Strategies for data collection in research
Strategies for data collection in research
Strategies for data collection in research
Explore distinct strategies for data collection in research, understand its purpose and the impact of technology on data quality.
Explore distinct strategies for data collection in research, understand its purpose and the impact of technology on data quality.
Explore distinct strategies for data collection in research, understand its purpose and the impact of technology on data quality.



Data is the lifeblood of research. It provides the foundation for evidence-based decision-making, fuels product development, and ultimately shapes our understanding of the world around us.
Data collection in research is the systematic process of gathering information relevant to a specific research question or hypothesis. This information can come from a variety of sources and can be quantitative (numerical) or qualitative (descriptive).
Why is Data Collection Important in Research?
A well-designed data collection strategy is crucial for the success of any research project. According to a 2023 study by the Harvard Business Review, 85% of executives believe that data-driven decision-making is essential for their business success. Data collection allows researchers to:
Test hypotheses and answer research questions
By collecting relevant data, researchers can validate or disprove their initial assumptions and gain deeper insights into the topic at hand.Inform decision-making
Data provides a clear picture of trends, patterns, and relationships, enabling researchers to make informed decisions about interventions, product development, or resource allocation.Support research findings
High-quality data strengthens the credibility and validity of research results.
Types of Data Collection Methods in Research
There are numerous data collection methods available to researchers, each with its own strengths and weaknesses. The choice of method depends on the research question, the type of data needed, and the resources available. Here's a breakdown of the two main categories:
Primary data collection: This involves gathering new data directly from the source. Common methods include surveys, interviews, focus groups, observations, and experiments.
Secondary data collection: This involves using existing data collected by others. Examples include government reports, academic journals, and industry publications.
Data collection methods can also be classified based on the type of data they produce:
Quantitative methods: These methods focus on collecting numerical data that can be statistically analyzed. Examples include surveys with multiple-choice questions, experiments that measure specific outcomes, and time series analysis of historical data.
Qualitative methods: These methods aim to capture descriptive data, such as people's experiences, opinions, and attitudes. Examples include open-ended interviews, focus groups, and textual analysis of documents or social media posts.
Mixed methods: This approach combines both quantitative and qualitative methods to provide a more comprehensive understanding of the research topic.
Planning Your Data Collection Process in research
The success of your research hinges on a well-crafted data collection strategy. Just like building a house requires a sturdy foundation, meticulous planning in this stage ensures your research project stands on solid ground. Here, we'll move into the intricacies of planning your data collection process, providing high-value tips to empower you as a researcher.
1. Define Your Research Objectives with Laser Focus
Before entering into method selection, take a step back and clearly articulate your research goals. What burning questions are you seeking answers to? Are you trying to understand user behavior for a new website design, or perhaps gauge public opinion on a social policy change?
Having a crystal-clear understanding of your objectives will serve as a compass, guiding every subsequent decision throughout the data collection process.
2. Identify the Data Gems You Need
Once you know your destination (research objectives), you need to identify the specific data treasures that will get you there.
Ask yourself,
What kind of information do I need to answer my research questions?
Is it the number of times a specific feature is used on an app (quantitative), or the in-depth emotions users experience while interacting with it (qualitative)?
Matching your data needs with the appropriate data collection methods is paramount.
Pro Tip: Here's a helpful trick to identify your data requirements. Imagine you're presenting your research findings to a panel of experts. What specific data points would solidify your arguments and convince them of your conclusions? Those are the data gems you need to collect.
3. Selecting the Right Tools for the Job:
The data collection toolbox is brimming with various methods, each with its strengths and weaknesses. Choosing the most suitable method depends on the type of data you need and the resources at your disposal.
For instance, if you're after a quick snapshot of user preferences for a new product design, an online survey with multiple-choice questions might be ideal (quantitative). But if you're aiming to understand the underlying reasons behind those preferences, in-depth interviews that delve into user experiences might be a better choice (qualitative).

4. Setting a Realistic Timeline
Data collection isn't a race to the finish line. Establish a realistic timeline that considers the complexity of your chosen methods and the resources available. Rushing through this stage can lead to errors, incomplete data, and ultimately, compromised research findings.
Here are some factors to consider when setting your timeline:
Number of participants needed for your chosen method (e.g., surveys vs. interviews)
Time required to collect data from each participant (e.g., short online surveys vs. lengthy in-person interviews)
Data analysis and processing time
5. Design Meticulously for Flawless Data Collection
Once you've chosen your methods, it's time to meticulously design the data collection instruments. This might involve crafting a survey with clear and concise questions (keeping the response rate in mind), developing a detailed interview protocol, or creating an observation guide to ensure consistent data recording.
Pilot testing your instruments with a small group beforehand is crucial to identify and iron out any potential kinks.
6. Standardize for Data Collection Consistency
Imagine a delicious recipe where each chef adds a different amount of each ingredient. The resulting dish would be a culinary catastrophe! Similarly, inconsistency in data collection can wreak havoc on your research. Standardization is key.
Ensure all participants or data sources encounter the same procedures and instruments during data collection. This guarantees the reliability and validity of your findings, allowing for accurate comparisons and analysis.
7. Establish Clear Data Collection Procedures
Develop a well-defined roadmap for your data collection journey. This roadmap should outline clear protocols for,
Data Collection: How will data be gathered (e.g., online platform, physical forms)?
Data Storage: Where will the collected data be securely stored?
Data Management: How will the data be organized, labeled, and maintained for easy access and analysis?
8. Plan for the Data Analysis Bonanza
Even the most magnificent collection of gemstones needs to be cut, polished, and arranged to reveal its true brilliance. Similarly, your collected data requires careful analysis to unlock its hidden insights.
Plan ahead for how you will analyze and interpret the data. This might involve choosing appropriate statistical software for quantitative data or thematic analysis techniques for qualitative data.
Exploration of Data Collection Methods in Research
Now that you've mastered the art of planning your data collection process, let's delve into the treasure trove of methods available to researchers. Each method offers unique strengths and considerations, so understanding them empowers you to choose the right tool for the job.

Challenges in Data Collection
The quest for valuable data isn't always a smooth ride. Data collection in research presents a unique set of hurdles that researchers must skillfully navigate to ensure the quality and credibility of their findings. Here, we'll explore some of the most common challenges encountered in data collection:
1. Big Data Environment
The digital age has ushered in an era of Big Data. While vast amounts of data offer exciting possibilities for research, they also present significant challenges.
A 2023 study by IBM found that 95% of organizations experienced more than one breach. Here's why Big Data can be tricky:
Volume: Storing, processing, and analyzing massive datasets requires robust infrastructure and powerful computing resources, which can be expensive for many research projects.
Complexity: Big Data often comes in various formats (structured, unstructured, semi-structured) and can be geographically dispersed. Integrating and harmonizing this data from diverse sources requires sophisticated data management techniques.
Distribution: Data relevant to your research might be scattered across different servers, databases, or even personal devices. Accessing and obtaining the necessary permissions to use this data can be a complex and time-consuming process.
2. Data Bias
Data bias can creep into the research process at various stages, jeopardizing the validity of your findings. Here are two main types of bias to be aware of:
Selection Bias: This occurs when the sample of data you collect is not representative of the larger population you're interested in studying. For instance, if you only survey social media users about a new product, you might miss the perspectives of people who don't use social media.
Measurement Bias: This arises from the way data is collected or measured. For example, poorly worded survey questions or leading interview techniques can influence participants' responses and skew the data.
3. Lack of Quality Assurance Processes
Data quality is the cornerstone of good research. Inaccurate or incomplete data can lead to misleading conclusions. The absence of robust quality assurance processes can leave your data vulnerable to errors:
Data Entry Errors: Typos, inconsistencies, or missing information during data entry can significantly impact the integrity of your dataset.
Inaccurate Measurements: Faulty instruments or imprecise measurement techniques can introduce errors into your data.
Incomplete Data: Missing data points due to participant drop-out or technical glitches can limit the generalizability of your findings.
4. Limited Access to Data
Data privacy concerns are paramount in today's digital landscape. Research involving sensitive data, such as personal health information or financial records, often requires stricter access controls and anonymization techniques. Here's why access to data can be restricted:
Privacy Regulations: Regulations like GDPR (General Data Protection Regulation) in Europe and CCPA (California Consumer Privacy Act) in the US mandate that researchers obtain informed consent from participants and implement measures to protect their data privacy.
Data Sensitivity: Certain types of data, such as medical records, require stricter access controls and anonymization procedures to safeguard confidentiality.
Data Security Concerns: Cybersecurity threats are a constant concern. Researchers must employ robust security measures to protect the data they collect from unauthorized access or breaches.
5. Legal and Compliance Regulations
The legal landscape surrounding data collection can be complex and ever-changing. Researchers must stay informed about relevant regulations to ensure their data collection practices comply. Here are some key considerations:
Privacy Laws: As mentioned earlier, privacy regulations like GDPR and CCPA dictate how data can be collected, stored, and used. Failing to comply with these regulations can result in hefty fines and reputational damage.
Intellectual Property Rights: Using copyrighted data or materials without proper permission can lead to legal trouble. Researchers must be mindful of copyright laws and obtain necessary licenses if required.
Ethical Research Guidelines: Ethical research protocols ensure participant welfare and responsible data collection practices. Researchers must adhere to guidelines established by institutional review boards or relevant professional organizations.
Role of Software and Tools in Data Collection
Pen-and-paper surveys no longer dominate the data collection landscape. Today, researchers have a powerful arsenal of software and tools at their disposal, streamlining processes, enhancing data quality, and empowering them to gather richer insights. Here's how technology is transforming data collection:
1. Utilization of Qualitative Analysis Software to Streamline Data Collection
Software has revolutionized qualitative data analysis, once a time-consuming and laborious process. Tools like NVivo, MAXQDA, and Atlas allow researchers to efficiently code, categorize, and analyze textual data from interviews, focus groups, and open-ended surveys.
These features streamline the data collection process, enabling researchers to identify emerging themes, patterns, and relationships within their data more quickly and effectively.
2. Survey Creation, Customization Features, and Data Analysis Tools
Gone are the days of clunky, paper surveys. Online survey platforms like SurveyMonkey, Google Forms, and Metaforms.ai offer a plethora of features to create engaging and user-friendly surveys.
These platforms often include customization options (question types, branching logic, skip logic), real-time data analysis, and data visualization tools, allowing researchers to easily gather, analyze, and interpret survey data.
3. Impact of Technology in Improving Efficiency and Data Quality
Technology has demonstrably improved the efficiency and quality of data collection. Here's how:
Reduced Costs: Online tools and automated data collection methods can significantly reduce the costs associated with traditional data collection methods (e.g., printing surveys, hiring interviewers).
Increased Response Rates: Engaging survey formats and convenient online access can lead to higher response rates, improving the generalizability of your research findings.
Improved Data Accuracy: Software can help minimize data entry errors and inconsistencies, ensuring the accuracy and reliability of your data.
Enhanced Data Security: Cloud-based platforms often offer robust security features to safeguard sensitive data.
Conclusion
By leveraging the power of software and tools, researchers can embark on data collection with greater confidence and efficiency. Metaforms is at the forefront of this data collection revolution, offering a user-friendly platform specifically designed to streamline qualitative and quantitative research. Visit metaforms to learn more about how we can empower your research journey.
Data is the lifeblood of research. It provides the foundation for evidence-based decision-making, fuels product development, and ultimately shapes our understanding of the world around us.
Data collection in research is the systematic process of gathering information relevant to a specific research question or hypothesis. This information can come from a variety of sources and can be quantitative (numerical) or qualitative (descriptive).
Why is Data Collection Important in Research?
A well-designed data collection strategy is crucial for the success of any research project. According to a 2023 study by the Harvard Business Review, 85% of executives believe that data-driven decision-making is essential for their business success. Data collection allows researchers to:
Test hypotheses and answer research questions
By collecting relevant data, researchers can validate or disprove their initial assumptions and gain deeper insights into the topic at hand.Inform decision-making
Data provides a clear picture of trends, patterns, and relationships, enabling researchers to make informed decisions about interventions, product development, or resource allocation.Support research findings
High-quality data strengthens the credibility and validity of research results.
Types of Data Collection Methods in Research
There are numerous data collection methods available to researchers, each with its own strengths and weaknesses. The choice of method depends on the research question, the type of data needed, and the resources available. Here's a breakdown of the two main categories:
Primary data collection: This involves gathering new data directly from the source. Common methods include surveys, interviews, focus groups, observations, and experiments.
Secondary data collection: This involves using existing data collected by others. Examples include government reports, academic journals, and industry publications.
Data collection methods can also be classified based on the type of data they produce:
Quantitative methods: These methods focus on collecting numerical data that can be statistically analyzed. Examples include surveys with multiple-choice questions, experiments that measure specific outcomes, and time series analysis of historical data.
Qualitative methods: These methods aim to capture descriptive data, such as people's experiences, opinions, and attitudes. Examples include open-ended interviews, focus groups, and textual analysis of documents or social media posts.
Mixed methods: This approach combines both quantitative and qualitative methods to provide a more comprehensive understanding of the research topic.
Planning Your Data Collection Process in research
The success of your research hinges on a well-crafted data collection strategy. Just like building a house requires a sturdy foundation, meticulous planning in this stage ensures your research project stands on solid ground. Here, we'll move into the intricacies of planning your data collection process, providing high-value tips to empower you as a researcher.
1. Define Your Research Objectives with Laser Focus
Before entering into method selection, take a step back and clearly articulate your research goals. What burning questions are you seeking answers to? Are you trying to understand user behavior for a new website design, or perhaps gauge public opinion on a social policy change?
Having a crystal-clear understanding of your objectives will serve as a compass, guiding every subsequent decision throughout the data collection process.
2. Identify the Data Gems You Need
Once you know your destination (research objectives), you need to identify the specific data treasures that will get you there.
Ask yourself,
What kind of information do I need to answer my research questions?
Is it the number of times a specific feature is used on an app (quantitative), or the in-depth emotions users experience while interacting with it (qualitative)?
Matching your data needs with the appropriate data collection methods is paramount.
Pro Tip: Here's a helpful trick to identify your data requirements. Imagine you're presenting your research findings to a panel of experts. What specific data points would solidify your arguments and convince them of your conclusions? Those are the data gems you need to collect.
3. Selecting the Right Tools for the Job:
The data collection toolbox is brimming with various methods, each with its strengths and weaknesses. Choosing the most suitable method depends on the type of data you need and the resources at your disposal.
For instance, if you're after a quick snapshot of user preferences for a new product design, an online survey with multiple-choice questions might be ideal (quantitative). But if you're aiming to understand the underlying reasons behind those preferences, in-depth interviews that delve into user experiences might be a better choice (qualitative).

4. Setting a Realistic Timeline
Data collection isn't a race to the finish line. Establish a realistic timeline that considers the complexity of your chosen methods and the resources available. Rushing through this stage can lead to errors, incomplete data, and ultimately, compromised research findings.
Here are some factors to consider when setting your timeline:
Number of participants needed for your chosen method (e.g., surveys vs. interviews)
Time required to collect data from each participant (e.g., short online surveys vs. lengthy in-person interviews)
Data analysis and processing time
5. Design Meticulously for Flawless Data Collection
Once you've chosen your methods, it's time to meticulously design the data collection instruments. This might involve crafting a survey with clear and concise questions (keeping the response rate in mind), developing a detailed interview protocol, or creating an observation guide to ensure consistent data recording.
Pilot testing your instruments with a small group beforehand is crucial to identify and iron out any potential kinks.
6. Standardize for Data Collection Consistency
Imagine a delicious recipe where each chef adds a different amount of each ingredient. The resulting dish would be a culinary catastrophe! Similarly, inconsistency in data collection can wreak havoc on your research. Standardization is key.
Ensure all participants or data sources encounter the same procedures and instruments during data collection. This guarantees the reliability and validity of your findings, allowing for accurate comparisons and analysis.
7. Establish Clear Data Collection Procedures
Develop a well-defined roadmap for your data collection journey. This roadmap should outline clear protocols for,
Data Collection: How will data be gathered (e.g., online platform, physical forms)?
Data Storage: Where will the collected data be securely stored?
Data Management: How will the data be organized, labeled, and maintained for easy access and analysis?
8. Plan for the Data Analysis Bonanza
Even the most magnificent collection of gemstones needs to be cut, polished, and arranged to reveal its true brilliance. Similarly, your collected data requires careful analysis to unlock its hidden insights.
Plan ahead for how you will analyze and interpret the data. This might involve choosing appropriate statistical software for quantitative data or thematic analysis techniques for qualitative data.
Exploration of Data Collection Methods in Research
Now that you've mastered the art of planning your data collection process, let's delve into the treasure trove of methods available to researchers. Each method offers unique strengths and considerations, so understanding them empowers you to choose the right tool for the job.

Challenges in Data Collection
The quest for valuable data isn't always a smooth ride. Data collection in research presents a unique set of hurdles that researchers must skillfully navigate to ensure the quality and credibility of their findings. Here, we'll explore some of the most common challenges encountered in data collection:
1. Big Data Environment
The digital age has ushered in an era of Big Data. While vast amounts of data offer exciting possibilities for research, they also present significant challenges.
A 2023 study by IBM found that 95% of organizations experienced more than one breach. Here's why Big Data can be tricky:
Volume: Storing, processing, and analyzing massive datasets requires robust infrastructure and powerful computing resources, which can be expensive for many research projects.
Complexity: Big Data often comes in various formats (structured, unstructured, semi-structured) and can be geographically dispersed. Integrating and harmonizing this data from diverse sources requires sophisticated data management techniques.
Distribution: Data relevant to your research might be scattered across different servers, databases, or even personal devices. Accessing and obtaining the necessary permissions to use this data can be a complex and time-consuming process.
2. Data Bias
Data bias can creep into the research process at various stages, jeopardizing the validity of your findings. Here are two main types of bias to be aware of:
Selection Bias: This occurs when the sample of data you collect is not representative of the larger population you're interested in studying. For instance, if you only survey social media users about a new product, you might miss the perspectives of people who don't use social media.
Measurement Bias: This arises from the way data is collected or measured. For example, poorly worded survey questions or leading interview techniques can influence participants' responses and skew the data.
3. Lack of Quality Assurance Processes
Data quality is the cornerstone of good research. Inaccurate or incomplete data can lead to misleading conclusions. The absence of robust quality assurance processes can leave your data vulnerable to errors:
Data Entry Errors: Typos, inconsistencies, or missing information during data entry can significantly impact the integrity of your dataset.
Inaccurate Measurements: Faulty instruments or imprecise measurement techniques can introduce errors into your data.
Incomplete Data: Missing data points due to participant drop-out or technical glitches can limit the generalizability of your findings.
4. Limited Access to Data
Data privacy concerns are paramount in today's digital landscape. Research involving sensitive data, such as personal health information or financial records, often requires stricter access controls and anonymization techniques. Here's why access to data can be restricted:
Privacy Regulations: Regulations like GDPR (General Data Protection Regulation) in Europe and CCPA (California Consumer Privacy Act) in the US mandate that researchers obtain informed consent from participants and implement measures to protect their data privacy.
Data Sensitivity: Certain types of data, such as medical records, require stricter access controls and anonymization procedures to safeguard confidentiality.
Data Security Concerns: Cybersecurity threats are a constant concern. Researchers must employ robust security measures to protect the data they collect from unauthorized access or breaches.
5. Legal and Compliance Regulations
The legal landscape surrounding data collection can be complex and ever-changing. Researchers must stay informed about relevant regulations to ensure their data collection practices comply. Here are some key considerations:
Privacy Laws: As mentioned earlier, privacy regulations like GDPR and CCPA dictate how data can be collected, stored, and used. Failing to comply with these regulations can result in hefty fines and reputational damage.
Intellectual Property Rights: Using copyrighted data or materials without proper permission can lead to legal trouble. Researchers must be mindful of copyright laws and obtain necessary licenses if required.
Ethical Research Guidelines: Ethical research protocols ensure participant welfare and responsible data collection practices. Researchers must adhere to guidelines established by institutional review boards or relevant professional organizations.
Role of Software and Tools in Data Collection
Pen-and-paper surveys no longer dominate the data collection landscape. Today, researchers have a powerful arsenal of software and tools at their disposal, streamlining processes, enhancing data quality, and empowering them to gather richer insights. Here's how technology is transforming data collection:
1. Utilization of Qualitative Analysis Software to Streamline Data Collection
Software has revolutionized qualitative data analysis, once a time-consuming and laborious process. Tools like NVivo, MAXQDA, and Atlas allow researchers to efficiently code, categorize, and analyze textual data from interviews, focus groups, and open-ended surveys.
These features streamline the data collection process, enabling researchers to identify emerging themes, patterns, and relationships within their data more quickly and effectively.
2. Survey Creation, Customization Features, and Data Analysis Tools
Gone are the days of clunky, paper surveys. Online survey platforms like SurveyMonkey, Google Forms, and Metaforms.ai offer a plethora of features to create engaging and user-friendly surveys.
These platforms often include customization options (question types, branching logic, skip logic), real-time data analysis, and data visualization tools, allowing researchers to easily gather, analyze, and interpret survey data.
3. Impact of Technology in Improving Efficiency and Data Quality
Technology has demonstrably improved the efficiency and quality of data collection. Here's how:
Reduced Costs: Online tools and automated data collection methods can significantly reduce the costs associated with traditional data collection methods (e.g., printing surveys, hiring interviewers).
Increased Response Rates: Engaging survey formats and convenient online access can lead to higher response rates, improving the generalizability of your research findings.
Improved Data Accuracy: Software can help minimize data entry errors and inconsistencies, ensuring the accuracy and reliability of your data.
Enhanced Data Security: Cloud-based platforms often offer robust security features to safeguard sensitive data.
Conclusion
By leveraging the power of software and tools, researchers can embark on data collection with greater confidence and efficiency. Metaforms is at the forefront of this data collection revolution, offering a user-friendly platform specifically designed to streamline qualitative and quantitative research. Visit metaforms to learn more about how we can empower your research journey.
Data is the lifeblood of research. It provides the foundation for evidence-based decision-making, fuels product development, and ultimately shapes our understanding of the world around us.
Data collection in research is the systematic process of gathering information relevant to a specific research question or hypothesis. This information can come from a variety of sources and can be quantitative (numerical) or qualitative (descriptive).
Why is Data Collection Important in Research?
A well-designed data collection strategy is crucial for the success of any research project. According to a 2023 study by the Harvard Business Review, 85% of executives believe that data-driven decision-making is essential for their business success. Data collection allows researchers to:
Test hypotheses and answer research questions
By collecting relevant data, researchers can validate or disprove their initial assumptions and gain deeper insights into the topic at hand.Inform decision-making
Data provides a clear picture of trends, patterns, and relationships, enabling researchers to make informed decisions about interventions, product development, or resource allocation.Support research findings
High-quality data strengthens the credibility and validity of research results.
Types of Data Collection Methods in Research
There are numerous data collection methods available to researchers, each with its own strengths and weaknesses. The choice of method depends on the research question, the type of data needed, and the resources available. Here's a breakdown of the two main categories:
Primary data collection: This involves gathering new data directly from the source. Common methods include surveys, interviews, focus groups, observations, and experiments.
Secondary data collection: This involves using existing data collected by others. Examples include government reports, academic journals, and industry publications.
Data collection methods can also be classified based on the type of data they produce:
Quantitative methods: These methods focus on collecting numerical data that can be statistically analyzed. Examples include surveys with multiple-choice questions, experiments that measure specific outcomes, and time series analysis of historical data.
Qualitative methods: These methods aim to capture descriptive data, such as people's experiences, opinions, and attitudes. Examples include open-ended interviews, focus groups, and textual analysis of documents or social media posts.
Mixed methods: This approach combines both quantitative and qualitative methods to provide a more comprehensive understanding of the research topic.
Planning Your Data Collection Process in research
The success of your research hinges on a well-crafted data collection strategy. Just like building a house requires a sturdy foundation, meticulous planning in this stage ensures your research project stands on solid ground. Here, we'll move into the intricacies of planning your data collection process, providing high-value tips to empower you as a researcher.
1. Define Your Research Objectives with Laser Focus
Before entering into method selection, take a step back and clearly articulate your research goals. What burning questions are you seeking answers to? Are you trying to understand user behavior for a new website design, or perhaps gauge public opinion on a social policy change?
Having a crystal-clear understanding of your objectives will serve as a compass, guiding every subsequent decision throughout the data collection process.
2. Identify the Data Gems You Need
Once you know your destination (research objectives), you need to identify the specific data treasures that will get you there.
Ask yourself,
What kind of information do I need to answer my research questions?
Is it the number of times a specific feature is used on an app (quantitative), or the in-depth emotions users experience while interacting with it (qualitative)?
Matching your data needs with the appropriate data collection methods is paramount.
Pro Tip: Here's a helpful trick to identify your data requirements. Imagine you're presenting your research findings to a panel of experts. What specific data points would solidify your arguments and convince them of your conclusions? Those are the data gems you need to collect.
3. Selecting the Right Tools for the Job:
The data collection toolbox is brimming with various methods, each with its strengths and weaknesses. Choosing the most suitable method depends on the type of data you need and the resources at your disposal.
For instance, if you're after a quick snapshot of user preferences for a new product design, an online survey with multiple-choice questions might be ideal (quantitative). But if you're aiming to understand the underlying reasons behind those preferences, in-depth interviews that delve into user experiences might be a better choice (qualitative).

4. Setting a Realistic Timeline
Data collection isn't a race to the finish line. Establish a realistic timeline that considers the complexity of your chosen methods and the resources available. Rushing through this stage can lead to errors, incomplete data, and ultimately, compromised research findings.
Here are some factors to consider when setting your timeline:
Number of participants needed for your chosen method (e.g., surveys vs. interviews)
Time required to collect data from each participant (e.g., short online surveys vs. lengthy in-person interviews)
Data analysis and processing time
5. Design Meticulously for Flawless Data Collection
Once you've chosen your methods, it's time to meticulously design the data collection instruments. This might involve crafting a survey with clear and concise questions (keeping the response rate in mind), developing a detailed interview protocol, or creating an observation guide to ensure consistent data recording.
Pilot testing your instruments with a small group beforehand is crucial to identify and iron out any potential kinks.
6. Standardize for Data Collection Consistency
Imagine a delicious recipe where each chef adds a different amount of each ingredient. The resulting dish would be a culinary catastrophe! Similarly, inconsistency in data collection can wreak havoc on your research. Standardization is key.
Ensure all participants or data sources encounter the same procedures and instruments during data collection. This guarantees the reliability and validity of your findings, allowing for accurate comparisons and analysis.
7. Establish Clear Data Collection Procedures
Develop a well-defined roadmap for your data collection journey. This roadmap should outline clear protocols for,
Data Collection: How will data be gathered (e.g., online platform, physical forms)?
Data Storage: Where will the collected data be securely stored?
Data Management: How will the data be organized, labeled, and maintained for easy access and analysis?
8. Plan for the Data Analysis Bonanza
Even the most magnificent collection of gemstones needs to be cut, polished, and arranged to reveal its true brilliance. Similarly, your collected data requires careful analysis to unlock its hidden insights.
Plan ahead for how you will analyze and interpret the data. This might involve choosing appropriate statistical software for quantitative data or thematic analysis techniques for qualitative data.
Exploration of Data Collection Methods in Research
Now that you've mastered the art of planning your data collection process, let's delve into the treasure trove of methods available to researchers. Each method offers unique strengths and considerations, so understanding them empowers you to choose the right tool for the job.

Challenges in Data Collection
The quest for valuable data isn't always a smooth ride. Data collection in research presents a unique set of hurdles that researchers must skillfully navigate to ensure the quality and credibility of their findings. Here, we'll explore some of the most common challenges encountered in data collection:
1. Big Data Environment
The digital age has ushered in an era of Big Data. While vast amounts of data offer exciting possibilities for research, they also present significant challenges.
A 2023 study by IBM found that 95% of organizations experienced more than one breach. Here's why Big Data can be tricky:
Volume: Storing, processing, and analyzing massive datasets requires robust infrastructure and powerful computing resources, which can be expensive for many research projects.
Complexity: Big Data often comes in various formats (structured, unstructured, semi-structured) and can be geographically dispersed. Integrating and harmonizing this data from diverse sources requires sophisticated data management techniques.
Distribution: Data relevant to your research might be scattered across different servers, databases, or even personal devices. Accessing and obtaining the necessary permissions to use this data can be a complex and time-consuming process.
2. Data Bias
Data bias can creep into the research process at various stages, jeopardizing the validity of your findings. Here are two main types of bias to be aware of:
Selection Bias: This occurs when the sample of data you collect is not representative of the larger population you're interested in studying. For instance, if you only survey social media users about a new product, you might miss the perspectives of people who don't use social media.
Measurement Bias: This arises from the way data is collected or measured. For example, poorly worded survey questions or leading interview techniques can influence participants' responses and skew the data.
3. Lack of Quality Assurance Processes
Data quality is the cornerstone of good research. Inaccurate or incomplete data can lead to misleading conclusions. The absence of robust quality assurance processes can leave your data vulnerable to errors:
Data Entry Errors: Typos, inconsistencies, or missing information during data entry can significantly impact the integrity of your dataset.
Inaccurate Measurements: Faulty instruments or imprecise measurement techniques can introduce errors into your data.
Incomplete Data: Missing data points due to participant drop-out or technical glitches can limit the generalizability of your findings.
4. Limited Access to Data
Data privacy concerns are paramount in today's digital landscape. Research involving sensitive data, such as personal health information or financial records, often requires stricter access controls and anonymization techniques. Here's why access to data can be restricted:
Privacy Regulations: Regulations like GDPR (General Data Protection Regulation) in Europe and CCPA (California Consumer Privacy Act) in the US mandate that researchers obtain informed consent from participants and implement measures to protect their data privacy.
Data Sensitivity: Certain types of data, such as medical records, require stricter access controls and anonymization procedures to safeguard confidentiality.
Data Security Concerns: Cybersecurity threats are a constant concern. Researchers must employ robust security measures to protect the data they collect from unauthorized access or breaches.
5. Legal and Compliance Regulations
The legal landscape surrounding data collection can be complex and ever-changing. Researchers must stay informed about relevant regulations to ensure their data collection practices comply. Here are some key considerations:
Privacy Laws: As mentioned earlier, privacy regulations like GDPR and CCPA dictate how data can be collected, stored, and used. Failing to comply with these regulations can result in hefty fines and reputational damage.
Intellectual Property Rights: Using copyrighted data or materials without proper permission can lead to legal trouble. Researchers must be mindful of copyright laws and obtain necessary licenses if required.
Ethical Research Guidelines: Ethical research protocols ensure participant welfare and responsible data collection practices. Researchers must adhere to guidelines established by institutional review boards or relevant professional organizations.
Role of Software and Tools in Data Collection
Pen-and-paper surveys no longer dominate the data collection landscape. Today, researchers have a powerful arsenal of software and tools at their disposal, streamlining processes, enhancing data quality, and empowering them to gather richer insights. Here's how technology is transforming data collection:
1. Utilization of Qualitative Analysis Software to Streamline Data Collection
Software has revolutionized qualitative data analysis, once a time-consuming and laborious process. Tools like NVivo, MAXQDA, and Atlas allow researchers to efficiently code, categorize, and analyze textual data from interviews, focus groups, and open-ended surveys.
These features streamline the data collection process, enabling researchers to identify emerging themes, patterns, and relationships within their data more quickly and effectively.
2. Survey Creation, Customization Features, and Data Analysis Tools
Gone are the days of clunky, paper surveys. Online survey platforms like SurveyMonkey, Google Forms, and Metaforms.ai offer a plethora of features to create engaging and user-friendly surveys.
These platforms often include customization options (question types, branching logic, skip logic), real-time data analysis, and data visualization tools, allowing researchers to easily gather, analyze, and interpret survey data.
3. Impact of Technology in Improving Efficiency and Data Quality
Technology has demonstrably improved the efficiency and quality of data collection. Here's how:
Reduced Costs: Online tools and automated data collection methods can significantly reduce the costs associated with traditional data collection methods (e.g., printing surveys, hiring interviewers).
Increased Response Rates: Engaging survey formats and convenient online access can lead to higher response rates, improving the generalizability of your research findings.
Improved Data Accuracy: Software can help minimize data entry errors and inconsistencies, ensuring the accuracy and reliability of your data.
Enhanced Data Security: Cloud-based platforms often offer robust security features to safeguard sensitive data.
Conclusion
By leveraging the power of software and tools, researchers can embark on data collection with greater confidence and efficiency. Metaforms is at the forefront of this data collection revolution, offering a user-friendly platform specifically designed to streamline qualitative and quantitative research. Visit metaforms to learn more about how we can empower your research journey.
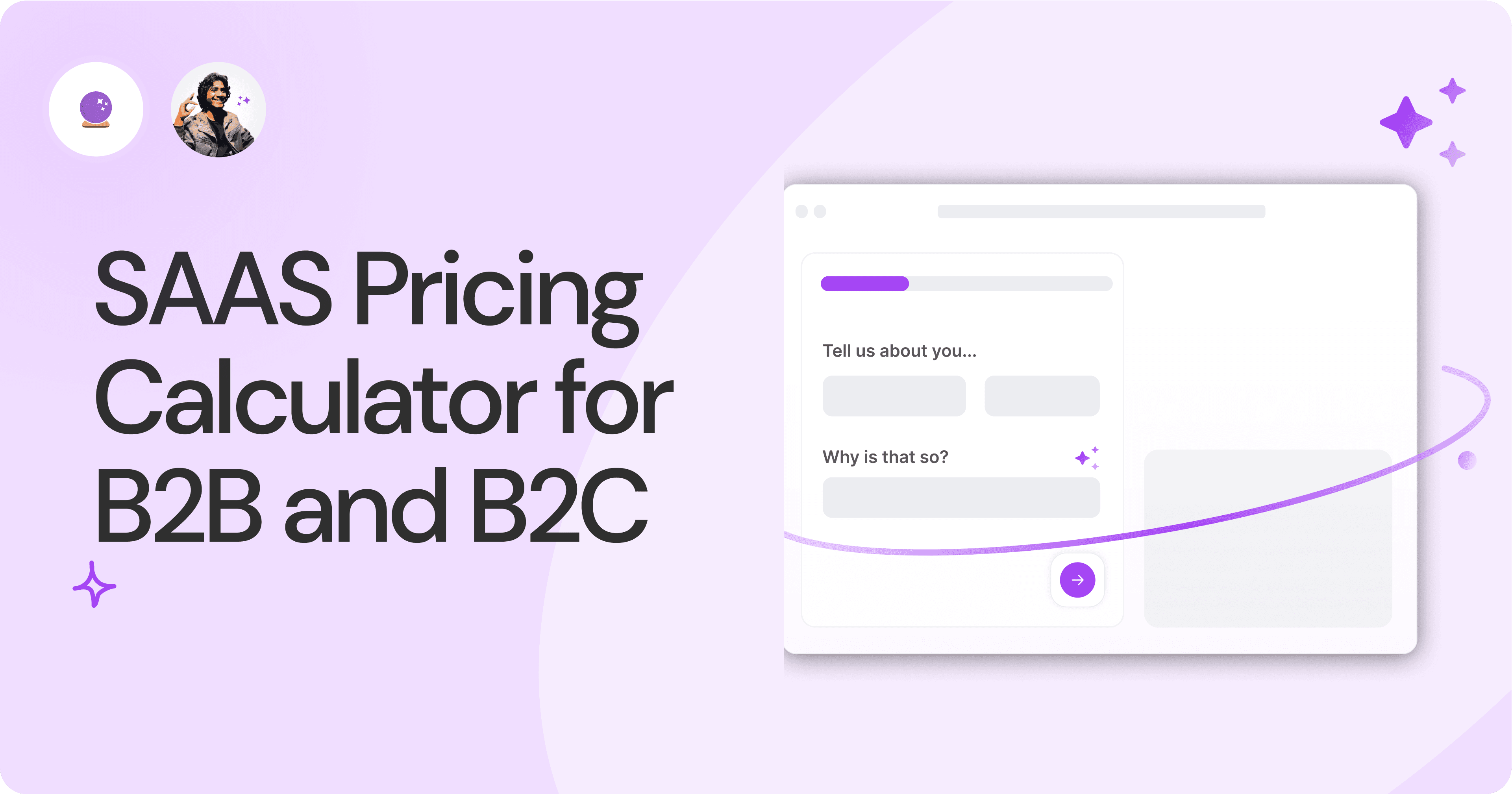
SAAS Pricing Calculators: B2B v B2C Online Forms.
The SaaS pricing calculator is an essential tool for both B2B and B2C SaaS companies. But how do you build it, right? We have you covered.
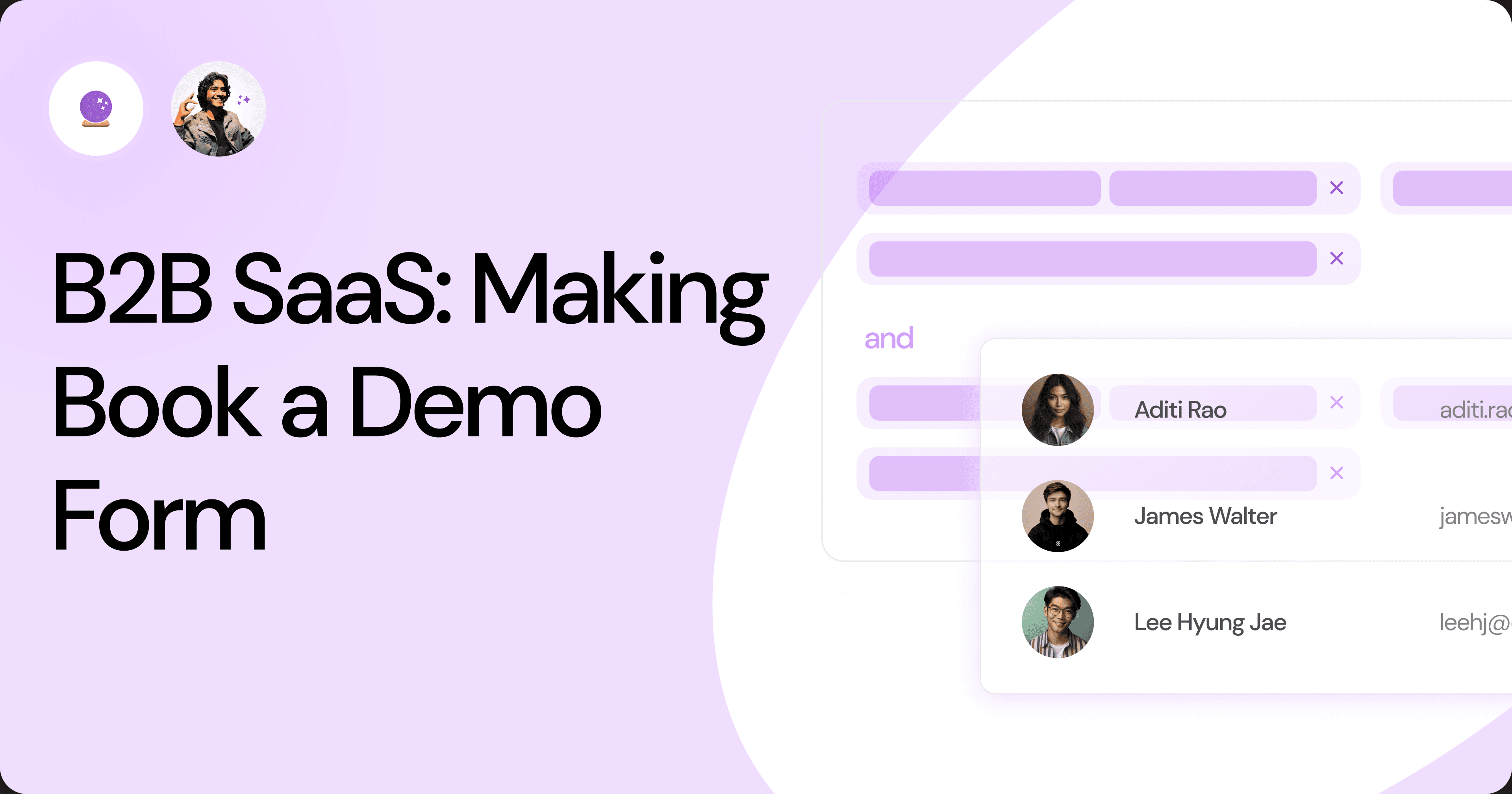
B2B SaaS: Making Book a Demo Form.
Having the perfect book a demo form for B2B SaaS is the first step in capturing leads. There are a few fundamental techniques to get this form right. Read on.
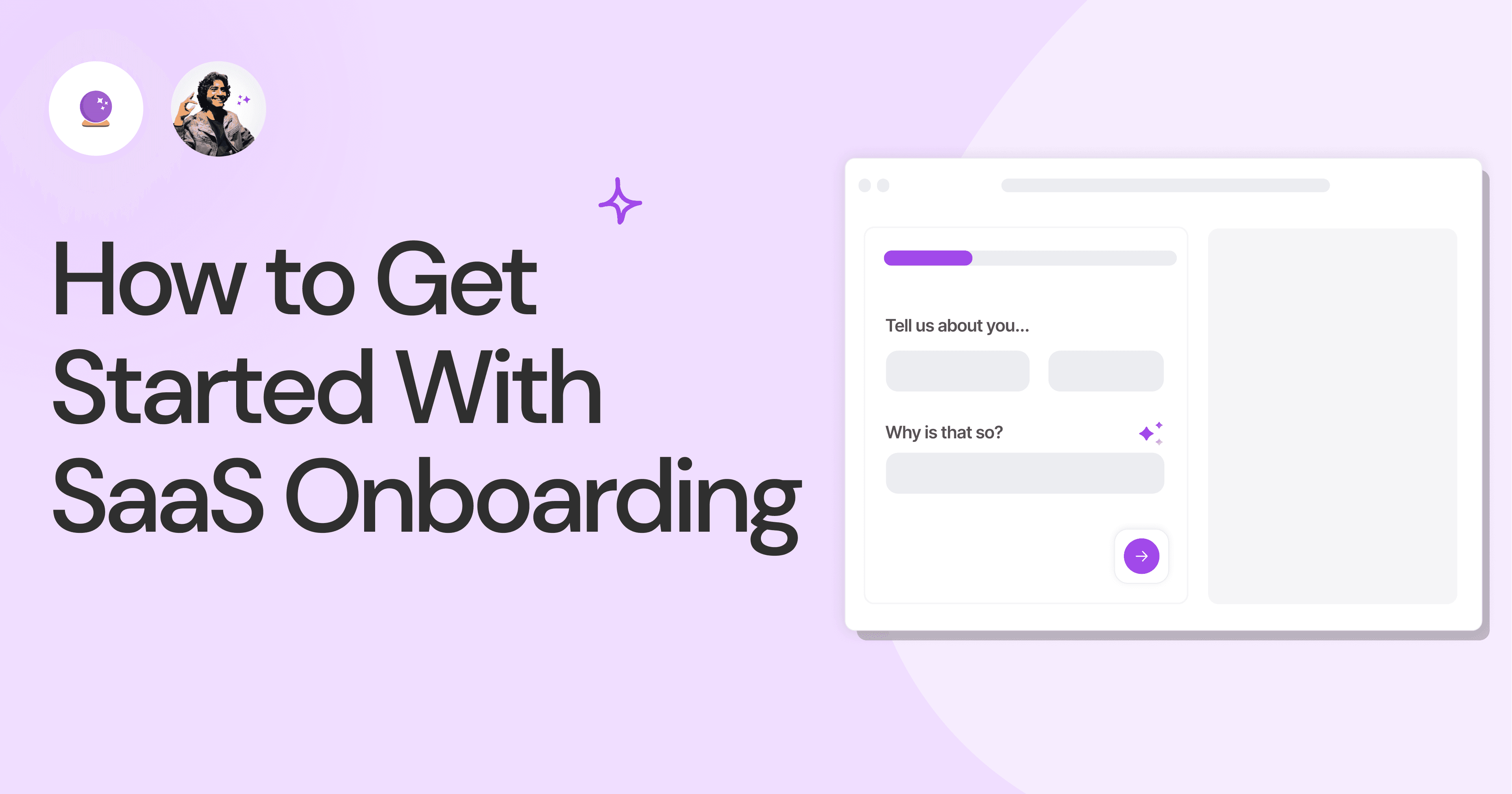
How to Get Started With SaaS Onboarding.
SaaS onboarding is essential for customer onboarding in B2B and B2C SaaS. Let’s understand its fundamentals, including the metrics.
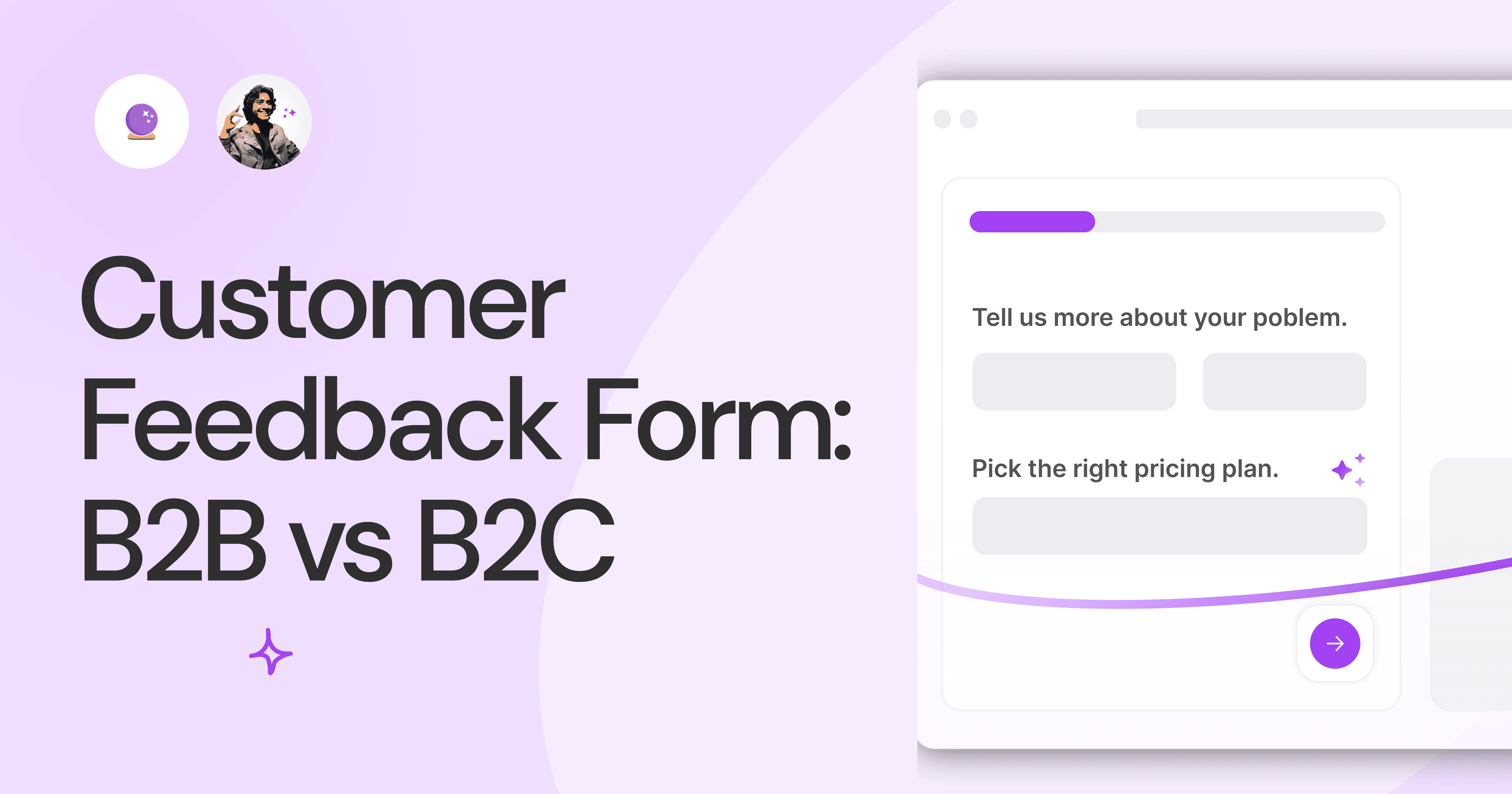
Customer Feedback Form: B2B vs B2C.
Why is customer feedback important? Because it dictates progress on B2B and B2B products and services for the customer to meet their goals.

SaaS Customer Feedback Form: 6 Main Types.
As much as SaaS is self-serve, the role of a customer feedback form is highly relevant. There are different types, each with its use case and sections.
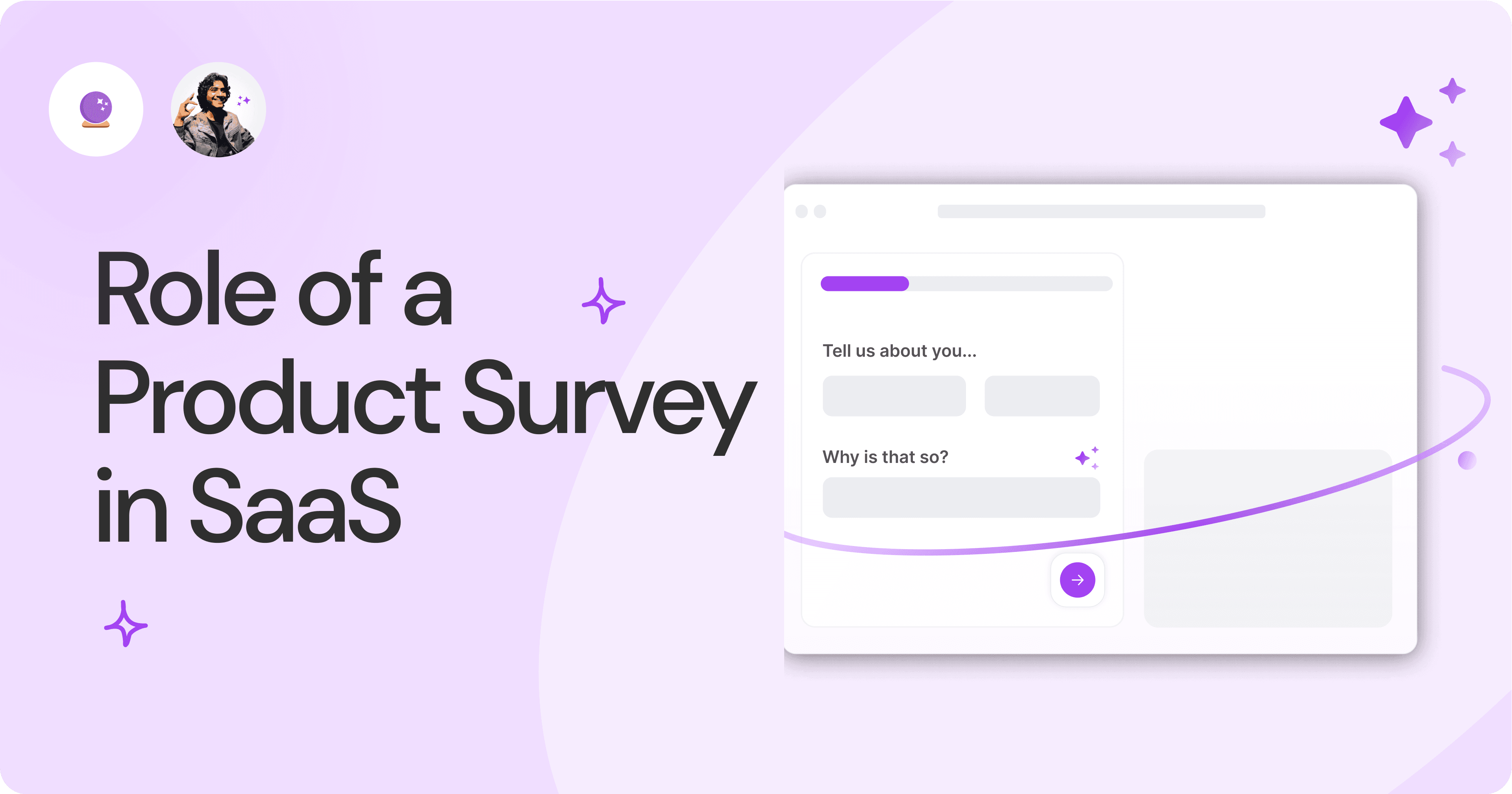
Role of a Product Survey in SaaS.
SaaS is all about creating products for specific use cases. This is where a product survey becomes all the more important to know the user deeply.

Should You Do a SaaS Market Survey?
Every SaaS company wants to grow fast. But without a market survey, growth isn't possible or sustainable. Let’s see how to do a market survey.
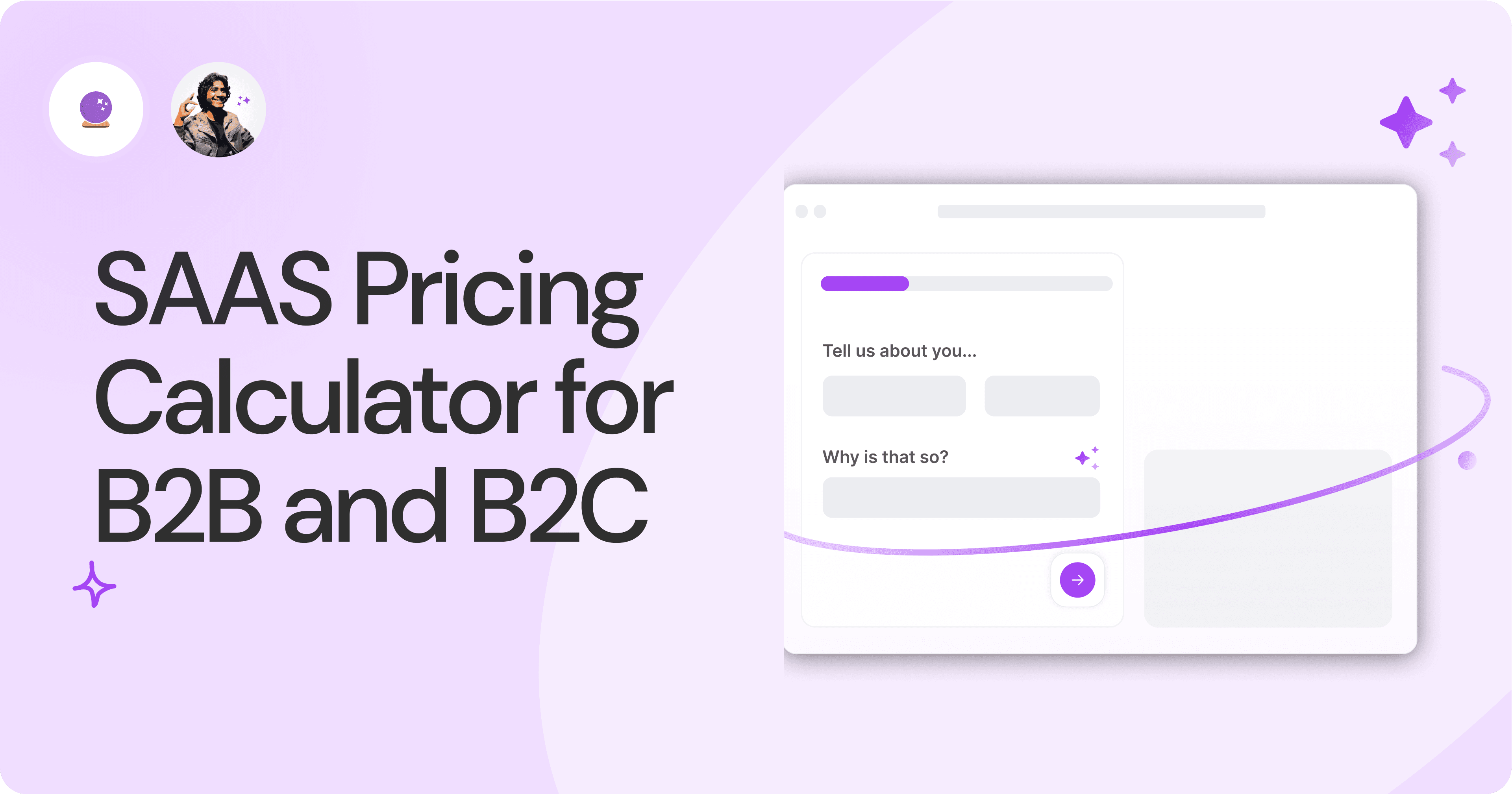
SAAS Pricing Calculators: B2B v B2C Online Forms.
The SaaS pricing calculator is an essential tool for both B2B and B2C SaaS companies. But how do you build it, right? We have you covered.
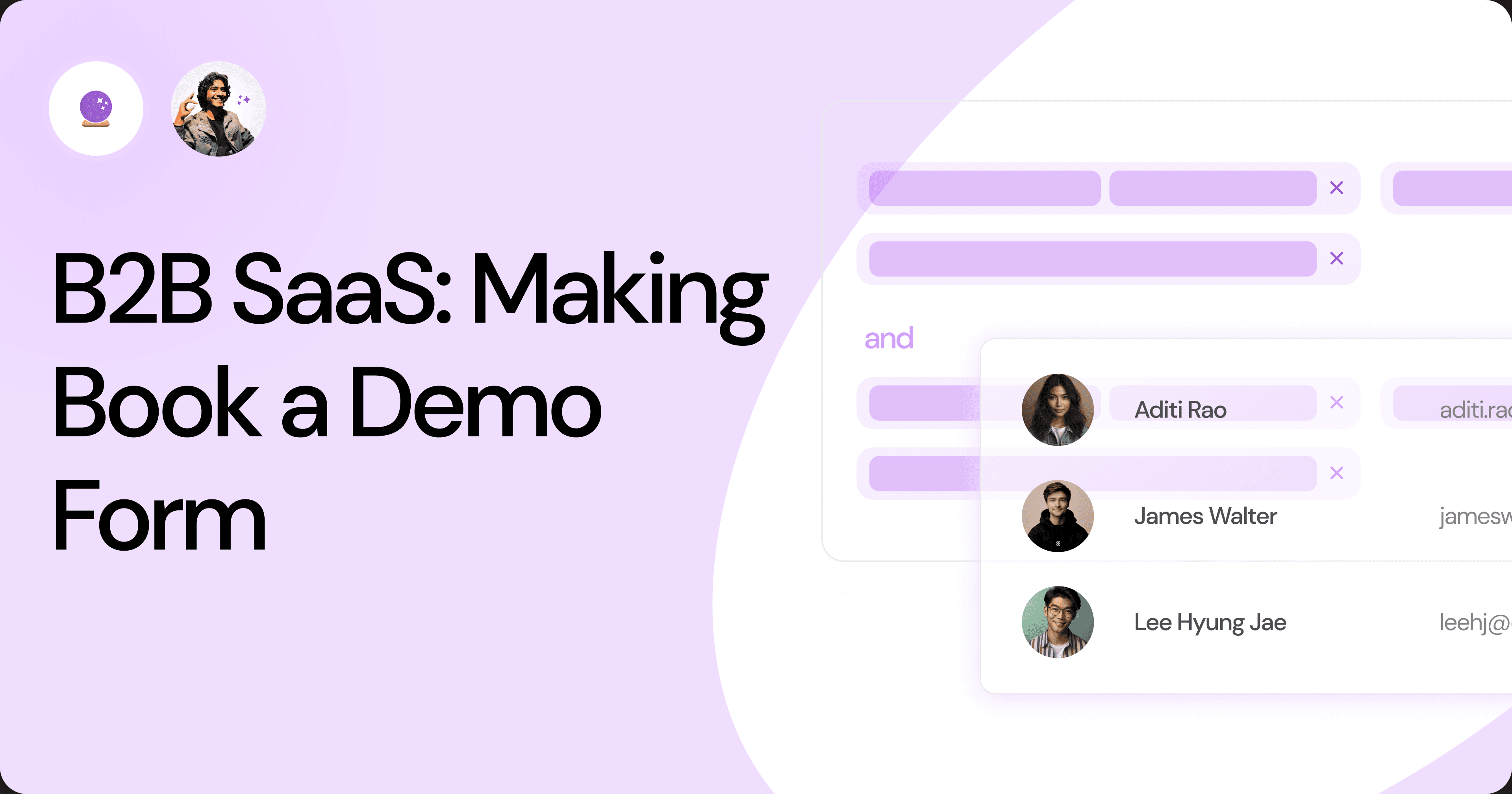
B2B SaaS: Making Book a Demo Form.
Having the perfect book a demo form for B2B SaaS is the first step in capturing leads. There are a few fundamental techniques to get this form right. Read on.
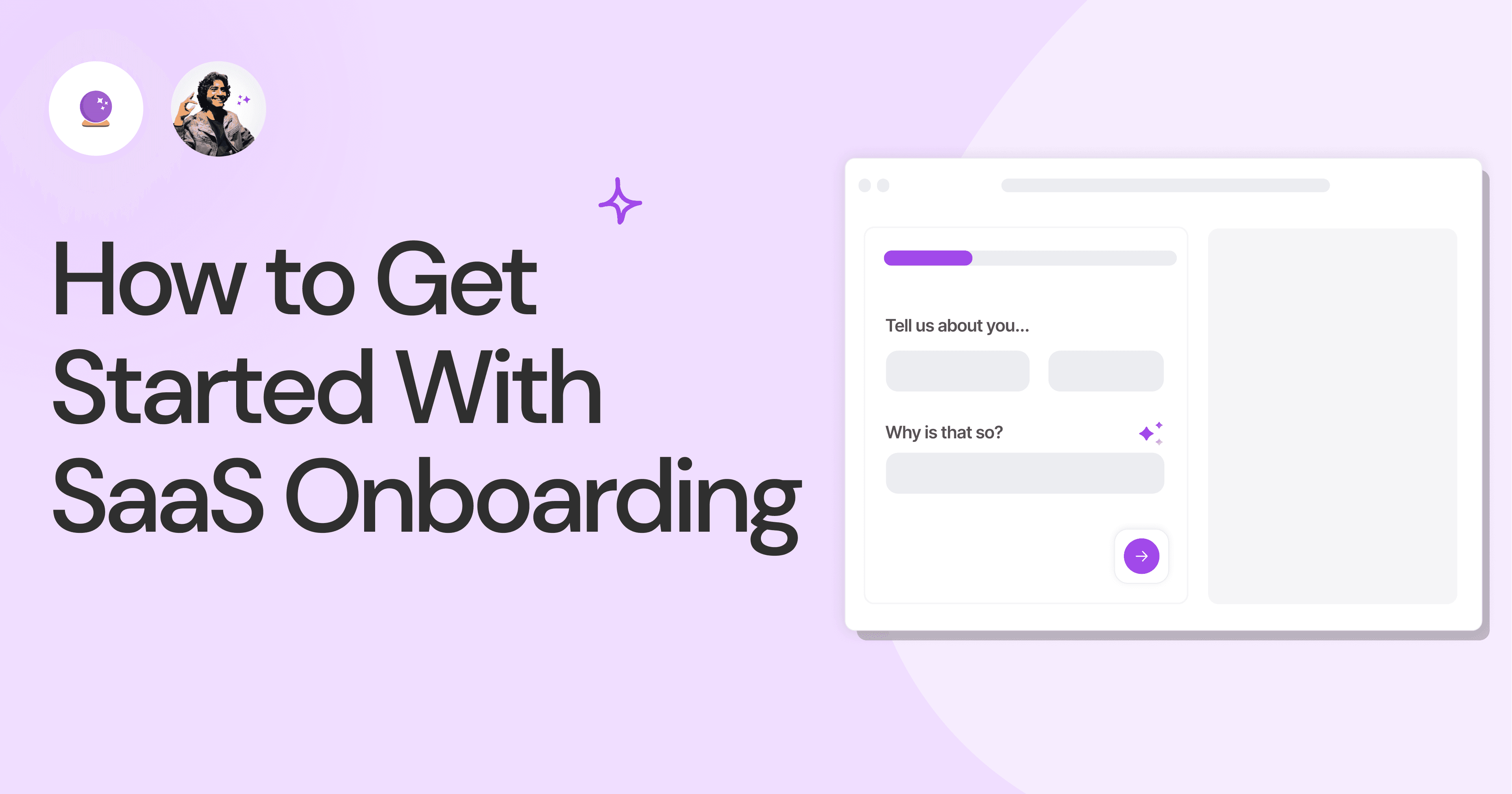
How to Get Started With SaaS Onboarding.
SaaS onboarding is essential for customer onboarding in B2B and B2C SaaS. Let’s understand its fundamentals, including the metrics.
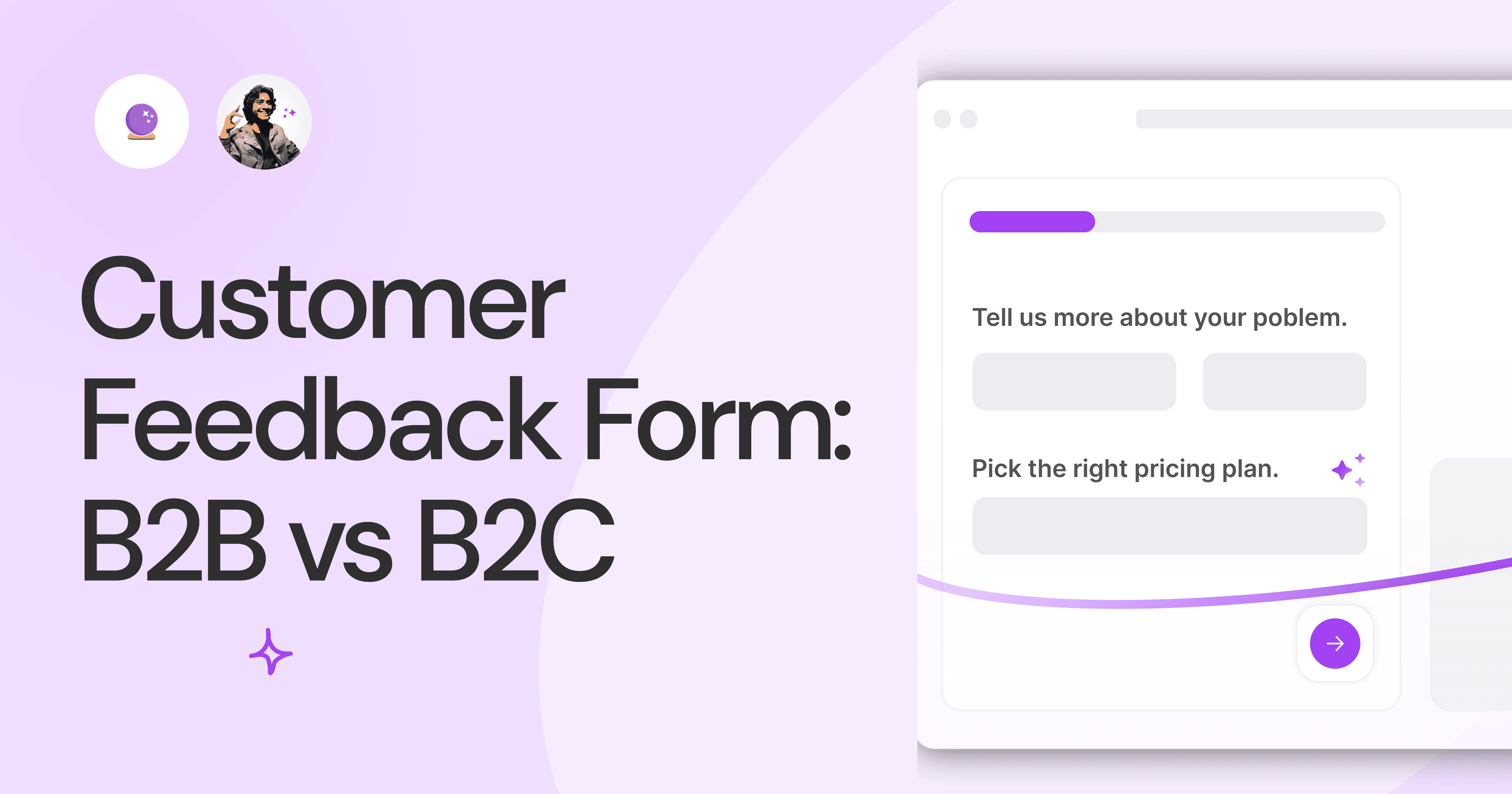
Customer Feedback Form: B2B vs B2C.
Why is customer feedback important? Because it dictates progress on B2B and B2B products and services for the customer to meet their goals.

SaaS Customer Feedback Form: 6 Main Types.
As much as SaaS is self-serve, the role of a customer feedback form is highly relevant. There are different types, each with its use case and sections.
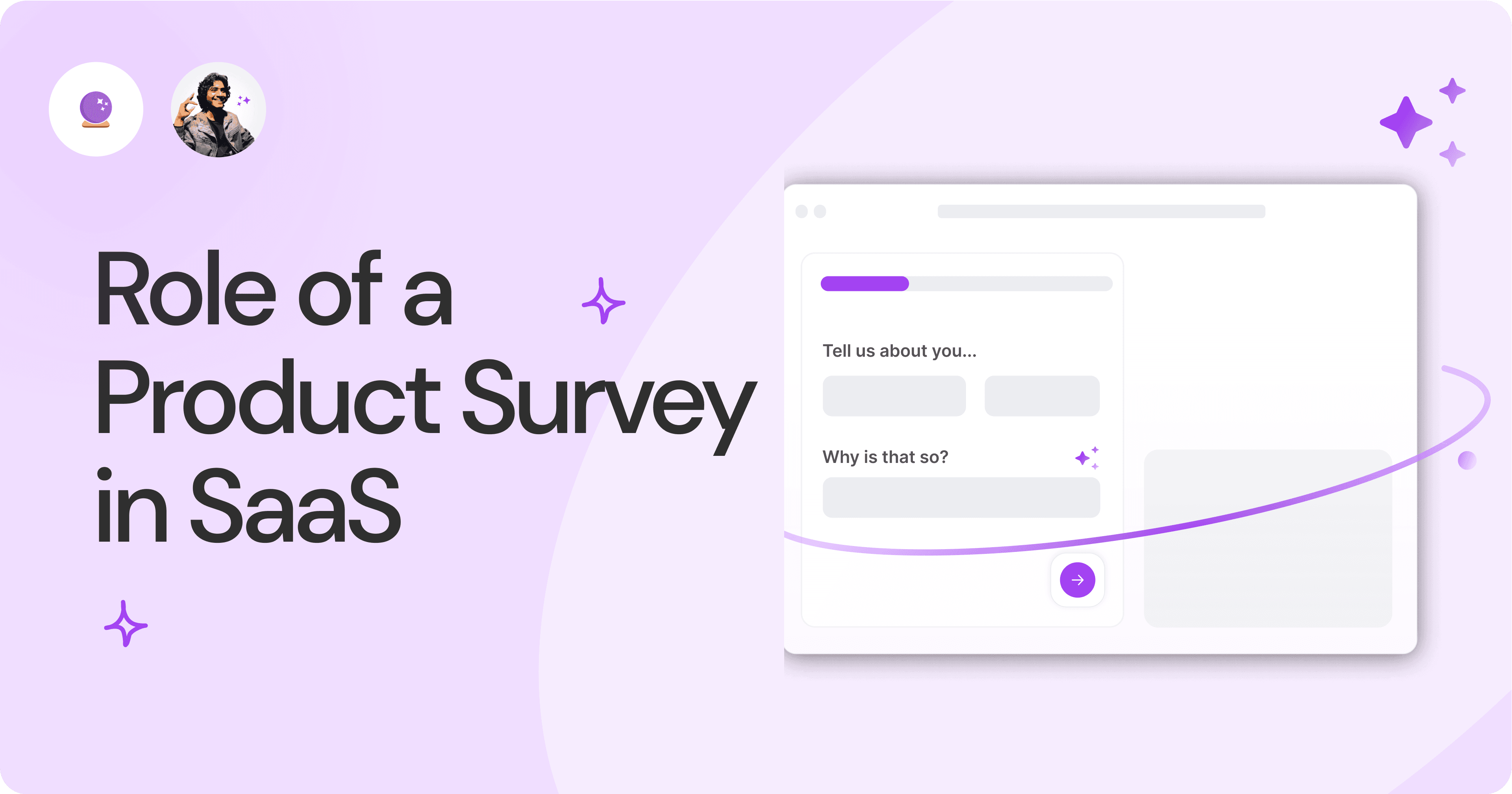
Role of a Product Survey in SaaS.
SaaS is all about creating products for specific use cases. This is where a product survey becomes all the more important to know the user deeply.

Should You Do a SaaS Market Survey?
Every SaaS company wants to grow fast. But without a market survey, growth isn't possible or sustainable. Let’s see how to do a market survey.
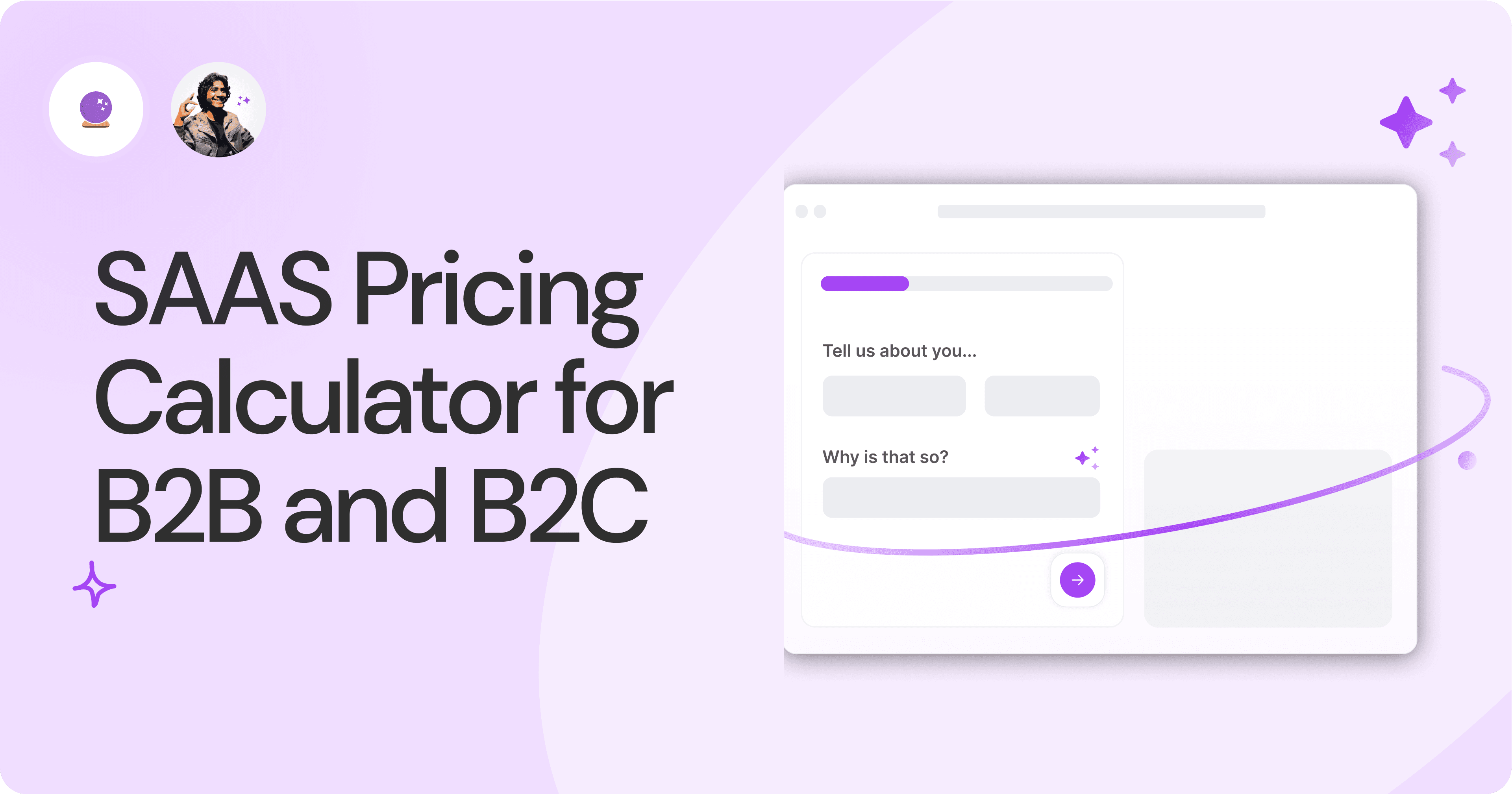
SAAS Pricing Calculators: B2B v B2C Online Forms.
The SaaS pricing calculator is an essential tool for both B2B and B2C SaaS companies. But how do you build it, right? We have you covered.
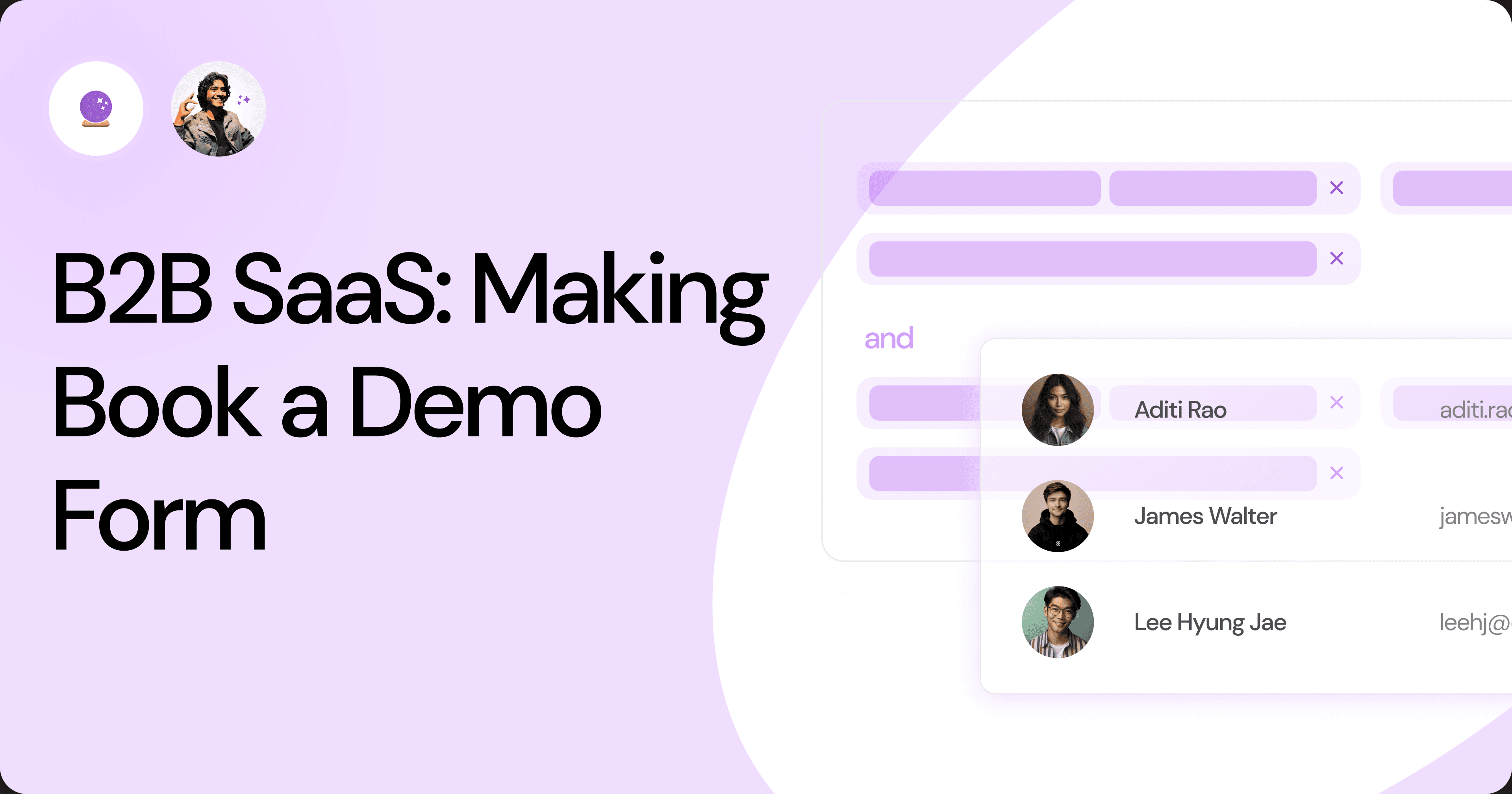
B2B SaaS: Making Book a Demo Form.
Having the perfect book a demo form for B2B SaaS is the first step in capturing leads. There are a few fundamental techniques to get this form right. Read on.
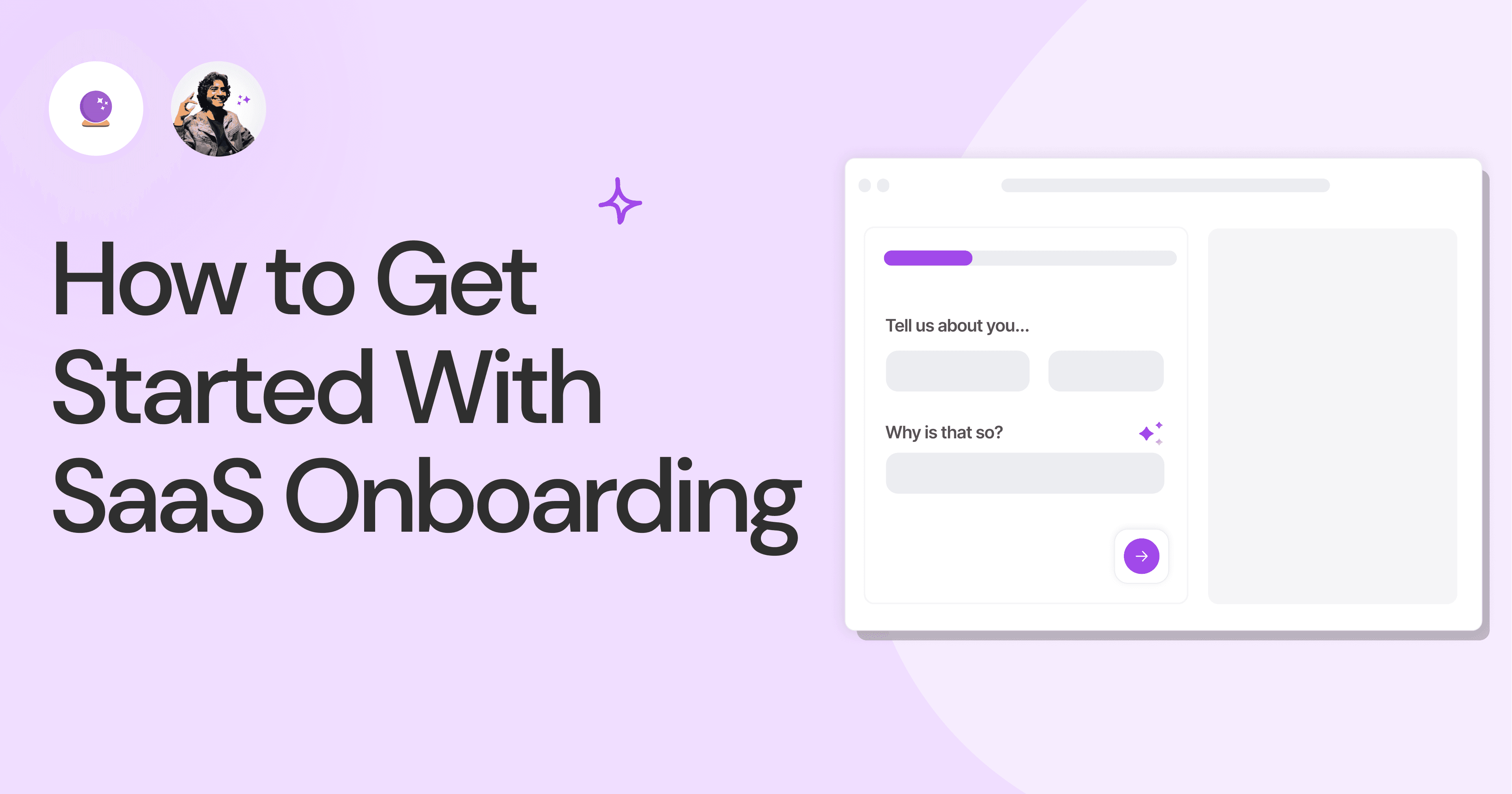
How to Get Started With SaaS Onboarding.
SaaS onboarding is essential for customer onboarding in B2B and B2C SaaS. Let’s understand its fundamentals, including the metrics.
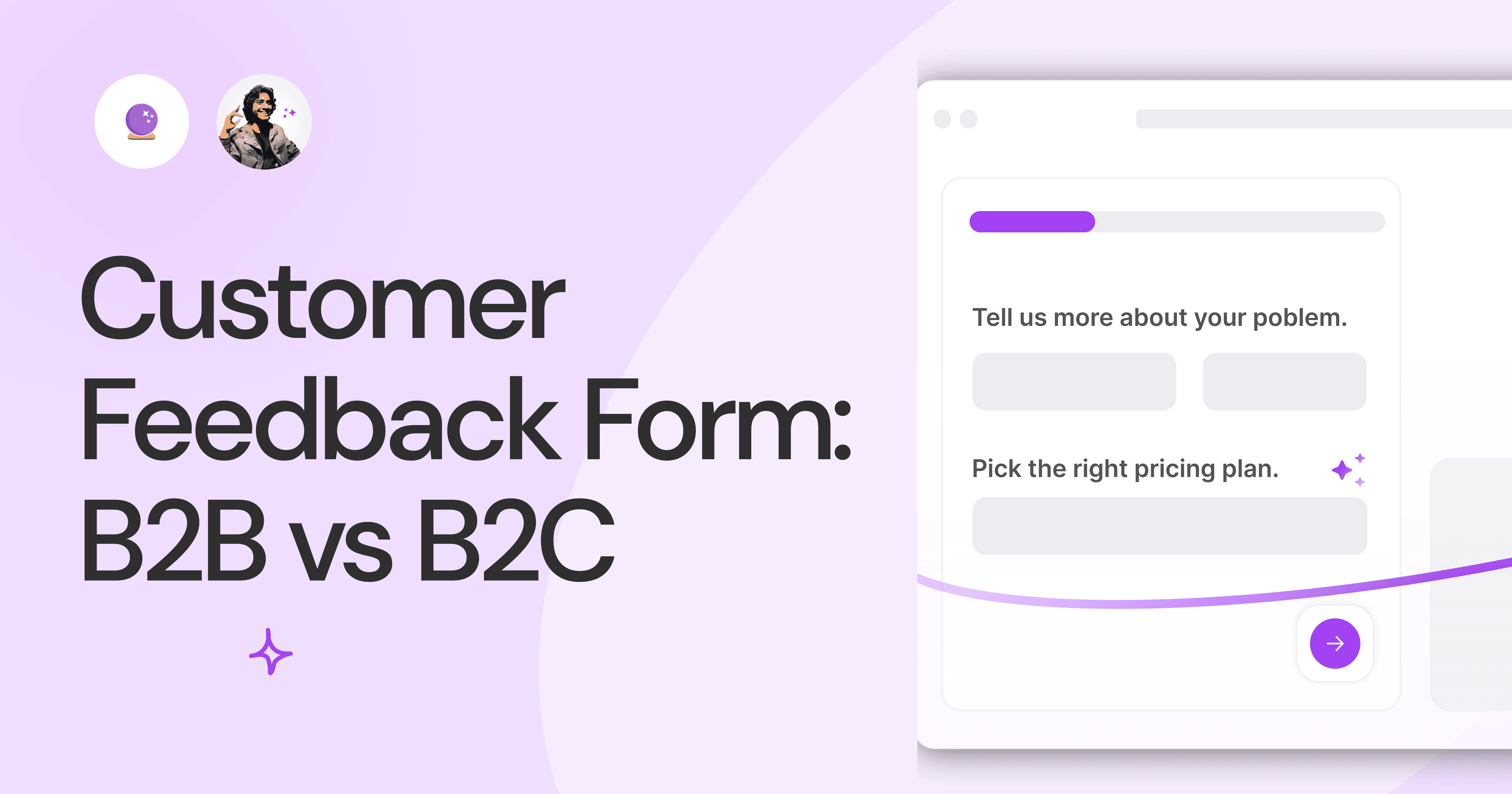
Customer Feedback Form: B2B vs B2C.
Why is customer feedback important? Because it dictates progress on B2B and B2B products and services for the customer to meet their goals.

SaaS Customer Feedback Form: 6 Main Types.
As much as SaaS is self-serve, the role of a customer feedback form is highly relevant. There are different types, each with its use case and sections.
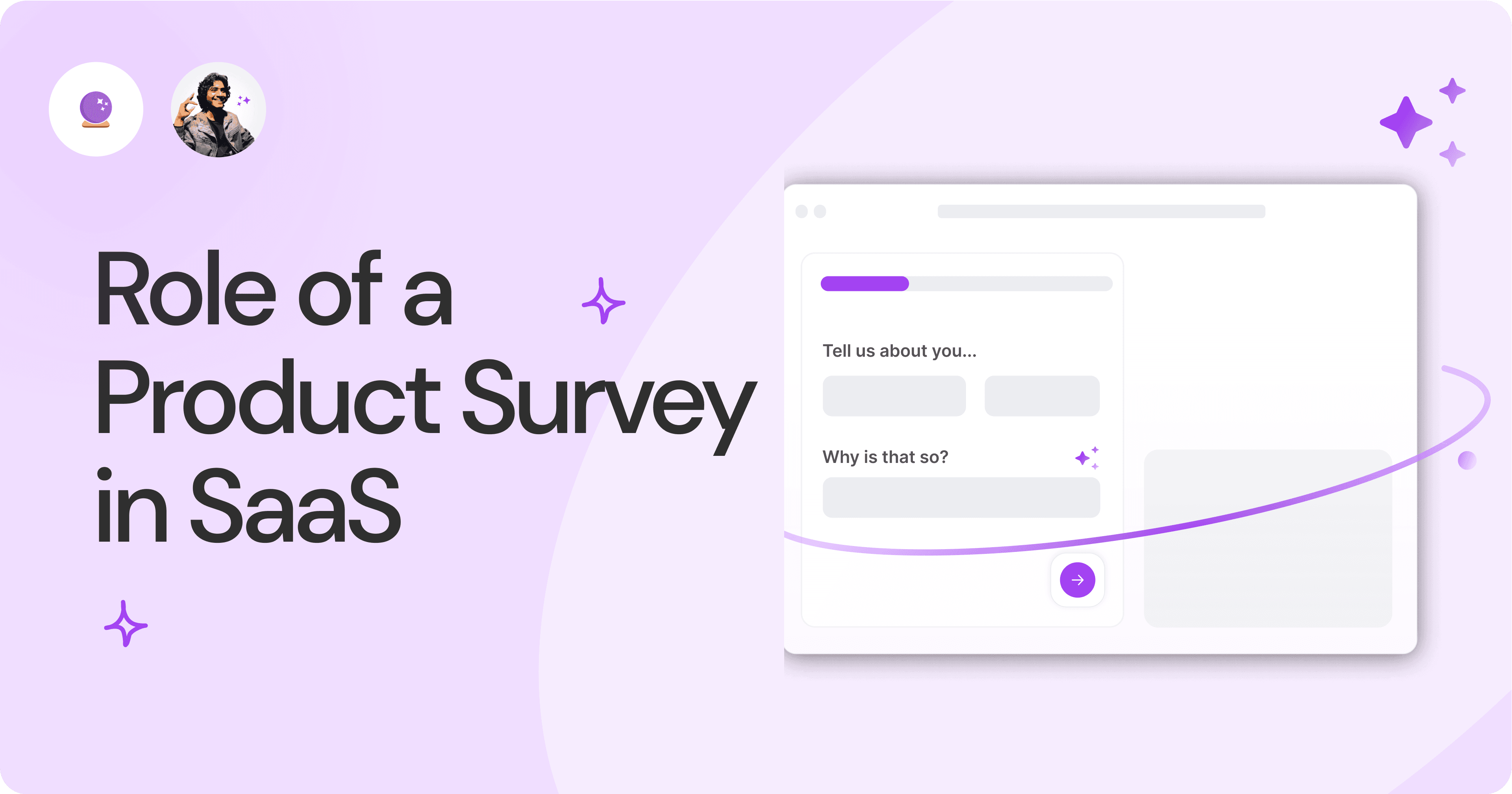
Role of a Product Survey in SaaS.
SaaS is all about creating products for specific use cases. This is where a product survey becomes all the more important to know the user deeply.

Should You Do a SaaS Market Survey?
Every SaaS company wants to grow fast. But without a market survey, growth isn't possible or sustainable. Let’s see how to do a market survey.
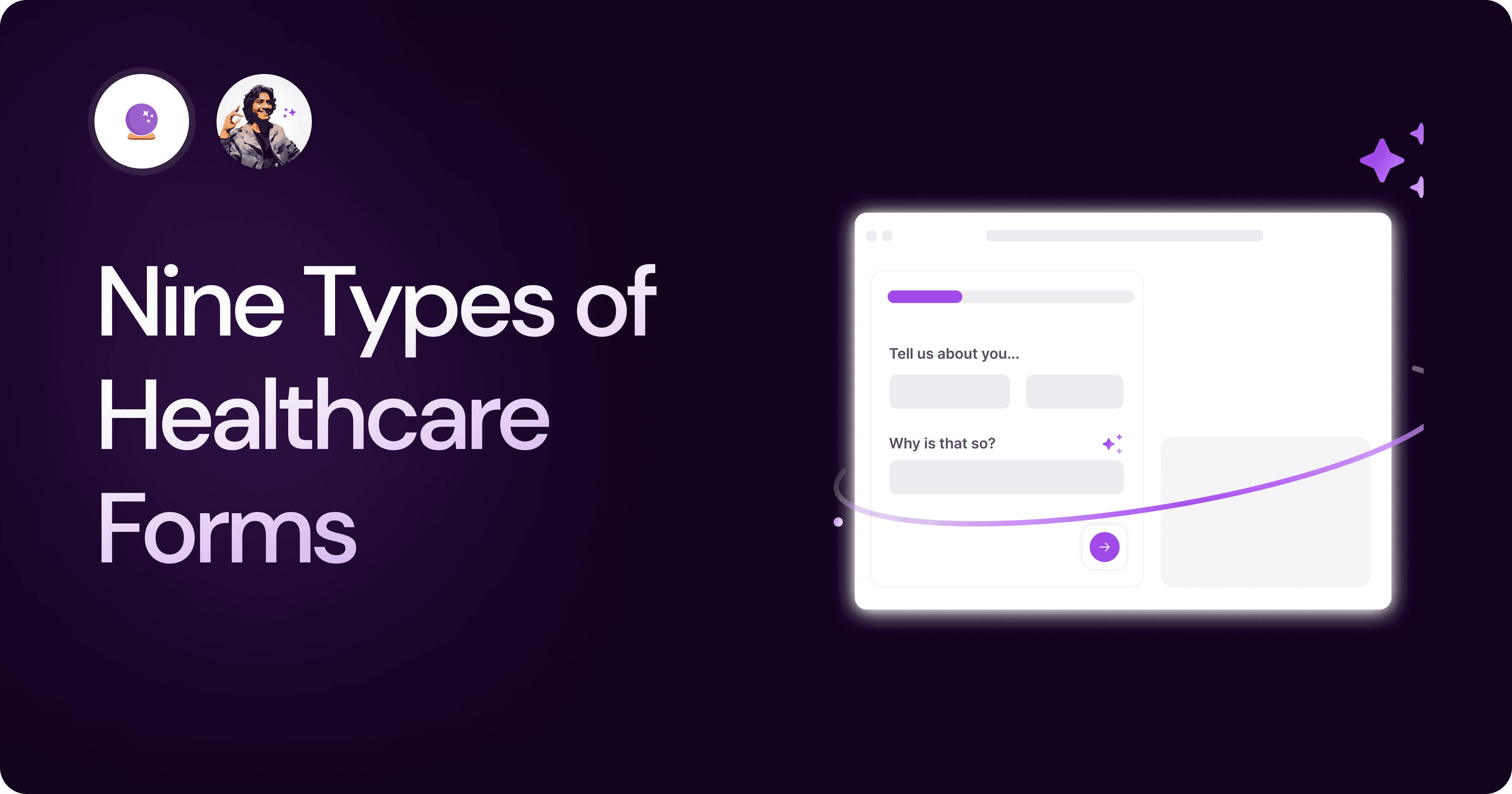
Nine Types of Healthcare and Medical Forms.
Medical forms are a must-have for any healthcare business or practitioner. Learn about the different kinds of medical and healthcare forms.
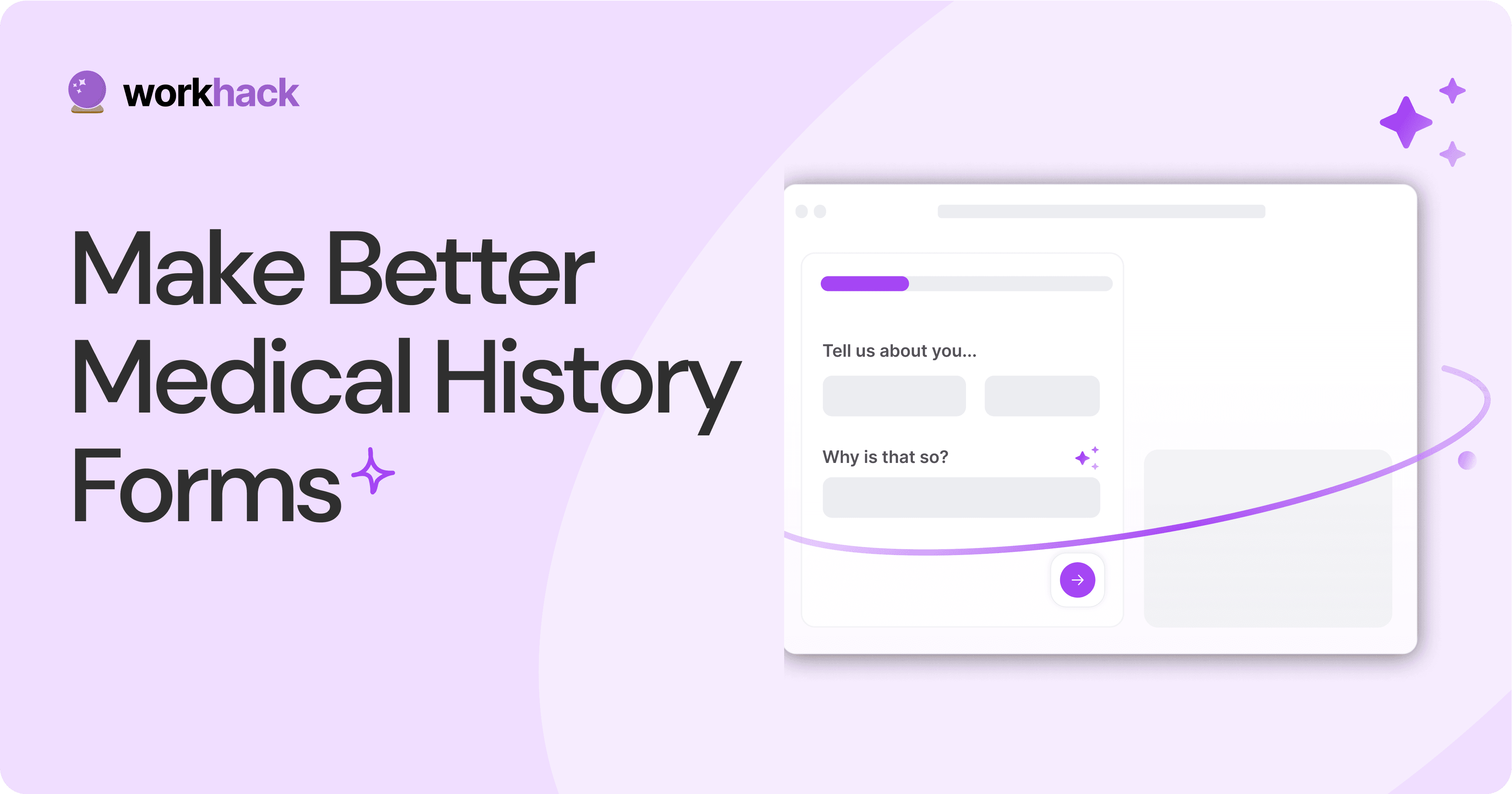
4 Tips for Better Medical History Forms.
Medical history forms are central to patient care, onboarding, and medical administration records. Learn how to make them easier to fill.
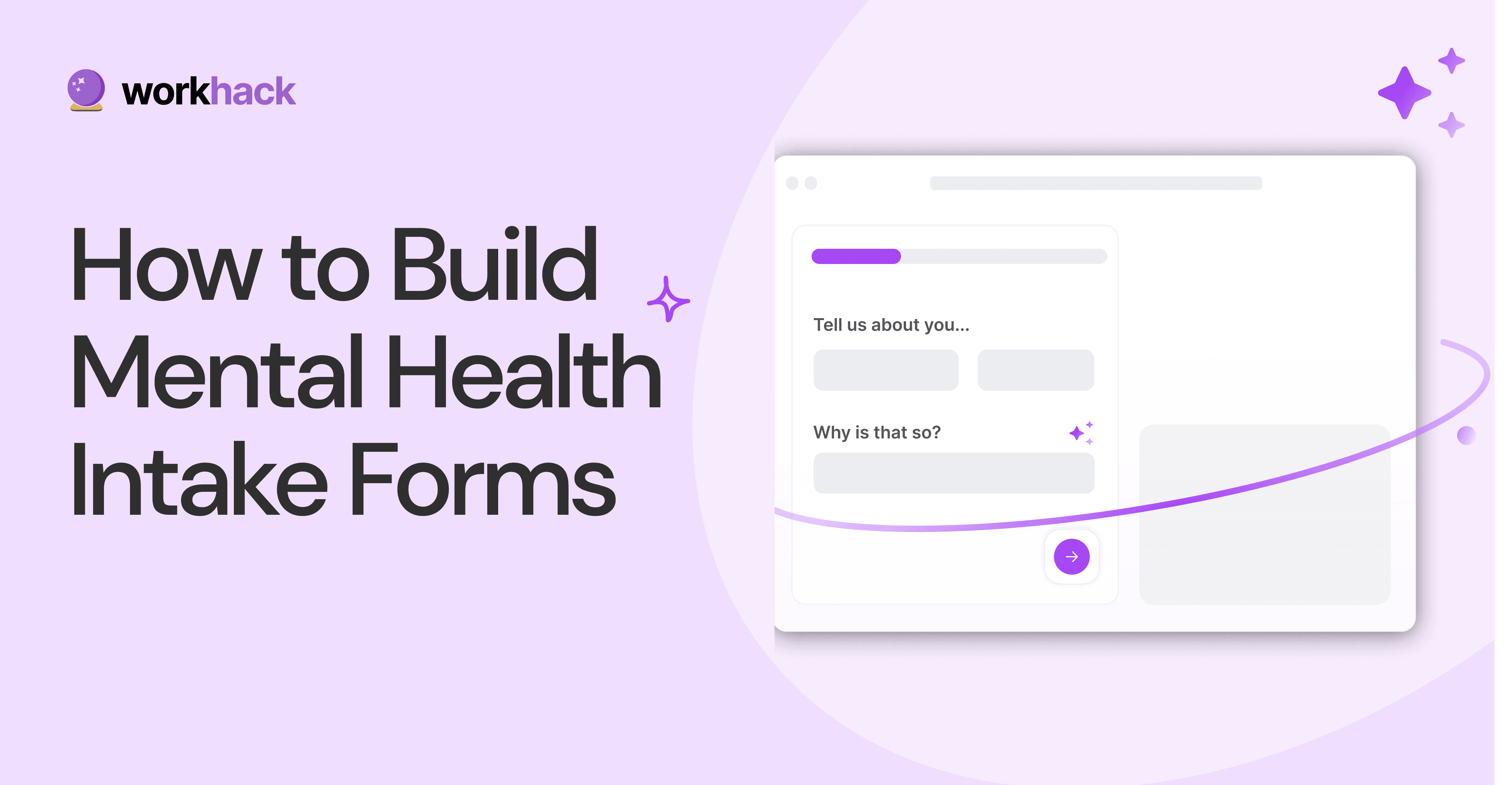
How to Build Mental Health Intake Forms?
Mental health intake forms are not like patient intake forms. Mental health intake forms deal with far more sensitive data and have specific design methods.
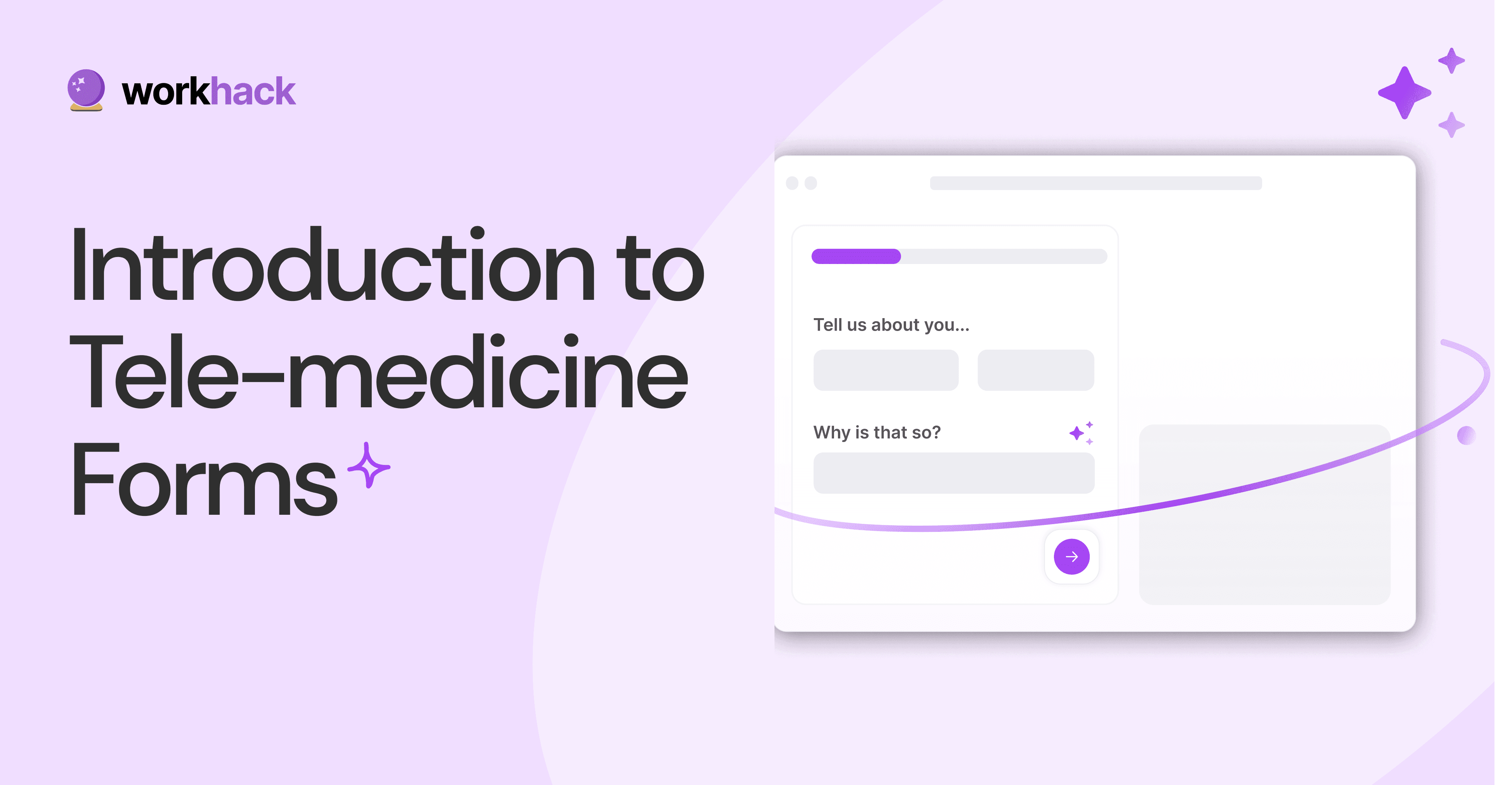
What, Why and How of Telemedicine Forms.
Telemedicine is on the rise and with different form builders out there, which one best suits your needs as a healthcare services provider?
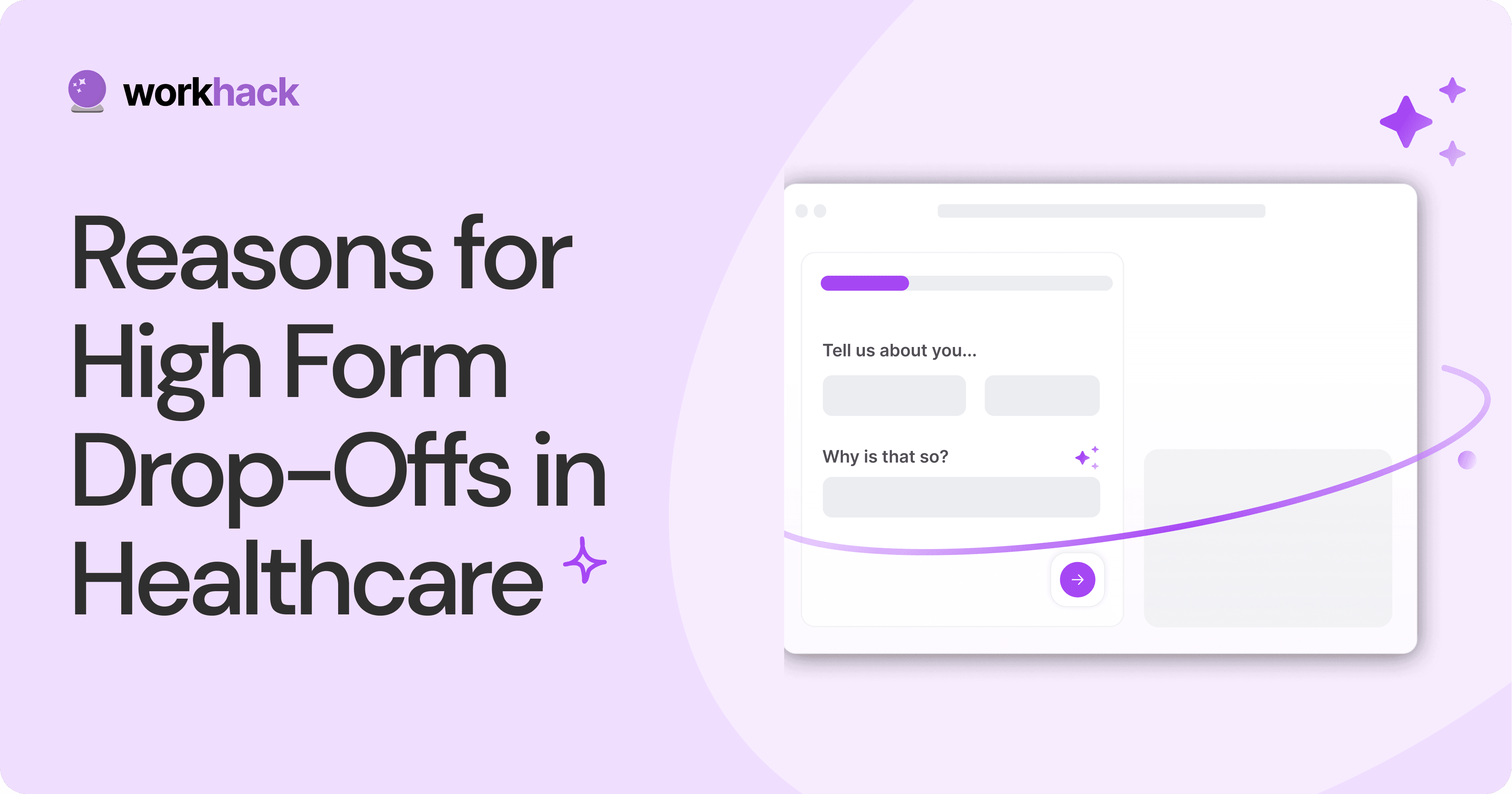
3 Reasons for Major Drop-Offs in Medical Forms.
No matter which healthcare form we pick, there are major drop-off reasons. We shall dive into the top 3 and learn how to resolve them in your next form.
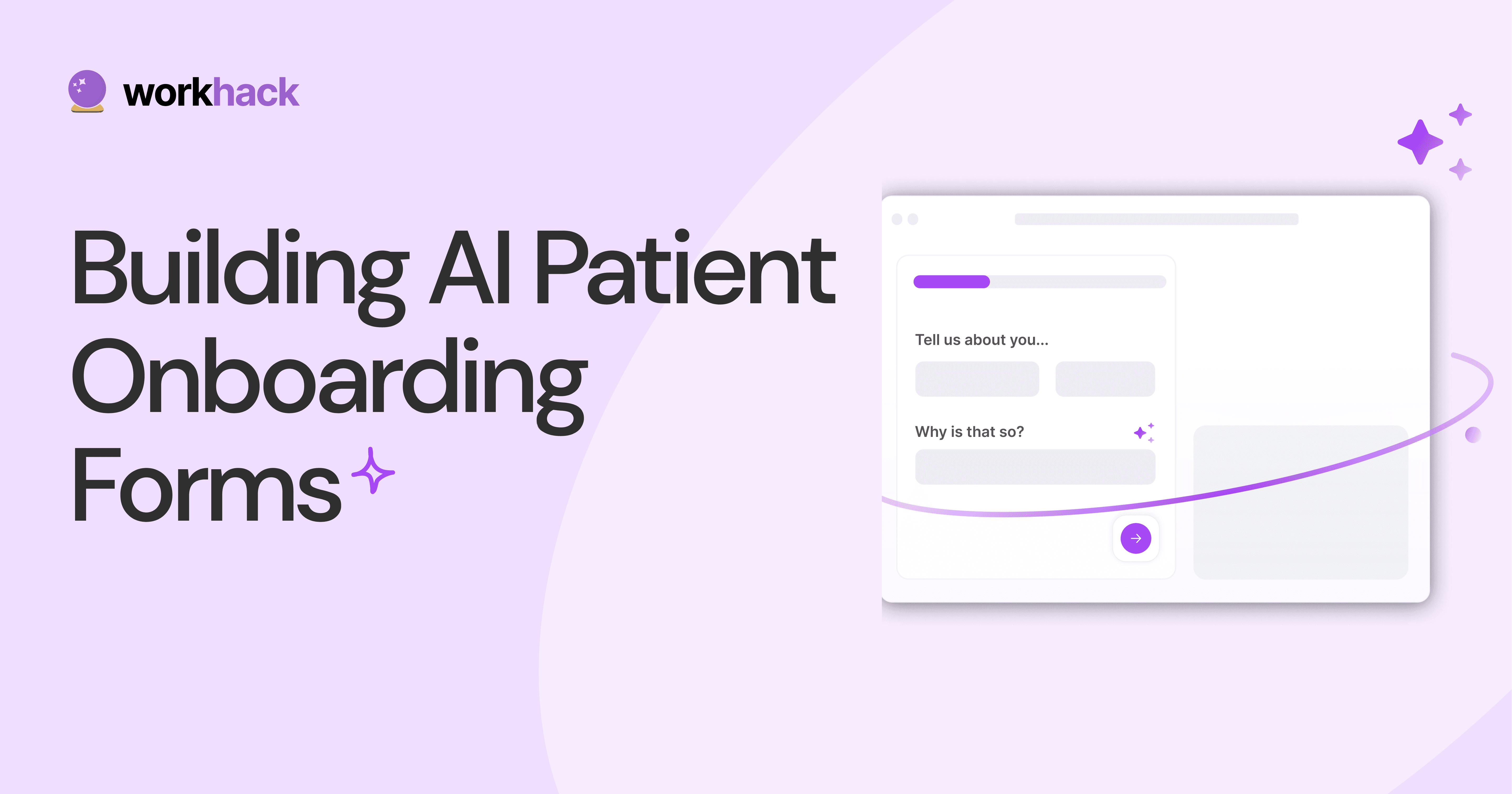
Patient Onboarding Forms - From Click to Clinic.
Patient onboarding forms are the first touchpoint for patients; getting this right for higher conversion rates is a must-have. Learn how to perfect them now.

5 Key Parts of a Good Patient Satisfaction Form.
The goal of patient satisfaction surveys is to course-correct the services of a healthcare provider. Patient feedback leads to a culture of patient-centric care.
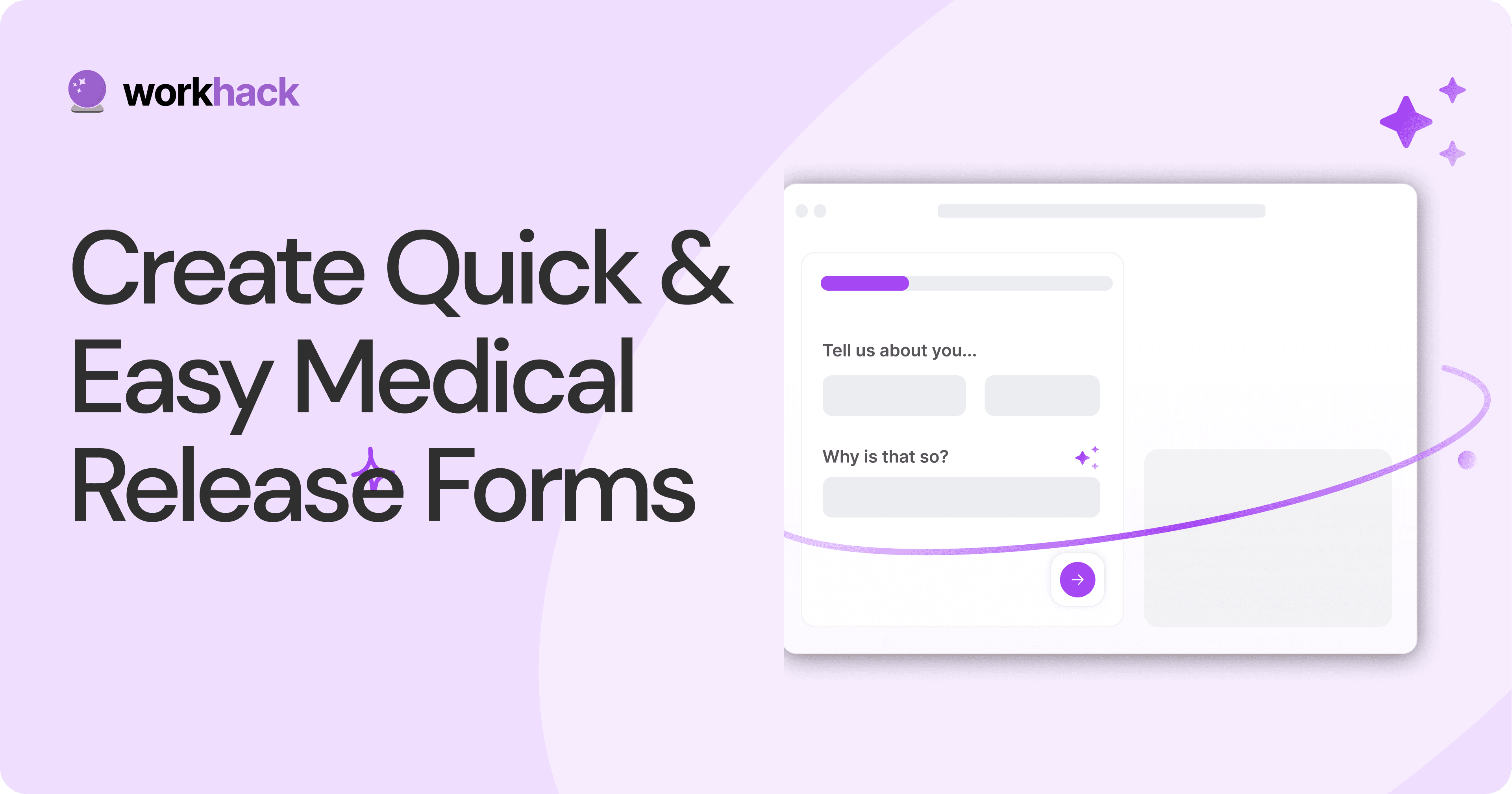
Build Quick and Easy Medical Release Forms.
Every HIPAA-compliant healthcare provider comes across medical release forms that involve details from medical history forms. Can they be shipped fast? Yes.
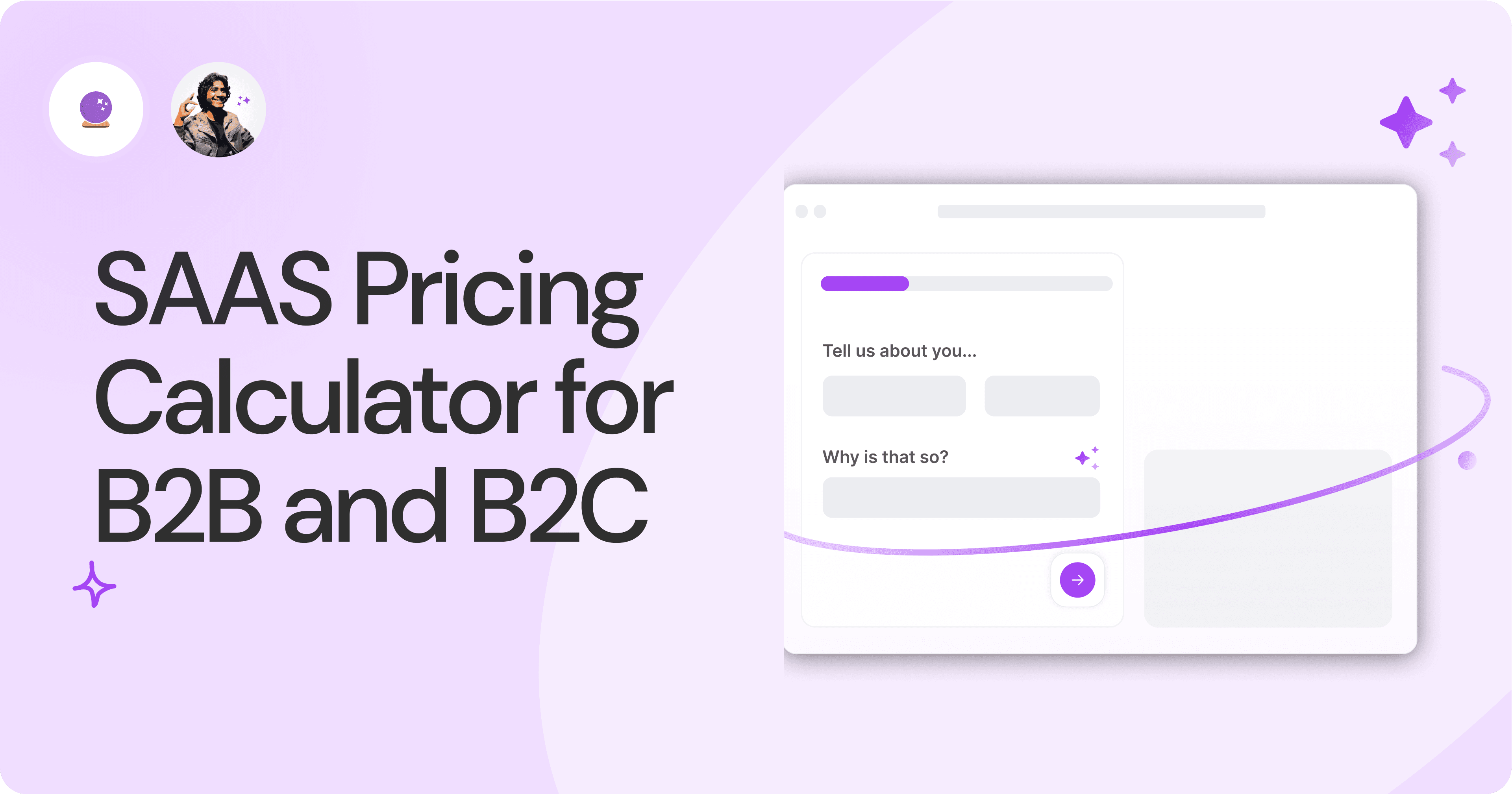
SAAS Pricing Calculators: B2B v B2C Online Forms.
The SaaS pricing calculator is an essential tool for both B2B and B2C SaaS companies. But how do you build it, right? We have you covered.
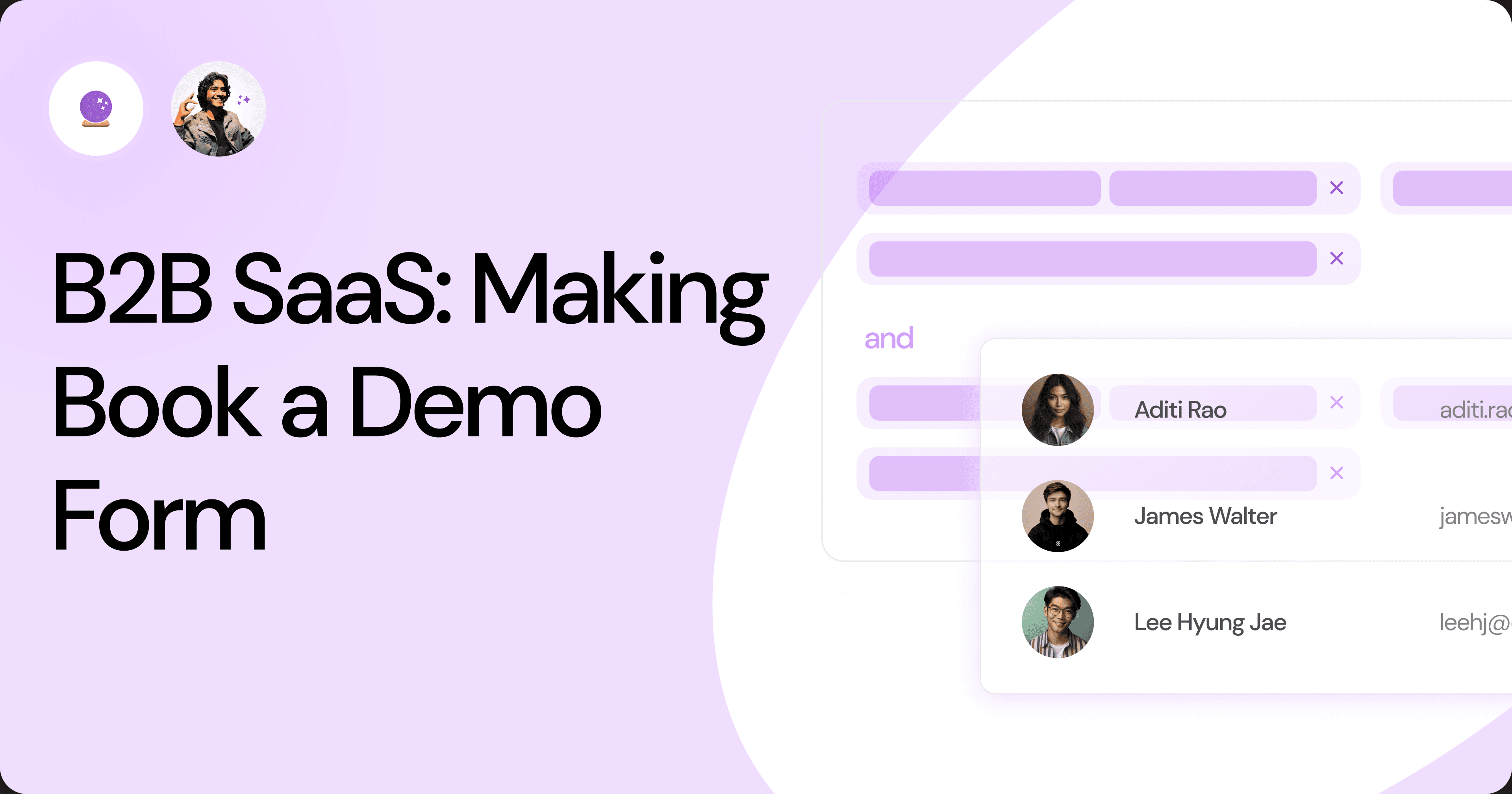
B2B SaaS: Making Book a Demo Form.
Having the perfect book a demo form for B2B SaaS is the first step in capturing leads. There are a few fundamental techniques to get this form right. Read on.
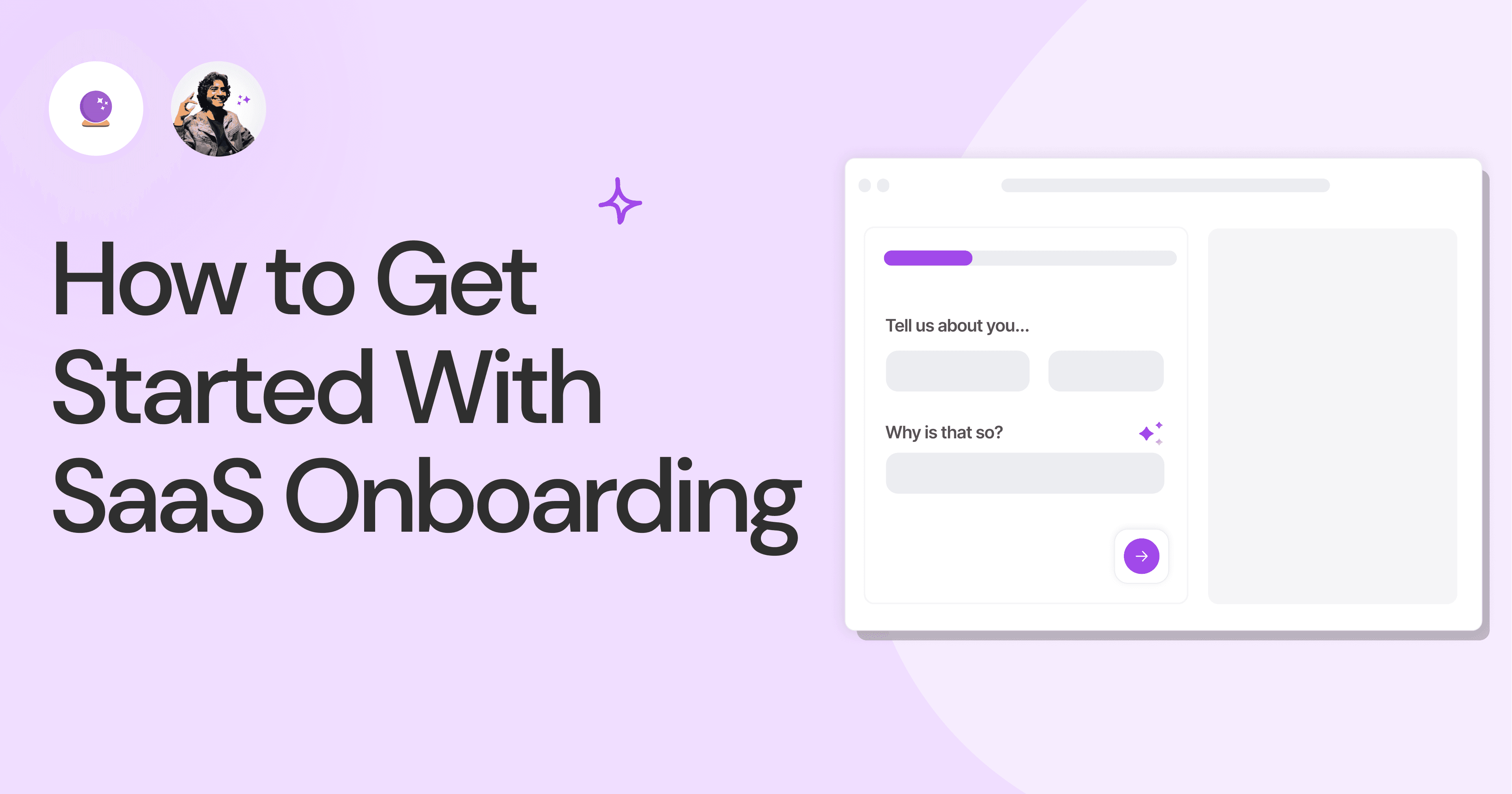
How to Get Started With SaaS Onboarding.
SaaS onboarding is essential for customer onboarding in B2B and B2C SaaS. Let’s understand its fundamentals, including the metrics.
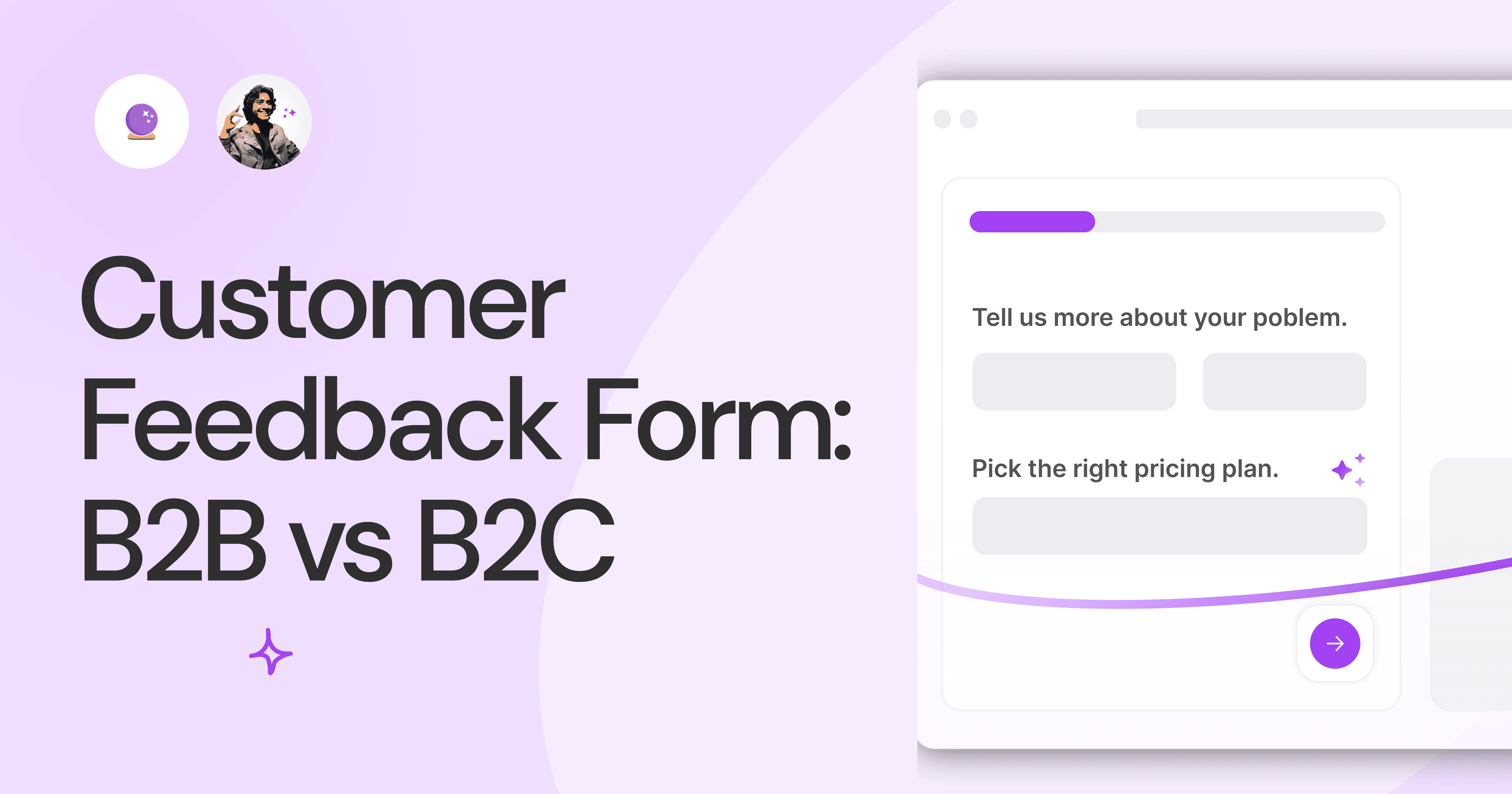
Customer Feedback Form: B2B vs B2C.
Why is customer feedback important? Because it dictates progress on B2B and B2B products and services for the customer to meet their goals.

SaaS Customer Feedback Form: 6 Main Types.
As much as SaaS is self-serve, the role of a customer feedback form is highly relevant. There are different types, each with its use case and sections.
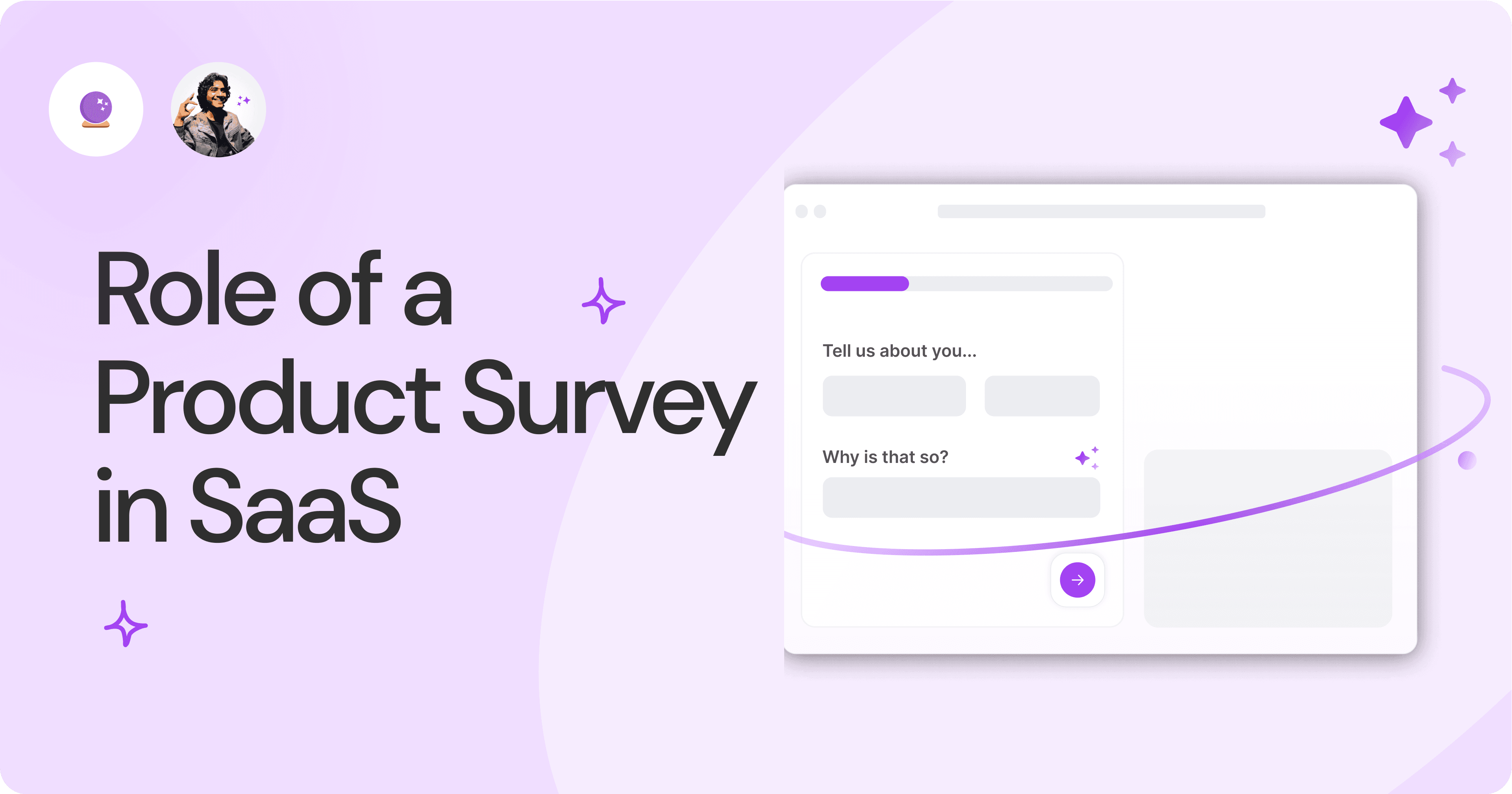
Role of a Product Survey in SaaS.
SaaS is all about creating products for specific use cases. This is where a product survey becomes all the more important to know the user deeply.

Should You Do a SaaS Market Survey?
Every SaaS company wants to grow fast. But without a market survey, growth isn't possible or sustainable. Let’s see how to do a market survey.
Subscribe to stay updated.
Subscribe to stay updated.
Subscribe to stay updated.
HC
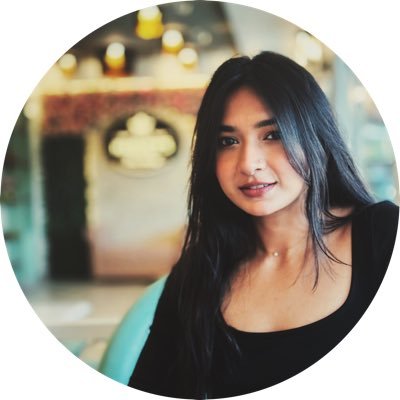
HC
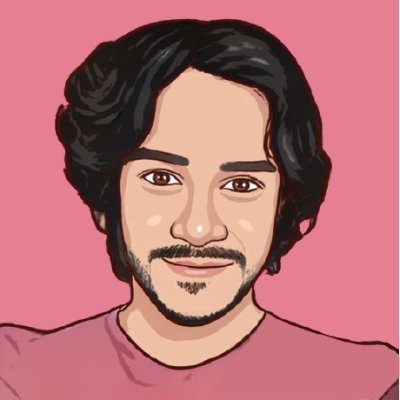
HC
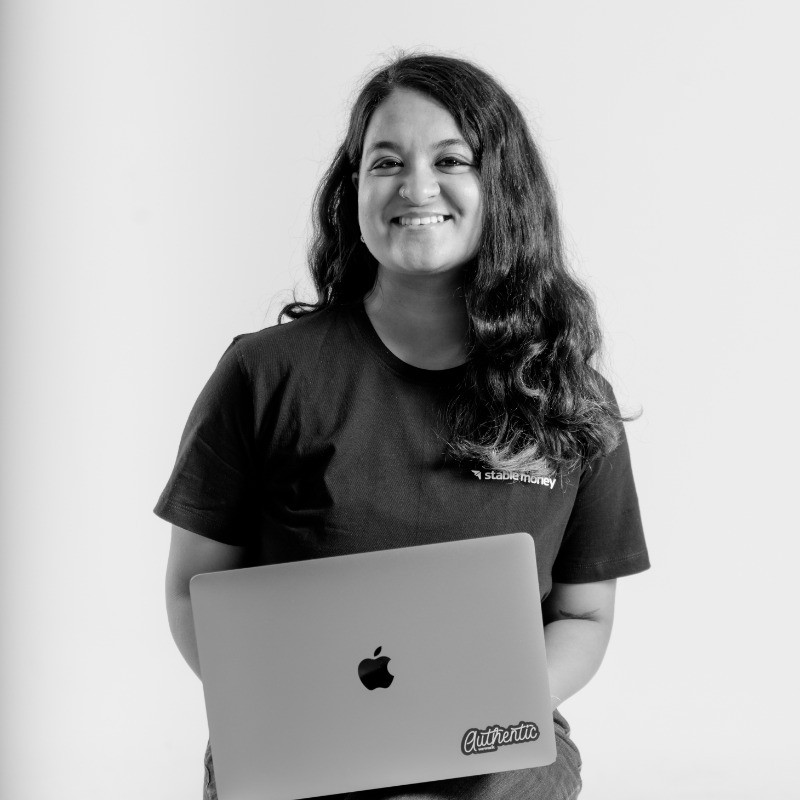
HC
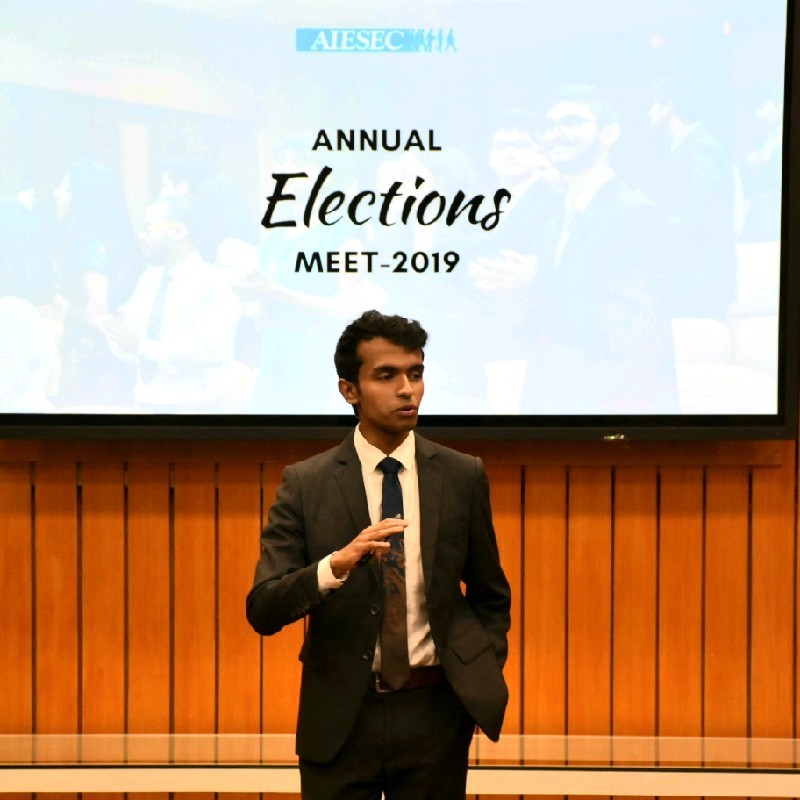
70+ people from across industries read our emails.
HC
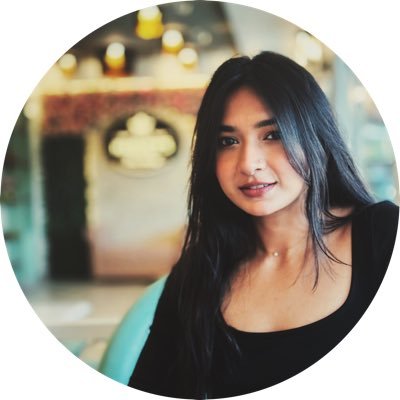
HC
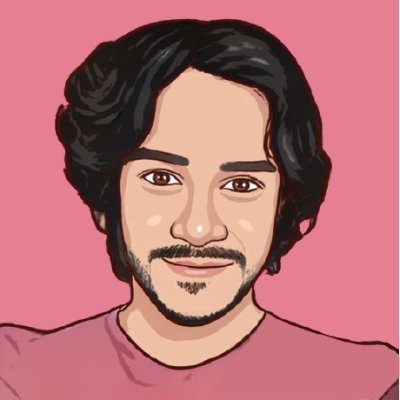
70+ people from across industries read our emails.
HC
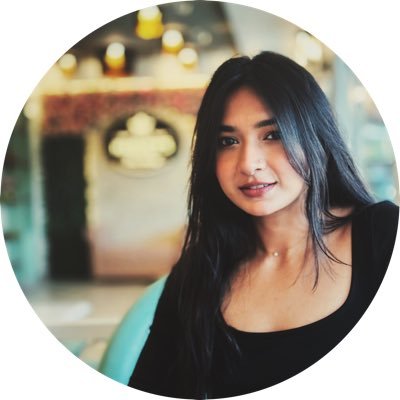
HC
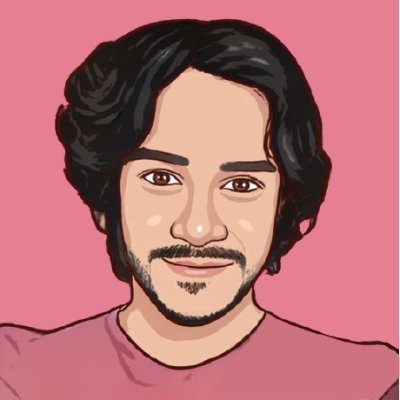
HC
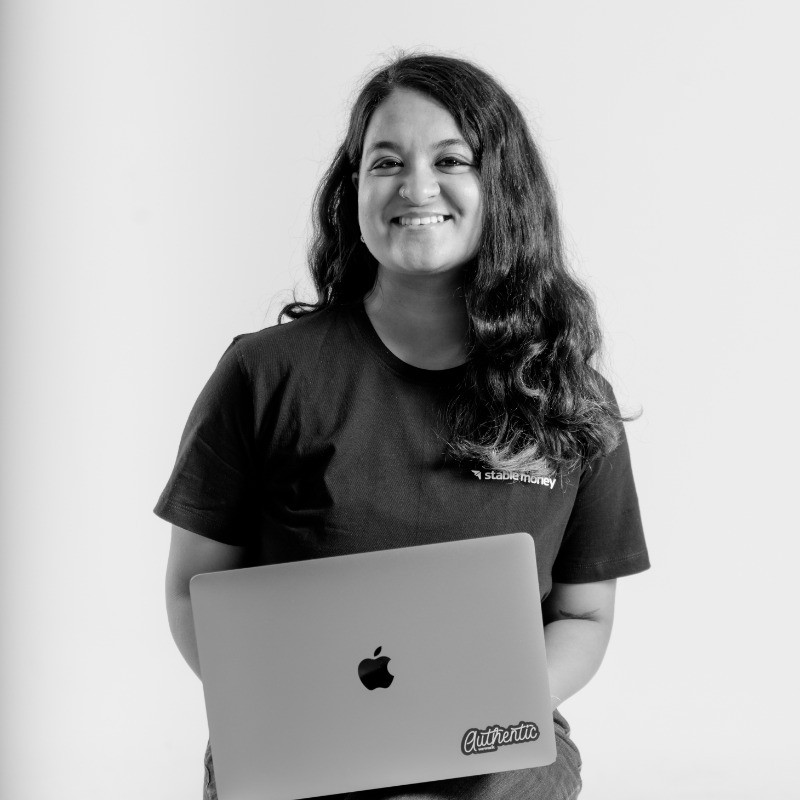
70+ people from across industries read our emails.
Bangalore, India / San Francisco, US
WorkHack Inc. 2023
Bangalore, India
San Francisco, US
WorkHack Inc. 2023
WorkHack Inc. 2023
Bangalore, India / San Francisco, US
WorkHack Inc. 2023
Bangalore, India / San Francisco, US