Product Research Redefined: Qualitative Insights of Today Vs. Data Science of Yesterday
Product Research Redefined: Qualitative Insights of Today Vs. Data Science of Yesterday
Product Research Redefined: Qualitative Insights of Today Vs. Data Science of Yesterday



In product research, we often find ourselves at the crossroads of data science and qualitative insights. These two domains have traditionally represented distinct approaches to understanding users and their needs. Yesterday's data science, with its rigorous statistical models and structured datasets, offered a firm grounding in numbers and trends. Today, qualitative insights are enriching our understanding by adding depth and context to the raw data, enabling us to see beyond the numbers.
This blog delves into how the landscape of product research has evolved from the quantitative data of yesteryear to the nuanced, qualitative methodologies of today. We’ll explore the significant contrasts and highlight how modern tools and approaches are reshaping how we uncover and act on user insights.
From Data Doldrums to Dynamic Discovery
In The Past: Limited and Structured Data
Back in the day, data science was all about numbers. We relied on structured data from controlled sources like surveys, transactional records, and standardized databases. These datasets were neatly arranged in rows and columns, awaiting the analytical prowess of statisticians tools like SPSS and SAS. Data collection was a painstaking process involving manual surveys, carefully designed forms, and rigorously controlled experiments.
While this approach provided clarity and precision, it often felt like navigating with a map that only showed major highways, leaving out the intricate side streets where valuable insights might be lurking. We had a solid understanding of what was happening, but we often lacked the why.
Today: Diverse and Rich Data
Today, data comes at us from all directions, and it’s anything but neat. We’re dealing with a deluge of diverse data sources—social media chatter, user forums, video feedback, and open-ended survey responses. This data is rich in context and bursting with nuances that structured datasets of the past simply couldn’t capture.
Modern qualitative tools, powered by AI, can handle this complexity. They scrape web data, process video feedback, and analyze social media interactions in real-time, giving us a dynamic and multi-faceted view of user experiences. It’s like upgrading from a static map to a real-time GPS, guiding us through the ever-changing landscape of user behavior.
From Statistical Silos to Smart Synthesis
In The Past: Statistical and Predictive Models
In traditional data science, statistical methods reigned supreme. We used regression analysis, hypothesis testing, and predictive modeling to make sense of our neatly structured data. These methods required clean, numeric datasets and provided us with valuable insights into trends and patterns. But there was a catch: they often lacked context.
Imagine analyzing sales data and finding a decline. The numbers were clear, but the story behind them wasn’t. We knew what was happening—sales were dropping—but without qualitative input, we were left guessing why. We were the analytical detectives, but the case files often had missing pages.
Today: Machine Learning and NLP
Fast forward to today, and our analytical toolkit has expanded significantly. Qualitative insights are now driven by advanced machine learning algorithms and natural language processing (NLP). These tools can parse through unstructured data, uncovering themes, sentiments, and correlations that would have been hidden in the numeric haze of traditional datasets.
AI-powered platforms like TensorFlow and SpaCy enable us to process vast amounts of text, voice, and even video data, transforming them into actionable insights. We can now understand not just that users are unhappy, but also the specific experiences and emotions driving their dissatisfaction. It’s like having a team of digital anthropologists at our disposal, turning raw data into rich, narrative insights.
From Data Drudgery to Dynamic Decisions
In The Past: Hypothesis-Driven Decisions
Earlier, our decisions were often hypothesis-driven, guided by statistical significance and historical trends. We spent considerable time collecting, cleaning, and analyzing data before reaching actionable conclusions. It was a meticulous process that, while thorough, often felt like steering a ship through foggy waters—slow and prone to unexpected obstacles.
We relied on periodic reports and static analyses, making it challenging to respond swiftly to new developments or unexpected trends. The pace of decision-making was often out of sync with the rapid changes in user behavior and market dynamics.
Today: Real-Time and Exploratory Decisions
Today’s qualitative insights, turbocharged by AI-powered survey builders like Metaforms, offer a breath of fresh air. Real-time analytics and exploratory analysis are the new norms, allowing us to adapt swiftly to emerging trends and user feedback. We can pivot our research focus almost instantaneously, reacting to competitor moves, market shifts, or unexpected user responses with agility and precision.
AI-native survey builders provide immediate feedback, transforming how we engage with data. We can act on insights as they emerge, making decisions in real-time based on the latest user sentiments and behaviors. It’s like having a high-speed sports car in the data race, leaving behind the slow-moving caravans of yesterday.
From Passive Reports to Participatory Insights
In The Past: Passive Engagement
In the not-so-distant past, stakeholder engagement was often a passive affair. We produced static reports and delivered lengthy presentations, hoping to illuminate the path forward. User feedback was similarly limited to structured surveys and controlled interviews, providing a narrow view of user experiences.
Insights were shared in formal, often delayed, communications, making it difficult to incorporate feedback in a timely manner. Stakeholders and researchers alike were navigating through the fog, trying to piece together a coherent strategy from fragmented data points.
Today: Active and Interactive Engagement
Modern qualitative insights have transformed stakeholder engagement into an active, participatory process. Interactive dashboards, real-time feedback loops, and participatory research methods are the new standard. We’re now engaging with stakeholders and users in real-time, using dynamic visualizations and live data feeds to drive discussions and decisions.
Findings are communicated through interactive platforms that allow for immediate input and iteration, aligning research efforts more closely with business needs and user expectations. It’s a collaborative dance between researchers, stakeholders, and users, turning insights into actionable strategies faster and more effectively than ever before.
Conclusion: Embracing the Best of Both Worlds
The evolution from the data science of yesterday to the qualitative insights of today reflects a broader shift in how we approach product research. It’s no longer enough to simply understand what is happening—we need to delve into the why, the how, and the what’s next.
By combining the numerical rigor of traditional data science with the rich, contextual understanding provided by modern qualitative insights, we’re better equipped to navigate the complexities of user behavior and market dynamics. This holistic approach not only enhances our research capabilities but also ensures that our products are more aligned with user needs and business goals.
So, as we move forward with product research, we leverage the strengths of both methodologies, using the precision of data science and the depth of qualitative insights using advanced product research survey builders like Metaforms.ai to drive innovation and create products that truly resonate with our users.
In product research, we often find ourselves at the crossroads of data science and qualitative insights. These two domains have traditionally represented distinct approaches to understanding users and their needs. Yesterday's data science, with its rigorous statistical models and structured datasets, offered a firm grounding in numbers and trends. Today, qualitative insights are enriching our understanding by adding depth and context to the raw data, enabling us to see beyond the numbers.
This blog delves into how the landscape of product research has evolved from the quantitative data of yesteryear to the nuanced, qualitative methodologies of today. We’ll explore the significant contrasts and highlight how modern tools and approaches are reshaping how we uncover and act on user insights.
From Data Doldrums to Dynamic Discovery
In The Past: Limited and Structured Data
Back in the day, data science was all about numbers. We relied on structured data from controlled sources like surveys, transactional records, and standardized databases. These datasets were neatly arranged in rows and columns, awaiting the analytical prowess of statisticians tools like SPSS and SAS. Data collection was a painstaking process involving manual surveys, carefully designed forms, and rigorously controlled experiments.
While this approach provided clarity and precision, it often felt like navigating with a map that only showed major highways, leaving out the intricate side streets where valuable insights might be lurking. We had a solid understanding of what was happening, but we often lacked the why.
Today: Diverse and Rich Data
Today, data comes at us from all directions, and it’s anything but neat. We’re dealing with a deluge of diverse data sources—social media chatter, user forums, video feedback, and open-ended survey responses. This data is rich in context and bursting with nuances that structured datasets of the past simply couldn’t capture.
Modern qualitative tools, powered by AI, can handle this complexity. They scrape web data, process video feedback, and analyze social media interactions in real-time, giving us a dynamic and multi-faceted view of user experiences. It’s like upgrading from a static map to a real-time GPS, guiding us through the ever-changing landscape of user behavior.
From Statistical Silos to Smart Synthesis
In The Past: Statistical and Predictive Models
In traditional data science, statistical methods reigned supreme. We used regression analysis, hypothesis testing, and predictive modeling to make sense of our neatly structured data. These methods required clean, numeric datasets and provided us with valuable insights into trends and patterns. But there was a catch: they often lacked context.
Imagine analyzing sales data and finding a decline. The numbers were clear, but the story behind them wasn’t. We knew what was happening—sales were dropping—but without qualitative input, we were left guessing why. We were the analytical detectives, but the case files often had missing pages.
Today: Machine Learning and NLP
Fast forward to today, and our analytical toolkit has expanded significantly. Qualitative insights are now driven by advanced machine learning algorithms and natural language processing (NLP). These tools can parse through unstructured data, uncovering themes, sentiments, and correlations that would have been hidden in the numeric haze of traditional datasets.
AI-powered platforms like TensorFlow and SpaCy enable us to process vast amounts of text, voice, and even video data, transforming them into actionable insights. We can now understand not just that users are unhappy, but also the specific experiences and emotions driving their dissatisfaction. It’s like having a team of digital anthropologists at our disposal, turning raw data into rich, narrative insights.
From Data Drudgery to Dynamic Decisions
In The Past: Hypothesis-Driven Decisions
Earlier, our decisions were often hypothesis-driven, guided by statistical significance and historical trends. We spent considerable time collecting, cleaning, and analyzing data before reaching actionable conclusions. It was a meticulous process that, while thorough, often felt like steering a ship through foggy waters—slow and prone to unexpected obstacles.
We relied on periodic reports and static analyses, making it challenging to respond swiftly to new developments or unexpected trends. The pace of decision-making was often out of sync with the rapid changes in user behavior and market dynamics.
Today: Real-Time and Exploratory Decisions
Today’s qualitative insights, turbocharged by AI-powered survey builders like Metaforms, offer a breath of fresh air. Real-time analytics and exploratory analysis are the new norms, allowing us to adapt swiftly to emerging trends and user feedback. We can pivot our research focus almost instantaneously, reacting to competitor moves, market shifts, or unexpected user responses with agility and precision.
AI-native survey builders provide immediate feedback, transforming how we engage with data. We can act on insights as they emerge, making decisions in real-time based on the latest user sentiments and behaviors. It’s like having a high-speed sports car in the data race, leaving behind the slow-moving caravans of yesterday.
From Passive Reports to Participatory Insights
In The Past: Passive Engagement
In the not-so-distant past, stakeholder engagement was often a passive affair. We produced static reports and delivered lengthy presentations, hoping to illuminate the path forward. User feedback was similarly limited to structured surveys and controlled interviews, providing a narrow view of user experiences.
Insights were shared in formal, often delayed, communications, making it difficult to incorporate feedback in a timely manner. Stakeholders and researchers alike were navigating through the fog, trying to piece together a coherent strategy from fragmented data points.
Today: Active and Interactive Engagement
Modern qualitative insights have transformed stakeholder engagement into an active, participatory process. Interactive dashboards, real-time feedback loops, and participatory research methods are the new standard. We’re now engaging with stakeholders and users in real-time, using dynamic visualizations and live data feeds to drive discussions and decisions.
Findings are communicated through interactive platforms that allow for immediate input and iteration, aligning research efforts more closely with business needs and user expectations. It’s a collaborative dance between researchers, stakeholders, and users, turning insights into actionable strategies faster and more effectively than ever before.
Conclusion: Embracing the Best of Both Worlds
The evolution from the data science of yesterday to the qualitative insights of today reflects a broader shift in how we approach product research. It’s no longer enough to simply understand what is happening—we need to delve into the why, the how, and the what’s next.
By combining the numerical rigor of traditional data science with the rich, contextual understanding provided by modern qualitative insights, we’re better equipped to navigate the complexities of user behavior and market dynamics. This holistic approach not only enhances our research capabilities but also ensures that our products are more aligned with user needs and business goals.
So, as we move forward with product research, we leverage the strengths of both methodologies, using the precision of data science and the depth of qualitative insights using advanced product research survey builders like Metaforms.ai to drive innovation and create products that truly resonate with our users.
In product research, we often find ourselves at the crossroads of data science and qualitative insights. These two domains have traditionally represented distinct approaches to understanding users and their needs. Yesterday's data science, with its rigorous statistical models and structured datasets, offered a firm grounding in numbers and trends. Today, qualitative insights are enriching our understanding by adding depth and context to the raw data, enabling us to see beyond the numbers.
This blog delves into how the landscape of product research has evolved from the quantitative data of yesteryear to the nuanced, qualitative methodologies of today. We’ll explore the significant contrasts and highlight how modern tools and approaches are reshaping how we uncover and act on user insights.
From Data Doldrums to Dynamic Discovery
In The Past: Limited and Structured Data
Back in the day, data science was all about numbers. We relied on structured data from controlled sources like surveys, transactional records, and standardized databases. These datasets were neatly arranged in rows and columns, awaiting the analytical prowess of statisticians tools like SPSS and SAS. Data collection was a painstaking process involving manual surveys, carefully designed forms, and rigorously controlled experiments.
While this approach provided clarity and precision, it often felt like navigating with a map that only showed major highways, leaving out the intricate side streets where valuable insights might be lurking. We had a solid understanding of what was happening, but we often lacked the why.
Today: Diverse and Rich Data
Today, data comes at us from all directions, and it’s anything but neat. We’re dealing with a deluge of diverse data sources—social media chatter, user forums, video feedback, and open-ended survey responses. This data is rich in context and bursting with nuances that structured datasets of the past simply couldn’t capture.
Modern qualitative tools, powered by AI, can handle this complexity. They scrape web data, process video feedback, and analyze social media interactions in real-time, giving us a dynamic and multi-faceted view of user experiences. It’s like upgrading from a static map to a real-time GPS, guiding us through the ever-changing landscape of user behavior.
From Statistical Silos to Smart Synthesis
In The Past: Statistical and Predictive Models
In traditional data science, statistical methods reigned supreme. We used regression analysis, hypothesis testing, and predictive modeling to make sense of our neatly structured data. These methods required clean, numeric datasets and provided us with valuable insights into trends and patterns. But there was a catch: they often lacked context.
Imagine analyzing sales data and finding a decline. The numbers were clear, but the story behind them wasn’t. We knew what was happening—sales were dropping—but without qualitative input, we were left guessing why. We were the analytical detectives, but the case files often had missing pages.
Today: Machine Learning and NLP
Fast forward to today, and our analytical toolkit has expanded significantly. Qualitative insights are now driven by advanced machine learning algorithms and natural language processing (NLP). These tools can parse through unstructured data, uncovering themes, sentiments, and correlations that would have been hidden in the numeric haze of traditional datasets.
AI-powered platforms like TensorFlow and SpaCy enable us to process vast amounts of text, voice, and even video data, transforming them into actionable insights. We can now understand not just that users are unhappy, but also the specific experiences and emotions driving their dissatisfaction. It’s like having a team of digital anthropologists at our disposal, turning raw data into rich, narrative insights.
From Data Drudgery to Dynamic Decisions
In The Past: Hypothesis-Driven Decisions
Earlier, our decisions were often hypothesis-driven, guided by statistical significance and historical trends. We spent considerable time collecting, cleaning, and analyzing data before reaching actionable conclusions. It was a meticulous process that, while thorough, often felt like steering a ship through foggy waters—slow and prone to unexpected obstacles.
We relied on periodic reports and static analyses, making it challenging to respond swiftly to new developments or unexpected trends. The pace of decision-making was often out of sync with the rapid changes in user behavior and market dynamics.
Today: Real-Time and Exploratory Decisions
Today’s qualitative insights, turbocharged by AI-powered survey builders like Metaforms, offer a breath of fresh air. Real-time analytics and exploratory analysis are the new norms, allowing us to adapt swiftly to emerging trends and user feedback. We can pivot our research focus almost instantaneously, reacting to competitor moves, market shifts, or unexpected user responses with agility and precision.
AI-native survey builders provide immediate feedback, transforming how we engage with data. We can act on insights as they emerge, making decisions in real-time based on the latest user sentiments and behaviors. It’s like having a high-speed sports car in the data race, leaving behind the slow-moving caravans of yesterday.
From Passive Reports to Participatory Insights
In The Past: Passive Engagement
In the not-so-distant past, stakeholder engagement was often a passive affair. We produced static reports and delivered lengthy presentations, hoping to illuminate the path forward. User feedback was similarly limited to structured surveys and controlled interviews, providing a narrow view of user experiences.
Insights were shared in formal, often delayed, communications, making it difficult to incorporate feedback in a timely manner. Stakeholders and researchers alike were navigating through the fog, trying to piece together a coherent strategy from fragmented data points.
Today: Active and Interactive Engagement
Modern qualitative insights have transformed stakeholder engagement into an active, participatory process. Interactive dashboards, real-time feedback loops, and participatory research methods are the new standard. We’re now engaging with stakeholders and users in real-time, using dynamic visualizations and live data feeds to drive discussions and decisions.
Findings are communicated through interactive platforms that allow for immediate input and iteration, aligning research efforts more closely with business needs and user expectations. It’s a collaborative dance between researchers, stakeholders, and users, turning insights into actionable strategies faster and more effectively than ever before.
Conclusion: Embracing the Best of Both Worlds
The evolution from the data science of yesterday to the qualitative insights of today reflects a broader shift in how we approach product research. It’s no longer enough to simply understand what is happening—we need to delve into the why, the how, and the what’s next.
By combining the numerical rigor of traditional data science with the rich, contextual understanding provided by modern qualitative insights, we’re better equipped to navigate the complexities of user behavior and market dynamics. This holistic approach not only enhances our research capabilities but also ensures that our products are more aligned with user needs and business goals.
So, as we move forward with product research, we leverage the strengths of both methodologies, using the precision of data science and the depth of qualitative insights using advanced product research survey builders like Metaforms.ai to drive innovation and create products that truly resonate with our users.
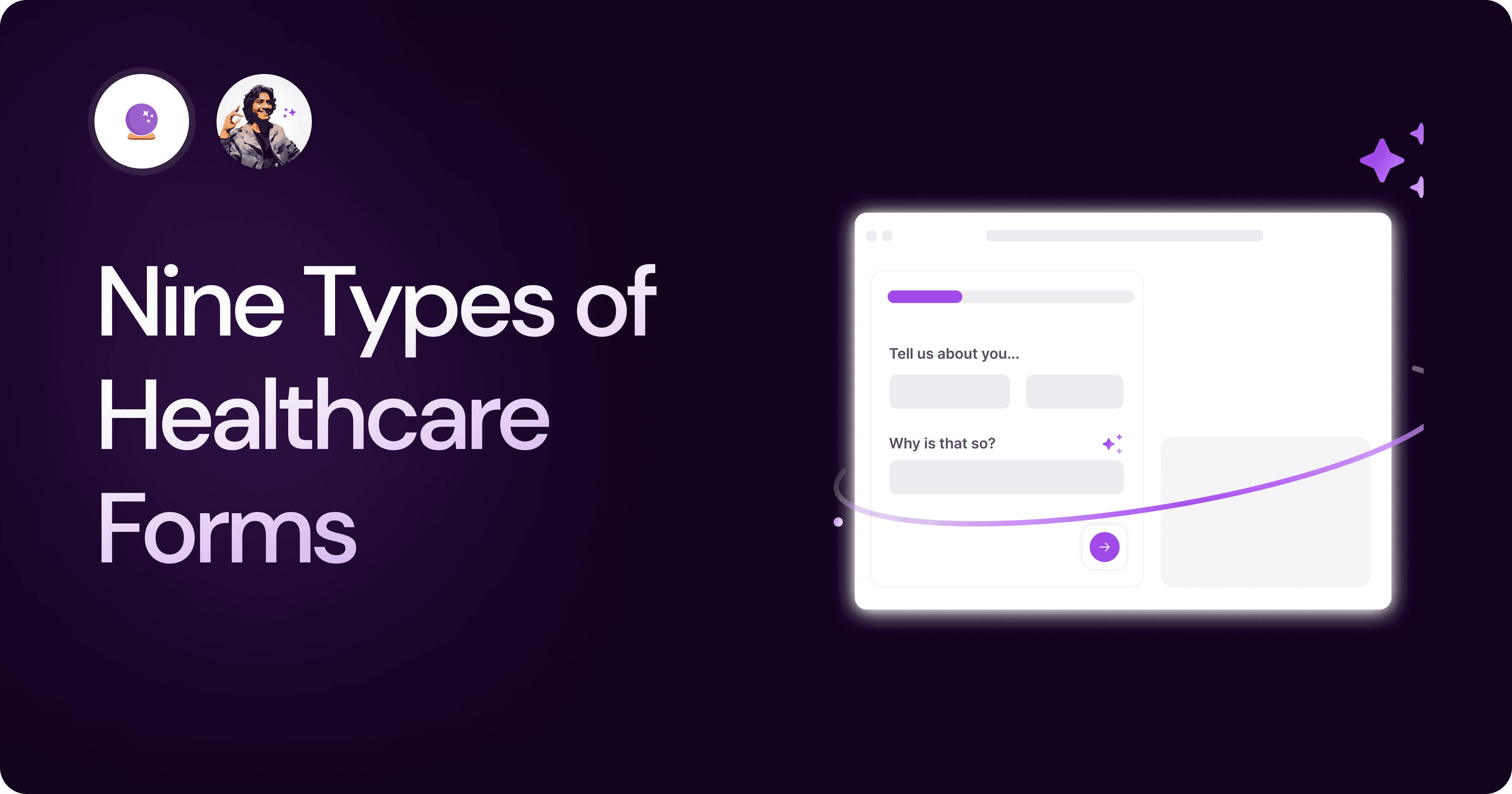
Nine Types of Healthcare and Medical Forms.
Medical forms are a must-have for any healthcare business or practitioner. Learn about the different kinds of medical and healthcare forms.
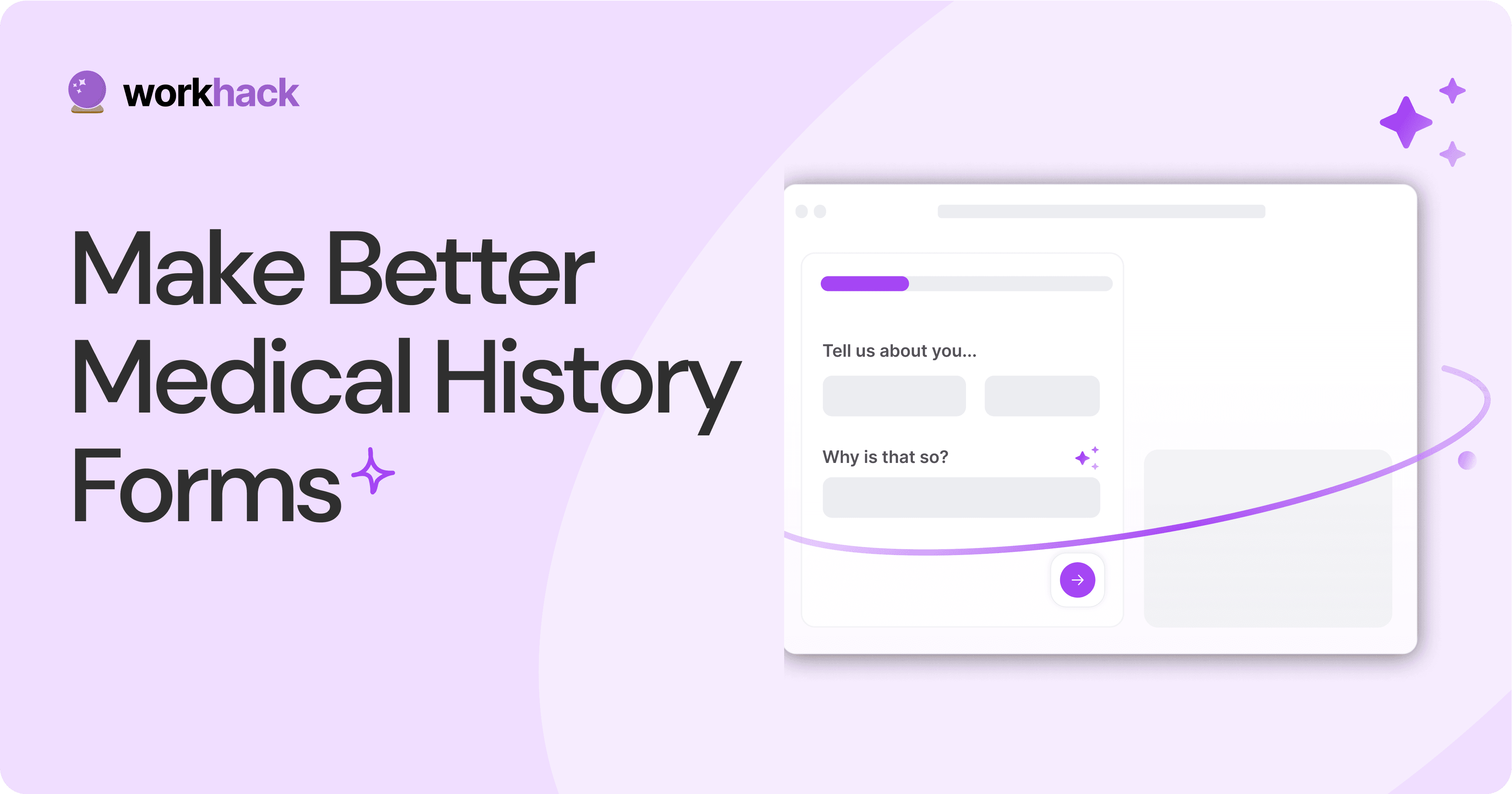
4 Tips for Better Medical History Forms.
Medical history forms are central to patient care, onboarding, and medical administration records. Learn how to make them easier to fill.
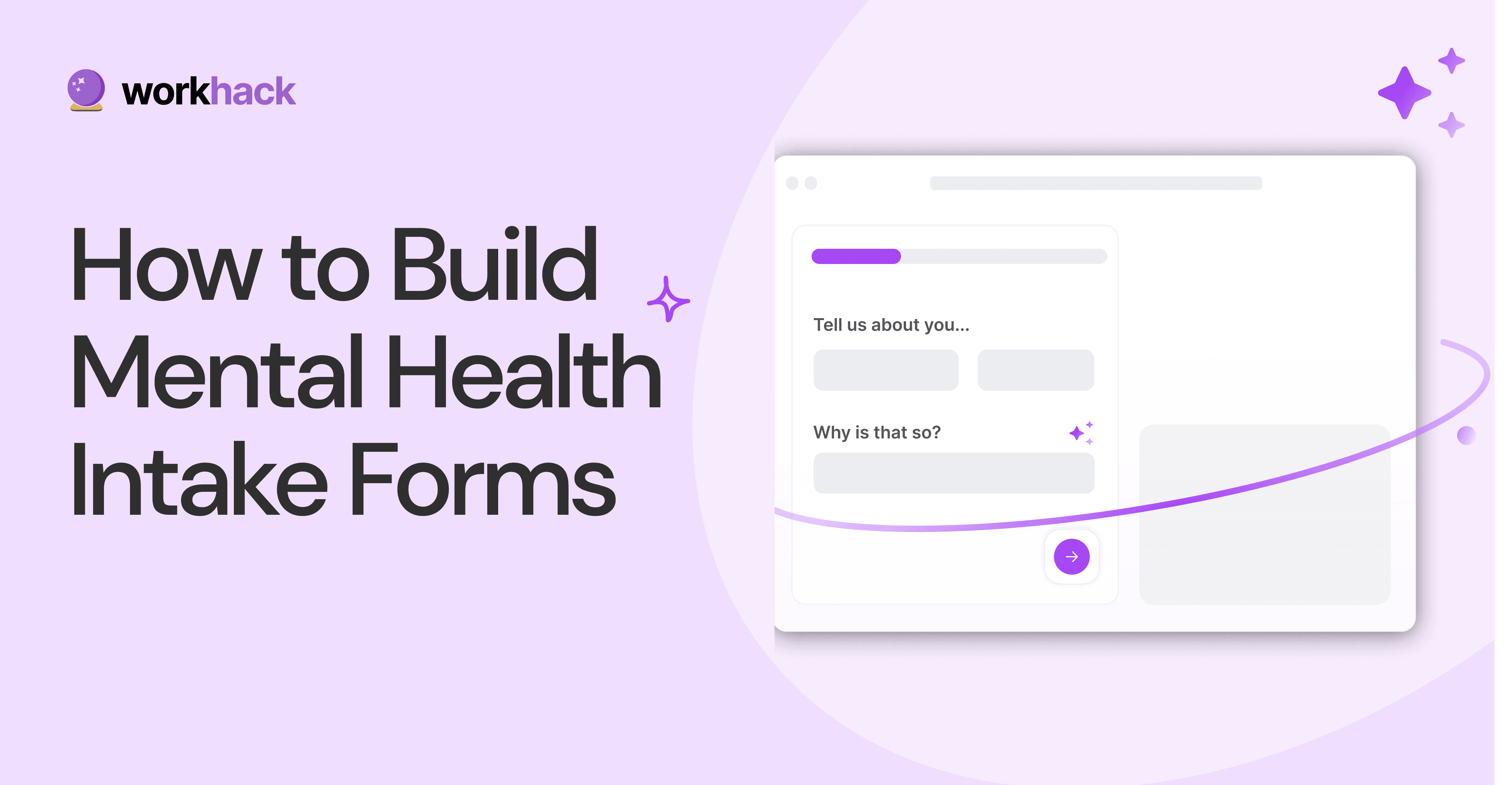
How to Build Mental Health Intake Forms?
Mental health intake forms are not like patient intake forms. Mental health intake forms deal with far more sensitive data and have specific design methods.
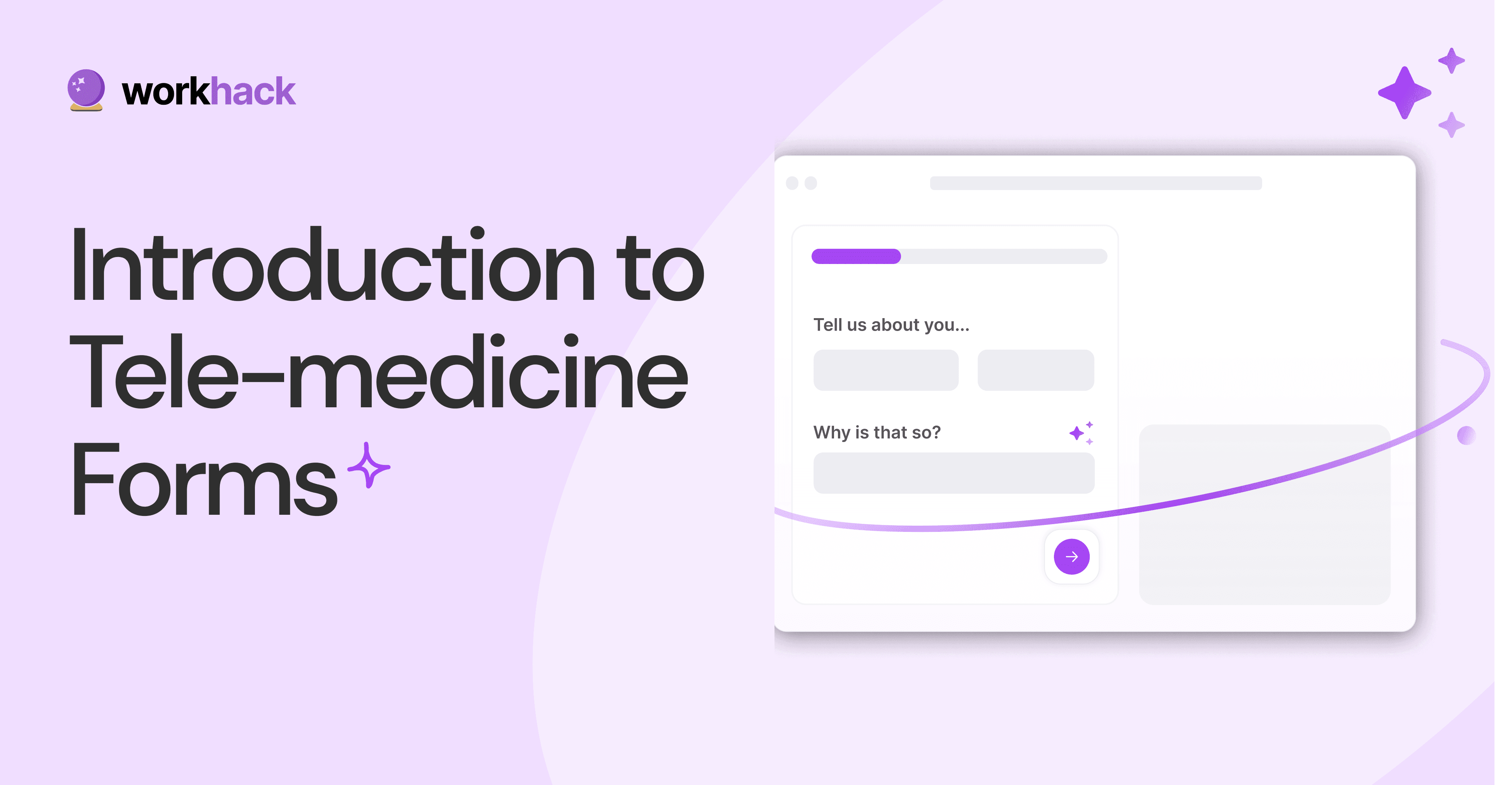
What, Why and How of Telemedicine Forms.
Telemedicine is on the rise and with different form builders out there, which one best suits your needs as a healthcare services provider?
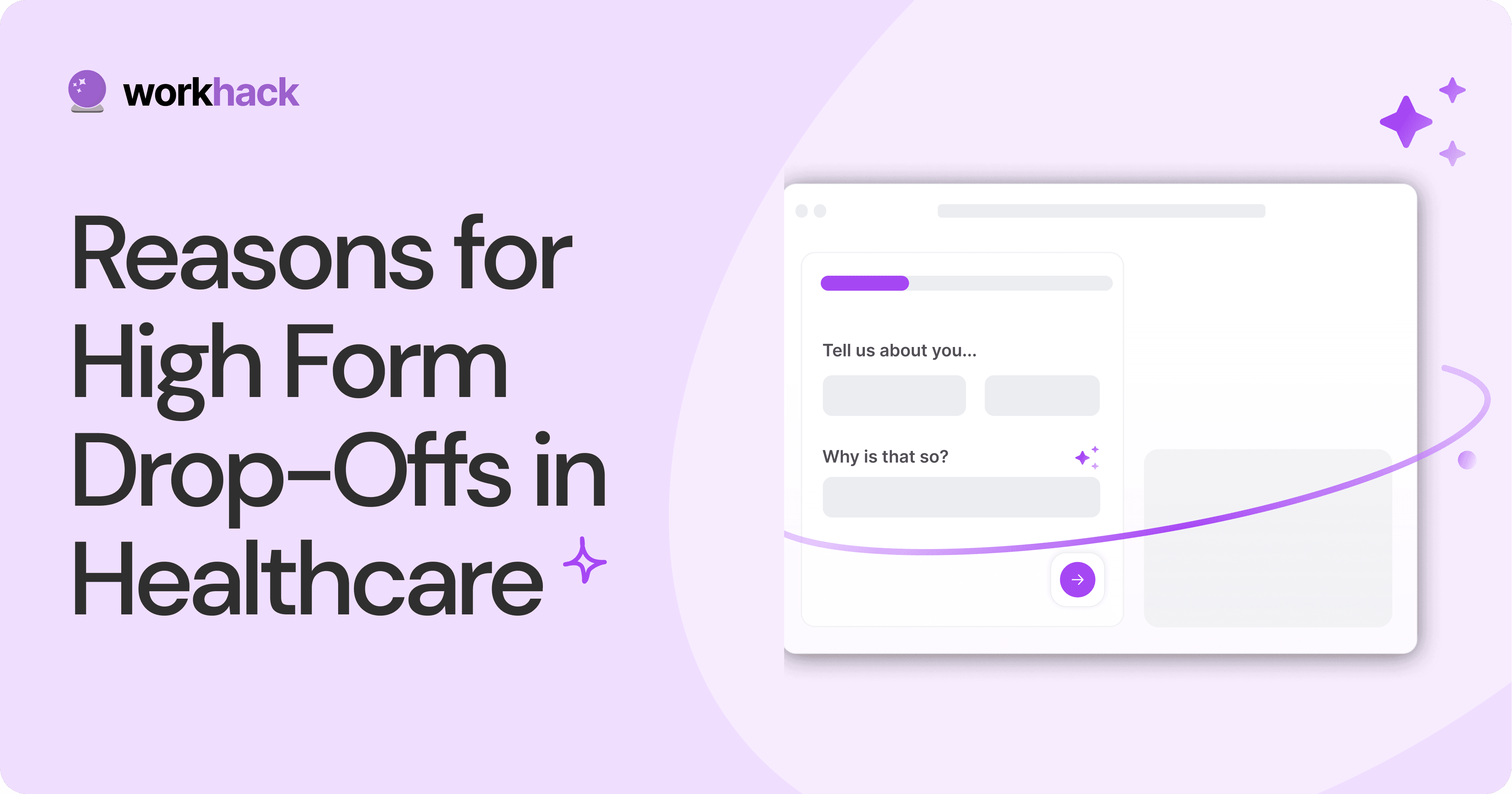
3 Reasons for Major Drop-Offs in Medical Forms.
No matter which healthcare form we pick, there are major drop-off reasons. We shall dive into the top 3 and learn how to resolve them in your next form.
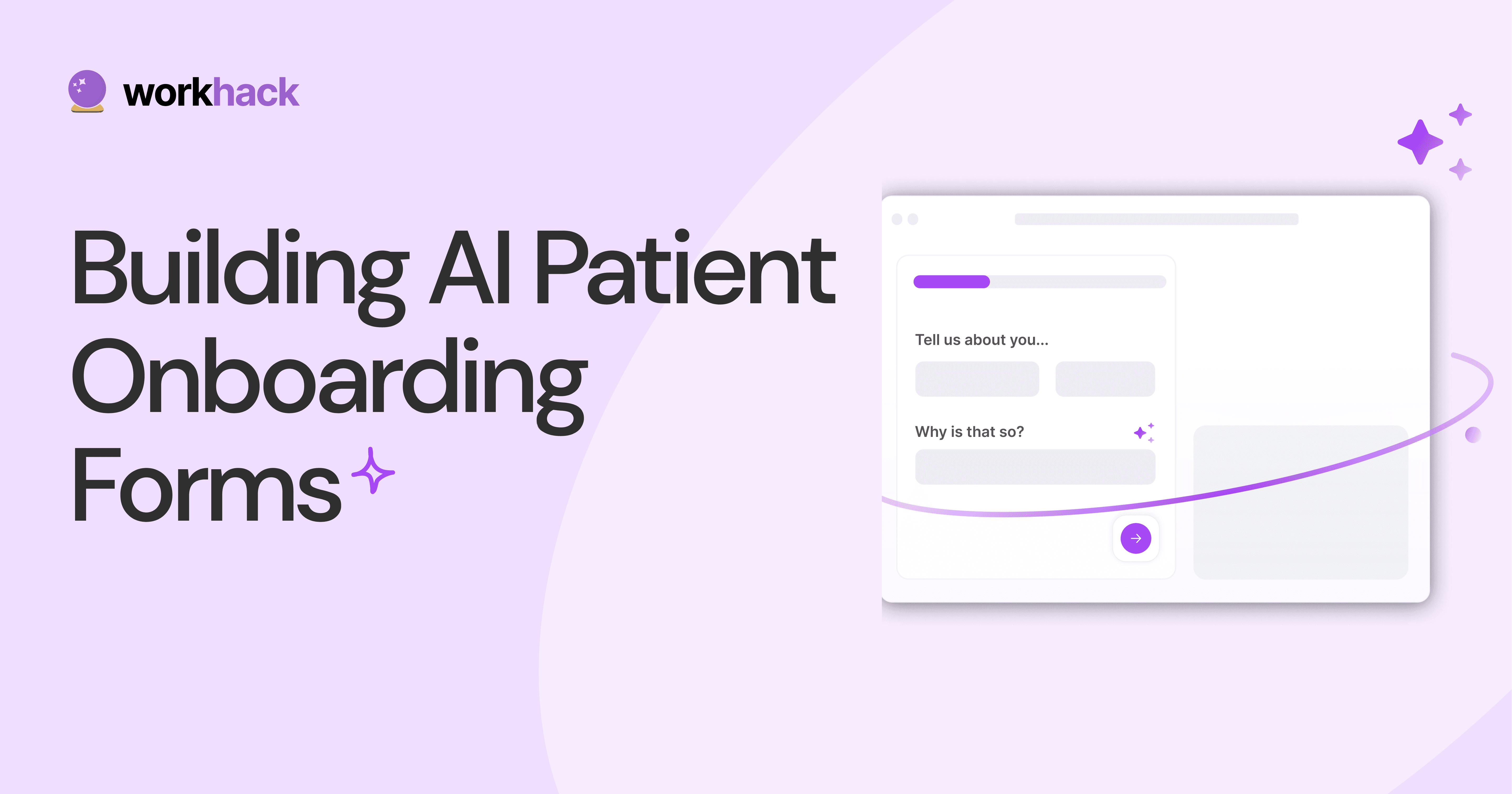
Patient Onboarding Forms - From Click to Clinic.
Patient onboarding forms are the first touchpoint for patients; getting this right for higher conversion rates is a must-have. Learn how to perfect them now.

5 Key Parts of a Good Patient Satisfaction Form.
The goal of patient satisfaction surveys is to course-correct the services of a healthcare provider. Patient feedback leads to a culture of patient-centric care.
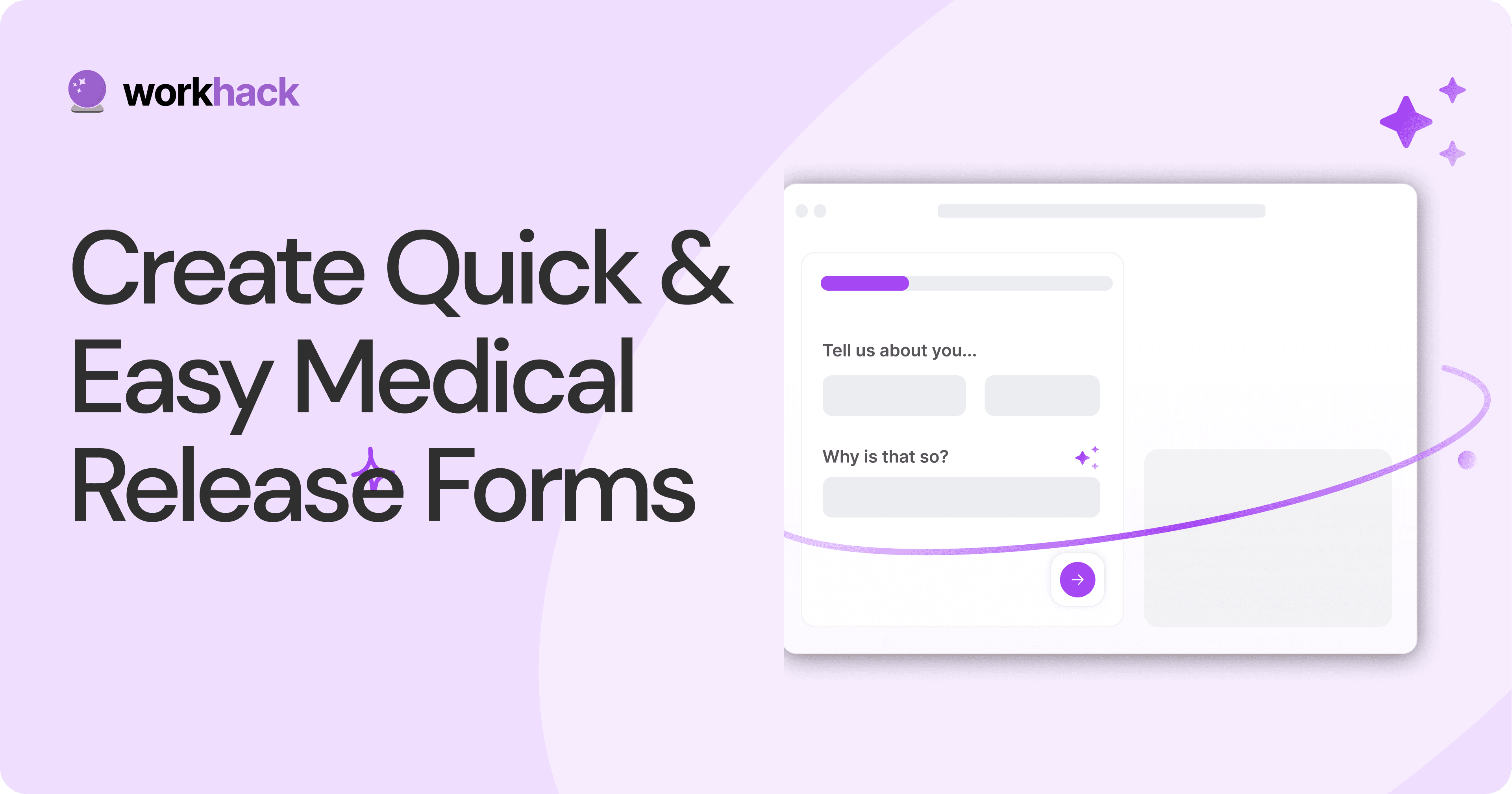
Build Quick and Easy Medical Release Forms.
Every HIPAA-compliant healthcare provider comes across medical release forms that involve details from medical history forms. Can they be shipped fast? Yes.
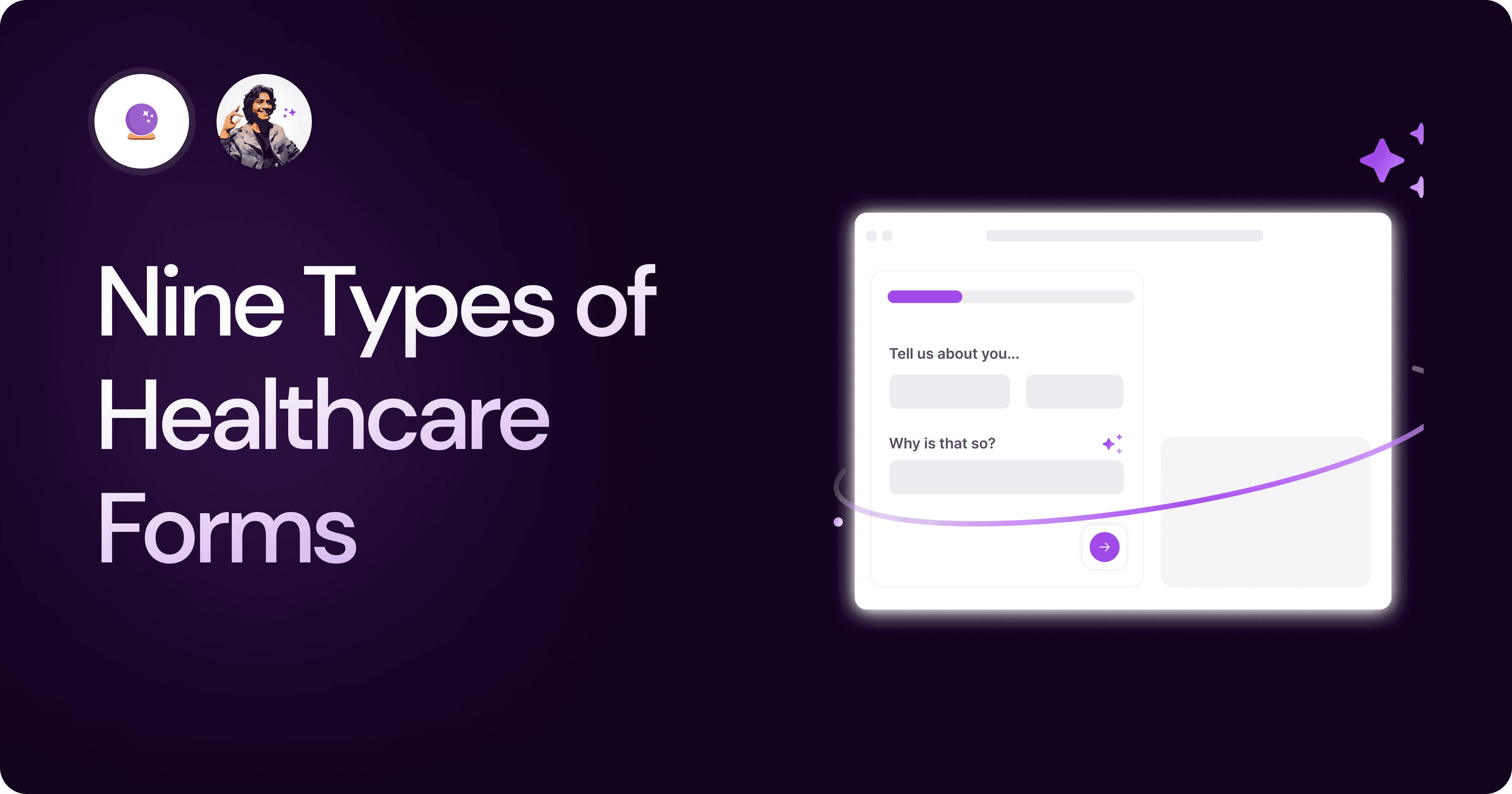
Nine Types of Healthcare and Medical Forms.
Medical forms are a must-have for any healthcare business or practitioner. Learn about the different kinds of medical and healthcare forms.
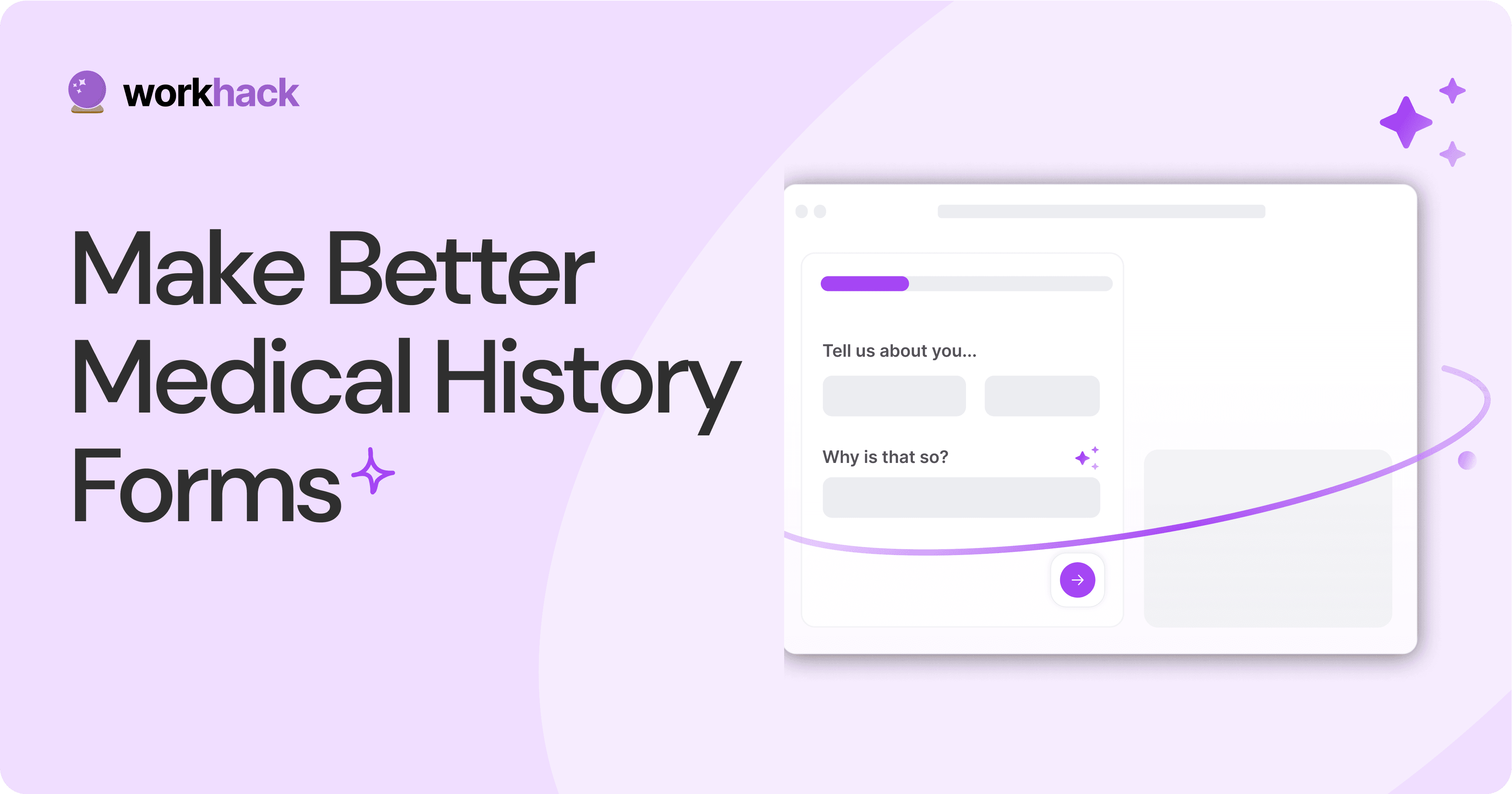
4 Tips for Better Medical History Forms.
Medical history forms are central to patient care, onboarding, and medical administration records. Learn how to make them easier to fill.
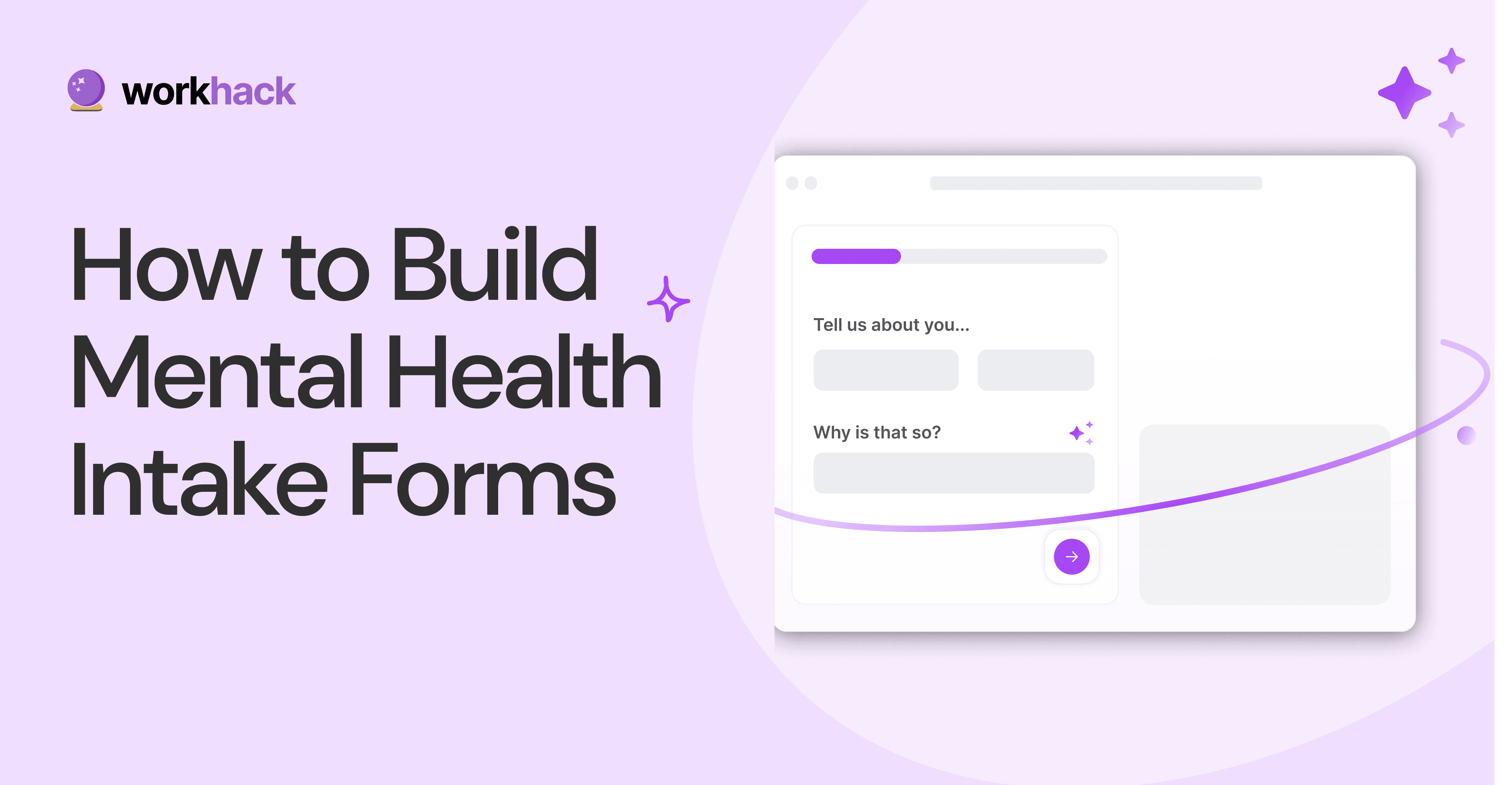
How to Build Mental Health Intake Forms?
Mental health intake forms are not like patient intake forms. Mental health intake forms deal with far more sensitive data and have specific design methods.
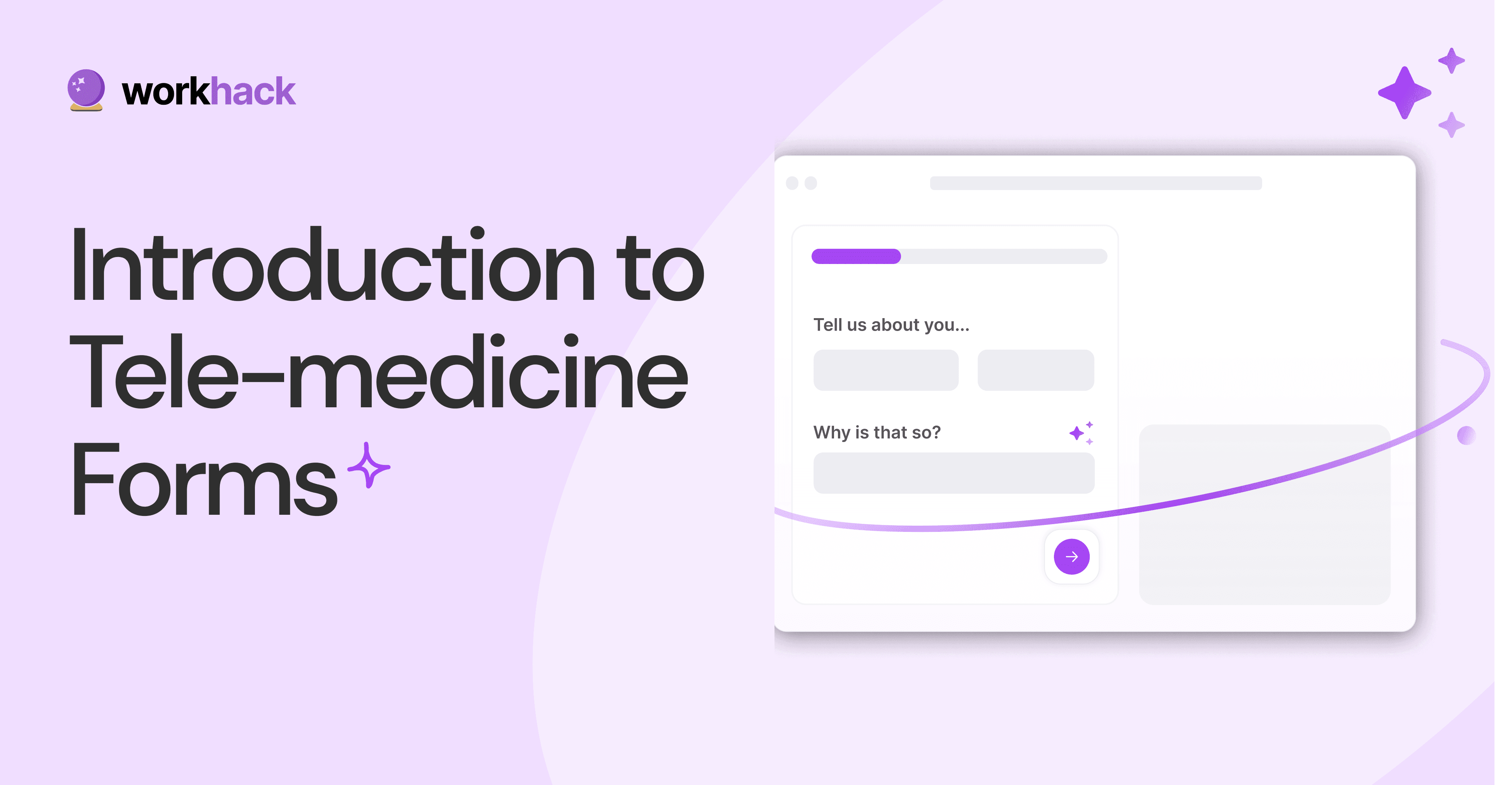
What, Why and How of Telemedicine Forms.
Telemedicine is on the rise and with different form builders out there, which one best suits your needs as a healthcare services provider?
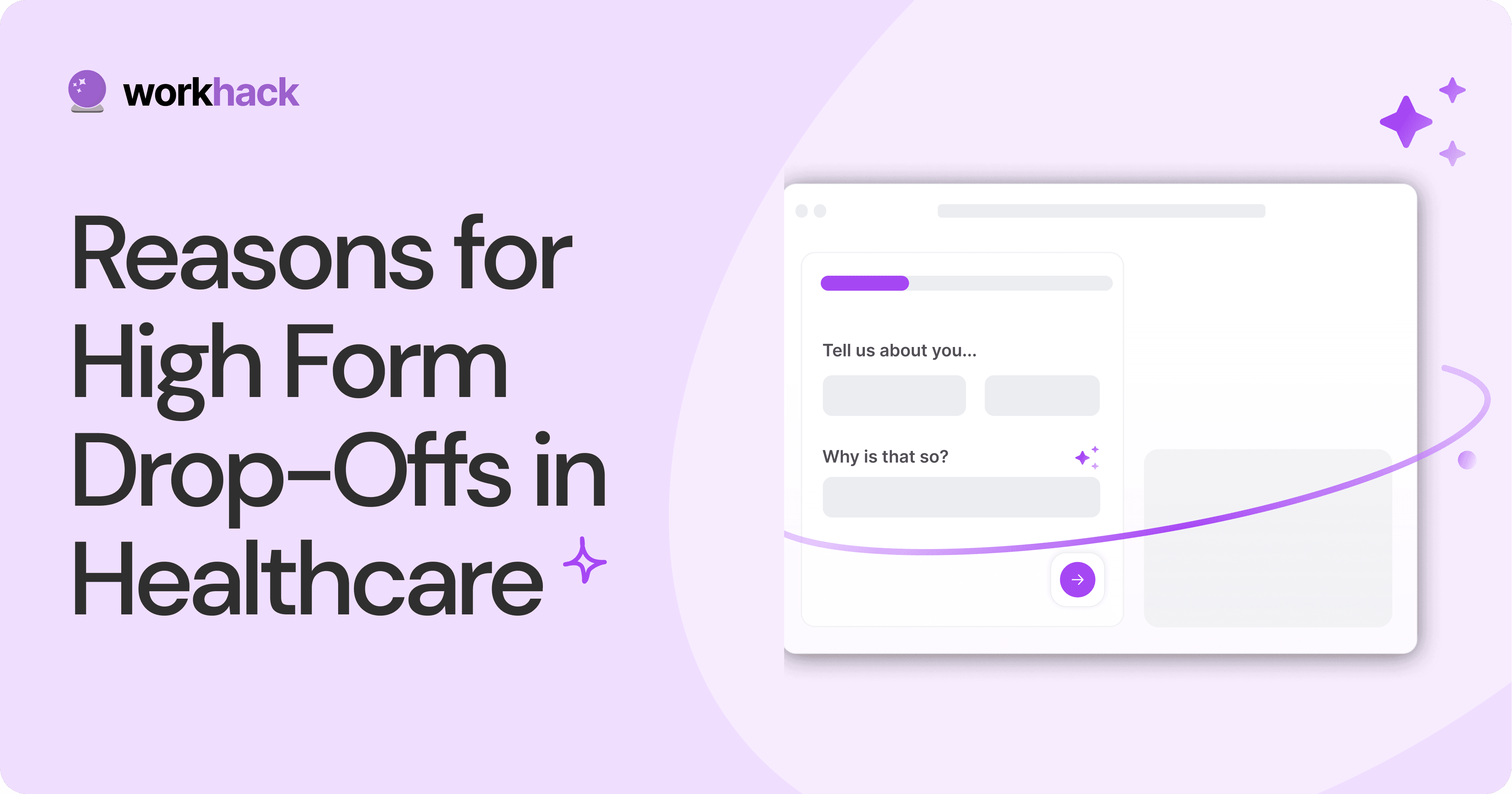
3 Reasons for Major Drop-Offs in Medical Forms.
No matter which healthcare form we pick, there are major drop-off reasons. We shall dive into the top 3 and learn how to resolve them in your next form.
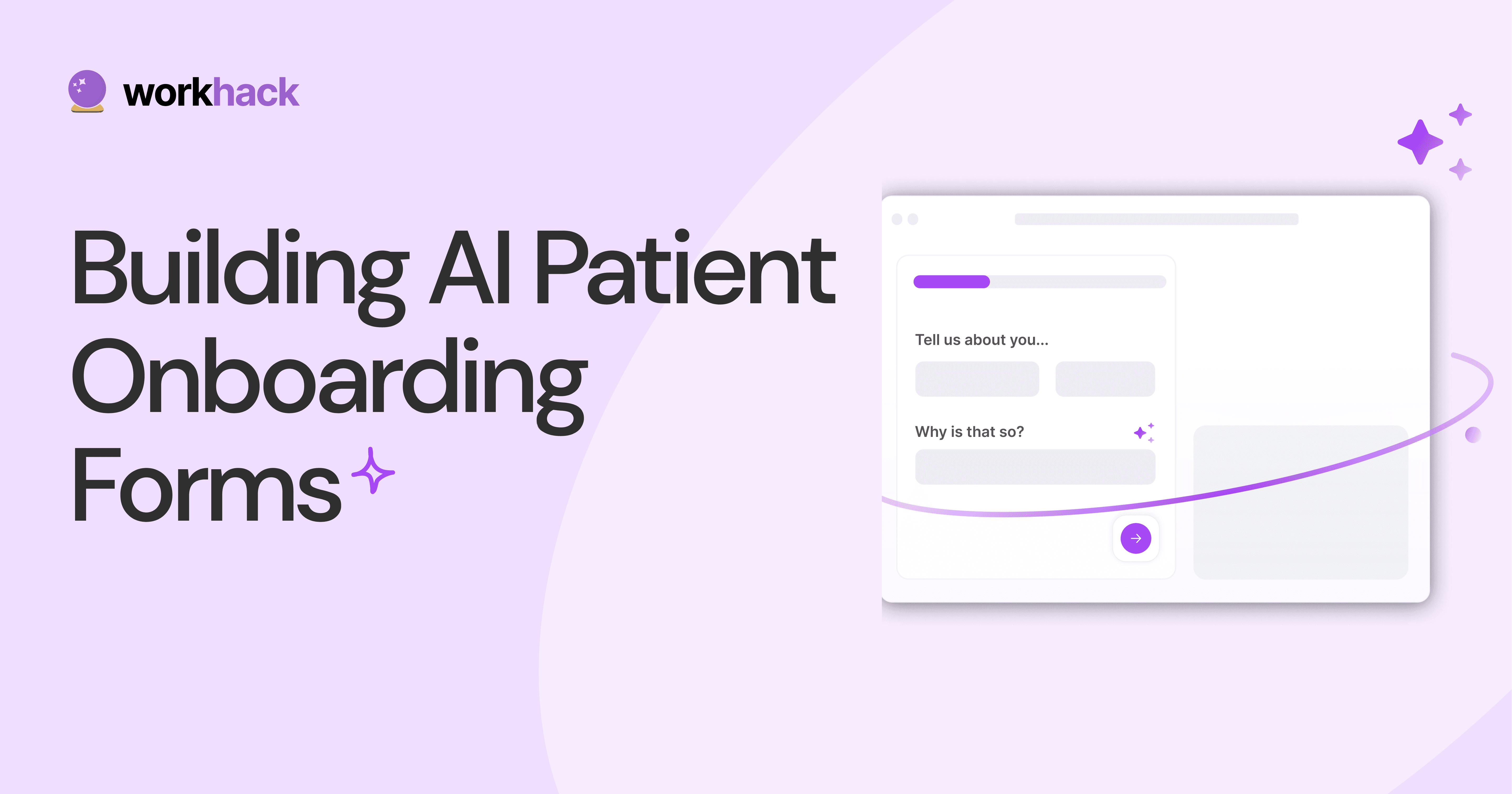
Patient Onboarding Forms - From Click to Clinic.
Patient onboarding forms are the first touchpoint for patients; getting this right for higher conversion rates is a must-have. Learn how to perfect them now.

5 Key Parts of a Good Patient Satisfaction Form.
The goal of patient satisfaction surveys is to course-correct the services of a healthcare provider. Patient feedback leads to a culture of patient-centric care.
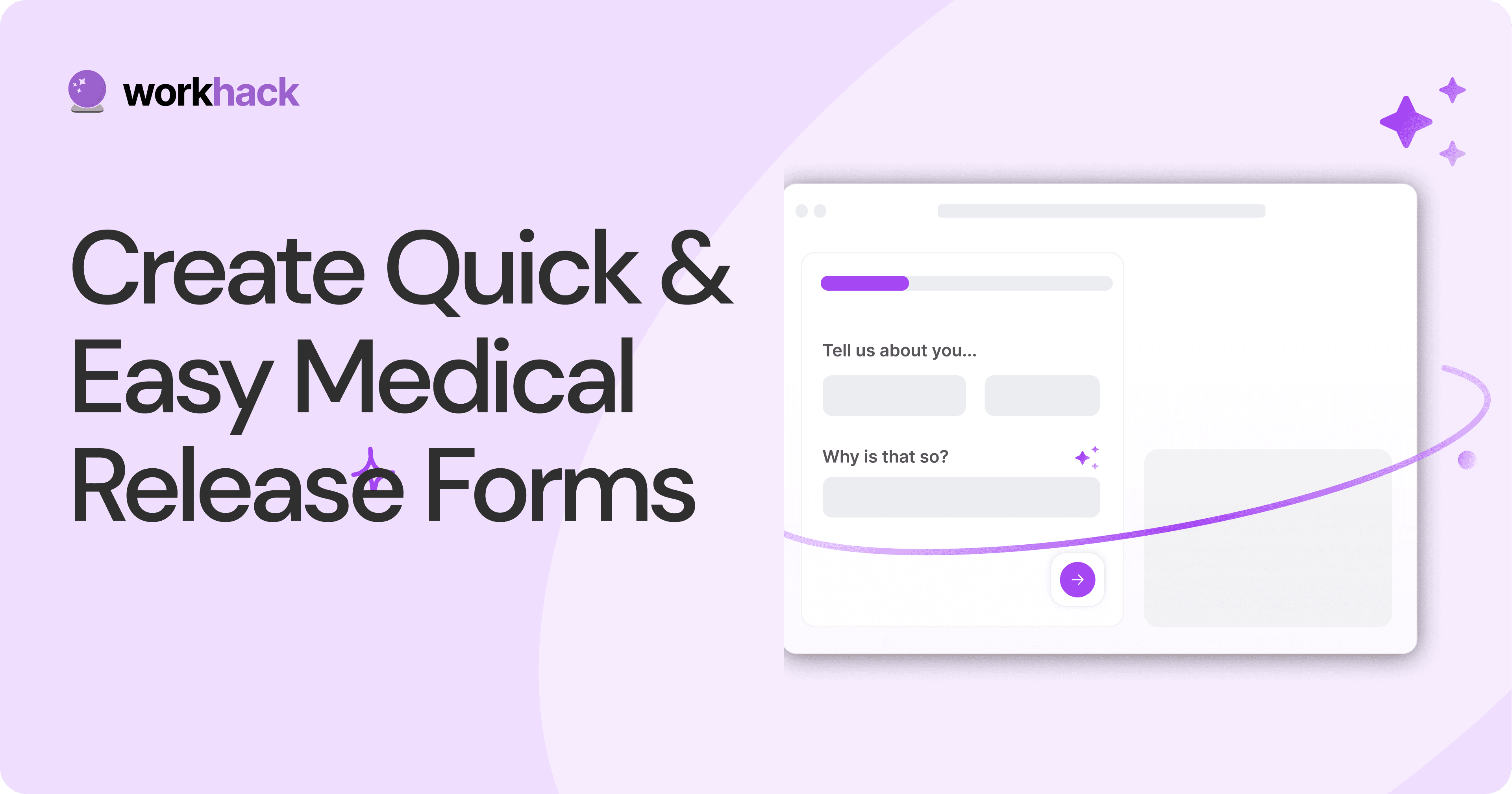
Build Quick and Easy Medical Release Forms.
Every HIPAA-compliant healthcare provider comes across medical release forms that involve details from medical history forms. Can they be shipped fast? Yes.
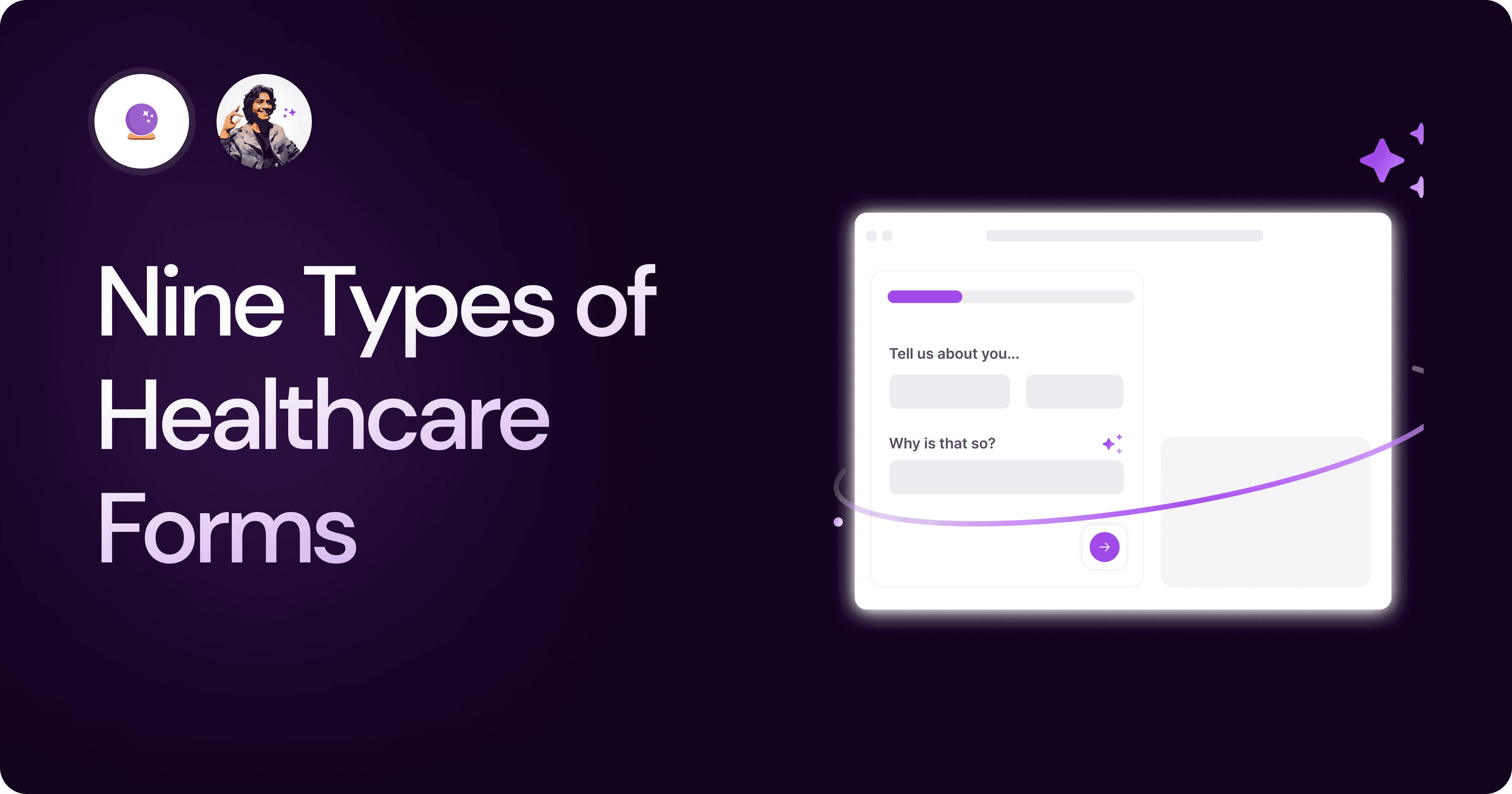
Nine Types of Healthcare and Medical Forms.
Medical forms are a must-have for any healthcare business or practitioner. Learn about the different kinds of medical and healthcare forms.
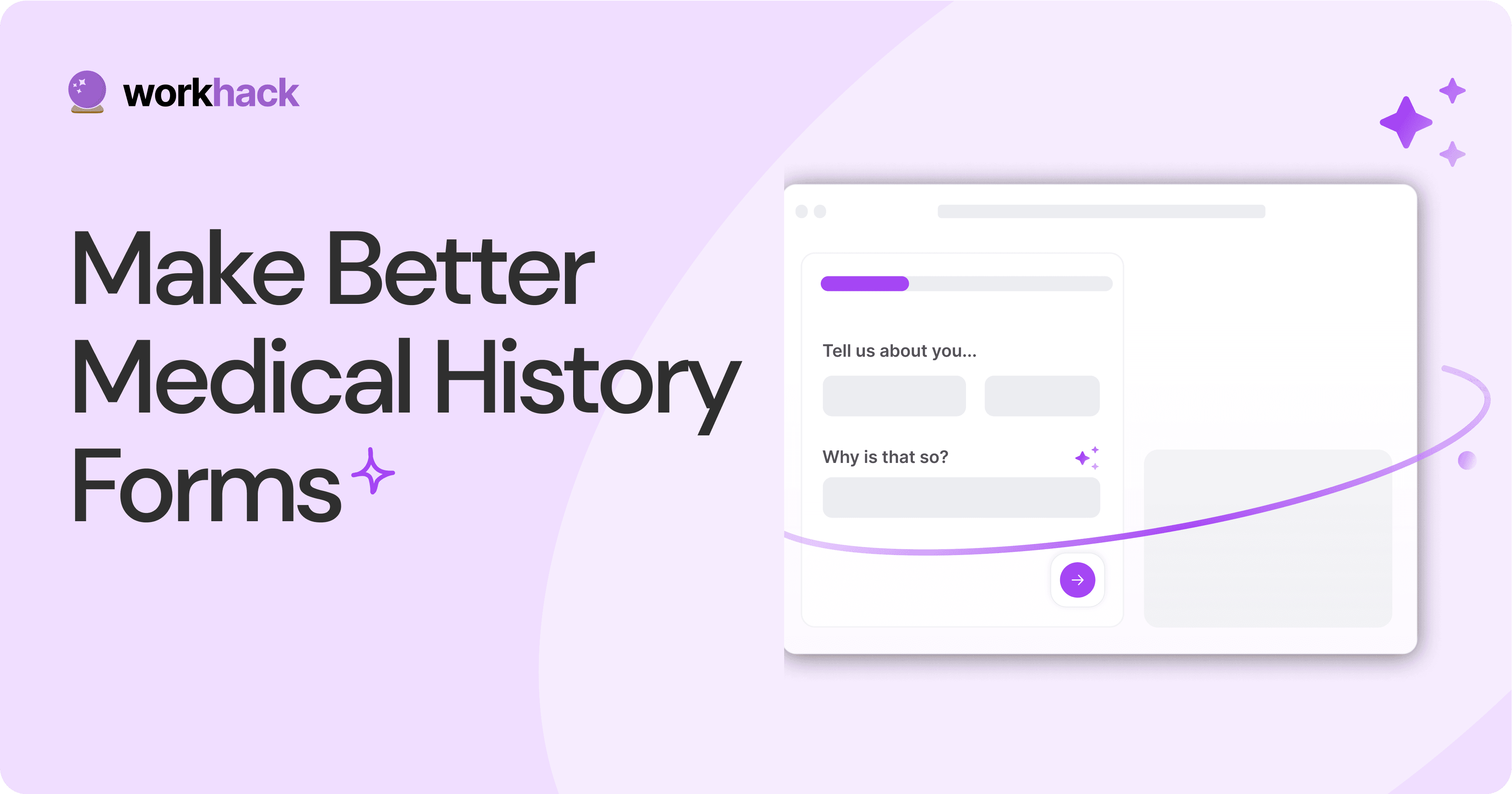
4 Tips for Better Medical History Forms.
Medical history forms are central to patient care, onboarding, and medical administration records. Learn how to make them easier to fill.
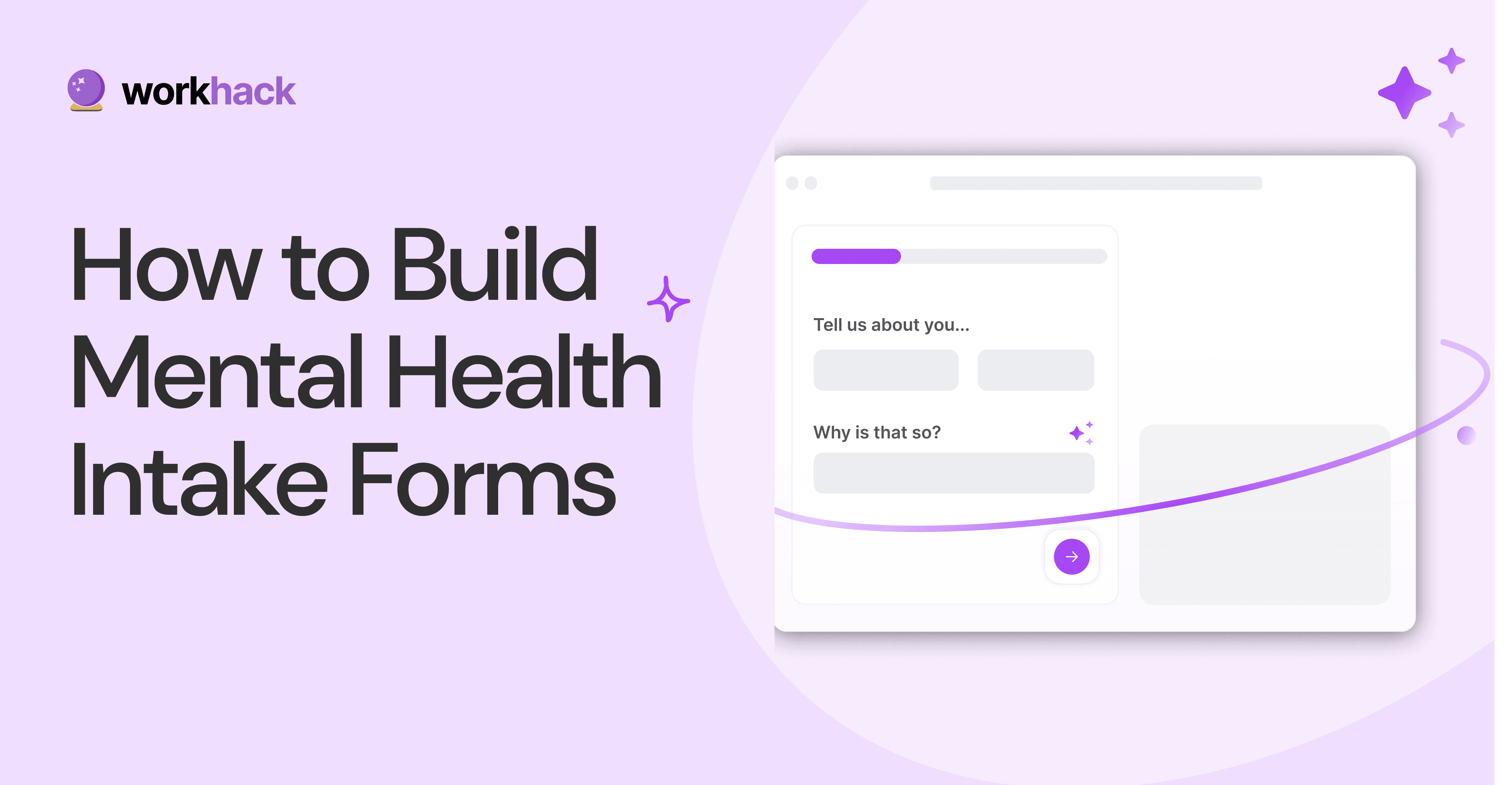
How to Build Mental Health Intake Forms?
Mental health intake forms are not like patient intake forms. Mental health intake forms deal with far more sensitive data and have specific design methods.
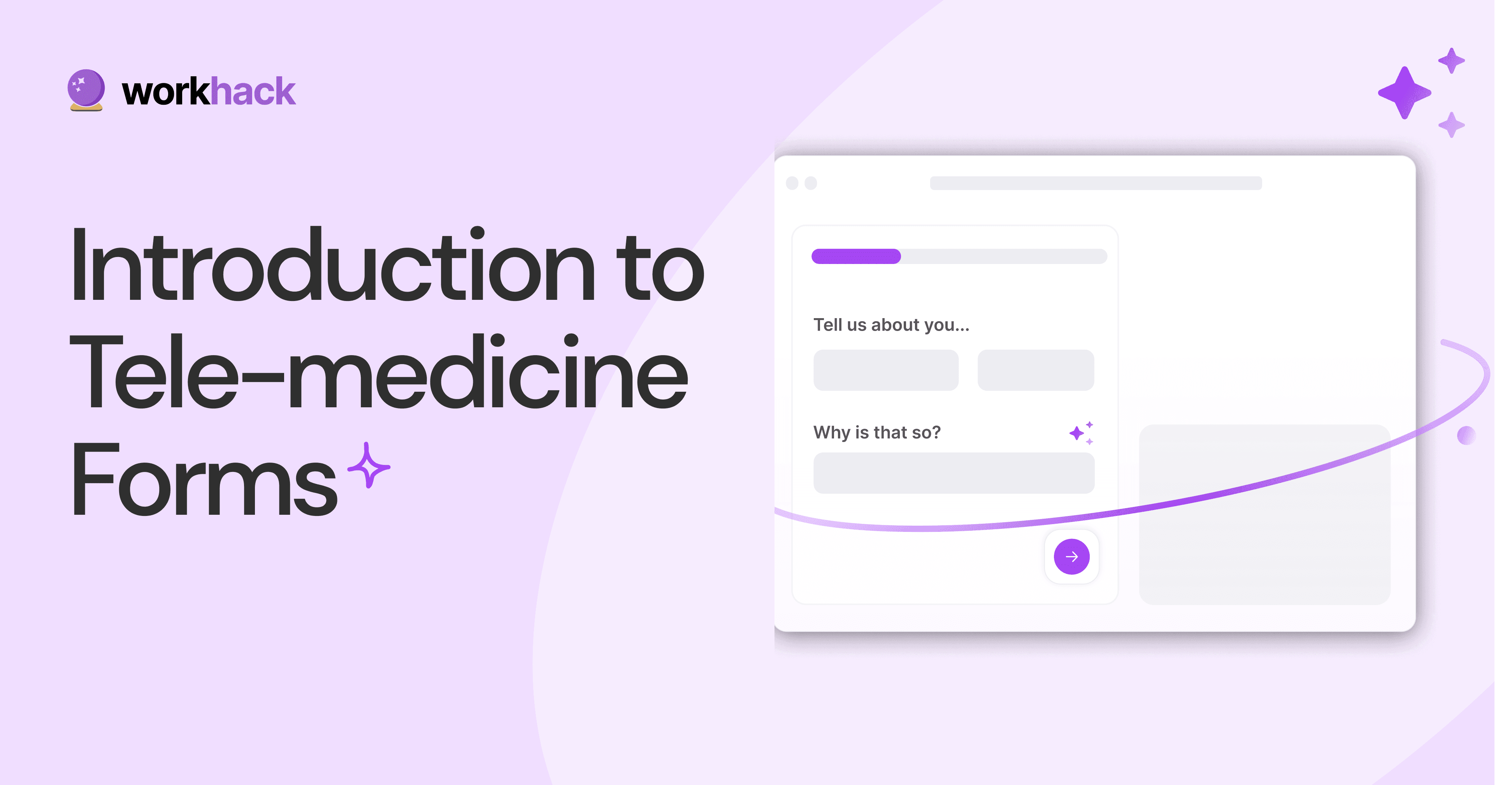
What, Why and How of Telemedicine Forms.
Telemedicine is on the rise and with different form builders out there, which one best suits your needs as a healthcare services provider?
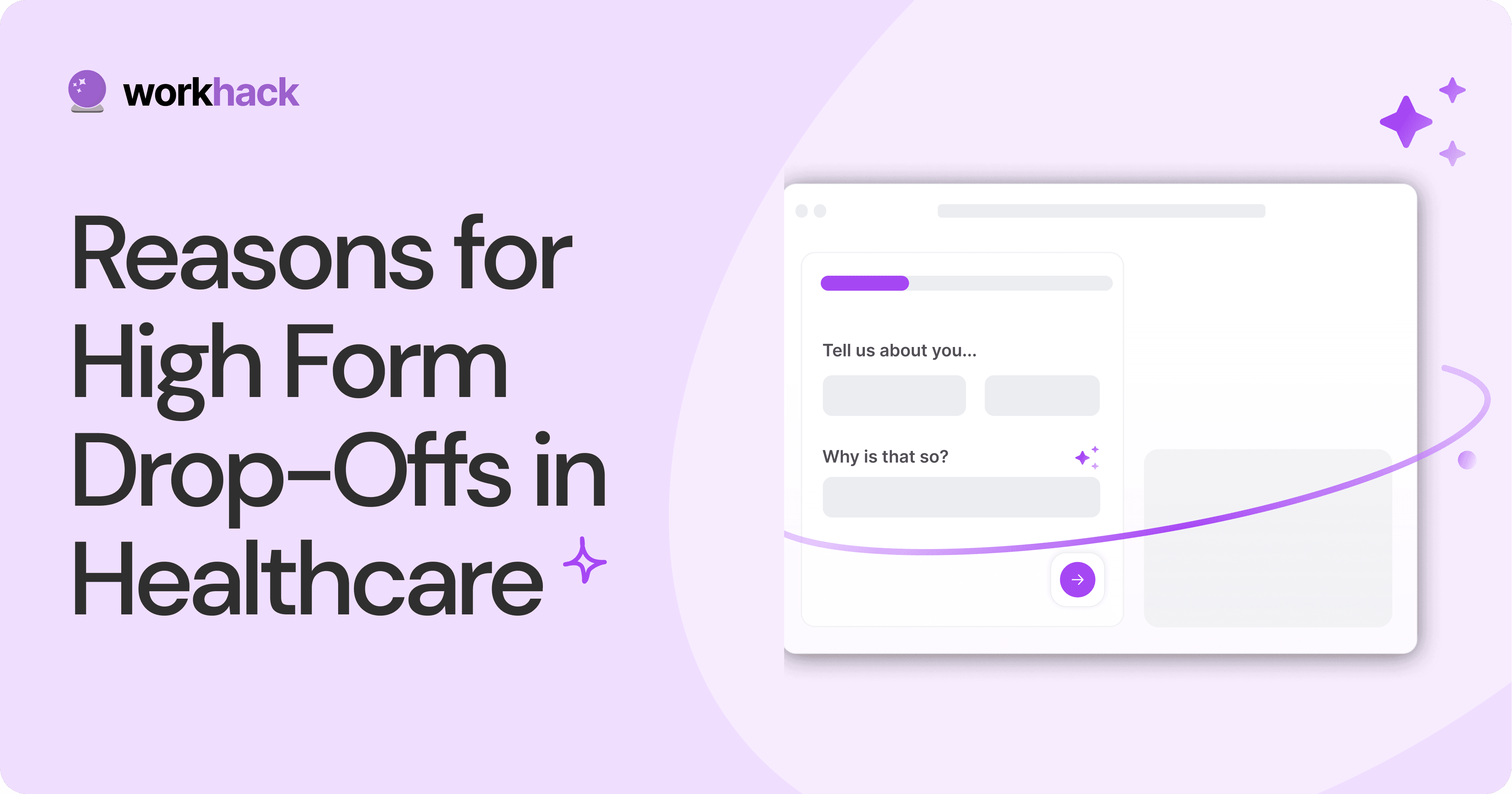
3 Reasons for Major Drop-Offs in Medical Forms.
No matter which healthcare form we pick, there are major drop-off reasons. We shall dive into the top 3 and learn how to resolve them in your next form.
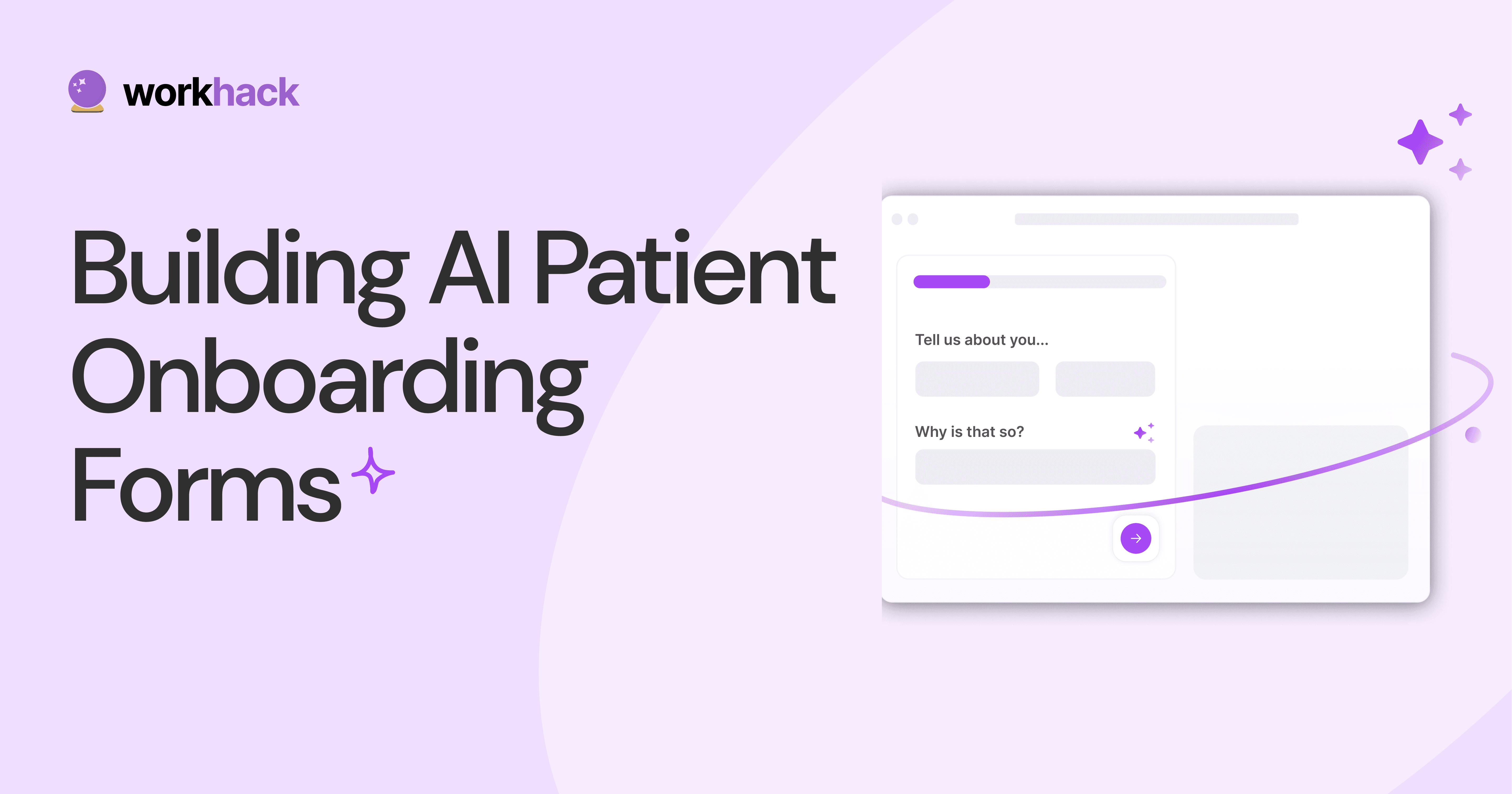
Patient Onboarding Forms - From Click to Clinic.
Patient onboarding forms are the first touchpoint for patients; getting this right for higher conversion rates is a must-have. Learn how to perfect them now.

5 Key Parts of a Good Patient Satisfaction Form.
The goal of patient satisfaction surveys is to course-correct the services of a healthcare provider. Patient feedback leads to a culture of patient-centric care.
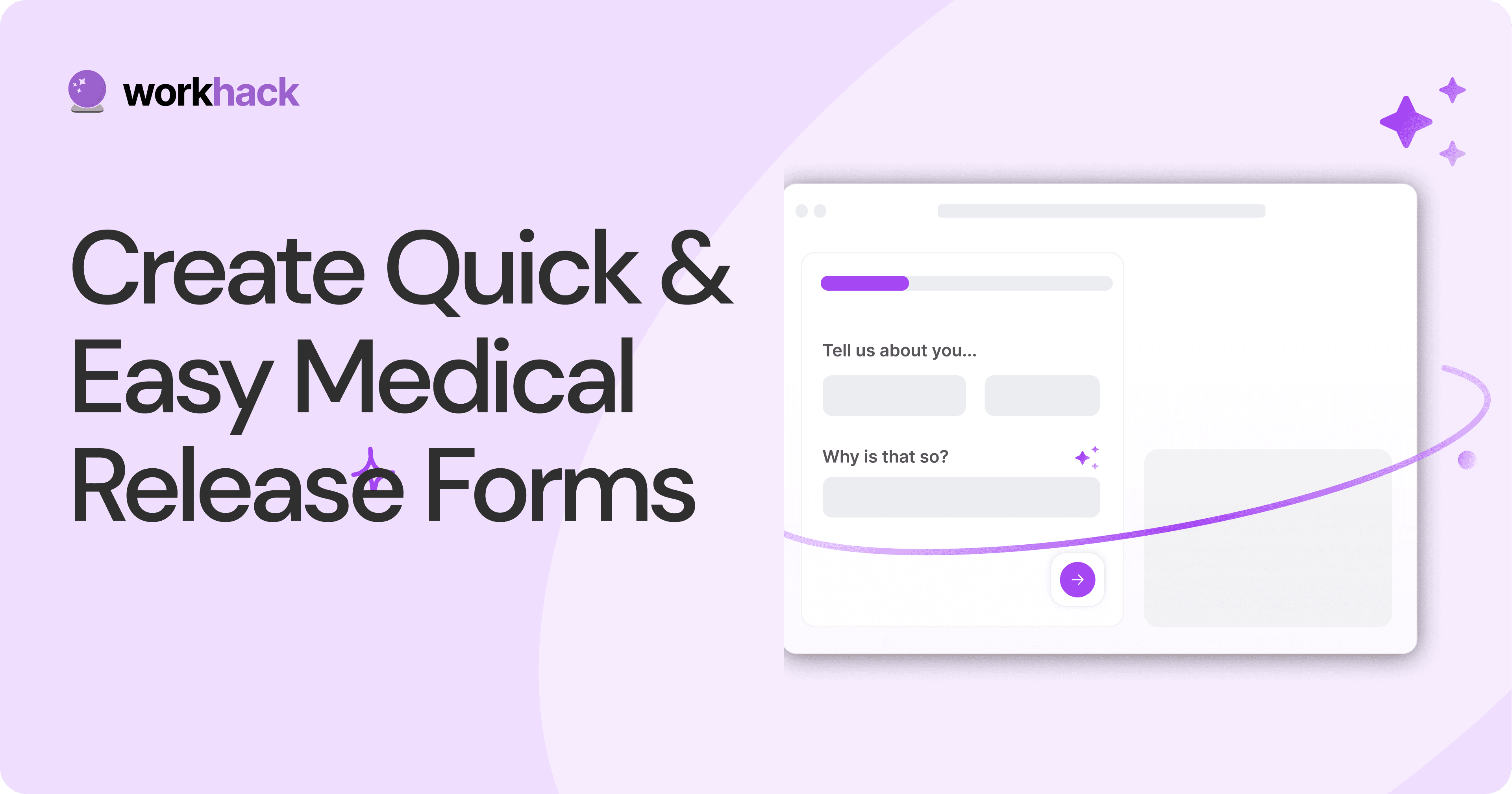
Build Quick and Easy Medical Release Forms.
Every HIPAA-compliant healthcare provider comes across medical release forms that involve details from medical history forms. Can they be shipped fast? Yes.
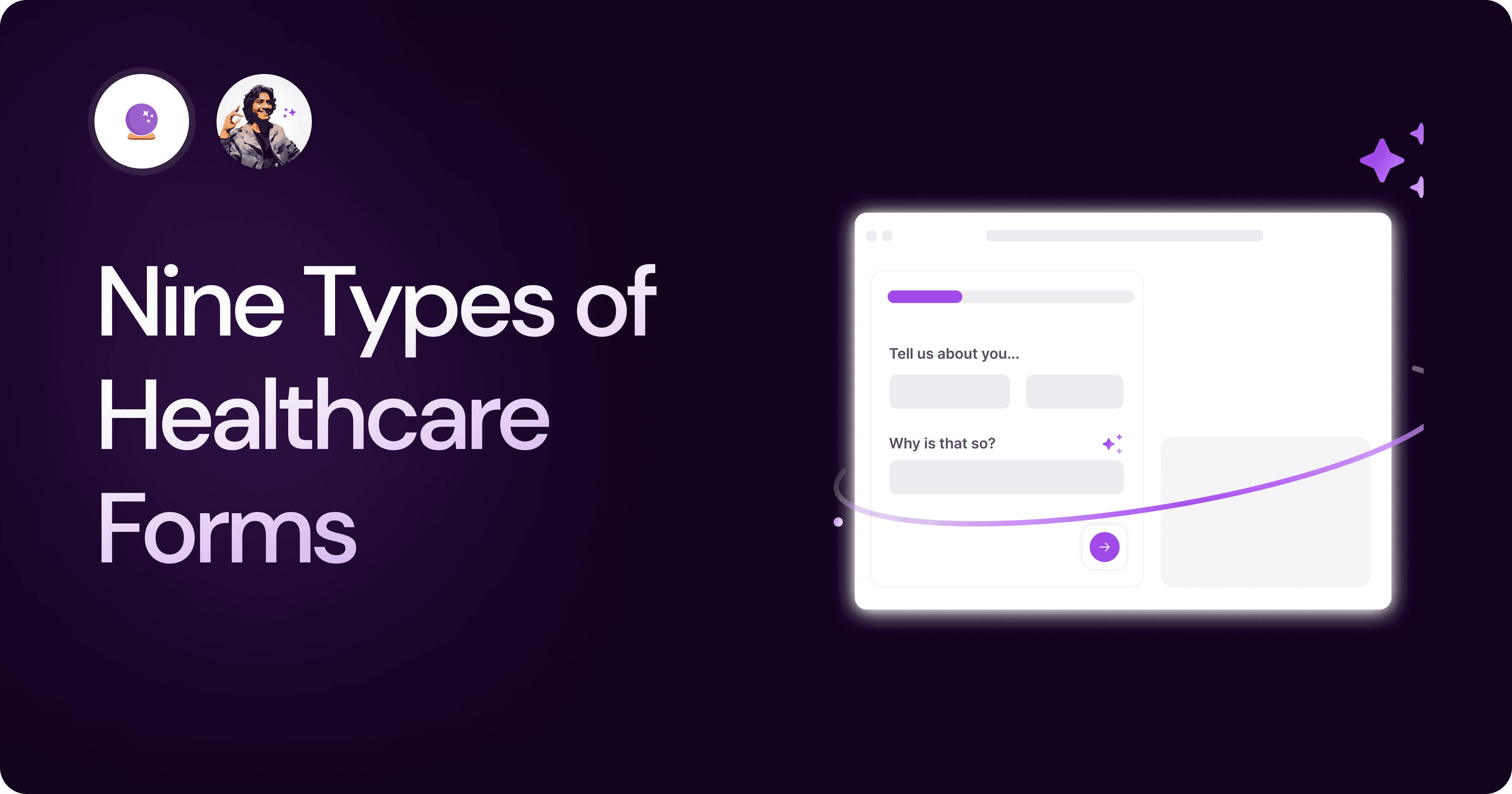
Nine Types of Healthcare and Medical Forms.
Medical forms are a must-have for any healthcare business or practitioner. Learn about the different kinds of medical and healthcare forms.
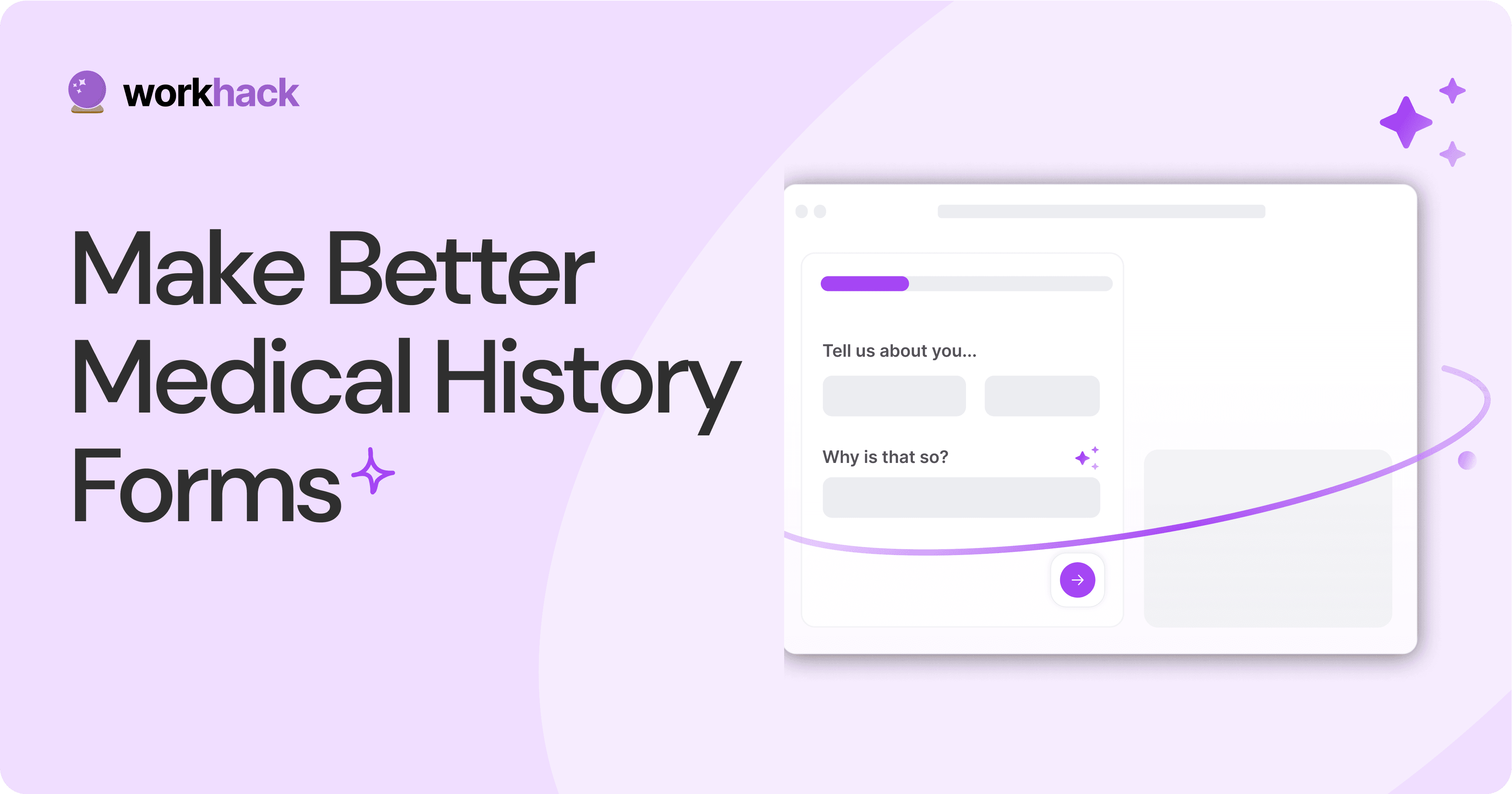
4 Tips for Better Medical History Forms.
Medical history forms are central to patient care, onboarding, and medical administration records. Learn how to make them easier to fill.
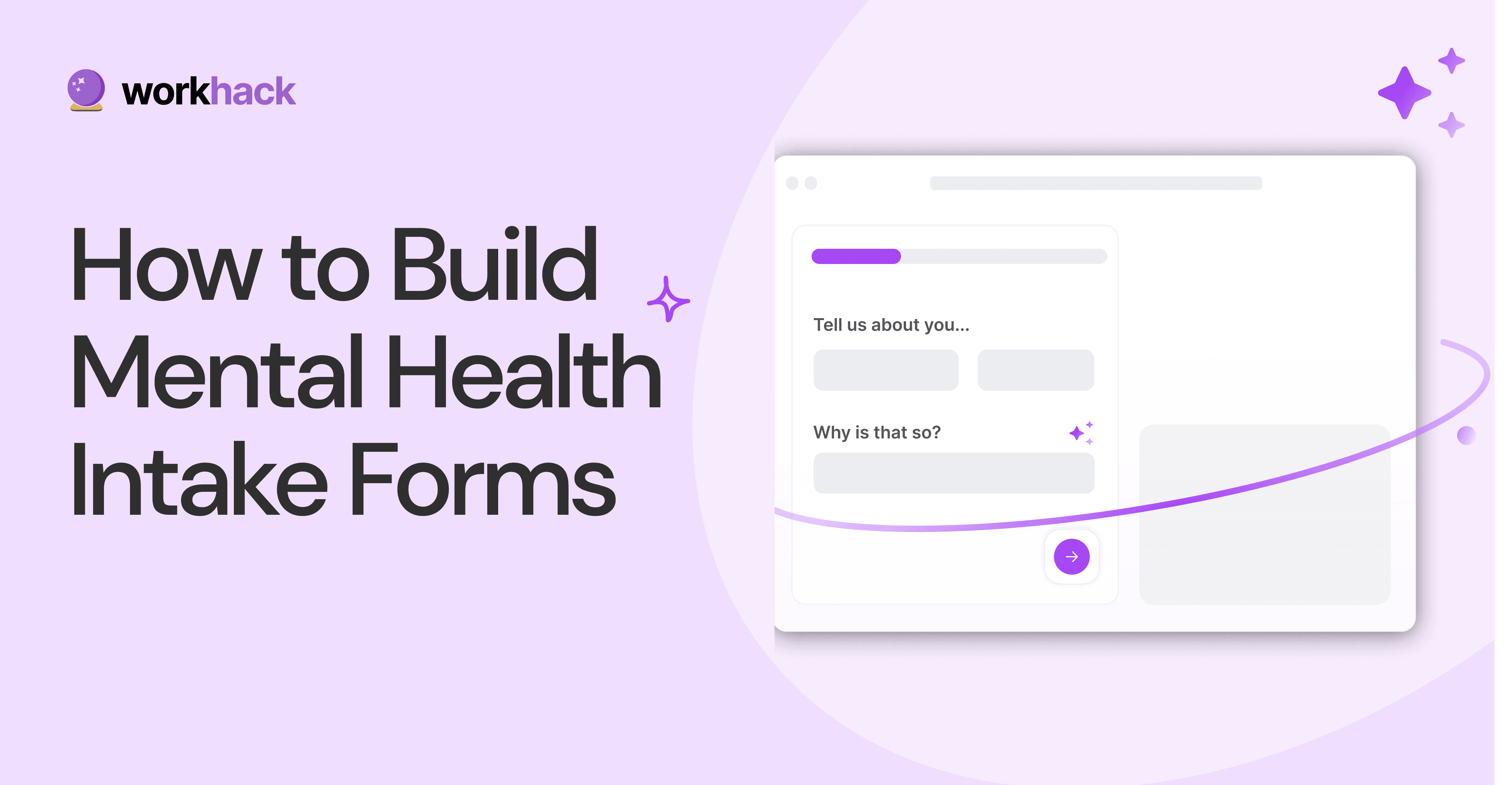
How to Build Mental Health Intake Forms?
Mental health intake forms are not like patient intake forms. Mental health intake forms deal with far more sensitive data and have specific design methods.
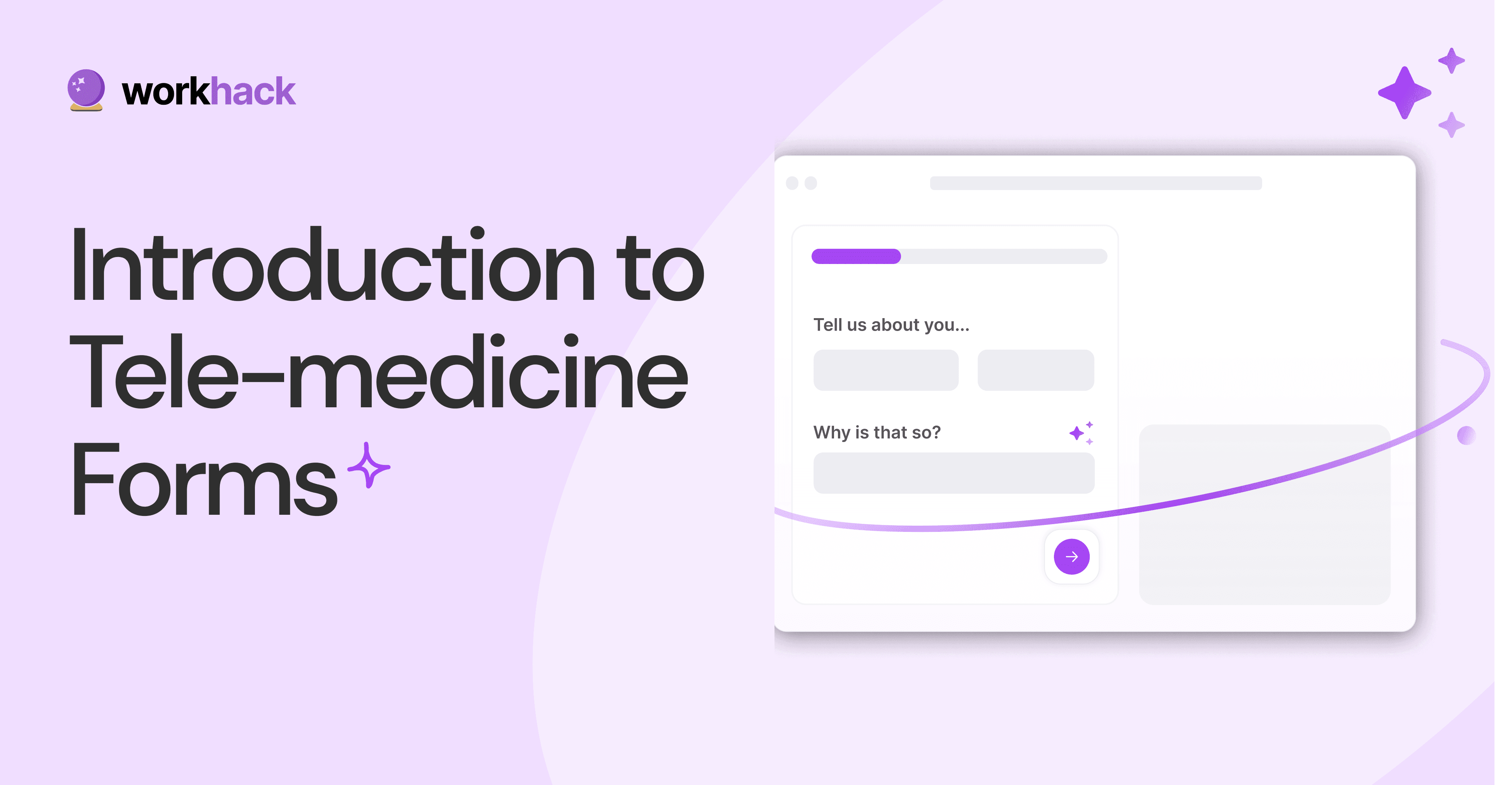
What, Why and How of Telemedicine Forms.
Telemedicine is on the rise and with different form builders out there, which one best suits your needs as a healthcare services provider?
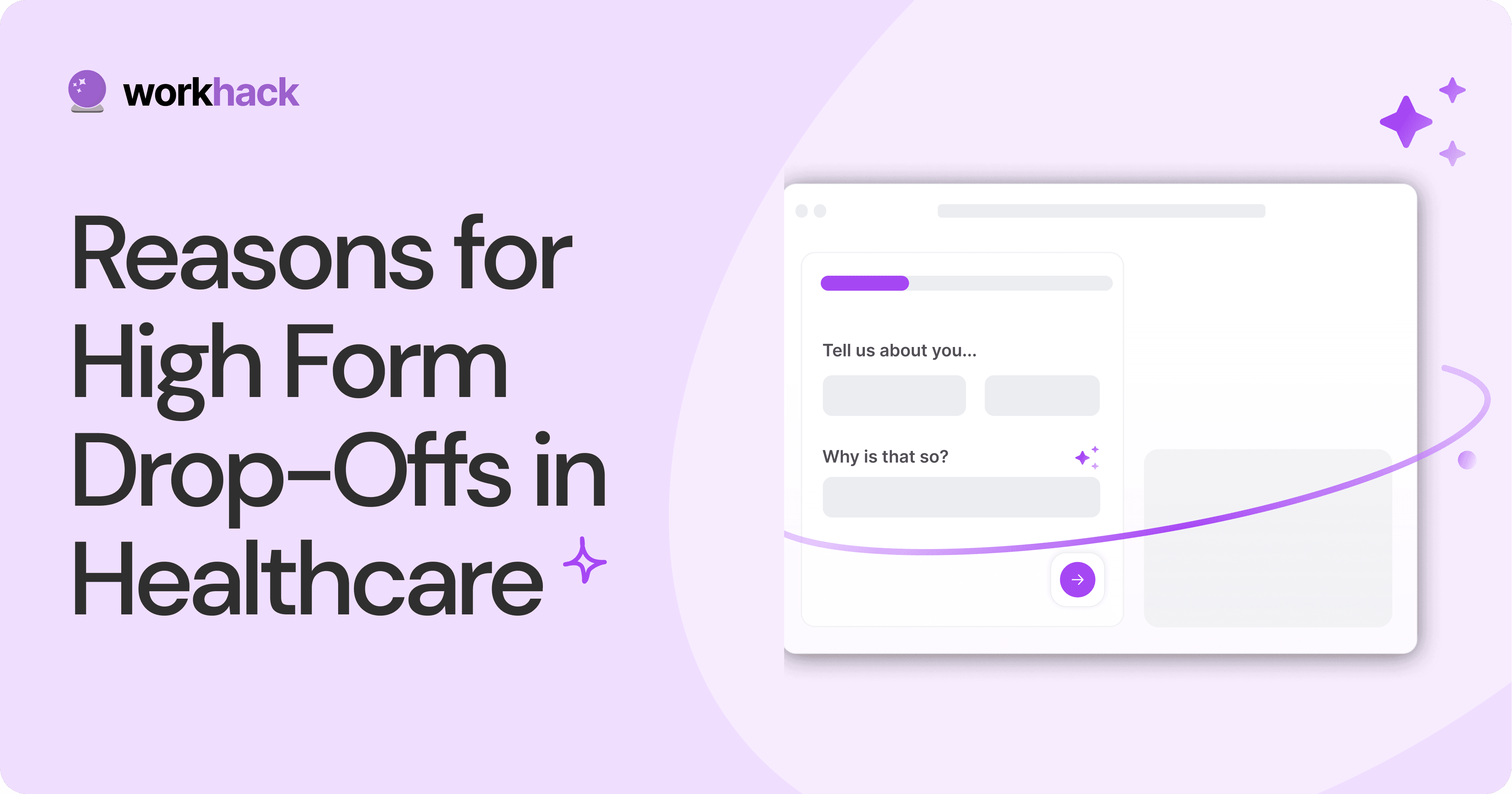
3 Reasons for Major Drop-Offs in Medical Forms.
No matter which healthcare form we pick, there are major drop-off reasons. We shall dive into the top 3 and learn how to resolve them in your next form.
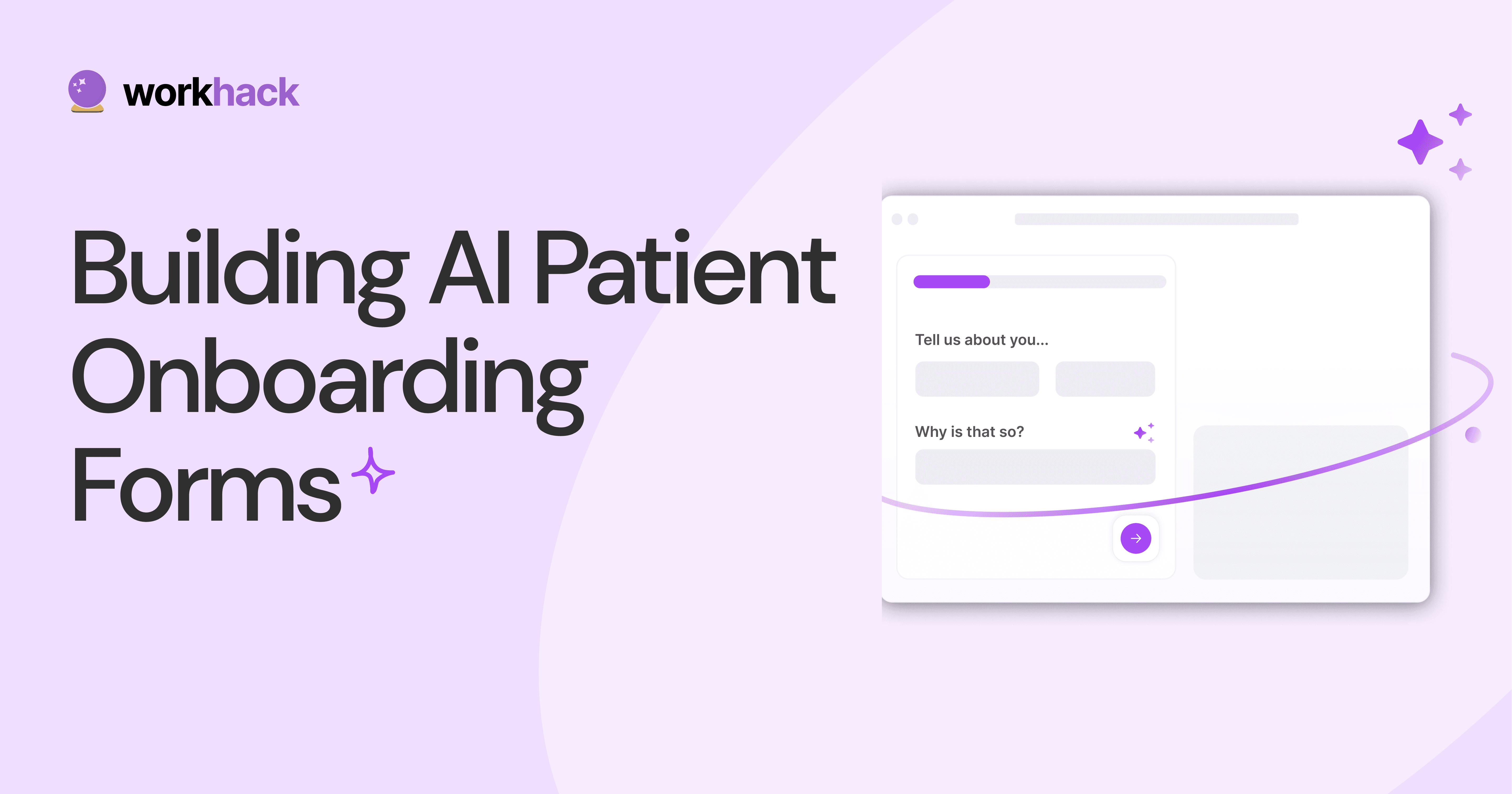
Patient Onboarding Forms - From Click to Clinic.
Patient onboarding forms are the first touchpoint for patients; getting this right for higher conversion rates is a must-have. Learn how to perfect them now.

5 Key Parts of a Good Patient Satisfaction Form.
The goal of patient satisfaction surveys is to course-correct the services of a healthcare provider. Patient feedback leads to a culture of patient-centric care.
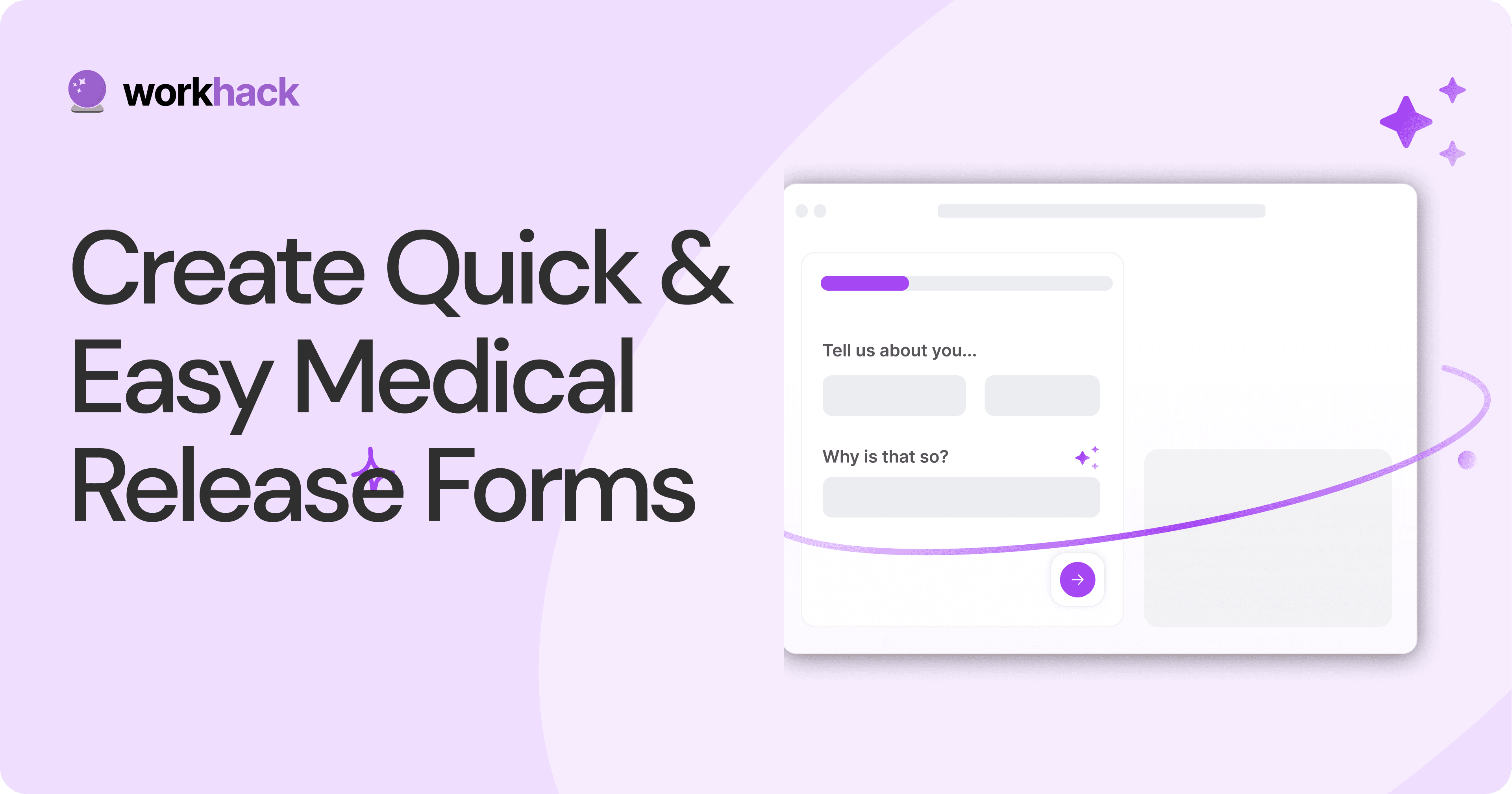
Build Quick and Easy Medical Release Forms.
Every HIPAA-compliant healthcare provider comes across medical release forms that involve details from medical history forms. Can they be shipped fast? Yes.
Subscribe to stay updated.
Subscribe to stay updated.
Subscribe to stay updated.
HC
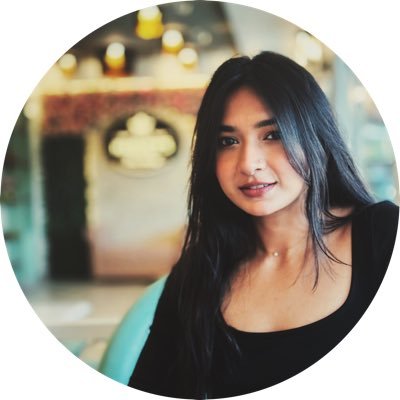
HC
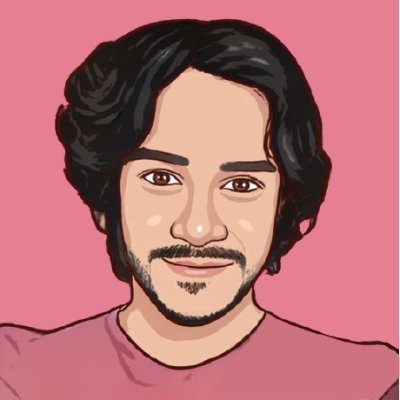
HC
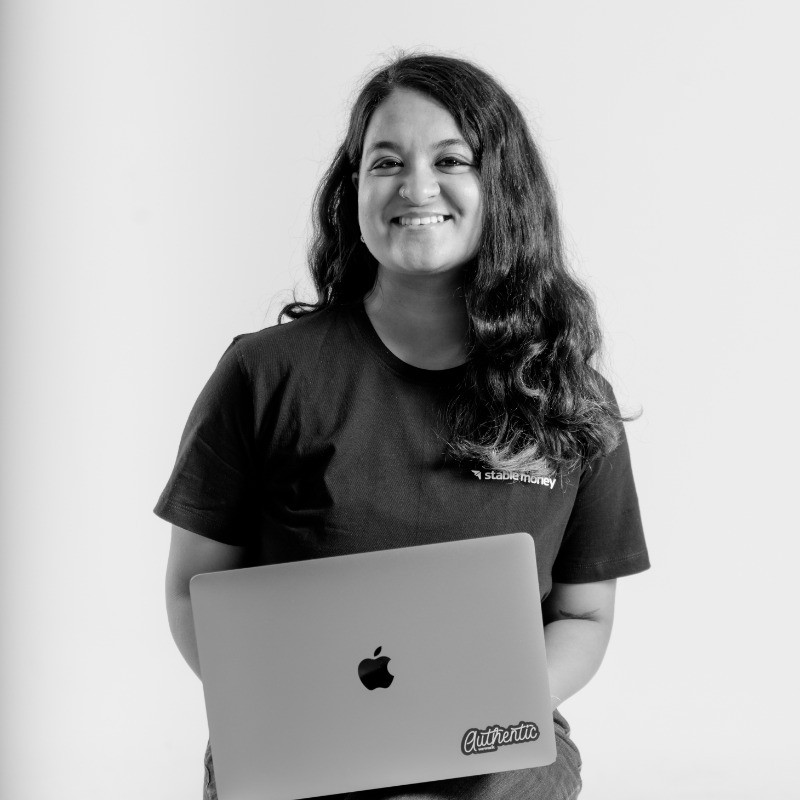
HC
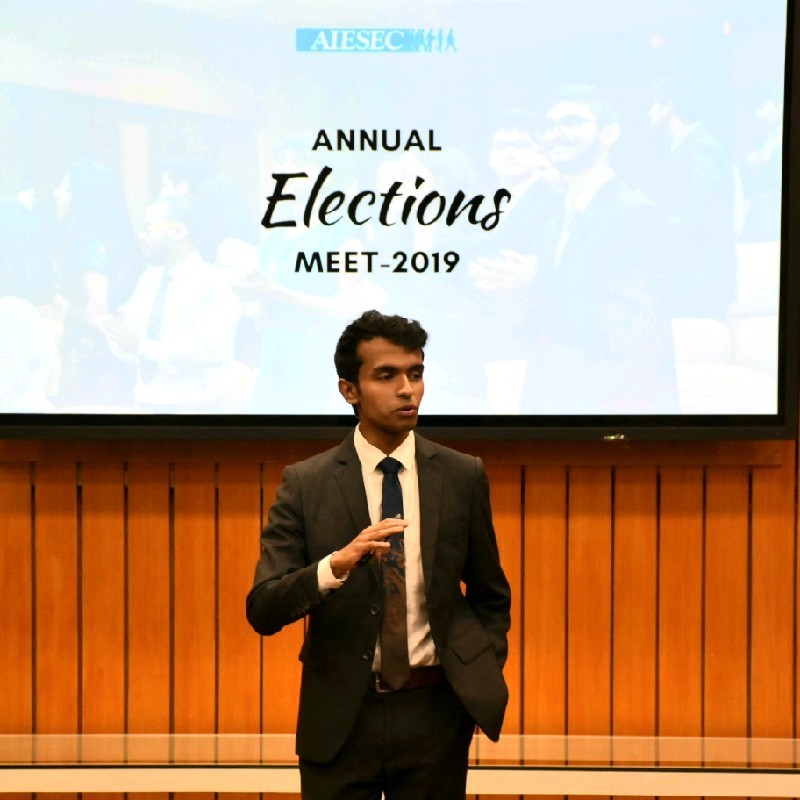
70+ people from across industries read our emails.
HC
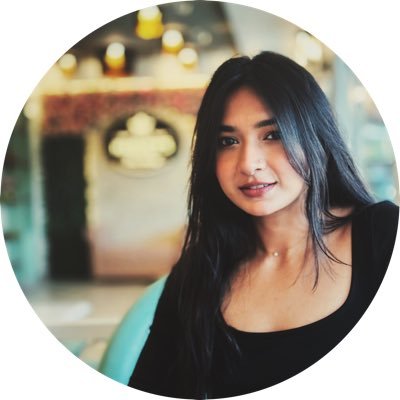
HC
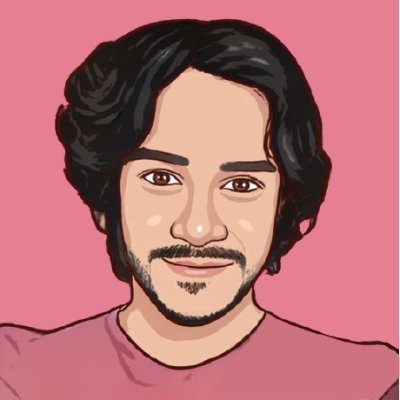
70+ people from across industries read our emails.
HC
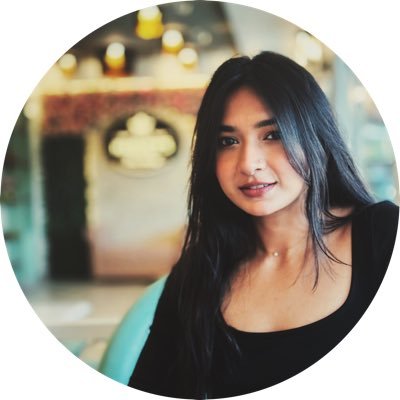
HC
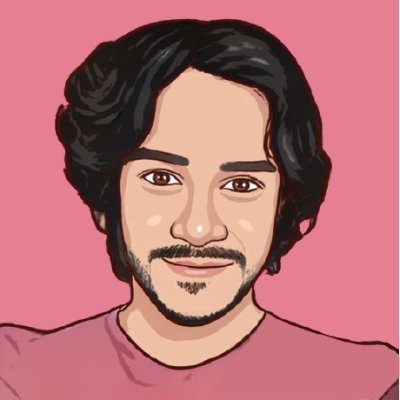
HC
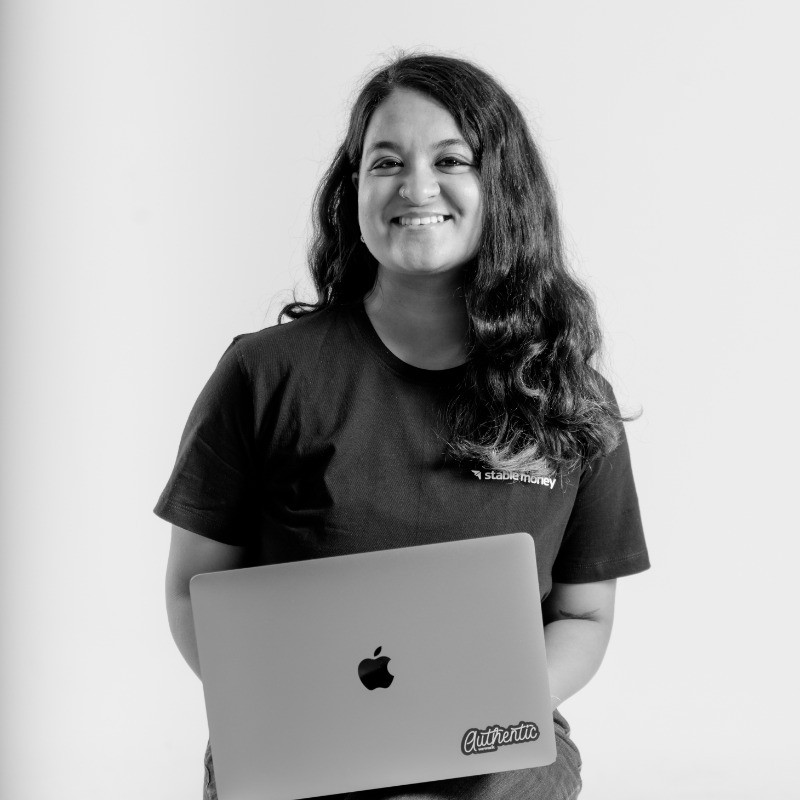
70+ people from across industries read our emails.
Bangalore, India / San Francisco, US
WorkHack Inc. 2023
Bangalore, India
San Francisco, US
WorkHack Inc. 2023
WorkHack Inc. 2023
Bangalore, India / San Francisco, US
WorkHack Inc. 2023
Bangalore, India / San Francisco, US