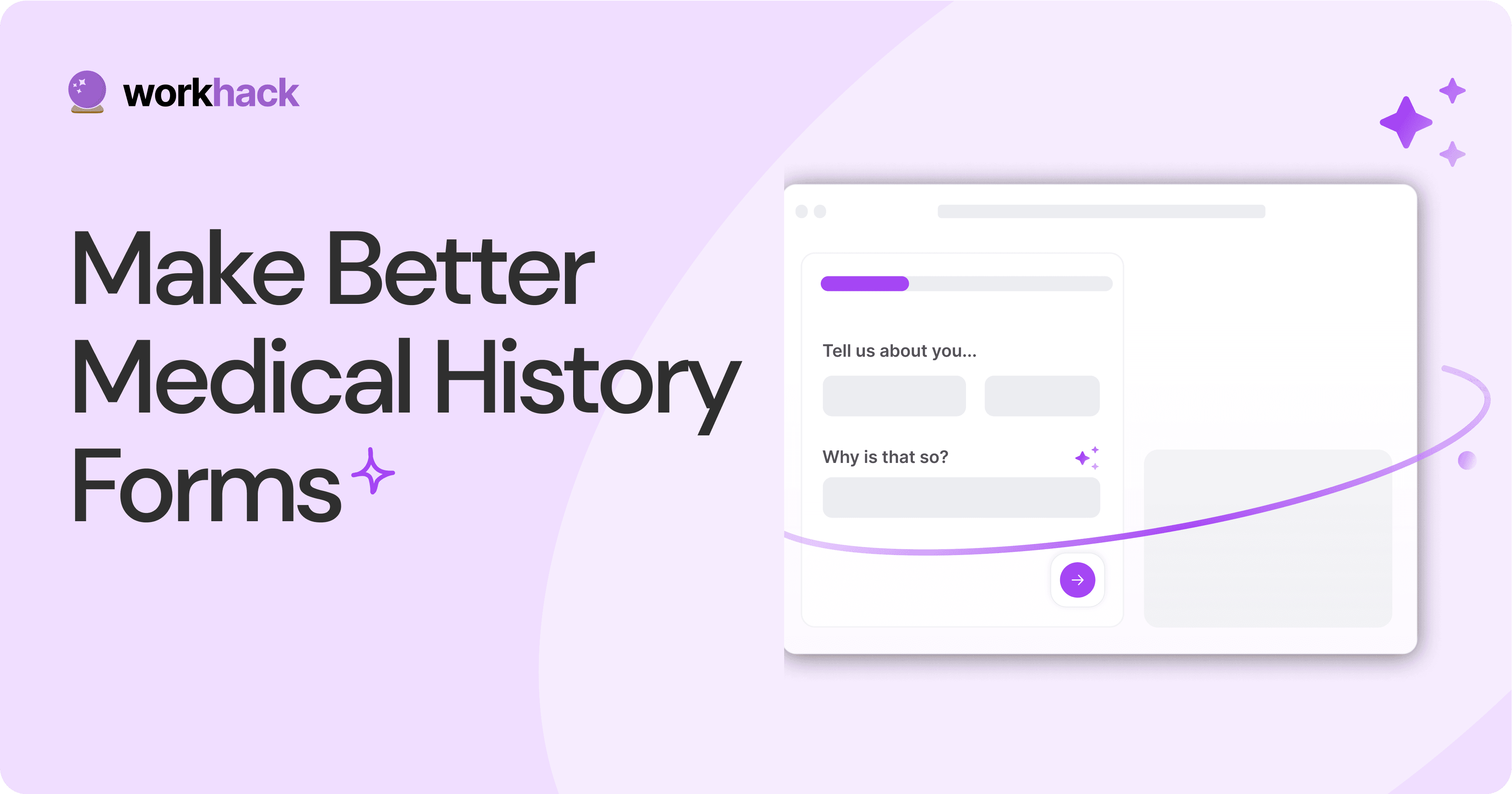
4 Tips for Better Medical History Forms.
Medical history forms are central to patient care, onboarding, and medical administration records. Learn how to make them easier to fill.
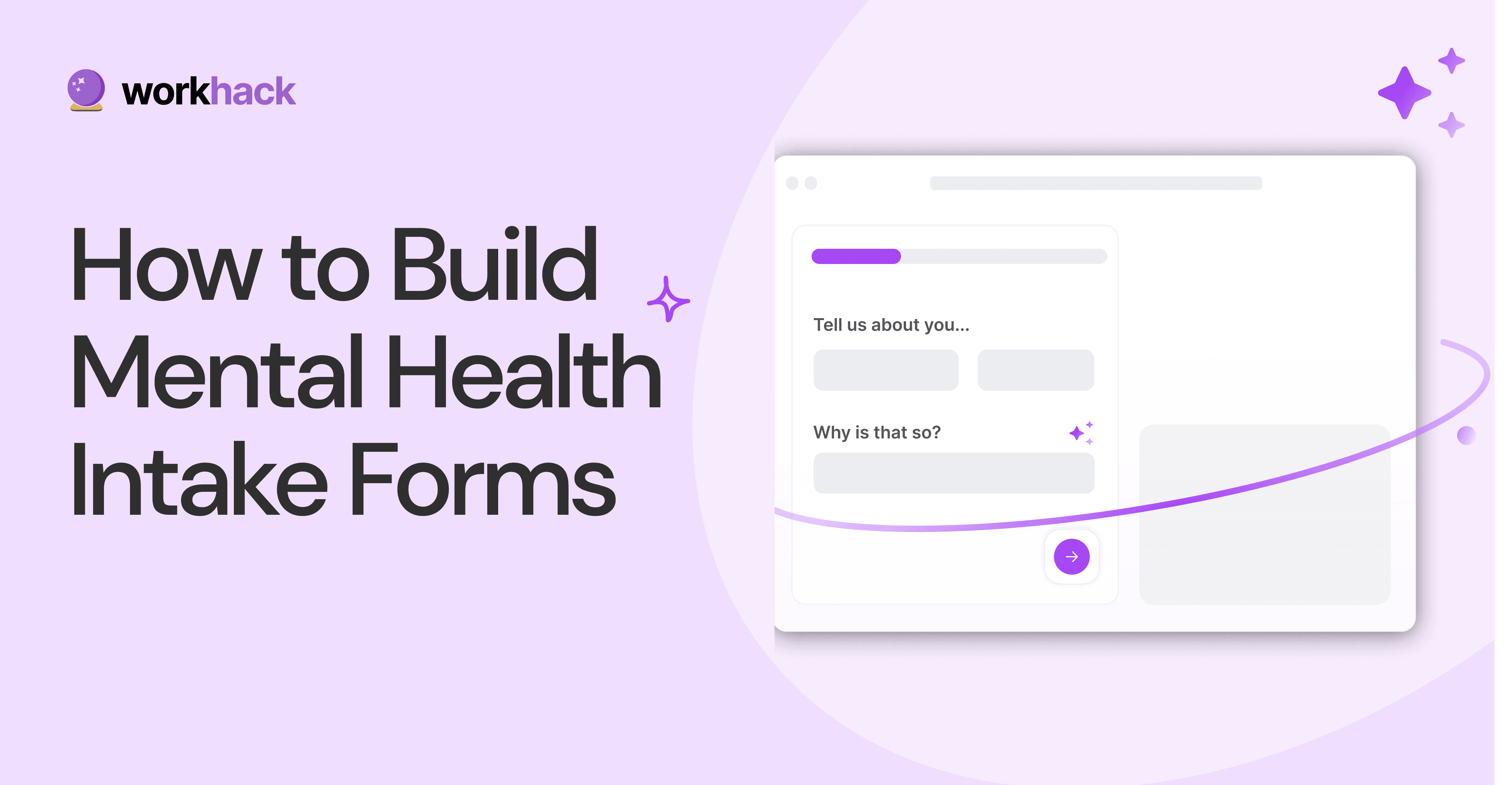
How to Build Mental Health Intake Forms?
Mental health intake forms are not like patient intake forms. Mental health intake forms deal with far more sensitive data and have specific design methods.
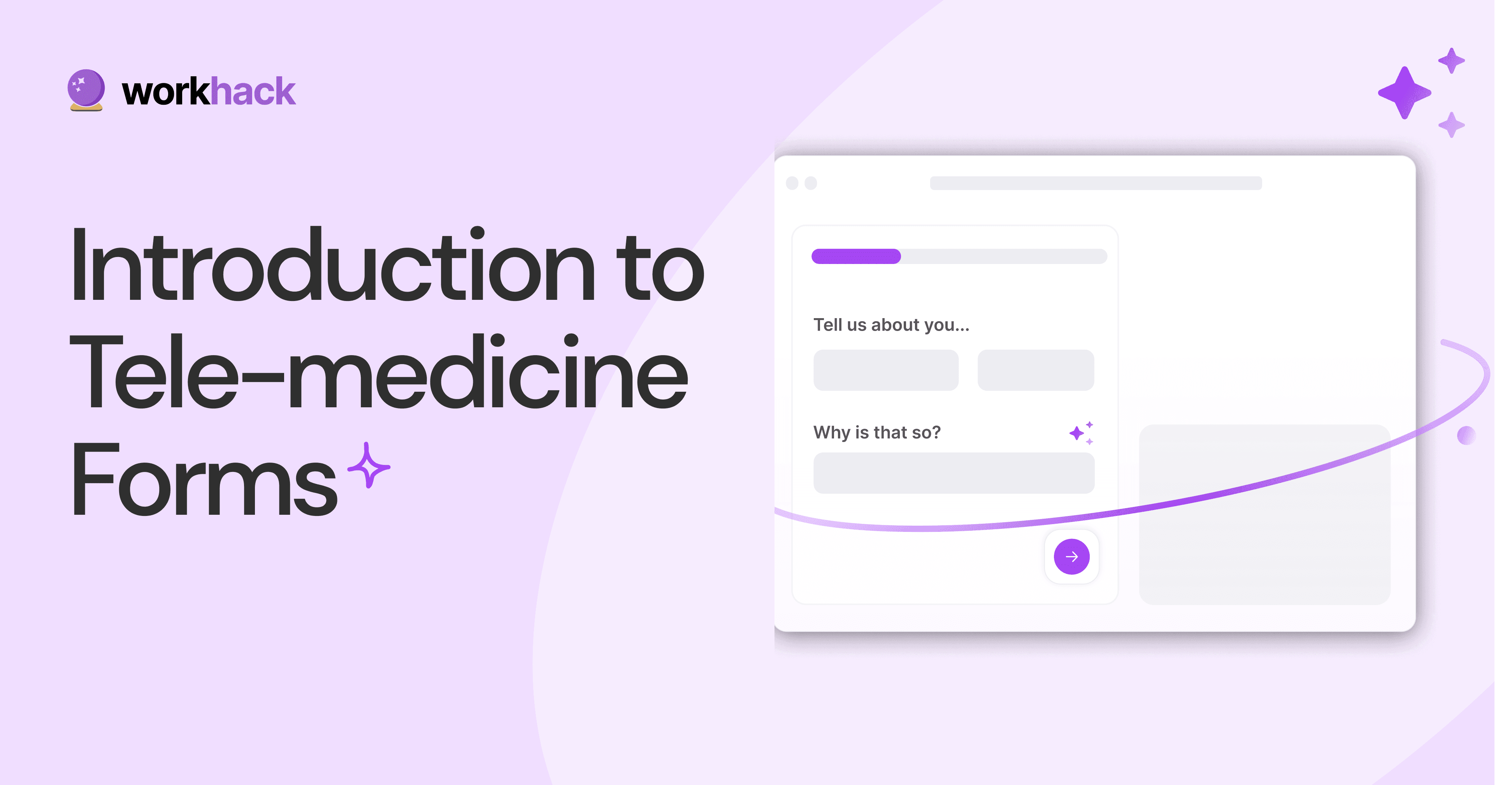
What, Why and How of Telemedicine Forms.
Telemedicine is on the rise and with different form builders out there, which one best suits your needs as a healthcare services provider?
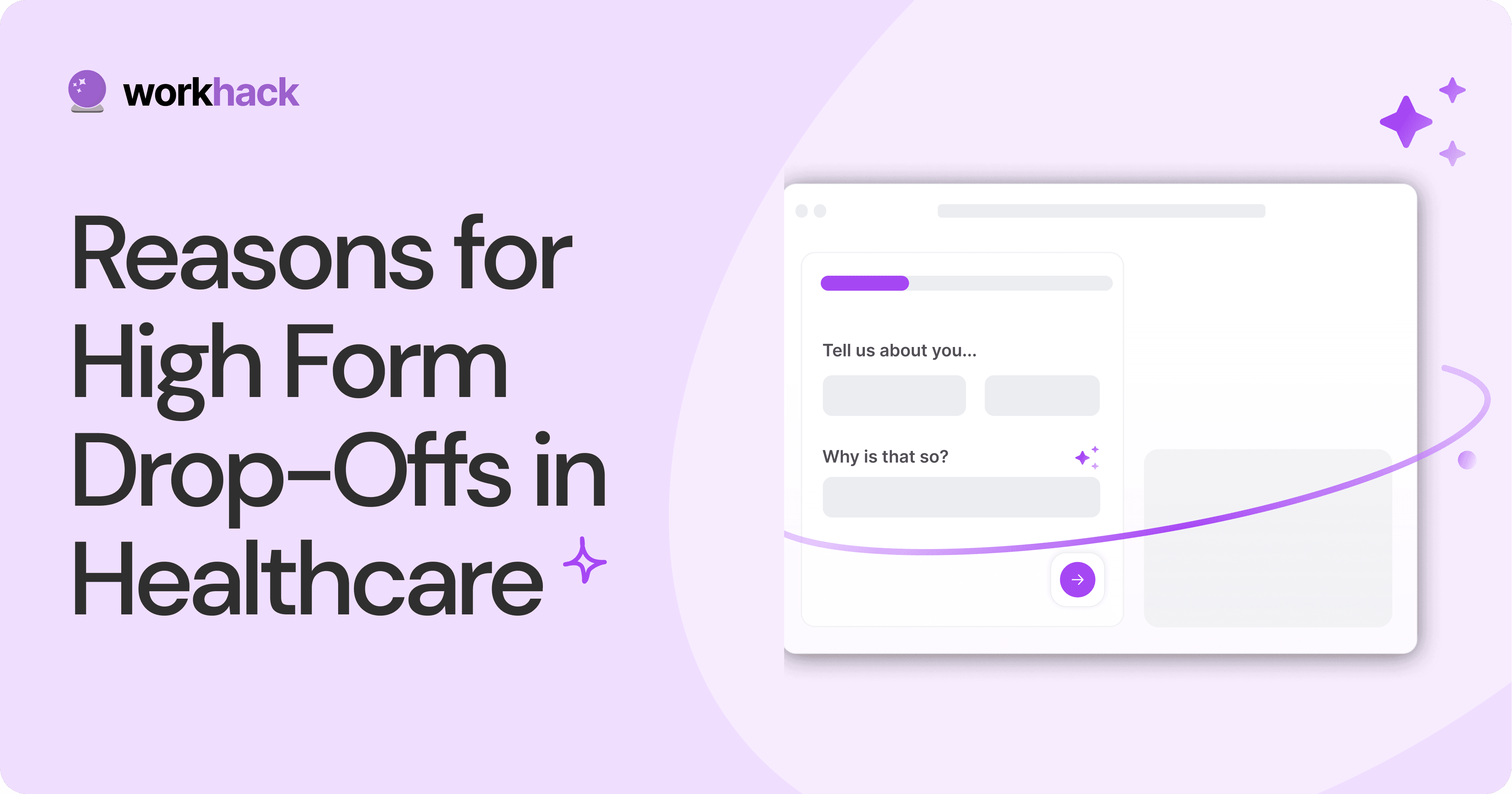
3 Reasons for Major Drop-Offs in Medical Forms.
No matter which healthcare form we pick, there are major drop-off reasons. We shall dive into the top 3 and learn how to resolve them in your next form.
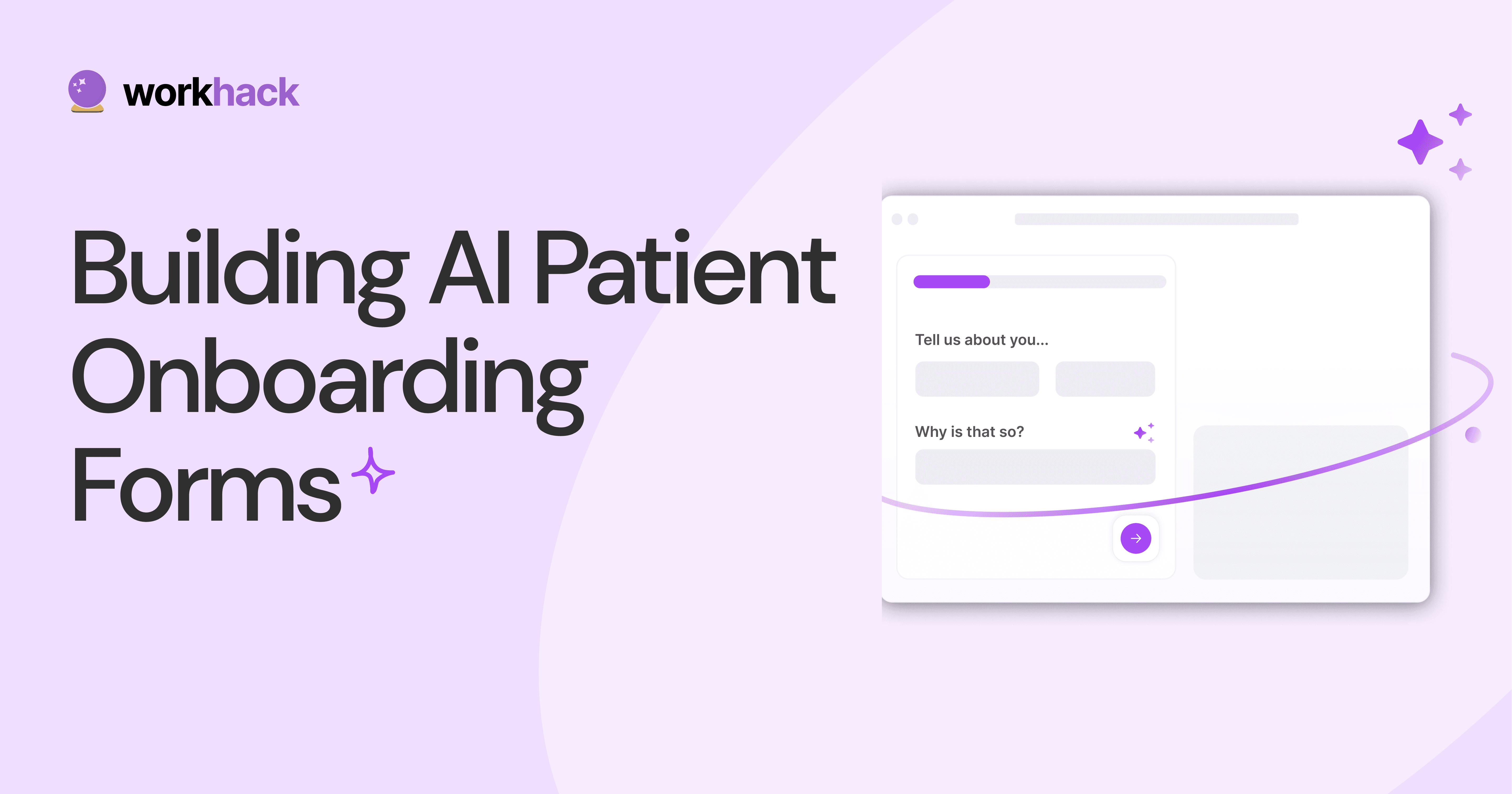
Patient Onboarding Forms - From Click to Clinic.
Patient onboarding forms are the first touchpoint for patients; getting this right for higher conversion rates is a must-have. Learn how to perfect them now.

5 Key Parts of a Good Patient Satisfaction Form.
The goal of patient satisfaction surveys is to course-correct the services of a healthcare provider. Patient feedback leads to a culture of patient-centric care.
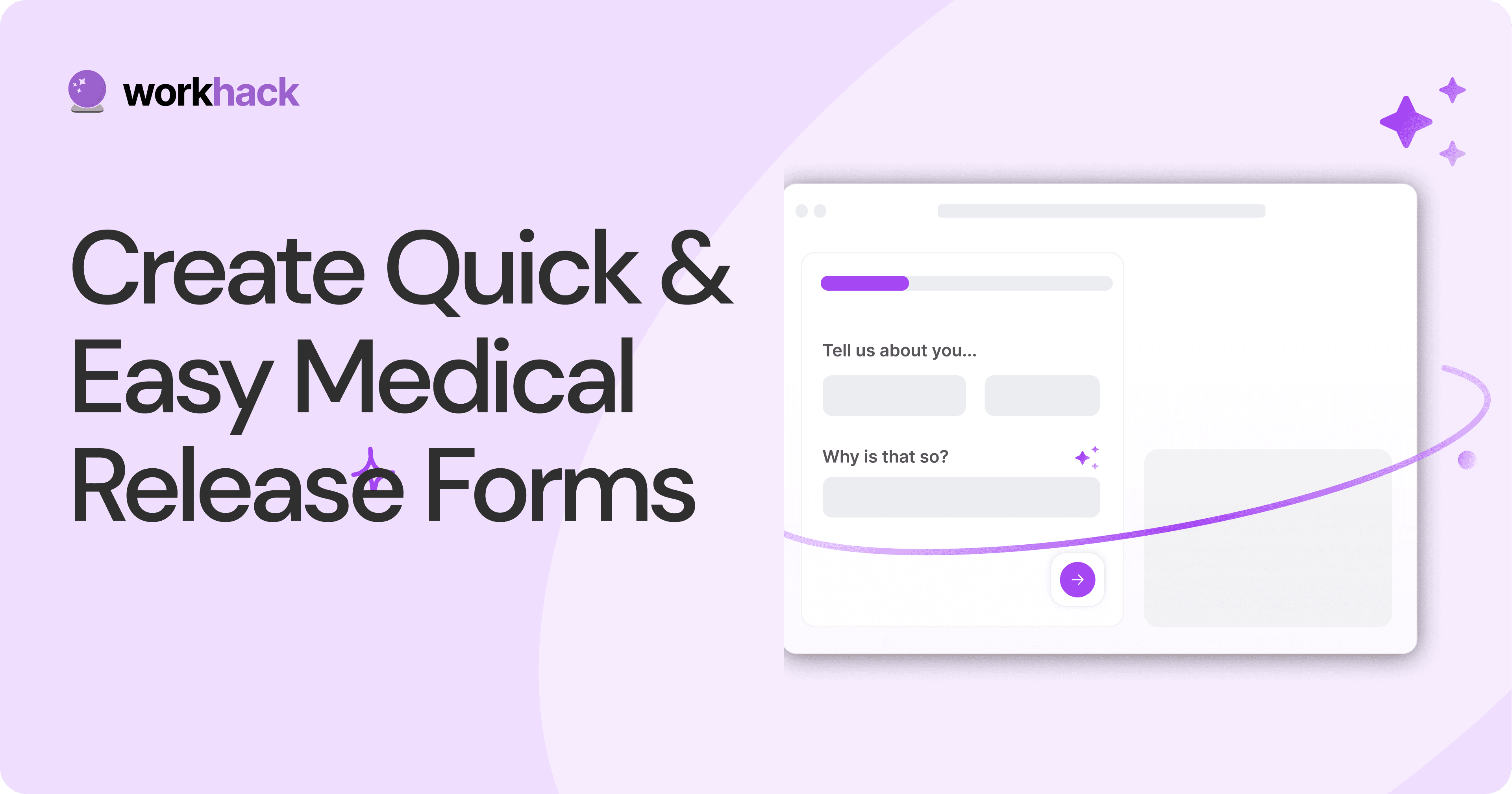
Build Quick and Easy Medical Release Forms.
Every HIPAA-compliant healthcare provider comes across medical release forms that involve details from medical history forms. Can they be shipped fast? Yes.
Subscribe to stay updated.
Subscribe to stay updated.
Subscribe to stay updated.
HC
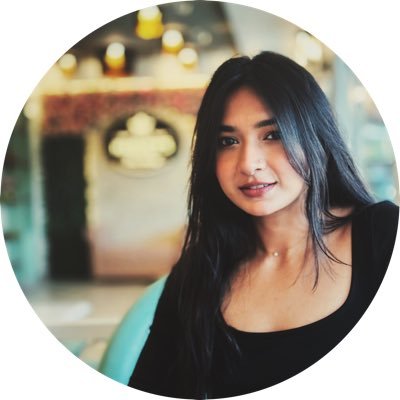
HC
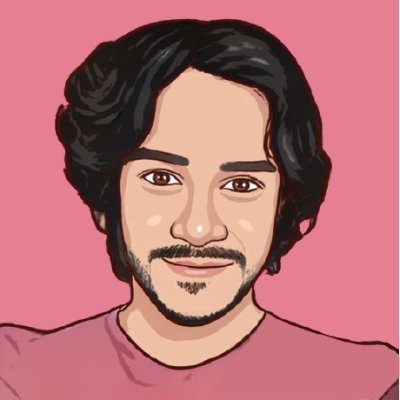
HC
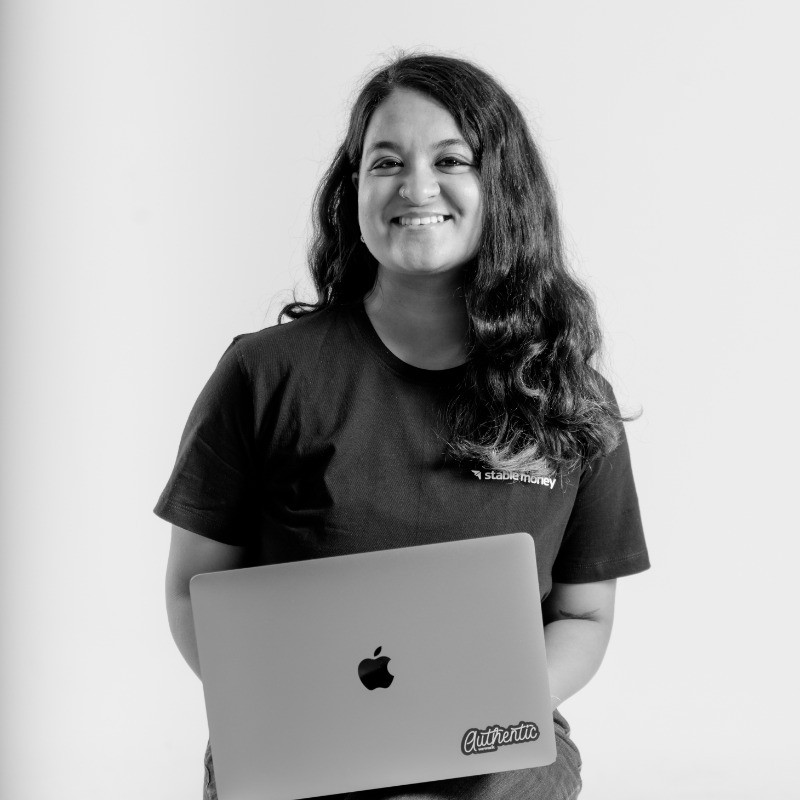
HC
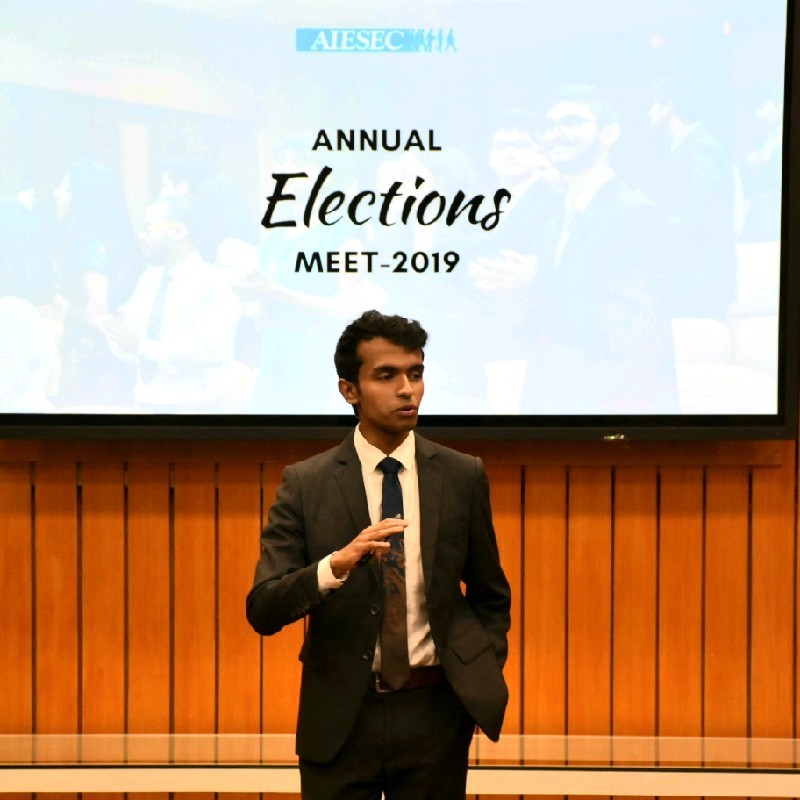
70+ people from across industries read our emails.
HC
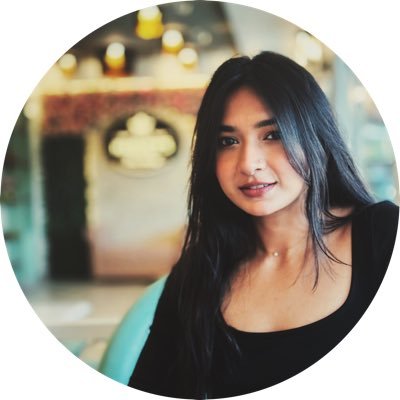
HC
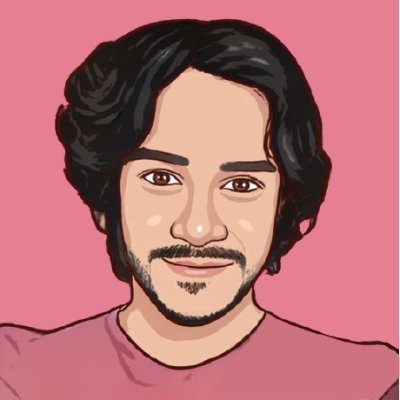
70+ people from across industries read our emails.
HC
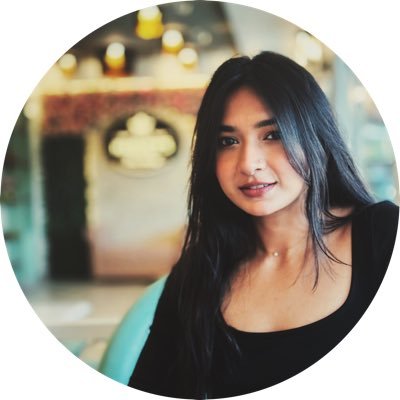
HC
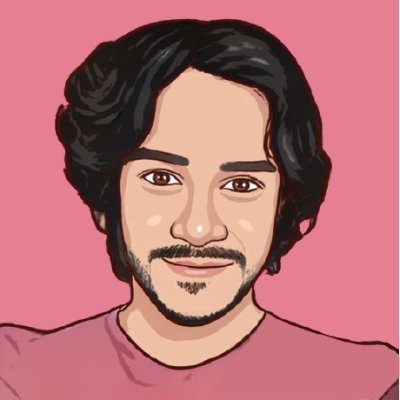
HC
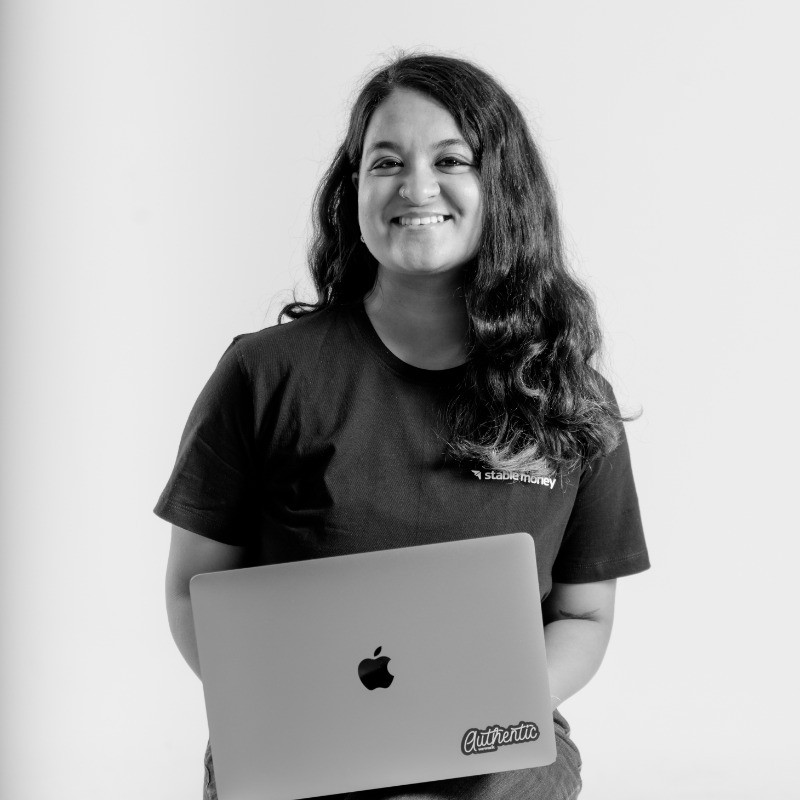
70+ people from across industries read our emails.
Bangalore, India / San Francisco, US
WorkHack Inc. 2023
Bangalore, India
San Francisco, US
WorkHack Inc. 2023
WorkHack Inc. 2023
Bangalore, India / San Francisco, US
WorkHack Inc. 2023
Bangalore, India / San Francisco, US